Thank you for visiting nature.com. You are using a browser version with limited support for CSS. To obtain the best experience, we recommend you use a more up to date browser (or turn off compatibility mode in Internet Explorer). In the meantime, to ensure continued support, we are displaying the site without styles and JavaScript.
- View all journals
- Explore content
- About the journal
- Publish with us
- Sign up for alerts
- Published: 29 May 2014
Points of significance

Designing comparative experiments
- Martin Krzywinski 1 &
- Naomi Altman 2
Nature Methods volume 11 , pages 597–598 ( 2014 ) Cite this article
48k Accesses
14 Citations
8 Altmetric
Metrics details
- Research data
- Statistical methods
Good experimental designs limit the impact of variability and reduce sample-size requirements.
You have full access to this article via your institution.
In a typical experiment, the effect of different conditions on a biological system is compared. Experimental design is used to identify data-collection schemes that achieve sensitivity and specificity requirements despite biological and technical variability, while keeping time and resource costs low. In the next series of columns we will use statistical concepts introduced so far and discuss design, analysis and reporting in common experimental scenarios.
In experimental design, the researcher-controlled independent variables whose effects are being studied (e.g., growth medium, drug and exposure to light) are called factors. A level is a subdivision of the factor and measures the type (if categorical) or amount (if continuous) of the factor. The goal of the design is to determine the effect and interplay of the factors on the response variable (e.g., cell size). An experiment that considers all combinations of N factors, each with n i levels, is a factorial design of type n 1 × n 2 × ... × n N . For example, a 3 × 4 design has two factors with three and four levels each and examines all 12 combinations of factor levels. We will review statistical methods in the context of a simple experiment to introduce concepts that apply to more complex designs.
Suppose that we wish to measure the cellular response to two different treatments, A and B, measured by fluorescence of an aliquot of cells. This is a single factor (treatment) design with three levels (untreated, A and B). We will assume that the fluorescence (in arbitrary units) of an aliquot of untreated cells has a normal distribution with μ = 10 and that real effect sizes of treatments A and B are d A = 0.6 and d B = 1 (A increases response by 6% to 10.6 and B by 10% to 11). To simulate variability owing to biological variation and measurement uncertainty (e.g., in the number of cells in an aliquot), we will use σ = 1 for the distributions. For all tests and calculations we use α = 0.05.
We start by assigning samples of cell aliquots to each level ( Fig. 1a ). To improve the precision (and power) in measuring the mean of the response, more than one aliquot is needed 1 . One sample will be a control (considered a level) to establish the baseline response, and capture biological and technical variability. The other two samples will be used to measure response to each treatment. Before we can carry out the experiment, we need to decide on the sample size.
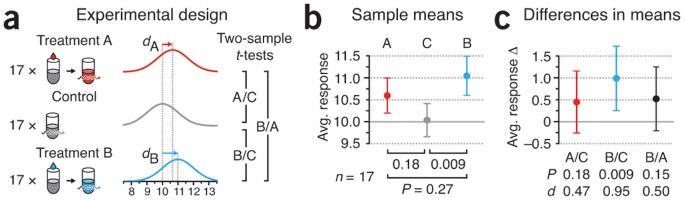
( a ) Two treated samples (A and B) with n = 17 are compared to a control (C) with n = 17 and to each other using two-sample t -tests. ( b ) Simulated means and P values for samples in a . Values are drawn from normal populations with σ = 1 and mean response of 10 (C), 10.6 (A) and 11 (B). ( c ) The preferred reporting method of results shown in b , illustrating difference in means with CIs, P values and effect size, d . All error bars show 95% CI.
We can fall back to our discussion about power 1 to suggest n . How large an effect size ( d ) do we wish to detect and at what sensitivity? Arbitrarily small effects can be detected with large enough sample size, but this makes for a very expensive experiment. We will need to balance our decision based on what we consider to be a biologically meaningful response and the resources at our disposal. If we are satisfied with an 80% chance (the lowest power we should accept) of detecting a 10% change in response, which corresponds to the real effect of treatment B ( d B = 1), the two-sample t -test requires n = 17. At this n value, the power to detect d A = 0.6 is 40%. Power calculations are easily computed with software; typically inputs are the difference in means (Δ μ ), standard deviation estimate ( σ ), α and the number of tails (we recommend always using two-tailed calculations).
Based on the design in Figure 1a , we show the simulated samples means and their 95% confidence interval (CI) in Figure 1b . The 95% CI captures the mean of the population 95% of the time; we recommend using it to report precision. Our results show a significant difference between B and control (referred to as B/C, P = 0.009) but not for A/C ( P = 0.18). Paradoxically, testing B/A does not return a significant outcome ( P = 0.15). Whenever we perform more than one test we should adjust the P values 2 . As we only have three tests, the adjusted B/C P value is still significant, P ′ = 3 P = 0.028. Although commonly used, the format used in Figure 1b is inappropriate for reporting our results: sample means, their uncertainty and P values alone do not present the full picture.
A more complete presentation of the results ( Fig. 1c ) combines the magnitude with uncertainty (as CI) in the difference in means. The effect size, d , defined as the difference in means in units of pooled standard deviation, expresses this combination of measurement and precision in a single value. Data in Figure 1c also explain better that the difference between a significant result (B/C, P = 0.009) and a nonsignificant result (A/C, P = 0.18) is not always significant (B/A, P = 0.15) 3 . Significance itself is a hard boundary at P = α , and two arbitrarily close results may straddle it. Thus, neither significance itself nor differences in significance status should ever be used to conclude anything about the magnitude of the underlying differences, which may be very small and not biologically relevant.
CIs explicitly show how close we are to making a positive inference and help assess the benefit of collecting more data. For example, the CIs of A/C and B/C closely overlap, which suggests that at our sample size we cannot reliably distinguish between the response to A and B ( Fig. 1c ). Furthermore, given that the CI of A/C just barely crosses zero, it is possible that A has a real effect that our test failed to detect. More information about our ability to detect an effect can be obtained from a post hoc power analysis, which assumes that the observed effect is the same as the real effect (normally unknown), and uses the observed difference in means and pooled variance. For A/C, the difference in means is 0.48 and the pooled s.d. ( s p ) = 1.03, which yields a post hoc power of 27%; we have little power to detect this difference. Other than increasing sample size, how could we improve our chances of detecting the effect of A?
Our ability to detect the effect of A is limited by variability in the difference between A and C, which has two random components. If we measure the same aliquot twice, we expect variability owing to technical variation inherent in our laboratory equipment and variability of the sample over time ( Fig. 2a ). This is called within-subject variation, σ wit . If we measure two different aliquots with the same factor level, we also expect biological variation, called between-subject variation, σ bet , in addition to the technical variation ( Fig. 2b ). Typically there is more biological than technical variability ( σ bet > σ wit ). In an unpaired design, the use of different aliquots adds both σ wit and σ bet to the measured difference ( Fig. 2c ). In a paired design, which uses the paired t -test 4 , the same aliquot is used and the impact of biological variation ( σ bet ) is mitigated ( Fig. 2c ). If differences in aliquots ( σ bet ) are appreciable, variance is markedly reduced (to within-subject variation) and the paired test has higher power.
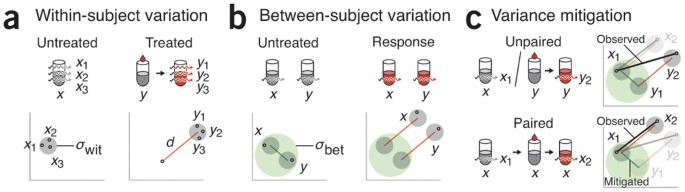
( a ) Limits of measurement and technical precision contribute to σ wit (gray circle) observed when the same aliquot is measured more than once. This variability is assumed to be the same in the untreated and treated condition, with effect d on aliquot x and y . ( b ) Biological variation gives rise to σ bet (green circle). ( c ) Paired design uses the same aliquot for both measurements, mitigating between-subject variation.
The link between σ bet and σ wit can be illustrated by an experiment to evaluate a weight-loss diet in which a control group eats normally and a treatment group follows the diet. A comparison of the mean weight after a month is confounded by the initial weights of the subjects in each group. If instead we focus on the change in weight, we remove much of the subject variability owing to the initial weight.
If we write the total variance as σ 2 = σ wit 2 + σ bet 2 , then the variance of the observed quantity in Figure 2c is 2 σ 2 for the unpaired design but 2 σ 2 (1 – ρ ) for the paired design, where ρ = σ bet 2 / σ 2 is the correlation coefficient (intraclass correlation). The relative difference is captured by ρ of two measurements on the same aliquot, which must be included because the measurements are no longer independent. If we ignore ρ in our analysis, we will overestimate the variance and obtain overly conservative P values and CIs. In the case where there is no additional variation between aliquots, there is no benefit to using the same aliquot: measurements on the same aliquot are uncorrelated ( ρ = 0) and variance of the paired test is the same as the variance of the unpaired. In contrast, if there is no variation in measurements on the same aliquot except for the treatment effect ( σ wit = 0), we have perfect correlation ( ρ = 1). Now, the difference measurement derived from the same aliquot removes all the noise; in fact, a single pair of aliquots suffices for an exact inference. Practically, both sources of variation are present, and it is their relative size—reflected in ρ —that determines the benefit of using the paired t-test.
We can see the improved sensitivity of the paired design ( Fig. 3a ) in decreased P values for the effects of A and B ( Fig. 3b versus Fig. 1b ). With the between-subject variance mitigated, we now detect an effect for A ( P = 0.013) and an even lower P value for B ( P = 0.0002) ( Fig. 3b ). Testing the difference between ΔA and ΔB requires the two-sample t -test because we are testing different aliquots, and this still does not produce a significant result ( P = 0.18). When reporting paired-test results, sample means ( Fig. 3b ) should never be shown; instead, the mean difference and confidence interval should be shown ( Fig. 3c ). The reason for this comes from our discussion above: the benefit of pairing comes from reduced variance because ρ > 0, something that cannot be gleaned from Figure 3b . We illustrate this in Figure 3c with two different sample simulations with same sample mean and variance but different correlation, achieved by changing the relative amount of σ bet 2 and σ wit 2 . When the component of biological variance is increased, ρ is increased from 0.5 to 0.8, total variance in difference in means drops and the test becomes more sensitive, reflected by the narrower CIs. We are now more certain that A has a real effect and have more reason to believe that the effects of A and B are different, evidenced by the lower P value for ΔB/ΔA from the two-sample t -test (0.06 versus 0.18; Fig. 3c ). As before, P values should be adjusted with multiple-test correction.
