- Research Process
- Manuscript Preparation
- Manuscript Review
- Publication Process
- Publication Recognition
- Language Editing Services
- Translation Services
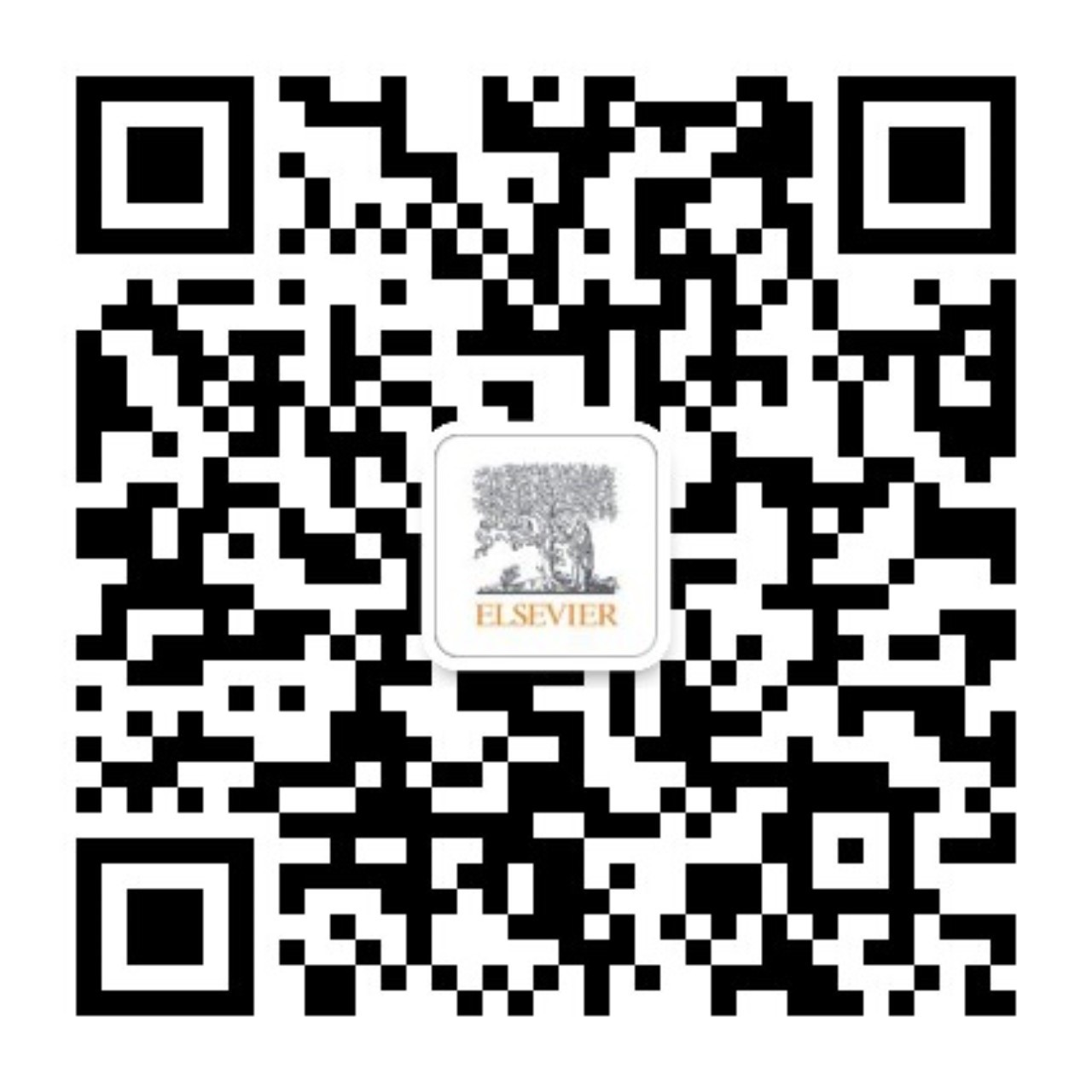

Choosing the Right Research Methodology: A Guide for Researchers
- 3 minute read
- 47.9K views
Table of Contents
Choosing an optimal research methodology is crucial for the success of any research project. The methodology you select will determine the type of data you collect, how you collect it, and how you analyse it. Understanding the different types of research methods available along with their strengths and weaknesses, is thus imperative to make an informed decision.