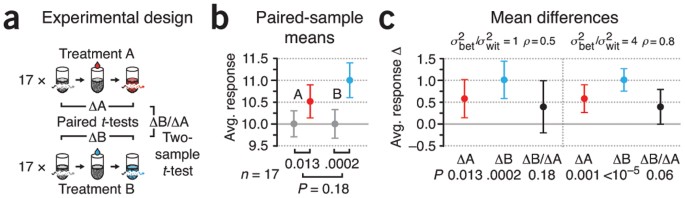
( a ) The same n = 17 sample is used to measure the difference between treatment and background (ΔA = A after − A before , ΔB = B after − B before ), analyzed with the paired t -test. Two-sample t -test is used to compare the difference between responses (ΔB versus ΔA). ( b ) Simulated sample means and P values for measurements and comparisons in a . ( c ) Mean difference, CIs and P values for two variance scenarios, σ bet 2 / σ wit 2 of 1 and 4, corresponding to ρ of 0.5 and 0.8. Total variance was fixed: σ bet 2 + σ wit 2 = 1. All error bars show 95% CI.
The paired design is a more efficient experiment. Fewer aliquots are needed: 34 instead of 51, although now 68 fluorescence measurements need to be taken instead of 51. If we assume σ wit = σ bet ( ρ = 0.5; Fig. 3c ), we can expect the paired design to have a power of 97%. This power increase is highly contingent on the value of ρ . If σ wit is appreciably larger than σ bet (i.e., ρ is small), the power of the paired test can be lower than for the two-sample variant. This is because total variance remains relatively unchanged (2 σ 2 (1 – ρ ) ≈ 2 σ 2 ) while the critical value of the test statistic can be markedly larger (particularly for small samples) because the number of degrees of freedom is now n – 1 instead of 2( n – 1). If the ratio of σ bet 2 to σ wit 2 is 1:4 ( ρ = 0.2), the paired test power drops from 97% to 86%.
To analyze experimental designs that have more than two levels, or additional factors, a method called analysis of variance is used. This generalizes the t -test for comparing three or more levels while maintaining better power than comparing all sets of two levels. Experiments with two or more levels will be our next topic.
Krzywinski, M.I. & Altman, N. Nat. Methods 10 , 1139–1140 (2013).
Article CAS Google Scholar
Krzywinski, M.I. & Altman, N. Nat. Methods 11 , 355–356 (2014).
Gelman, A. & Stern, H. Am. Stat. 60 , 328–331 (2006).
Article Google Scholar
Krzywinski, M.I. & Altman, N. Nat. Methods 11 , 215–216 (2014).
Download references
Author information
Authors and affiliations.
Martin Krzywinski is a staff scientist at Canada's Michael Smith Genome Sciences Centre.,
- Martin Krzywinski
Naomi Altman is a Professor of Statistics at The Pennsylvania State University.,
- Naomi Altman
You can also search for this author in PubMed Google Scholar
Ethics declarations
Competing interests.
The authors declare no competing financial interests.
Rights and permissions
Reprints and permissions
About this article
Cite this article.
Krzywinski, M., Altman, N. Designing comparative experiments. Nat Methods 11 , 597–598 (2014). https://doi.org/10.1038/nmeth.2974
Download citation
Published : 29 May 2014
Issue Date : June 2014
DOI : https://doi.org/10.1038/nmeth.2974
Share this article
Anyone you share the following link with will be able to read this content:
Sorry, a shareable link is not currently available for this article.
Provided by the Springer Nature SharedIt content-sharing initiative
This article is cited by
Sources of variation.
Nature Methods (2015)
ETD Outperforms CID and HCD in the Analysis of the Ubiquitylated Proteome
- Tanya R. Porras-Yakushi
- Michael J. Sweredoski
Journal of the American Society for Mass Spectrometry (2015)
Analysis of variance and blocking
Nature Methods (2014)
Nested designs
- Paul Blainey
Quick links
- Explore articles by subject
- Guide to authors
- Editorial policies
Sign up for the Nature Briefing newsletter — what matters in science, free to your inbox daily.

DOE Simplified, 3rd Edition by Mark J. Anderson, Patrick J. Whitcomb
Get full access to DOE Simplified, 3rd Edition and 60K+ other titles, with a free 10-day trial of O'Reilly.
There are also live events, courses curated by job role, and more.
Simple Comparative Experiments
Many of the most useful designs are extremely simple.
Sir Ronald Fisher
We now look at a method for making simple comparisons of two or more “treatments,” such as varying brands of toothpaste, and testing their efficacy for preventing cavities. Called the F-test , it is named after Sir Ronald Fisher, a geneticist who developed the technique for application to agricultural experiments. The F-test compares the variance among the treatment means versus the variance of individuals within the specific treatments. High values of F indicate that one (or more) of the means differs from another. This can be very valuable information when, for example, you must select from several suppliers or materials or levels ...
Get DOE Simplified, 3rd Edition now with the O’Reilly learning platform.
O’Reilly members experience books, live events, courses curated by job role, and more from O’Reilly and nearly 200 top publishers.
Don’t leave empty-handed
Get Mark Richards’s Software Architecture Patterns ebook to better understand how to design components—and how they should interact.
It’s yours, free.
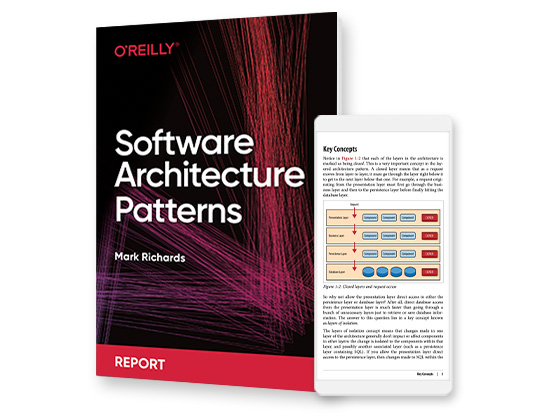
Check it out now on O’Reilly
Dive in for free with a 10-day trial of the O’Reilly learning platform—then explore all the other resources our members count on to build skills and solve problems every day.
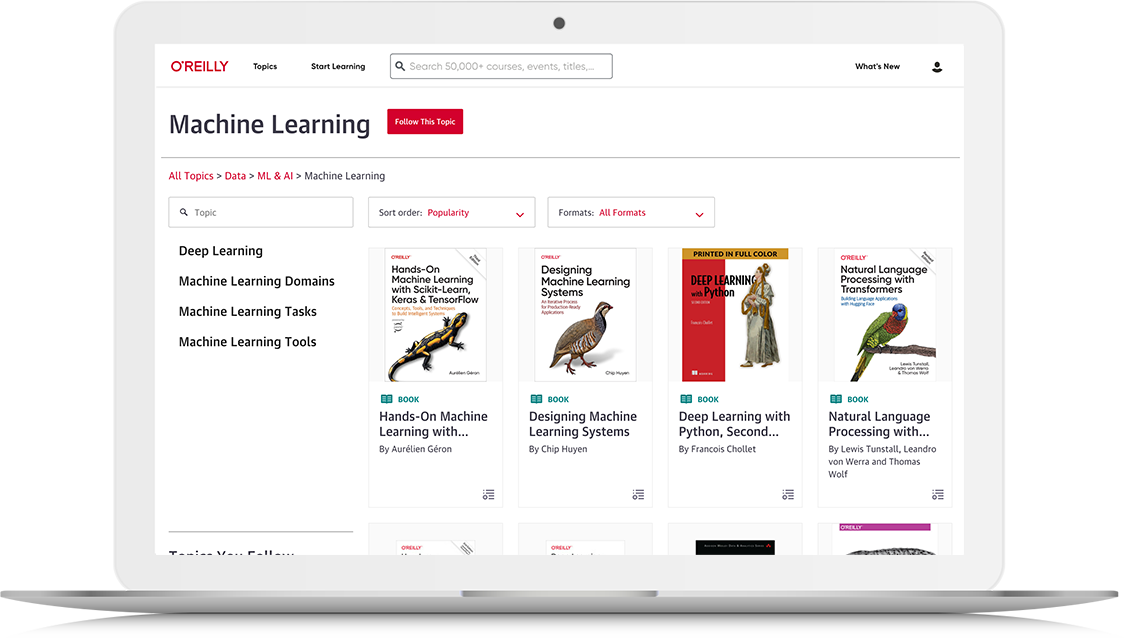
Sciencing_Icons_Science SCIENCE
Sciencing_icons_biology biology, sciencing_icons_cells cells, sciencing_icons_molecular molecular, sciencing_icons_microorganisms microorganisms, sciencing_icons_genetics genetics, sciencing_icons_human body human body, sciencing_icons_ecology ecology, sciencing_icons_chemistry chemistry, sciencing_icons_atomic & molecular structure atomic & molecular structure, sciencing_icons_bonds bonds, sciencing_icons_reactions reactions, sciencing_icons_stoichiometry stoichiometry, sciencing_icons_solutions solutions, sciencing_icons_acids & bases acids & bases, sciencing_icons_thermodynamics thermodynamics, sciencing_icons_organic chemistry organic chemistry, sciencing_icons_physics physics, sciencing_icons_fundamentals-physics fundamentals, sciencing_icons_electronics electronics, sciencing_icons_waves waves, sciencing_icons_energy energy, sciencing_icons_fluid fluid, sciencing_icons_astronomy astronomy, sciencing_icons_geology geology, sciencing_icons_fundamentals-geology fundamentals, sciencing_icons_minerals & rocks minerals & rocks, sciencing_icons_earth scructure earth structure, sciencing_icons_fossils fossils, sciencing_icons_natural disasters natural disasters, sciencing_icons_nature nature, sciencing_icons_ecosystems ecosystems, sciencing_icons_environment environment, sciencing_icons_insects insects, sciencing_icons_plants & mushrooms plants & mushrooms, sciencing_icons_animals animals, sciencing_icons_math math, sciencing_icons_arithmetic arithmetic, sciencing_icons_addition & subtraction addition & subtraction, sciencing_icons_multiplication & division multiplication & division, sciencing_icons_decimals decimals, sciencing_icons_fractions fractions, sciencing_icons_conversions conversions, sciencing_icons_algebra algebra, sciencing_icons_working with units working with units, sciencing_icons_equations & expressions equations & expressions, sciencing_icons_ratios & proportions ratios & proportions, sciencing_icons_inequalities inequalities, sciencing_icons_exponents & logarithms exponents & logarithms, sciencing_icons_factorization factorization, sciencing_icons_functions functions, sciencing_icons_linear equations linear equations, sciencing_icons_graphs graphs, sciencing_icons_quadratics quadratics, sciencing_icons_polynomials polynomials, sciencing_icons_geometry geometry, sciencing_icons_fundamentals-geometry fundamentals, sciencing_icons_cartesian cartesian, sciencing_icons_circles circles, sciencing_icons_solids solids, sciencing_icons_trigonometry trigonometry, sciencing_icons_probability-statistics probability & statistics, sciencing_icons_mean-median-mode mean/median/mode, sciencing_icons_independent-dependent variables independent/dependent variables, sciencing_icons_deviation deviation, sciencing_icons_correlation correlation, sciencing_icons_sampling sampling, sciencing_icons_distributions distributions, sciencing_icons_probability probability, sciencing_icons_calculus calculus, sciencing_icons_differentiation-integration differentiation/integration, sciencing_icons_application application, sciencing_icons_projects projects, sciencing_icons_news news.