Understanding different research methods:
There are several research methods available depending on the type of study you are conducting, i.e., whether it is laboratory-based, clinical, epidemiological, or survey based . Some common methodologies include qualitative research, quantitative research, experimental research, survey-based research, and action research. Each method can be opted for and modified, depending on the type of research hypotheses and objectives.
Qualitative vs quantitative research:
When deciding on a research methodology, one of the key factors to consider is whether your research will be qualitative or quantitative. Qualitative research is used to understand people’s experiences, concepts, thoughts, or behaviours . Quantitative research, on the contrary, deals with numbers, graphs, and charts, and is used to test or confirm hypotheses, assumptions, and theories.
Qualitative research methodology:
Qualitative research is often used to examine issues that are not well understood, and to gather additional insights on these topics. Qualitative research methods include open-ended survey questions, observations of behaviours described through words, and reviews of literature that has explored similar theories and ideas. These methods are used to understand how language is used in real-world situations, identify common themes or overarching ideas, and describe and interpret various texts. Data analysis for qualitative research typically includes discourse analysis, thematic analysis, and textual analysis.
Quantitative research methodology:
The goal of quantitative research is to test hypotheses, confirm assumptions and theories, and determine cause-and-effect relationships. Quantitative research methods include experiments, close-ended survey questions, and countable and numbered observations. Data analysis for quantitative research relies heavily on statistical methods.
Analysing qualitative vs quantitative data:
The methods used for data analysis also differ for qualitative and quantitative research. As mentioned earlier, quantitative data is generally analysed using statistical methods and does not leave much room for speculation. It is more structured and follows a predetermined plan. In quantitative research, the researcher starts with a hypothesis and uses statistical methods to test it. Contrarily, methods used for qualitative data analysis can identify patterns and themes within the data, rather than provide statistical measures of the data. It is an iterative process, where the researcher goes back and forth trying to gauge the larger implications of the data through different perspectives and revising the analysis if required.
When to use qualitative vs quantitative research:
The choice between qualitative and quantitative research will depend on the gap that the research project aims to address, and specific objectives of the study. If the goal is to establish facts about a subject or topic, quantitative research is an appropriate choice. However, if the goal is to understand people’s experiences or perspectives, qualitative research may be more suitable.
Conclusion:
In conclusion, an understanding of the different research methods available, their applicability, advantages, and disadvantages is essential for making an informed decision on the best methodology for your project. If you need any additional guidance on which research methodology to opt for, you can head over to Elsevier Author Services (EAS). EAS experts will guide you throughout the process and help you choose the perfect methodology for your research goals.
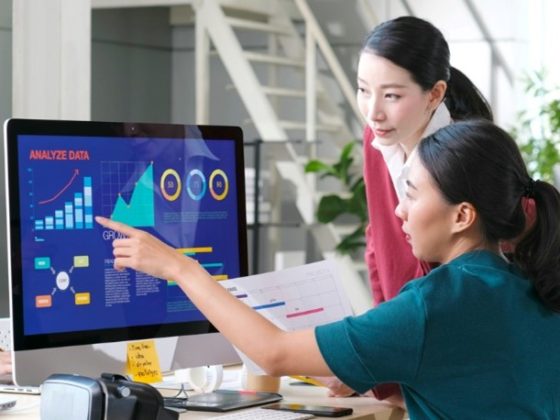
Why is data validation important in research?
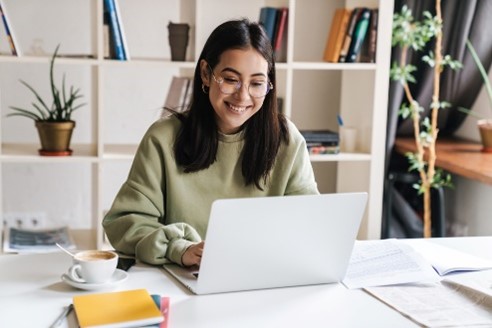
When Data Speak, Listen: Importance of Data Collection and Analysis Methods
You may also like.
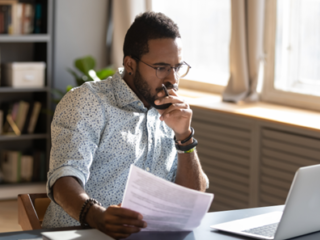
Descriptive Research Design and Its Myriad Uses
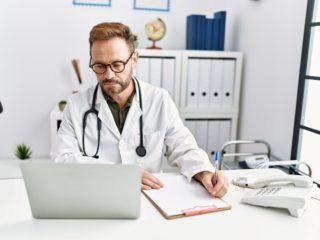
Five Common Mistakes to Avoid When Writing a Biomedical Research Paper
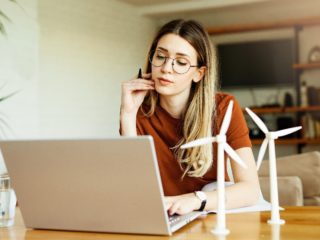
Making Technical Writing in Environmental Engineering Accessible
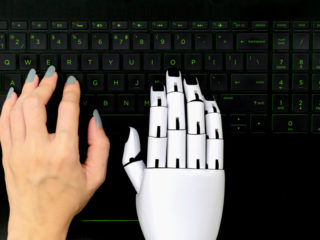
To Err is Not Human: The Dangers of AI-assisted Academic Writing
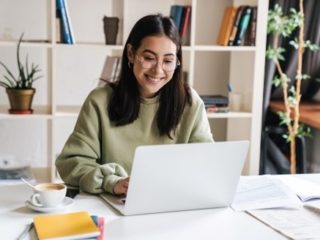
Writing a good review article
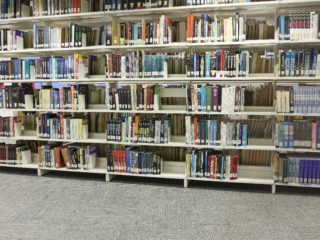
Scholarly Sources: What are They and Where can You Find Them?
Input your search keywords and press Enter.
Have a language expert improve your writing
Run a free plagiarism check in 10 minutes, automatically generate references for free.
- Knowledge Base
- Methodology
Research Methods | Definition, Types, Examples
Research methods are specific procedures for collecting and analysing data. Developing your research methods is an integral part of your research design . When planning your methods, there are two key decisions you will make.
First, decide how you will collect data . Your methods depend on what type of data you need to answer your research question :
- Qualitative vs quantitative : Will your data take the form of words or numbers?
- Primary vs secondary : Will you collect original data yourself, or will you use data that have already been collected by someone else?
- Descriptive vs experimental : Will you take measurements of something as it is, or will you perform an experiment?
Second, decide how you will analyse the data .
- For quantitative data, you can use statistical analysis methods to test relationships between variables.
- For qualitative data, you can use methods such as thematic analysis to interpret patterns and meanings in the data.
Table of contents
Methods for collecting data, examples of data collection methods, methods for analysing data, examples of data analysis methods, frequently asked questions about methodology.
Data are the information that you collect for the purposes of answering your research question . The type of data you need depends on the aims of your research.
Qualitative vs quantitative data
Your choice of qualitative or quantitative data collection depends on the type of knowledge you want to develop.
For questions about ideas, experiences and meanings, or to study something that can’t be described numerically, collect qualitative data .
If you want to develop a more mechanistic understanding of a topic, or your research involves hypothesis testing , collect quantitative data .
Qualitative | ||
---|---|---|
Quantitative | . |
You can also take a mixed methods approach, where you use both qualitative and quantitative research methods.
Primary vs secondary data
Primary data are any original information that you collect for the purposes of answering your research question (e.g. through surveys , observations and experiments ). Secondary data are information that has already been collected by other researchers (e.g. in a government census or previous scientific studies).
If you are exploring a novel research question, you’ll probably need to collect primary data. But if you want to synthesise existing knowledge, analyse historical trends, or identify patterns on a large scale, secondary data might be a better choice.
Primary | ||
---|---|---|
Secondary |
Descriptive vs experimental data
In descriptive research , you collect data about your study subject without intervening. The validity of your research will depend on your sampling method .
In experimental research , you systematically intervene in a process and measure the outcome. The validity of your research will depend on your experimental design .
To conduct an experiment, you need to be able to vary your independent variable , precisely measure your dependent variable, and control for confounding variables . If it’s practically and ethically possible, this method is the best choice for answering questions about cause and effect.
Descriptive | ||
---|---|---|
Experimental |
Prevent plagiarism, run a free check.