- Share Tweet Email Print
- Home ⋅
- Math ⋅
- Algebra ⋅
What Are Comparative Experiments?
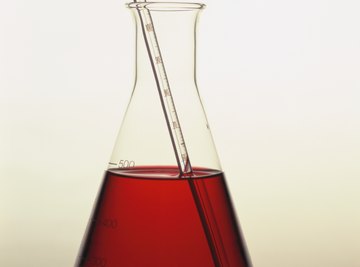
Definitions of Control, Constant, Independent and Dependent Variables ...
Many students of science understand the basic idea of the comparative experiment because the name "comparative experiment" mostly explains itself. Students would be correct in defining a comparative experiment as one that compares the effects of two treatments. However, like most anything in science, the comparative experiment has advantages and disadvantages. Students must understand these aspects at a deep level before fully understanding the comparative experiment itself.
Asking the Right Question
According to Penn State, a comparative experiment starts with a question or hypothesis that asks how two or more treatments affect some response. When a scientist wants to know the difference between the effects of treatment A and treatment B on dependent variable C, he will run an experiment in which all of the conditions are the same except for one: the treatment -- A or B -- given to the subject. After receiving the results of the experiment, the scientist can then compare the difference in the dependent variable C for each treatment, concluding either that one treatment is more effective than the other or that both treatments have about the same effectiveness.
The keys to a comparative treatment are control and randomization. Control refers to holding constant all of the other variables that could affect the outcome. For example, a comparative experiment comparing the effects of two diets of different nutritional value on the growth of mice should ensure that the mice eat at the same time, regardless of which diet they are assigned to eat. Randomization refers to randomly assigning the experiment’s subjects, such as mice, to the two or more treatment groups. This randomization allows for valid conclusions and statistical analysis across treatments.
The Advantage
To many students of science, the comparative experiment is a time-saver. Standard, non-comparative experiments use a “control,” which refers to a group of subjects that receive no treatment or a placebo. Scientists engaging in non-comparative experiments in their research would need to run the experiment twice, once with each treatment. For many experiments, however, running just one experiment can be a remarkable expense in both time and money. Thus, a comparative experiment can save a scientist the trouble of having to allocate resources to a second run with a different treatment.
Comparative treatments do not need to include a control, which can be a problem if both treatments yield similar results. For example, if two different injections lead to a similar amount of increased activity in mice, a scientist might be tempted to conclude that both of the injected drugs are effective at inciting activity. The truth is that without a control, the scientist cannot make such a conclusion, as other factors might be influencing the enhanced activity of the mice, such as anxiety from the injection or being handled by the scientists. A comparative experiment is generally limited to conclude the relative effectiveness of one treatment compared to the other.
Related Articles
Definitions of control, constant, independent and dependent..., why should you only test for one variable at a time..., what is a positive control in microbiology, how to calculate significance, differences between within & between subjects design, what is a constant in the scientific method, what is the difference between a control & a controlled..., what is a standardized variable in biology, experiments with hand sanitizer, five characteristics of the scientific method, can a science experiment have two manipulated variables, bottoms up: drinking beer can increase creativity, experiments on which mouthwash kills bacteria, distinguishing between descriptive & causal studies, how to determine the sample size in a quantitative..., what is a constant in a science fair project, what is an independent variable in quantitative research, how to minimize a sampling error, how to use the pearson correlation coefficient.
- Experiment Design and Statistical Methods for Behavioural and Social Research; David Boniface
- Encyclopedia of Research Design; Neil Salkind
- East Tennessee State University: Producing Data: Experiments
About the Author
Having obtained a Master of Science in psychology in East Asia, Damon Verial has been applying his knowledge to related topics since 2010. Having written professionally since 2001, he has been featured in financial publications such as SafeHaven and the McMillian Portfolio. He also runs a financial newsletter at Stock Barometer.
Photo Credits
Stockbyte/Stockbyte/Getty Images
Find Your Next Great Science Fair Project! GO

Snapsolve any problem by taking a picture. Try it in the Numerade app?
Design and Analysis of Experiments
Douglas c. montgomery, simple comparative experiments - all with video answers.
Chapter Questions
The breaking strength of a fiber is required to be at least 150 psi. Past experience has indicated that the standard deviation of breaking strength is $\sigma=3$ psi. A random sample of four specimens is tested, and the results are $y_{1}=145, y_{2}=153, y_{3}=150$, and $y_{4}=$ 147. (a) State the hypotheses that you think should be tested in this experiment. (b) Test these hypotheses using $\alpha=0.05 .$ What are your conclusions? (c) Find the $P$-value for the test in part (b). (d) Construct a 95 percent confidence interval on the mean breaking strength.
The viscosity of a liquid detergent is supposed to average 800 centistokes at $25^{\circ} \mathrm{C} . \mathrm{A}$ random sample of 16 batches of detergent is collected, and the average viscosity is $812 .$ Suppose we know that the standard deviation of viscosity is $\sigma=25$ centistokes. (a) State the hypotheses that should be tested. (b) Test these hypotheses using $\alpha=0.05 .$ What are your conclusions? (c) What is the $P$-value for the test? (d) Find a 95 percent confidence interval on the mean.
The diameters of steel shafts produced by a certain manufacturing process should have a mean diameter of $0.255$ inches. The diameter is known to have a standard deviation of $\sigma=0.0001$ inch. A random sample of 10 shafts has an average diameter of $0.2545$ inch. (a) Set up appropriate hypotheses on the mean $\mu$. (b) Test these hypotheses using $\alpha=0.05 .$ What are your conclusions? (c) Find the $P$-value for this test. (d) Construct a 95 percent confidence interval on the mean shaft diameter.
A normally distributed random variable has an unknown mean $\mu$ and a known variance $\sigma^{2}=9$. Find the sample size required to construct a 95 percent confidence interval on the mean that has total width of $10 .$
The sheif life of a carbonated beverage is of interest. Ten bottles are randomly selected and tested, and the following results are obtained: $$ \begin{array}{lr} \hline \multicolumn{2}{c}{\text { Days }} \\ \hline 108 & 138 \\ 124 & 163 \\ 124 & 159 \\ 106 & 134 \\ 115 & 139 \\ \hline \end{array} $$ We would like to demonstrate that the mean shelf life exceeds 120 days. Set up appropriate hypotheses for investigating this claim. Test these hypotheses using $\alpha=0.01$. What are your conclusions? (c) Find the $P$-value for the test in part (b). (d) Construct a 99 percent confidence interval on the mean shelf life.
Consider the shelf life data in Problem 2-5. Can shelf life be described or modeled adequately by a normal distribution? What effect would violation of this assumption have on the test procedure you used in solving Problem 2-5?
The time to repair an electronic instrument is a normally distributed random variable measured in hours. The repair times for 16 such instruments chosen at random are as follows: $$ \begin{array}{lccc} \hline \multicolumn{4}{c}{\text { Hours }} \\ \hline 159 & 280 & 101 & 212 \\ 224 & 379 & 179 & 264 \\ 222 & 362 & 168 & 250 \\ 149 & 260 & 485 & 170 \\ \hline \end{array} $$ (a) You wish to know if the mean repair time exceeds 225 hours. Set up appropriate hypotheses for investigating this issue. (b) Test the hypotheses you formulated in part (a). What are your conclusions? Use $\alpha=0.05$ (c) Find the $P$-value for the test. (d) Construct a 95 percent confidence interval on mean repair time.
Reconsider the repair time data in Problem 2-7. Can repair time, in your opinion, be adequately modeled by a normal distribution?
Two machines are used for filling plastic bottles with a net volume of $16.0$ ounces. The filling processes can be assumed to be normal, with standard deviations of $\sigma_{1}=0.015$ and $\sigma_{2}=0.018$. The quality engineering department suspects that both machines fill to the same net volume, whether or not this volume is $16.0$ ounces. An experiment is performed by taking a random sample from the output of each machine. $$ \begin{array}{llll} \hline \multicolumn{2}{c}{\text { Machine 1 }} & \multicolumn{2}{c}{\text { Machine 2 }} \\ \hline 16.03 & 16.01 & 16.02 & 16.03 \\ 16.04 & 15.96 & 15.97 & 16.04 \\ 16.05 & 15.98 & 15.96 & 16.02 \\ 16.05 & 16.02 & 16.01 & 16.01 \\ 16.02 & 15.99 & 15.99 & 16.00 \\ \hline \end{array} $$ (a) State the hypotheses that should be tested in this experiment. (b) Test these hypotheses using $\alpha=0.05$. What are your conclusions? (c) Find the $P$-value for this test. (d) Find a 95 percent confidence interval on the difference in mean fill volume for the two machines.
Two types of plastic are suitable for use by an electronic calculator manufacturer. The breaking strength of this plastic is important. It is known that $\sigma_{1}=\sigma_{2}=1.0$ psi. From random samples of $n_{1}=10$ and $n_{y}=12$ we obtain $\bar{y}_{1}=162.5$ and $\bar{y}_{2}=155.0$. The company will not adopt plastic 1 unless its breaking strength exceeds that of plastic 2 by at least 10 psi. Based on the sample information, should they use plastic $1 ?$ In answering this question, set up and test appropriate hypotheses using $\alpha=0.01$. Construct a 99 percent confidence interval on the true mean difference in breaking strength.