Research method | Primary or secondary? | Qualitative or quantitative? | When to use |
---|---|---|---|
Primary | Quantitative | To test cause-and-effect relationships. | |
Primary | Quantitative | To understand general characteristics of a population. | |
Interview/focus group | Primary | Qualitative | To gain more in-depth understanding of a topic. |
Observation | Primary | Either | To understand how something occurs in its natural setting. |
Secondary | Either | To situate your research in an existing body of work, or to evaluate trends within a research topic. | |
Either | Either | To gain an in-depth understanding of a specific group or context, or when you don’t have the resources for a large study. |
Your data analysis methods will depend on the type of data you collect and how you prepare them for analysis.
Data can often be analysed both quantitatively and qualitatively. For example, survey responses could be analysed qualitatively by studying the meanings of responses or quantitatively by studying the frequencies of responses.
Qualitative analysis methods
Qualitative analysis is used to understand words, ideas, and experiences. You can use it to interpret data that were collected:
- From open-ended survey and interview questions, literature reviews, case studies, and other sources that use text rather than numbers.
- Using non-probability sampling methods .
Qualitative analysis tends to be quite flexible and relies on the researcher’s judgement, so you have to reflect carefully on your choices and assumptions.
Quantitative analysis methods
Quantitative analysis uses numbers and statistics to understand frequencies, averages and correlations (in descriptive studies) or cause-and-effect relationships (in experiments).
You can use quantitative analysis to interpret data that were collected either:
- During an experiment.
- Using probability sampling methods .
Because the data are collected and analysed in a statistically valid way, the results of quantitative analysis can be easily standardised and shared among researchers.
Research method | Qualitative or quantitative? | When to use |
---|---|---|
Quantitative | To analyse data collected in a statistically valid manner (e.g. from experiments, surveys, and observations). | |
Meta-analysis | Quantitative | To statistically analyse the results of a large collection of studies. Can only be applied to studies that collected data in a statistically valid manner. |
Qualitative | To analyse data collected from interviews, focus groups or textual sources. To understand general themes in the data and how they are communicated. | |
Either | To analyse large volumes of textual or visual data collected from surveys, literature reviews, or other sources. Can be quantitative (i.e. frequencies of words) or qualitative (i.e. meanings of words). |
Quantitative research deals with numbers and statistics, while qualitative research deals with words and meanings.
Quantitative methods allow you to test a hypothesis by systematically collecting and analysing data, while qualitative methods allow you to explore ideas and experiences in depth.
In mixed methods research , you use both qualitative and quantitative data collection and analysis methods to answer your research question .
A sample is a subset of individuals from a larger population. Sampling means selecting the group that you will actually collect data from in your research.
For example, if you are researching the opinions of students in your university, you could survey a sample of 100 students.
Statistical sampling allows you to test a hypothesis about the characteristics of a population. There are various sampling methods you can use to ensure that your sample is representative of the population as a whole.
The research methods you use depend on the type of data you need to answer your research question .
- If you want to measure something or test a hypothesis , use quantitative methods . If you want to explore ideas, thoughts, and meanings, use qualitative methods .
- If you want to analyse a large amount of readily available data, use secondary data. If you want data specific to your purposes with control over how they are generated, collect primary data.
- If you want to establish cause-and-effect relationships between variables , use experimental methods. If you want to understand the characteristics of a research subject, use descriptive methods.
Methodology refers to the overarching strategy and rationale of your research project . It involves studying the methods used in your field and the theories or principles behind them, in order to develop an approach that matches your objectives.
Methods are the specific tools and procedures you use to collect and analyse data (e.g. experiments, surveys , and statistical tests ).
In shorter scientific papers, where the aim is to report the findings of a specific study, you might simply describe what you did in a methods section .
In a longer or more complex research project, such as a thesis or dissertation , you will probably include a methodology section , where you explain your approach to answering the research questions and cite relevant sources to support your choice of methods.
Is this article helpful?
More interesting articles.