The following are the buming times of chemical flares of two different formulations. The design engineers are interested in both the mean and variance of the burning times. \begin{array}{lllr} \hline \multicolumn{2}{l}{\text { Type 1 }} & \multicolumn{2}{c}{\text { Type 2 }} \\ \hline 65 & 82 & 64 & 56 \\ (a) Test the hypothesis that the two variances are equal. Use $\alpha=0.05$. (b) Using the results of (a), test the hypothesis that the mean burning times are equal. Use $\alpha=0.05$. What is the $P$-value for this test? (c) Discuss the role of the normality assumption in this problem. Check the assumption of normality for both types of flares. 81 & 67 & 71 & 69 \\ 57 & 59 & 83 & 74 \\ 66 & 75 & 59 & 82 \\ 82 & 70 & 65 & 79 \\ \hline \end{array}
An article in Solid State Technology, "Orthogonal Design for Process Optimization and Its Application to Plasma Etching" by G. Z. Yin and D. W. Jillie (May, 1987 ) describes an experiment to determine the effect of the $\mathrm{C}_{2} \mathrm{~F}_{6}$ flow rate on the uniformity of the etch on a silicon wafer used in integrated circuit manufacturing. Data for two flow rates are as follows: \begin{array}{ccccccc} \hline \multirow{3}{\mathrm{C}_{2}\mathrm{F}_{6}\text{Flow}}{{\text { (SCCM) }}} & \multicolumn{5}{c}{\text { Uniformity Observation }} \\ \cline { 1 - 7 } & 1 & 2 & 3 & 4 & 5 & 6 \\ \hline 125 & 2.7 & 4.6 & 2.6 & 3.0 & 3.2 & 3.8 \\ 200 & 4.6 & 3.4 & 2.9 & 3.5 & 4.1 & 5.1 \\ \hline \end{array} (a) Does the $\mathrm{C}_{2} \mathrm{~F}_{6}$ flow rate affect average etch uniformity? Use $\alpha=0.05$. (b) What is the $P$-value for the test in part (a)? (c) Does the $\mathrm{C}_{2} \mathrm{~F}_{6}$ flow rate affect the wafer-to-wafer variability in etch uniformity? Use $\alpha=0.05$. (d) Draw box plots to assist in the interpretation of the data from this experiment.
A new filtering device is installed in a chemical unit. Before its installation, a random sample yielded the following information about the percentage of impurity: $\bar{y}_{1}=12.5$, $S_{1}^{2}=101.17$, and $n_{1}=8$. Afler installation, a random sample yielded $\bar{y}_{2}=10.2$. $S_{2}^{2}=94.73, n_{2}=9$ (a) Can you conclude that the two variances are equal? Use $\alpha=0.05$. (b) Has the filtering device reduced the percentage of impurity significantly? Use $\alpha=0.05$
Twenty observations on etch uniformity on silicon wafers are taken during a qualification experiment for a plasma etcher. The data are as follows: $$ \begin{array}{lllll} 5.34 & 6.65 & 4.76 & 5.98 & 7.25 \\ 6.00 & 7.55 & 5.54 & 5.62 & 6.21 \\ 5.97 & 7.35 & 5.44 & 4.39 & 4.98 \\ 5.25 & 6.35 & 4.61 & 6.00 & 5.32 \end{array} $$ (a) Construct a 95 percent confidence interval estimate of $\sigma^{2}$. (b) Test the hypothesis that $\sigma^{2}=1.0$. Use $\alpha=0.05$. What are your conclusions? (c) Discuss the normality assumption and its role in this problem. (d) Check nomality by constructing a normal probability plot. What are your conclusions?
The diameter of a ball bearing was measured by 12 inspectors, each using two different kinds of calipers. The results were \begin{array}{ccc} \hline \text { Inspector } & \text { Caliper 1 } & \text { Caliper 2 } \\ \hline 1 & 0.265 & 0.264 \\ 2 & 0.265 & 0.265 \\ 3 & 0.266 & 0.264 \\ 4 & 0.267 & 0.266 \\ 5 & 0.267 & 0.267 \\ 6 & 0.265 & 0.268 \\ 7 & 0.267 & 0.264 \\ 8 & 0.267 & 0.265 \\ 9 & 0.265 & 0.265 \\ 10 & 0.268 & 0.267 \\ 11 & 0.268 & 0.268 \\ 12 & 0.265 & 0.269 \\ \hline \end{array} (a) Is there a significant difference between the means of the population of measurements from which the two samples were selected? Use $\alpha=0.05$. (b) Find the $P$-value for the test in part (a). (c) Construct a 95 percent confidence interval on the difference in mean diameter measurements for the two types of calipers.
An article in the Journal of Strain Analysis (vol. 18, no. 2,1983$)$ compares several procedures for predicting the shear strength for steel plate girders. Data for nine girders in the form of the ratio of predicted to observed load for two of these procedures, the Karlsruhe and Lehigh methods, are as follows: \begin{array}{lcc} \hline \text { Girder } & \text { Karlsruhe Method } & \text { Lehigh Method } \\ \hline \mathrm{S} 1 / 1 & 1.186 & 1.061 \\ \mathrm{~S} 2 / 1 & 1.151 & 0.992 \\ \mathrm{~S} 3 / 1 & 1.322 & 1.063 \\ S 4 / 1 & 1.339 & 1.062 \\ \mathrm{~S} 5 / 1 & 1.200 & 1.065 \\ \mathrm{~S} 2 / 1 & 1.402 & 1.178 \\ \mathrm{~S} 2 / 2 & 1.365 & 1.037 \\ \$ 2 / 3 & 1.537 & 1.086 \\ \$ 2 / 4 & 1.559 & 1.052 \\ \hline \end{array} (a) Is there any evidence to support a claim that there is a difference in mean performance between the two methods? Use $\alpha=0.05$. (b) What is the $P$-value for the test in part (a)? (c) Construct a 95 percent confidence interval for the difference in mean predicted to observed load. (d) Investigate the normality assumption for both samples. (e) Investigate the normality assumption for the difference in ratios for the two methods. (f) Discuss the role of the normality assumption in the paired t-test.
The deflection temperature under load for two different formulations of ABS plastic pipe is being studied. Two samples of 12 observations each are prepared using each formulation and the deflection temperatures (in ${ }^{\circ} \mathrm{F}$ ) are reported below: \begin{array}{lcllcr} \hline \multicolumn{3}{c}{\text { Formulation 1 }} & \multicolumn{3}{c}{\text { Formulation 2 }} \\ \hline 206 & 193 & 192 & 177 & 176 & 198 \\ 188 & 207 & 210 & 197 & 185 & 188 \\ 205 & 185 & 194 & 206 & 200 & 189 \\ 187 & 189 & 178 & 201 & 197 & 203 \\ \hline \end{array} (a) Construct normal probability plots for both samples. Do these plots support assumptions of normality and equal variance for both samples? (b) Does the data support the claim that the mean deflection temperature under load for formulation 1 exceeds that of formulation $2 ?$ Use $\alpha=0.05$. (c) What is the $P$-value for the test in part (a)?
Refer to the data in Problem 2-17. Do the data support a claim that the mean deflection temperature under load for formulation 1 exceeds that of formulation 2 by at least $3^{\circ} \mathrm{F}$ ?
In semiconductor manufacturing wet chemical etching is often used to remove silicon from the backs of wafers prior to metalization. The etch rate is an important characteristic of this process. Two different etching solutions are being evaluated. Eight randomly selected wafers have been etched in each solution and the observed etch rates (in mils/min) are shown below. \begin{array}{rrrr} \hline \multicolumn{2}{c}{\text { Solution 1 }} & \multicolumn{2}{c}{\text { Solution 2 }} \\ \hline 9.9 & 10.6 & 10.2 & 10.6 \\ 9.4 & 10.3 & 10.0 & 10.2 \\ 10.0 & 9.3 & 10.7 & 10.4 \\ 10.3 & 9.8 & 10.5 & 10.3 \\ \hline \end{array} (a) Do the data indicate that the claim that both solutions have the same mean etch rate is valid? Use $\alpha=0.05$ and assume equal variances. (b) Find a 95 percent confidence interval on the difference in mean etch rates. (c) Use normal probability plots to investigate the adequacy of the assumptions of normality and eaual variances.
Two popular pain medications are being compared on the basis of the speed of absorption by the body. Specifically, tablet 1 is claimed to be absorbed twice as fast as tablet 2 . Assume that $\sigma_{1}^{2}$ and $\sigma_{2}^{2}$ are known. Develop a test statistic for $$ \begin{aligned} &H_{0}: 2 \mu_{1}=\mu_{2} \\ &H_{1}: 2 \mu_{1} \neq \mu_{2} \end{aligned} $$
Suppose we are testing $$ \begin{aligned} &H_{0}: \mu_{1}=\mu_{2} \\ &H_{1}: \mu_{1} \neq \mu_{2} \end{aligned} $$ where $\sigma_{1}^{2}$ and $\sigma_{2}^{2}$ are known. Our sampling resources are constrained such that $n_{1}+n_{2}=N .$ How should we allocate the $N$ observations between the two populations to obtain the most powerful test?
Develop Equation $2-46$ for a $100(1-\alpha)$ percent confidence interval for the variance of a normal distribution.
Develop Equation $2-50$ for a $100(1-\alpha)$ percent confidence interval for the ratio $\sigma_{7}^{2} / \sigma_{2}^{2}$ where $\sigma_{1}^{2}$ and $\sigma_{2}^{2}$ are the variances of two normal distributions.
Develop an equation for finding a $100(1-\alpha)$ percent confidence interval on the difference in the means of two normal distributions where $\sigma_{1}^{2} \neq \sigma_{2}^{2}$. Apply your equation to the portland cement experiment data, and find a 95 pecent confidence interval.
Construct a data set for which the paired $t$-test statistic is very large, but for which the usual two-sample or pooled $t$-test statistic is small. In general, describe how you created the data. Does this give you any insight regarding how the paired $t$-test works?