- A Quick Guide to Experimental Design | 5 Steps & Examples
- Between-Subjects Design | Examples, Pros & Cons
- Case Study | Definition, Examples & Methods
- Cluster Sampling | A Simple Step-by-Step Guide with Examples
- Confounding Variables | Definition, Examples & Controls
- Construct Validity | Definition, Types, & Examples
- Content Analysis | A Step-by-Step Guide with Examples
- Control Groups and Treatment Groups | Uses & Examples
- Controlled Experiments | Methods & Examples of Control
- Correlation vs Causation | Differences, Designs & Examples
- Correlational Research | Guide, Design & Examples
- Critical Discourse Analysis | Definition, Guide & Examples
- Cross-Sectional Study | Definitions, Uses & Examples
- Data Cleaning | A Guide with Examples & Steps
- Data Collection Methods | Step-by-Step Guide & Examples
- Descriptive Research Design | Definition, Methods & Examples
- Doing Survey Research | A Step-by-Step Guide & Examples
- Ethical Considerations in Research | Types & Examples
- Explanatory Research | Definition, Guide, & Examples
- Explanatory vs Response Variables | Definitions & Examples
- Exploratory Research | Definition, Guide, & Examples
- External Validity | Types, Threats & Examples
- Extraneous Variables | Examples, Types, Controls
- Face Validity | Guide with Definition & Examples
- How to Do Thematic Analysis | Guide & Examples
- How to Write a Strong Hypothesis | Guide & Examples
- Inclusion and Exclusion Criteria | Examples & Definition
- Independent vs Dependent Variables | Definition & Examples
- Inductive Reasoning | Types, Examples, Explanation
- Inductive vs Deductive Research Approach (with Examples)
- Internal Validity | Definition, Threats & Examples
- Internal vs External Validity | Understanding Differences & Examples
- Longitudinal Study | Definition, Approaches & Examples
- Mediator vs Moderator Variables | Differences & Examples
- Mixed Methods Research | Definition, Guide, & Examples
- Multistage Sampling | An Introductory Guide with Examples
- Naturalistic Observation | Definition, Guide & Examples
- Operationalisation | A Guide with Examples, Pros & Cons
- Population vs Sample | Definitions, Differences & Examples
- Primary Research | Definition, Types, & Examples
- Qualitative vs Quantitative Research | Examples & Methods
- Quasi-Experimental Design | Definition, Types & Examples
- Questionnaire Design | Methods, Question Types & Examples
- Random Assignment in Experiments | Introduction & Examples
- Reliability vs Validity in Research | Differences, Types & Examples
- Reproducibility vs Replicability | Difference & Examples
- Research Design | Step-by-Step Guide with Examples
- Sampling Methods | Types, Techniques, & Examples
- Semi-Structured Interview | Definition, Guide & Examples
- Simple Random Sampling | Definition, Steps & Examples
- Stratified Sampling | A Step-by-Step Guide with Examples
- Structured Interview | Definition, Guide & Examples
- Systematic Review | Definition, Examples & Guide
- Systematic Sampling | A Step-by-Step Guide with Examples
- Textual Analysis | Guide, 3 Approaches & Examples
- The 4 Types of Reliability in Research | Definitions & Examples
- The 4 Types of Validity | Types, Definitions & Examples
- Transcribing an Interview | 5 Steps & Transcription Software
- Triangulation in Research | Guide, Types, Examples
- Types of Interviews in Research | Guide & Examples
- Types of Research Designs Compared | Examples
- Types of Variables in Research | Definitions & Examples
- Unstructured Interview | Definition, Guide & Examples
- What Are Control Variables | Definition & Examples
- What Is a Case-Control Study? | Definition & Examples
- What Is a Cohort Study? | Definition & Examples
- What Is a Conceptual Framework? | Tips & Examples
- What Is a Double-Barrelled Question?
- What Is a Double-Blind Study? | Introduction & Examples
- What Is a Focus Group? | Step-by-Step Guide & Examples
- What Is a Likert Scale? | Guide & Examples
- What is a Literature Review? | Guide, Template, & Examples
- What Is a Prospective Cohort Study? | Definition & Examples
- What Is a Retrospective Cohort Study? | Definition & Examples
- What Is Action Research? | Definition & Examples
- What Is an Observational Study? | Guide & Examples
- What Is Concurrent Validity? | Definition & Examples
- What Is Content Validity? | Definition & Examples
- What Is Convenience Sampling? | Definition & Examples
- What Is Convergent Validity? | Definition & Examples
- What Is Criterion Validity? | Definition & Examples
- What Is Deductive Reasoning? | Explanation & Examples
- What Is Discriminant Validity? | Definition & Example
- What Is Ecological Validity? | Definition & Examples
- What Is Ethnography? | Meaning, Guide & Examples
- What Is Non-Probability Sampling? | Types & Examples
- What Is Participant Observation? | Definition & Examples
- What Is Peer Review? | Types & Examples
- What Is Predictive Validity? | Examples & Definition
- What Is Probability Sampling? | Types & Examples
- What Is Purposive Sampling? | Definition & Examples
- What Is Qualitative Observation? | Definition & Examples