- Search Menu
Sign in through your institution
- Browse content in Arts and Humanities
- Browse content in Archaeology
- Anglo-Saxon and Medieval Archaeology
- Archaeological Methodology and Techniques
- Archaeology by Region
- Archaeology of Religion
- Archaeology of Trade and Exchange
- Biblical Archaeology
- Contemporary and Public Archaeology
- Environmental Archaeology
- Historical Archaeology
- History and Theory of Archaeology
- Industrial Archaeology
- Landscape Archaeology
- Mortuary Archaeology
- Prehistoric Archaeology
- Underwater Archaeology
- Zooarchaeology
- Browse content in Architecture
- Architectural Structure and Design
- History of Architecture
- Residential and Domestic Buildings
- Theory of Architecture
- Browse content in Art
- Art Subjects and Themes
- History of Art
- Industrial and Commercial Art
- Theory of Art
- Biographical Studies
- Byzantine Studies
- Browse content in Classical Studies
- Classical History
- Classical Philosophy
- Classical Mythology
- Classical Numismatics
- Classical Literature
- Classical Reception
- Classical Art and Architecture
- Classical Oratory and Rhetoric
- Greek and Roman Epigraphy
- Greek and Roman Law
- Greek and Roman Archaeology
- Greek and Roman Papyrology
- Late Antiquity
- Religion in the Ancient World
- Social History
- Digital Humanities
- Browse content in History
- Colonialism and Imperialism
- Diplomatic History
- Environmental History
- Genealogy, Heraldry, Names, and Honours
- Genocide and Ethnic Cleansing
- Historical Geography
- History by Period
- History of Agriculture
- History of Education
- History of Emotions
- History of Gender and Sexuality
- Industrial History
- Intellectual History
- International History
- Labour History
- Legal and Constitutional History
- Local and Family History
- Maritime History
- Military History
- National Liberation and Post-Colonialism
- Oral History
- Political History
- Public History
- Regional and National History
- Revolutions and Rebellions
- Slavery and Abolition of Slavery
- Social and Cultural History
- Theory, Methods, and Historiography
- Urban History
- World History
- Browse content in Language Teaching and Learning
- Language Learning (Specific Skills)
- Language Teaching Theory and Methods
- Browse content in Linguistics
- Applied Linguistics
- Cognitive Linguistics
- Computational Linguistics
- Forensic Linguistics
- Grammar, Syntax and Morphology
- Historical and Diachronic Linguistics
- History of English
- Language Acquisition
- Language Variation
- Language Families
- Language Evolution
- Language Reference
- Lexicography
- Linguistic Theories
- Linguistic Typology
- Linguistic Anthropology
- Phonetics and Phonology
- Psycholinguistics
- Sociolinguistics
- Translation and Interpretation
- Writing Systems
- Browse content in Literature
- Bibliography
- Children's Literature Studies
- Literary Studies (Asian)
- Literary Studies (European)
- Literary Studies (Eco-criticism)
- Literary Studies (Modernism)
- Literary Studies (Romanticism)
- Literary Studies (American)
- Literary Studies - World
- Literary Studies (1500 to 1800)
- Literary Studies (19th Century)
- Literary Studies (20th Century onwards)
- Literary Studies (African American Literature)
- Literary Studies (British and Irish)
- Literary Studies (Early and Medieval)
- Literary Studies (Fiction, Novelists, and Prose Writers)
- Literary Studies (Gender Studies)
- Literary Studies (Graphic Novels)
- Literary Studies (History of the Book)
- Literary Studies (Plays and Playwrights)
- Literary Studies (Poetry and Poets)
- Literary Studies (Postcolonial Literature)
- Literary Studies (Queer Studies)
- Literary Studies (Science Fiction)
- Literary Studies (Travel Literature)
- Literary Studies (War Literature)
- Literary Studies (Women's Writing)
- Literary Theory and Cultural Studies
- Mythology and Folklore
- Shakespeare Studies and Criticism
- Browse content in Media Studies
- Browse content in Music
- Applied Music
- Dance and Music
- Ethics in Music
- Ethnomusicology
- Gender and Sexuality in Music
- Medicine and Music
- Music Cultures
- Music and Religion
- Music and Culture
- Music and Media
- Music Education and Pedagogy
- Music Theory and Analysis
- Musical Scores, Lyrics, and Libretti
- Musical Structures, Styles, and Techniques
- Musicology and Music History
- Performance Practice and Studies
- Race and Ethnicity in Music
- Sound Studies
- Browse content in Performing Arts
- Browse content in Philosophy
- Aesthetics and Philosophy of Art
- Epistemology
- Feminist Philosophy
- History of Western Philosophy
- Metaphysics
- Moral Philosophy
- Non-Western Philosophy
- Philosophy of Science
- Philosophy of Action
- Philosophy of Law
- Philosophy of Religion
- Philosophy of Language
- Philosophy of Mind
- Philosophy of Perception
- Philosophy of Mathematics and Logic
- Practical Ethics
- Social and Political Philosophy
- Browse content in Religion
- Biblical Studies
- Christianity
- East Asian Religions
- History of Religion
- Judaism and Jewish Studies
- Qumran Studies
- Religion and Education
- Religion and Health
- Religion and Politics
- Religion and Science
- Religion and Law
- Religion and Art, Literature, and Music
- Religious Studies
- Browse content in Society and Culture
- Cookery, Food, and Drink
- Cultural Studies
- Customs and Traditions
- Ethical Issues and Debates
- Hobbies, Games, Arts and Crafts
- Natural world, Country Life, and Pets
- Popular Beliefs and Controversial Knowledge
- Sports and Outdoor Recreation
- Technology and Society
- Travel and Holiday
- Visual Culture
- Browse content in Law
- Arbitration
- Browse content in Company and Commercial Law
- Commercial Law
- Company Law
- Browse content in Comparative Law
- Systems of Law
- Competition Law
- Browse content in Constitutional and Administrative Law
- Government Powers
- Judicial Review
- Local Government Law
- Military and Defence Law
- Parliamentary and Legislative Practice
- Construction Law
- Contract Law
- Browse content in Criminal Law
- Criminal Procedure
- Criminal Evidence Law
- Sentencing and Punishment
- Employment and Labour Law
- Environment and Energy Law
- Browse content in Financial Law
- Banking Law
- Insolvency Law
- History of Law
- Human Rights and Immigration
- Intellectual Property Law
- Browse content in International Law
- Private International Law and Conflict of Laws
- Public International Law
- IT and Communications Law
- Jurisprudence and Philosophy of Law
- Law and Politics
- Law and Society
- Browse content in Legal System and Practice
- Courts and Procedure
- Legal Skills and Practice
- Legal System - Costs and Funding
- Primary Sources of Law
- Regulation of Legal Profession
- Medical and Healthcare Law
- Browse content in Policing
- Criminal Investigation and Detection
- Police and Security Services
- Police Procedure and Law
- Police Regional Planning
- Browse content in Property Law
- Personal Property Law
- Restitution
- Study and Revision
- Terrorism and National Security Law
- Browse content in Trusts Law
- Wills and Probate or Succession
- Browse content in Medicine and Health
- Browse content in Allied Health Professions
- Arts Therapies
- Clinical Science
- Dietetics and Nutrition
- Occupational Therapy
- Operating Department Practice
- Physiotherapy
- Radiography
- Speech and Language Therapy
- Browse content in Anaesthetics
- General Anaesthesia
- Browse content in Clinical Medicine
- Acute Medicine
- Cardiovascular Medicine
- Clinical Genetics
- Clinical Pharmacology and Therapeutics
- Dermatology
- Endocrinology and Diabetes
- Gastroenterology
- Genito-urinary Medicine
- Geriatric Medicine
- Infectious Diseases
- Medical Oncology
- Medical Toxicology
- Pain Medicine
- Palliative Medicine
- Rehabilitation Medicine
- Respiratory Medicine and Pulmonology
- Rheumatology
- Sleep Medicine
- Sports and Exercise Medicine
- Clinical Neuroscience
- Community Medical Services
- Critical Care
- Emergency Medicine
- Forensic Medicine
- Haematology
- History of Medicine
- Browse content in Medical Dentistry
- Oral and Maxillofacial Surgery
- Paediatric Dentistry
- Restorative Dentistry and Orthodontics
- Surgical Dentistry
- Medical Ethics
- Browse content in Medical Skills
- Clinical Skills
- Communication Skills
- Nursing Skills
- Surgical Skills
- Medical Statistics and Methodology
- Browse content in Neurology
- Clinical Neurophysiology
- Neuropathology
- Nursing Studies
- Browse content in Obstetrics and Gynaecology
- Gynaecology
- Occupational Medicine
- Ophthalmology
- Otolaryngology (ENT)
- Browse content in Paediatrics
- Neonatology
- Browse content in Pathology
- Chemical Pathology
- Clinical Cytogenetics and Molecular Genetics
- Histopathology
- Medical Microbiology and Virology
- Patient Education and Information
- Browse content in Pharmacology
- Psychopharmacology
- Browse content in Popular Health
- Caring for Others
- Complementary and Alternative Medicine
- Self-help and Personal Development
- Browse content in Preclinical Medicine
- Cell Biology
- Molecular Biology and Genetics
- Reproduction, Growth and Development
- Primary Care
- Professional Development in Medicine
- Browse content in Psychiatry
- Addiction Medicine
- Child and Adolescent Psychiatry
- Forensic Psychiatry
- Learning Disabilities
- Old Age Psychiatry
- Psychotherapy
- Browse content in Public Health and Epidemiology
- Epidemiology
- Public Health
- Browse content in Radiology
- Clinical Radiology
- Interventional Radiology
- Nuclear Medicine
- Radiation Oncology
- Reproductive Medicine
- Browse content in Surgery
- Cardiothoracic Surgery
- Gastro-intestinal and Colorectal Surgery
- General Surgery
- Neurosurgery
- Paediatric Surgery
- Peri-operative Care
- Plastic and Reconstructive Surgery
- Surgical Oncology
- Transplant Surgery
- Trauma and Orthopaedic Surgery
- Vascular Surgery
- Browse content in Science and Mathematics
- Browse content in Biological Sciences
- Aquatic Biology
- Biochemistry
- Bioinformatics and Computational Biology
- Developmental Biology
- Ecology and Conservation
- Evolutionary Biology
- Genetics and Genomics
- Microbiology
- Molecular and Cell Biology
- Natural History
- Plant Sciences and Forestry
- Research Methods in Life Sciences
- Structural Biology
- Systems Biology
- Zoology and Animal Sciences
- Browse content in Chemistry
- Analytical Chemistry
- Computational Chemistry
- Crystallography
- Environmental Chemistry
- Industrial Chemistry
- Inorganic Chemistry
- Materials Chemistry
- Medicinal Chemistry
- Mineralogy and Gems
- Organic Chemistry
- Physical Chemistry
- Polymer Chemistry
- Study and Communication Skills in Chemistry
- Theoretical Chemistry
- Browse content in Computer Science
- Artificial Intelligence
- Computer Architecture and Logic Design
- Game Studies
- Human-Computer Interaction
- Mathematical Theory of Computation
- Programming Languages
- Software Engineering
- Systems Analysis and Design
- Virtual Reality
- Browse content in Computing
- Business Applications
- Computer Security
- Computer Games
- Computer Networking and Communications
- Digital Lifestyle
- Graphical and Digital Media Applications
- Operating Systems
- Browse content in Earth Sciences and Geography
- Atmospheric Sciences
- Environmental Geography
- Geology and the Lithosphere
- Maps and Map-making
- Meteorology and Climatology
- Oceanography and Hydrology
- Palaeontology
- Physical Geography and Topography
- Regional Geography
- Soil Science
- Urban Geography
- Browse content in Engineering and Technology
- Agriculture and Farming
- Biological Engineering
- Civil Engineering, Surveying, and Building
- Electronics and Communications Engineering
- Energy Technology
- Engineering (General)
- Environmental Science, Engineering, and Technology
- History of Engineering and Technology
- Mechanical Engineering and Materials
- Technology of Industrial Chemistry
- Transport Technology and Trades
- Browse content in Environmental Science
- Applied Ecology (Environmental Science)
- Conservation of the Environment (Environmental Science)
- Environmental Sustainability
- Environmentalist Thought and Ideology (Environmental Science)
- Management of Land and Natural Resources (Environmental Science)
- Natural Disasters (Environmental Science)
- Nuclear Issues (Environmental Science)
- Pollution and Threats to the Environment (Environmental Science)
- Social Impact of Environmental Issues (Environmental Science)