- What Is Qualitative Research? | Methods & Examples
- What Is Quantitative Observation? | Definition & Examples
- What Is Quantitative Research? | Definition & Methods
- What Is Quota Sampling? | Definition & Examples
- What is Secondary Research? | Definition, Types, & Examples
- What Is Snowball Sampling? | Definition & Examples
- Within-Subjects Design | Explanation, Approaches, Examples
- USC Libraries
- Research Guides
Organizing Your Social Sciences Research Paper
- 6. The Methodology
- Purpose of Guide
- Design Flaws to Avoid
- Independent and Dependent Variables
- Glossary of Research Terms
- Reading Research Effectively
- Narrowing a Topic Idea
- Broadening a Topic Idea
- Extending the Timeliness of a Topic Idea
- Academic Writing Style
- Applying Critical Thinking
- Choosing a Title
- Making an Outline
- Paragraph Development
- Research Process Video Series
- Executive Summary
- The C.A.R.S. Model
- Background Information
- The Research Problem/Question
- Theoretical Framework
- Citation Tracking
- Content Alert Services
- Evaluating Sources
- Primary Sources
- Secondary Sources
- Tiertiary Sources
- Scholarly vs. Popular Publications
- Qualitative Methods
- Quantitative Methods
- Insiderness
- Using Non-Textual Elements
- Limitations of the Study
- Common Grammar Mistakes
- Writing Concisely
- Avoiding Plagiarism
- Footnotes or Endnotes?
- Further Readings
- Generative AI and Writing
- USC Libraries Tutorials and Other Guides
- Bibliography
The methods section describes actions taken to investigate a research problem and the rationale for the application of specific procedures or techniques used to identify, select, process, and analyze information applied to understanding the problem, thereby, allowing the reader to critically evaluate a study’s overall validity and reliability. The methodology section of a research paper answers two main questions: How was the data collected or generated? And, how was it analyzed? The writing should be direct and precise and always written in the past tense.
Kallet, Richard H. "How to Write the Methods Section of a Research Paper." Respiratory Care 49 (October 2004): 1229-1232.
Importance of a Good Methodology Section
You must explain how you obtained and analyzed your results for the following reasons:
- Readers need to know how the data was obtained because the method you chose affects the results and, by extension, how you interpreted their significance in the discussion section of your paper.
- Methodology is crucial for any branch of scholarship because an unreliable method produces unreliable results and, as a consequence, undermines the value of your analysis of the findings.
- In most cases, there are a variety of different methods you can choose to investigate a research problem. The methodology section of your paper should clearly articulate the reasons why you have chosen a particular procedure or technique.
- The reader wants to know that the data was collected or generated in a way that is consistent with accepted practice in the field of study. For example, if you are using a multiple choice questionnaire, readers need to know that it offered your respondents a reasonable range of answers to choose from.
- The method must be appropriate to fulfilling the overall aims of the study. For example, you need to ensure that you have a large enough sample size to be able to generalize and make recommendations based upon the findings.
- The methodology should discuss the problems that were anticipated and the steps you took to prevent them from occurring. For any problems that do arise, you must describe the ways in which they were minimized or why these problems do not impact in any meaningful way your interpretation of the findings.
- In the social and behavioral sciences, it is important to always provide sufficient information to allow other researchers to adopt or replicate your methodology. This information is particularly important when a new method has been developed or an innovative use of an existing method is utilized.
Bem, Daryl J. Writing the Empirical Journal Article. Psychology Writing Center. University of Washington; Denscombe, Martyn. The Good Research Guide: For Small-Scale Social Research Projects . 5th edition. Buckingham, UK: Open University Press, 2014; Lunenburg, Frederick C. Writing a Successful Thesis or Dissertation: Tips and Strategies for Students in the Social and Behavioral Sciences . Thousand Oaks, CA: Corwin Press, 2008.
Structure and Writing Style
I. Groups of Research Methods
There are two main groups of research methods in the social sciences:
- The e mpirical-analytical group approaches the study of social sciences in a similar manner that researchers study the natural sciences . This type of research focuses on objective knowledge, research questions that can be answered yes or no, and operational definitions of variables to be measured. The empirical-analytical group employs deductive reasoning that uses existing theory as a foundation for formulating hypotheses that need to be tested. This approach is focused on explanation.
- The i nterpretative group of methods is focused on understanding phenomenon in a comprehensive, holistic way . Interpretive methods focus on analytically disclosing the meaning-making practices of human subjects [the why, how, or by what means people do what they do], while showing how those practices arrange so that it can be used to generate observable outcomes. Interpretive methods allow you to recognize your connection to the phenomena under investigation. However, the interpretative group requires careful examination of variables because it focuses more on subjective knowledge.
II. Content
The introduction to your methodology section should begin by restating the research problem and underlying assumptions underpinning your study. This is followed by situating the methods you used to gather, analyze, and process information within the overall “tradition” of your field of study and within the particular research design you have chosen to study the problem. If the method you choose lies outside of the tradition of your field [i.e., your review of the literature demonstrates that the method is not commonly used], provide a justification for how your choice of methods specifically addresses the research problem in ways that have not been utilized in prior studies.
The remainder of your methodology section should describe the following:
- Decisions made in selecting the data you have analyzed or, in the case of qualitative research, the subjects and research setting you have examined,
- Tools and methods used to identify and collect information, and how you identified relevant variables,
- The ways in which you processed the data and the procedures you used to analyze that data, and
- The specific research tools or strategies that you utilized to study the underlying hypothesis and research questions.
In addition, an effectively written methodology section should:
- Introduce the overall methodological approach for investigating your research problem . Is your study qualitative or quantitative or a combination of both (mixed method)? Are you going to take a special approach, such as action research, or a more neutral stance?
- Indicate how the approach fits the overall research design . Your methods for gathering data should have a clear connection to your research problem. In other words, make sure that your methods will actually address the problem. One of the most common deficiencies found in research papers is that the proposed methodology is not suitable to achieving the stated objective of your paper.
- Describe the specific methods of data collection you are going to use , such as, surveys, interviews, questionnaires, observation, archival research. If you are analyzing existing data, such as a data set or archival documents, describe how it was originally created or gathered and by whom. Also be sure to explain how older data is still relevant to investigating the current research problem.
- Explain how you intend to analyze your results . Will you use statistical analysis? Will you use specific theoretical perspectives to help you analyze a text or explain observed behaviors? Describe how you plan to obtain an accurate assessment of relationships, patterns, trends, distributions, and possible contradictions found in the data.
- Provide background and a rationale for methodologies that are unfamiliar for your readers . Very often in the social sciences, research problems and the methods for investigating them require more explanation/rationale than widely accepted rules governing the natural and physical sciences. Be clear and concise in your explanation.
- Provide a justification for subject selection and sampling procedure . For instance, if you propose to conduct interviews, how do you intend to select the sample population? If you are analyzing texts, which texts have you chosen, and why? If you are using statistics, why is this set of data being used? If other data sources exist, explain why the data you chose is most appropriate to addressing the research problem.
- Provide a justification for case study selection . A common method of analyzing research problems in the social sciences is to analyze specific cases. These can be a person, place, event, phenomenon, or other type of subject of analysis that are either examined as a singular topic of in-depth investigation or multiple topics of investigation studied for the purpose of comparing or contrasting findings. In either method, you should explain why a case or cases were chosen and how they specifically relate to the research problem.
- Describe potential limitations . Are there any practical limitations that could affect your data collection? How will you attempt to control for potential confounding variables and errors? If your methodology may lead to problems you can anticipate, state this openly and show why pursuing this methodology outweighs the risk of these problems cropping up.
NOTE: Once you have written all of the elements of the methods section, subsequent revisions should focus on how to present those elements as clearly and as logically as possibly. The description of how you prepared to study the research problem, how you gathered the data, and the protocol for analyzing the data should be organized chronologically. For clarity, when a large amount of detail must be presented, information should be presented in sub-sections according to topic. If necessary, consider using appendices for raw data.
ANOTHER NOTE: If you are conducting a qualitative analysis of a research problem , the methodology section generally requires a more elaborate description of the methods used as well as an explanation of the processes applied to gathering and analyzing of data than is generally required for studies using quantitative methods. Because you are the primary instrument for generating the data [e.g., through interviews or observations], the process for collecting that data has a significantly greater impact on producing the findings. Therefore, qualitative research requires a more detailed description of the methods used.
YET ANOTHER NOTE: If your study involves interviews, observations, or other qualitative techniques involving human subjects , you may be required to obtain approval from the university's Office for the Protection of Research Subjects before beginning your research. This is not a common procedure for most undergraduate level student research assignments. However, i f your professor states you need approval, you must include a statement in your methods section that you received official endorsement and adequate informed consent from the office and that there was a clear assessment and minimization of risks to participants and to the university. This statement informs the reader that your study was conducted in an ethical and responsible manner. In some cases, the approval notice is included as an appendix to your paper.