- History of Science and Technology
- Browse content in Materials Science
- Ceramics and Glasses
- Composite Materials
- Metals, Alloying, and Corrosion
- Nanotechnology
- Browse content in Mathematics
- Applied Mathematics
- Biomathematics and Statistics
- History of Mathematics
- Mathematical Education
- Mathematical Finance
- Mathematical Analysis
- Numerical and Computational Mathematics
- Probability and Statistics
- Pure Mathematics
- Browse content in Neuroscience
- Cognition and Behavioural Neuroscience
- Development of the Nervous System
- Disorders of the Nervous System
- History of Neuroscience
- Invertebrate Neurobiology
- Molecular and Cellular Systems
- Neuroendocrinology and Autonomic Nervous System
- Neuroscientific Techniques
- Sensory and Motor Systems
- Browse content in Physics
- Astronomy and Astrophysics
- Atomic, Molecular, and Optical Physics
- Biological and Medical Physics
- Classical Mechanics
- Computational Physics
- Condensed Matter Physics
- Electromagnetism, Optics, and Acoustics
- History of Physics
- Mathematical and Statistical Physics
- Measurement Science
- Nuclear Physics
- Particles and Fields
- Plasma Physics
- Quantum Physics
- Relativity and Gravitation
- Semiconductor and Mesoscopic Physics
- Browse content in Psychology
- Affective Sciences
- Clinical Psychology
- Cognitive Neuroscience
- Cognitive Psychology
- Criminal and Forensic Psychology
- Developmental Psychology
- Educational Psychology
- Evolutionary Psychology
- Health Psychology
- History and Systems in Psychology
- Music Psychology
- Neuropsychology
- Organizational Psychology
- Psychological Assessment and Testing
- Psychology of Human-Technology Interaction
- Psychology Professional Development and Training
- Research Methods in Psychology
- Social Psychology
- Browse content in Social Sciences
- Browse content in Anthropology
- Anthropology of Religion
- Human Evolution
- Medical Anthropology
- Physical Anthropology
- Regional Anthropology
- Social and Cultural Anthropology
- Theory and Practice of Anthropology
- Browse content in Business and Management
- Business Strategy
- Business History
- Business Ethics
- Business and Government
- Business and Technology
- Business and the Environment
- Comparative Management
- Corporate Governance
- Corporate Social Responsibility
- Entrepreneurship
- Health Management
- Human Resource Management
- Industrial and Employment Relations
- Industry Studies
- Information and Communication Technologies
- International Business
- Knowledge Management
- Management and Management Techniques
- Operations Management
- Organizational Theory and Behaviour
- Pensions and Pension Management
- Public and Nonprofit Management
- Social Issues in Business and Management
- Strategic Management
- Supply Chain Management
- Browse content in Criminology and Criminal Justice
- Criminal Justice
- Criminology
- Forms of Crime
- International and Comparative Criminology
- Youth Violence and Juvenile Justice
- Development Studies
- Browse content in Economics
- Agricultural, Environmental, and Natural Resource Economics
- Asian Economics
- Behavioural Finance
- Behavioural Economics and Neuroeconomics
- Econometrics and Mathematical Economics
- Economic Systems
- Economic Methodology
- Economic History
- Economic Development and Growth
- Financial Markets
- Financial Institutions and Services
- General Economics and Teaching
- Health, Education, and Welfare
- History of Economic Thought
- International Economics
- Labour and Demographic Economics
- Law and Economics
- Macroeconomics and Monetary Economics
- Microeconomics
- Public Economics
- Urban, Rural, and Regional Economics
- Welfare Economics
- Browse content in Education
- Adult Education and Continuous Learning
- Care and Counselling of Students
- Early Childhood and Elementary Education
- Educational Equipment and Technology
- Educational Strategies and Policy
- Higher and Further Education
- Organization and Management of Education
- Philosophy and Theory of Education
- Schools Studies
- Secondary Education
- Teaching of a Specific Subject
- Teaching of Specific Groups and Special Educational Needs
- Teaching Skills and Techniques
- Browse content in Environment
- Applied Ecology (Social Science)
- Climate Change
- Conservation of the Environment (Social Science)
- Environmentalist Thought and Ideology (Social Science)
- Management of Land and Natural Resources (Social Science)
- Natural Disasters (Environment)
- Pollution and Threats to the Environment (Social Science)
- Social Impact of Environmental Issues (Social Science)
- Sustainability
- Browse content in Human Geography
- Cultural Geography
- Economic Geography
- Political Geography
- Browse content in Interdisciplinary Studies
- Communication Studies
- Museums, Libraries, and Information Sciences
- Browse content in Politics
- African Politics
- Asian Politics
- Chinese Politics
- Comparative Politics
- Conflict Politics
- Elections and Electoral Studies
- Environmental Politics
- Ethnic Politics
- European Union
- Foreign Policy
- Gender and Politics
- Human Rights and Politics
- Indian Politics
- International Relations
- International Organization (Politics)
- Irish Politics
- Latin American Politics
- Middle Eastern Politics
- Political Methodology
- Political Communication
- Political Philosophy
- Political Sociology
- Political Theory
- Political Behaviour
- Political Economy
- Political Institutions
- Politics and Law
- Politics of Development
- Public Administration
- Public Policy
- Qualitative Political Methodology
- Quantitative Political Methodology
- Regional Political Studies
- Russian Politics
- Security Studies
- State and Local Government
- UK Politics
- US Politics
- Browse content in Regional and Area Studies
- African Studies
- Asian Studies
- East Asian Studies
- Japanese Studies
- Latin American Studies
- Middle Eastern Studies
- Native American Studies
- Scottish Studies
- Browse content in Research and Information
- Research Methods
- Browse content in Social Work
- Addictions and Substance Misuse
- Adoption and Fostering
- Care of the Elderly
- Child and Adolescent Social Work
- Couple and Family Social Work
- Direct Practice and Clinical Social Work
- Emergency Services
- Human Behaviour and the Social Environment
- International and Global Issues in Social Work
- Mental and Behavioural Health
- Social Justice and Human Rights
- Social Policy and Advocacy
- Social Work and Crime and Justice
- Social Work Macro Practice
- Social Work Practice Settings
- Social Work Research and Evidence-based Practice
- Welfare and Benefit Systems
- Browse content in Sociology
- Childhood Studies
- Community Development
- Comparative and Historical Sociology
- Disability Studies
- Economic Sociology
- Gender and Sexuality
- Gerontology and Ageing
- Health, Illness, and Medicine
- Marriage and the Family
- Migration Studies
- Occupations, Professions, and Work
- Organizations
- Population and Demography
- Race and Ethnicity
- Social Theory
- Social Movements and Social Change
- Social Research and Statistics
- Social Stratification, Inequality, and Mobility
- Sociology of Religion
- Sociology of Education
- Sport and Leisure
- Urban and Rural Studies
- Browse content in Warfare and Defence
- Defence Strategy, Planning, and Research
- Land Forces and Warfare
- Military Administration
- Military Life and Institutions
- Naval Forces and Warfare
- Other Warfare and Defence Issues
- Peace Studies and Conflict Resolution
- Weapons and Equipment

- < Previous chapter
- Next chapter >

6 6 Simple comparative experiments: comparing drug treatments for chronic asthmatics
- Published: August 2011
- Cite Icon Cite
- Permissions Icon Permissions
This chapter describes a clinical trial to be used to compare two anti-congestant drugs, Formoterol and Salbutamol. It considers the ways in which the trial might have been designed (parallel group design and paired design), and in each case how the data would have been analysed.

Personal account
- Sign in with email/username & password
- Get email alerts
- Save searches
- Purchase content
- Activate your purchase/trial code
- Add your ORCID iD
Institutional access
Sign in with a library card.
- Sign in with username/password
- Recommend to your librarian
- Institutional account management
- Get help with access
Access to content on Oxford Academic is often provided through institutional subscriptions and purchases. If you are a member of an institution with an active account, you may be able to access content in one of the following ways:
IP based access
Typically, access is provided across an institutional network to a range of IP addresses. This authentication occurs automatically, and it is not possible to sign out of an IP authenticated account.
Choose this option to get remote access when outside your institution. Shibboleth/Open Athens technology is used to provide single sign-on between your institution’s website and Oxford Academic.
- Click Sign in through your institution.
- Select your institution from the list provided, which will take you to your institution's website to sign in.
- When on the institution site, please use the credentials provided by your institution. Do not use an Oxford Academic personal account.
- Following successful sign in, you will be returned to Oxford Academic.
If your institution is not listed or you cannot sign in to your institution’s website, please contact your librarian or administrator.
Enter your library card number to sign in. If you cannot sign in, please contact your librarian.
Society Members
Society member access to a journal is achieved in one of the following ways:
Sign in through society site
Many societies offer single sign-on between the society website and Oxford Academic. If you see ‘Sign in through society site’ in the sign in pane within a journal:
- Click Sign in through society site.
- When on the society site, please use the credentials provided by that society. Do not use an Oxford Academic personal account.
If you do not have a society account or have forgotten your username or password, please contact your society.
Sign in using a personal account
Some societies use Oxford Academic personal accounts to provide access to their members. See below.
A personal account can be used to get email alerts, save searches, purchase content, and activate subscriptions.
Some societies use Oxford Academic personal accounts to provide access to their members.
Viewing your signed in accounts
Click the account icon in the top right to:
- View your signed in personal account and access account management features.
- View the institutional accounts that are providing access.
Signed in but can't access content
Oxford Academic is home to a wide variety of products. The institutional subscription may not cover the content that you are trying to access. If you believe you should have access to that content, please contact your librarian.
For librarians and administrators, your personal account also provides access to institutional account management. Here you will find options to view and activate subscriptions, manage institutional settings and access options, access usage statistics, and more.
Our books are available by subscription or purchase to libraries and institutions.
Month: | Total Views: |
---|---|
October 2022 | 4 |
November 2022 | 4 |
December 2022 | 7 |
January 2023 | 2 |
February 2023 | 4 |
March 2023 | 2 |
April 2023 | 2 |
June 2023 | 4 |
July 2023 | 6 |
August 2023 | 3 |
October 2023 | 3 |
November 2023 | 6 |
December 2023 | 3 |
January 2024 | 2 |
April 2024 | 6 |
May 2024 | 2 |
June 2024 | 6 |
July 2024 | 4 |
- About Oxford Academic
- Publish journals with us
- University press partners
- What we publish
- New features
- Open access
- Rights and permissions
- Accessibility
- Advertising
- Media enquiries
- Oxford University Press
- Oxford Languages
- University of Oxford
Oxford University Press is a department of the University of Oxford. It furthers the University's objective of excellence in research, scholarship, and education by publishing worldwide
- Copyright © 2024 Oxford University Press
- Cookie settings
- Cookie policy
- Privacy policy
- Legal notice
This Feature Is Available To Subscribers Only
Sign In or Create an Account
This PDF is available to Subscribers Only
For full access to this pdf, sign in to an existing account, or purchase an annual subscription.
Academia.edu no longer supports Internet Explorer.
To browse Academia.edu and the wider internet faster and more securely, please take a few seconds to upgrade your browser .
Enter the email address you signed up with and we'll email you a reset link.
- We're Hiring!
- Help Center
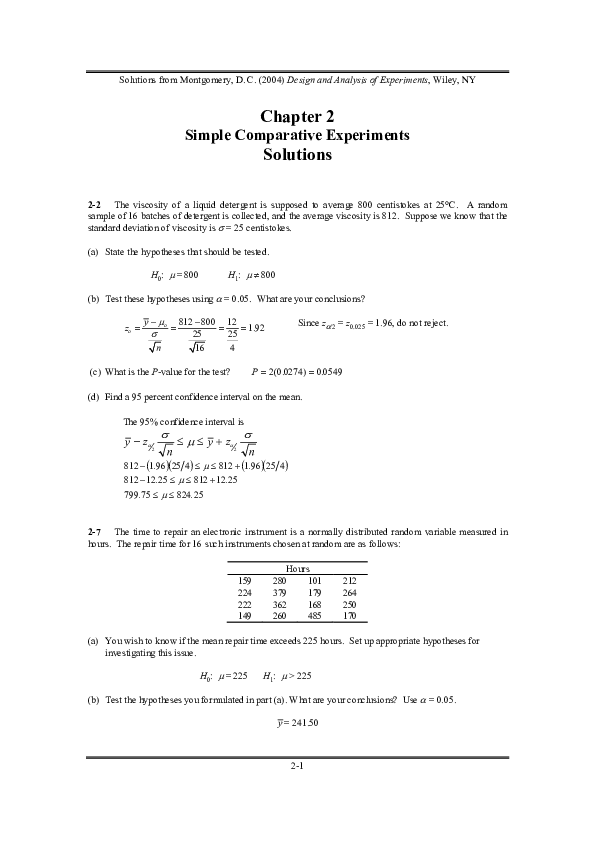
Chapter 2 Simple Comparative Experiments

Solutions 2-2 The viscosity of a liquid detergent is supposed to average 800 centistokes at 25°C. A random sample of 16 batches of detergent is collected, and the average viscosity is 812. Suppose we know that the standard deviation of viscosity is σ = 25 centistokes. (a) State the hypotheses that should be tested. H 0 : µ = 800 H 1 : µ ≠ 800 (b) Test these hypotheses using α = 0.05. What are your conclusions? 812 800 12 1.92 25 25 4 16 o o y z n µ σ − − = = = = Since z α/2 = z 0.025 = 1.96, do not reject. (c) What is the P-value for the test? P = = 2 0 0274 0 0549 (.). (d) Find a 95 percent confidence interval on the mean.
Related Papers
Juan Valencia
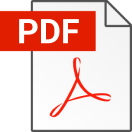
Uriel Vazquez
Vinh San Nguyen
1. There are two requirements for using the methods of this section, and each of them is violated. (1) The samples should be two sample random samples that are independent. These samples are convenience samples, not simple random samples. These samples are likely not independent. Since she surveyed her friends, she may well have males and females that are dating each other (or least that associate with each other) – and people tend to associate with those that have similar behaviors. (2) The number of successes for each sample should be at least 5, and the number of failures for each sample should be at least 5. This is not true for the males, for which x=4. NOTE: This is the same requirement from previous chapters for using the normal distribution to approximate the binomial that required np ≥ 5 and nq ≥ 5. Usingˆp =x/n to estimate p andˆq = 1-x/n = (n-x)/n to estimate q, nˆp ≥ 5 n ˆ q ≥ 5 n[x/n] ≥ 5 n[(n-x)/n] ≥ 5 x≥ 5 (n-x) ≥ 5 These inequalities state that the number of successes must be greater than 5, and the number of failures must be greater than 5. 2. We have 95% confidence that the limits of-0.0518 and 0.0194 contain the true difference between the population proportions of subjects who experience headaches. Repeating the trials many times would result in confidence limits that would include the true difference between the population proportions 95% of the time. Since the interval includes the value 0, there is no significant difference between the two populations proportions. 3. In this context, 1 ˆ p = 15/1583 = 0.00948 2 ˆ p = 8/157 = 0.05096 p = (15+8)/(1583+157) = 23/1740 = 0.01322 p 1 denotes the rue proportion of all Zocor users who experience headaches p 2 denotes the true proportion of all placebo users who experience headaches 4. No. The P-value method and the traditional method will always agree, but it is possible for the confidence interval approach to lead to a different conclusion. The P-value and traditional methods used a standard deviation for the sampling distribution of 1
Nirian Martín , Ayanendranath Basu
Loading Preview
Sorry, preview is currently unavailable. You can download the paper by clicking the button above.
- We're Hiring!
- Help Center
- Find new research papers in:
- Health Sciences
- Earth Sciences
- Cognitive Science
- Mathematics
- Computer Science
- Academia ©2024
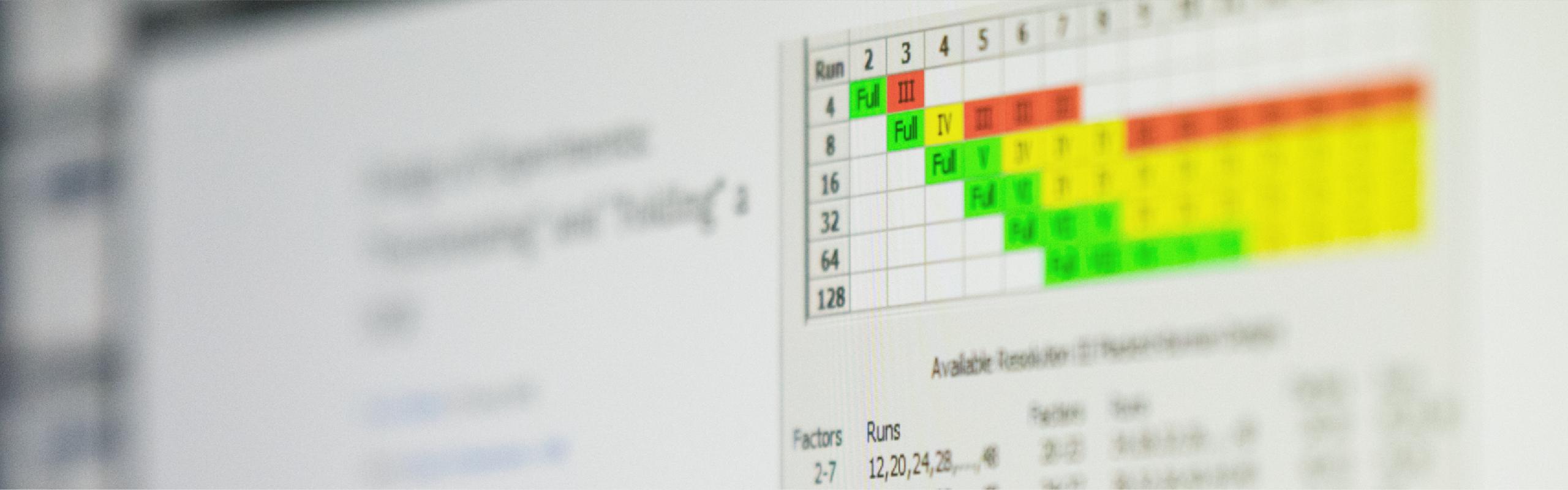
User Preferences
Content preview.
Arcu felis bibendum ut tristique et egestas quis:
- Ut enim ad minim veniam, quis nostrud exercitation ullamco laboris
- Duis aute irure dolor in reprehenderit in voluptate
- Excepteur sint occaecat cupidatat non proident
Keyboard Shortcuts
Welcome to stat 503, about this course.
Welcome to the course notes for STAT 503: Design of Experiments . These notes are designed and developed by Penn State's Department of Statistics and offered as open educational resources. These notes are free to use under Creative Commons license CC BY-NC 4.0 .
This course is part of the Online Master of Applied Statistics program offered by Penn State's World Campus.
Currently enrolled?
If you are a current student in this course, please see Canvas for your syllabus, assignments, lesson videos and communication from your instructor.
How to enroll?
If you would like to enroll and experience the entire course for credit please see ' How to enroll in a course ' on the World Campus website.
Course Overview Section
Statistics is often taught as though the design of the data collection and the data cleaning have already been done in advance. However, as most practicing statisticians quickly learn, typically problems that arise at the analysis stage, could have been avoided if the experimenter had consulted a statistician before the experiment was done and the data were conducted. This course is created to provide an understanding of how experiments should be designed so that when the data are collected, these shortcomings are avoided.
This course covers the following topics:
- Understanding basic design principles
- Working in simple comparative experimental contexts
- Working with single factors or one-way ANOVA in completely randomized experimental design contexts
- Implementing randomized blocks, Latin square designs and extensions of these
- Understanding factorial design contexts
- Working with two level, \(2^k\), designs
- Implementing confounding and blocking in \(2^k\) designs
- Working with 2-level fractional factorial designs
- Working with 3-level and mixed-level factorials and fractional factorial designs
- Simple linear regression models
- Understanding and implementing response surface methodologies
- Understanding robust parameter designs
- Working with random and mixed effects models
- Understanding and implementing nested and split-plot and strip-plot designs
- Using repeated measures designs, unbalanced AOV and ANCOVA
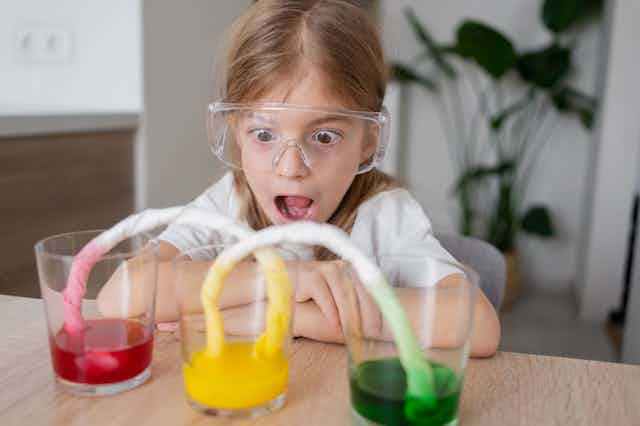
Summer holiday science: turn your home into a lab with these three easy experiments
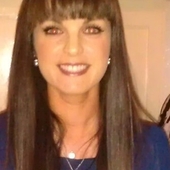
Associate Professor in Biology, University of Limerick
Disclosure statement
Audrey O'Grady receives funding from Science Foundation Ireland. She is affiliated with Department of Biological Sciences, University of Limerick.
University of Limerick provides funding as a member of The Conversation UK.
View all partners
Many people think science is difficult and needs special equipment, but that’s not true.
Science can be explored at home using everyday materials. Everyone, especially children, naturally ask questions about the world around them, and science offers a structured way to find answers.
Misconceptions about the difficulty of science often stem from a lack of exposure to its fun and engaging side. Science can be as simple as observing nature, mixing ingredients or exploring the properties of objects. It’s not just for experts in white coats, but for everyone.
Don’t take my word for it. Below are three experiments that can be done at home with children who are primary school age and older.
Extract DNA from bananas
DNA is all the genetic information inside cells. Every living thing has DNA, including bananas.
Did you know you can extract DNA from banana cells?
What you need: ¼ ripe banana, Ziploc bag, salt, water, washing-up liquid, rubbing alcohol (from a pharmacy), coffee filter paper, stirrer.
What you do:
Place a pinch of salt into about 20ml of water in a cup.
Add the salty water to the Ziploc bag with a quarter of a banana and mash the banana up with the salty water inside the bag, using your hands. Mashing the banana separates out the banana cells. The salty water helps clump the DNA together.
Once the banana is mashed up well, pour the banana and salty water into a coffee filter (you can lay the filter in the cup you used to make the salty water). Filtering removes the big clumps of banana cells.
Once a few ml have filtered out, add a drop of washing-up liquid and swirl gently. Washing-up liquid breaks down the fats in the cell membranes which makes the DNA separate from the other parts of the cell.
Slowly add some rubbing alcohol (about 10ml) to the filtered solution. DNA is insoluble in alcohol, therefore the DNA will clump together away from the alcohol and float, making it easy to see.
DNA will start to precipitate out looking slightly cloudy and stringy. What you’re seeing is thousands of DNA strands – the strands are too small to be seen even with a normal microscope. Scientists use powerful equipment to see individual strands.
Learn how plants ‘drink’ water
What you need: celery stalks (with their leaves), glass or clear cup, water, food dye, camera.
- Fill the glass ¾ full with water and add 10 drops of food dye.
- Place a celery stalk into the glass of coloured water. Take a photograph of the celery.
- For two to three days, photograph the celery at the same time every day. Make sure you take a photograph at the very start of the experiment.
What happens and why?
All plants, such as celery, have vertical tubes that act like a transport system. These narrow tubes draw up water using a phenomenon known as capillarity.
Imagine you have a thin straw and you dip it into a glass of water. Have you ever noticed how the water climbs up the straw a little bit, even though you didn’t suck on it? This is because of capillarity.
In plants, capillarity helps move water from the roots to the leaves. Plants have tiny tubes inside them, like thin straws, called capillaries. The water sticks to the sides of these tubes and climbs up. In your experiment, you will see the food dye in the water make its way to the leaves.
Build a balloon-powered racecar
What you need: tape, scissors, two skewers, cardboard, four bottle caps, one straw, one balloon.
- Cut the cardboard to about 10cm long and 5cm wide. This will form the base of your car.
- Make holes in the centre of four bottle caps. These are your wheels.
- To make the axles insert the wooden skewers through the holes in the cap. You will need to cut the skewers to fit the width of the cardboard base, but leave room for the wheels.
- Secure the wheels to the skewers with tape.
- Attach the axles to the underside of the car base with tape, ensuring the wheels can spin freely.
- Insert a straw into the opening of a balloon and secure it with tape, ensuring there are no air leaks.
- Attach the other end of the straw to the top of the car base, positioning it so the balloon can inflate and deflate towards the back of the car. Secure the straw with tape.
- Inflate the balloon through the straw, pinch the straw to hold the air, place the car on a flat surface, then release the straw.
The inflated balloon stores potential energy when blown up. When the air is released, Newton’s third law of motion kicks into gear: for every action, there is an equal and opposite reaction.
As the air rushes out of the balloon (action), it pushes the car in the opposite direction (reaction). The escaping air propels the car forward, making it move across the surface.
- Science experiments

Senior Laboratory Technician

Manager, Centre Policy and Translation

Newsletter and Deputy Social Media Producer

College Director and Principal | Curtin College

Head of School: Engineering, Computer and Mathematical Sciences

IMAGES
COMMENTS
2.1 - Simple Comparative Experiments. Simple comparative experiments are not only preliminary to this course but this takes you back probably into your first course in statistics. We will look at both hypothesis testing and estimation and from these perspectives, we will look at sample size determination.
Statistics 514: Simple Comparative Experiments Fall 2021 Power/Sample Size Calculations • Can form Operating Characteristic Curve (Power curve) for different levels of α, δ/σ and n - If σ known, use Normal distribution in calculations - If σ to be estimated, use non-central t (or table) • Assume σ is known and n1 = n2 = n H0: Y 1 − Y 2 ∼ N (0, 2σ2/n) H1: Y 1 − Y 2 ∼ N ...
2.1 - Simple Comparative Experiments. Simple comparative experiments are not only preliminary to this course but this takes you back probably into your first course in statistics. We will look at both hypothesis testing and estimation and from these perspectives, we will look at sample size determination.
Statistics 514: Basis Concepts and Comparative Experiments Statistical Inference: Testing Hypotheses • P-value is the probability that test statistic takes on a value that is at least as extreme as the observed value of the statistic when H0 is true. "Extreme" in the sense of the alternative hypothesis H1. Example 1:
Objectives. Upon completion of this lesson, you should be able to: review basic statistical concepts. review sample size calculation for two sample problems based on the t -test. review the difference between two independent samples and paired comparison design. review the assumptions underlying the t -test and how to test for these assumptions.
The right-tail probability for t = 2.101 is 0.025 and for t = 2.552 is 0.01. Double these probabilities because this is a two-sided test. Therefore the P-value must lie between these two probabilities, or. 0.05 < P-value < 0.02. These are upper and lower bounds on the P-value. We know that the actual P-value is 0.042.
Before we can carry out the experiment, we need to decide on the sample size. Figure 1: Design and reporting of a single-factor experiment with three levels using a two-sample t-test. ( a) Two ...
CHAPTER 2Simple Comparative Experiments CHAPTER OUTLINE 2.1 INTRODUCTION 2.2 BASIC STATISTICAL CONCEPTS 2.3 SAMPLING AND SAMPLING DISTRIBUTIONS 2.4 INFERENCES ABOUT THE DIFFERENCES IN MEANS, RANDOMIZED DESIGNS 2.4.1 Hypothesis Testing … - Selection from Design and Analysis of Experiments, 9th Edition [Book]
This video is part 1 of 2 on simple comparative experiments. It goes over response variable, explanatory variables, extraneous variables, experimental condi...
Statistics 514: Simple Comparative Experiments Fall 2015 Power/Sample Size Calculations • Can form Operating Characteristic Curve (Power curve) for different levels of α, δ/σ and n - If σ known, use Normal distribution in calculations - If σ to be estimated, use non-central t (or table) • Assume σ is known and n1 = n2 = n H0: Y 1 − Y 2 ∼ N(0,2σ2/n) H1: Y 1 − Y 2 ∼ N(δ ...
Statistics 514: Basis Concepts and Comparative Experiments Statistical Inference: Testing Hypotheses • P-valueis the probability that test statistic takes on a value that is at least as extreme as the observed value of the statistic when H0 is true. "Extreme" in the sense of the alternative hypothesis H1. Example 1:
Design of Comparative Experiments This book should be on the shelf of every practising statistician who designs experi-ments. ... 3 Simple treatment structure 43 3.1 Replication of control treatments 43 3.2 Comparing new treatments in the presence of a control 44
1.3 - Steps for Planning, Conducting and Analyzing an Experiment; Lesson 2: Simple Comparative Experiments. 2.1 - Simple Comparative Experiments; 2.2 - Sample Size Determination; 2.3 - Determining Power; Lesson 3: Experiments with a Single Factor - the Oneway ANOVA - in the Completely Randomized Design (CRD)
Simple Comparative Experiments. Many of the most useful designs are extremely simple. Sir Ronald Fisher. We now look at a method for making simple comparisons of two or more "treatments," such as varying brands of toothpaste, and testing their efficacy for preventing cavities. Called the F-test, it is named after Sir Ronald Fisher, a ...
According to Penn State, a comparative experiment starts with a question or hypothesis that asks how two or more treatments affect some response. When a scientist wants to know the difference between the effects of treatment A and treatment B on dependent variable C, he will run an experiment in which all of the conditions are the same except ...
Simple comparave experiments • Consider experiments to compare two condions • Simple comparave experiments • Example: - The strength of portland cement mortar - Two different formulaons: modified v.s. unmodified - Collect 10 observaons for each formulaons - Formulaons = Treatments (levels) 4
Simple Comparative Experiments One-Way ANOVA 1. Mark Anderson and Pat Whitcomb (2000), DOE Simplified, Productivity Inc., Chapter 2. ... form of design of experiment (DOE) can compare two levels of a single factor, e.g. two or more vendors. Or perhaps to compare the current process versus a
Simple Comparative Experiments; Design and Analysis of Experiments Douglas C. Montgomery. Chapter 2 Simple Comparative Experiments - all with Video Answers. Educators. Chapter Questions. 02:40. Problem 1 The breaking strength of a fiber is required to be at least 150 psi. Past experience has indicated that the standard deviation of breaking ...
P -value is the probability that test statistic takes on a value that is at least as extreme as the observed value of the statistic when H. "Extreme" in the sense of the alternative hypothesisH is true. 0. 1. Example 1: P − value = P(T. −1:71 or T.
The asthma trial described above is an example of a simple comparative experiment, whose defining features are the following. Each replicate of the experiment generates a single measure, called a response. Replicates can be performed under each of several qualitatively different experimental conditions which collectively define a single design variable, or treatment.
Chapter 2 Simple Comparative Experiments. 俊彦 章. Solutions 2-2 The viscosity of a liquid detergent is supposed to average 800 centistokes at 25°C. A random sample of 16 batches of detergent is collected, and the average viscosity is 812. Suppose we know that the standard deviation of viscosity is σ = 25 centistokes.
1.3 - Steps for Planning, Conducting and Analyzing an Experiment; Lesson 2: Simple Comparative Experiments. 2.1 - Simple Comparative Experiments; 2.2 - Sample Size Determination; 2.3 - Determining Power; Lesson 3: Experiments with a Single Factor - the Oneway ANOVA - in the Completely Randomized Design (CRD)
This course is created to provide an understanding of how experiments should be designed so that when the data are collected, these shortcomings are avoided. This course covers the following topics: Understanding basic design principles. Working in simple comparative experimental contexts. Working with single factors or one-way ANOVA in ...
In your experiment, you will see the food dye in the water make its way to the leaves. Build a balloon-powered racecar What you need: tape, scissors, two skewers, cardboard, four bottle caps, one ...
Don't worry if you were more of an arts student—these science experiments are extremely easy and a ton of fun. Plus, it's a great way to spend quality time together and learn some things you may ...