III. Problems to Avoid
Irrelevant Detail The methodology section of your paper should be thorough but concise. Do not provide any background information that does not directly help the reader understand why a particular method was chosen, how the data was gathered or obtained, and how the data was analyzed in relation to the research problem [note: analyzed, not interpreted! Save how you interpreted the findings for the discussion section]. With this in mind, the page length of your methods section will generally be less than any other section of your paper except the conclusion.
Unnecessary Explanation of Basic Procedures Remember that you are not writing a how-to guide about a particular method. You should make the assumption that readers possess a basic understanding of how to investigate the research problem on their own and, therefore, you do not have to go into great detail about specific methodological procedures. The focus should be on how you applied a method , not on the mechanics of doing a method. An exception to this rule is if you select an unconventional methodological approach; if this is the case, be sure to explain why this approach was chosen and how it enhances the overall process of discovery.
Problem Blindness It is almost a given that you will encounter problems when collecting or generating your data, or, gaps will exist in existing data or archival materials. Do not ignore these problems or pretend they did not occur. Often, documenting how you overcame obstacles can form an interesting part of the methodology. It demonstrates to the reader that you can provide a cogent rationale for the decisions you made to minimize the impact of any problems that arose.
Literature Review Just as the literature review section of your paper provides an overview of sources you have examined while researching a particular topic, the methodology section should cite any sources that informed your choice and application of a particular method [i.e., the choice of a survey should include any citations to the works you used to help construct the survey].
It’s More than Sources of Information! A description of a research study's method should not be confused with a description of the sources of information. Such a list of sources is useful in and of itself, especially if it is accompanied by an explanation about the selection and use of the sources. The description of the project's methodology complements a list of sources in that it sets forth the organization and interpretation of information emanating from those sources.
Azevedo, L.F. et al. "How to Write a Scientific Paper: Writing the Methods Section." Revista Portuguesa de Pneumologia 17 (2011): 232-238; Blair Lorrie. “Choosing a Methodology.” In Writing a Graduate Thesis or Dissertation , Teaching Writing Series. (Rotterdam: Sense Publishers 2016), pp. 49-72; Butin, Dan W. The Education Dissertation A Guide for Practitioner Scholars . Thousand Oaks, CA: Corwin, 2010; Carter, Susan. Structuring Your Research Thesis . New York: Palgrave Macmillan, 2012; Kallet, Richard H. “How to Write the Methods Section of a Research Paper.” Respiratory Care 49 (October 2004):1229-1232; Lunenburg, Frederick C. Writing a Successful Thesis or Dissertation: Tips and Strategies for Students in the Social and Behavioral Sciences . Thousand Oaks, CA: Corwin Press, 2008. Methods Section. The Writer’s Handbook. Writing Center. University of Wisconsin, Madison; Rudestam, Kjell Erik and Rae R. Newton. “The Method Chapter: Describing Your Research Plan.” In Surviving Your Dissertation: A Comprehensive Guide to Content and Process . (Thousand Oaks, Sage Publications, 2015), pp. 87-115; What is Interpretive Research. Institute of Public and International Affairs, University of Utah; Writing the Experimental Report: Methods, Results, and Discussion. The Writing Lab and The OWL. Purdue University; Methods and Materials. The Structure, Format, Content, and Style of a Journal-Style Scientific Paper. Department of Biology. Bates College.
Writing Tip
Statistical Designs and Tests? Do Not Fear Them!
Don't avoid using a quantitative approach to analyzing your research problem just because you fear the idea of applying statistical designs and tests. A qualitative approach, such as conducting interviews or content analysis of archival texts, can yield exciting new insights about a research problem, but it should not be undertaken simply because you have a disdain for running a simple regression. A well designed quantitative research study can often be accomplished in very clear and direct ways, whereas, a similar study of a qualitative nature usually requires considerable time to analyze large volumes of data and a tremendous burden to create new paths for analysis where previously no path associated with your research problem had existed.
To locate data and statistics, GO HERE .
Another Writing Tip
Knowing the Relationship Between Theories and Methods
There can be multiple meaning associated with the term "theories" and the term "methods" in social sciences research. A helpful way to delineate between them is to understand "theories" as representing different ways of characterizing the social world when you research it and "methods" as representing different ways of generating and analyzing data about that social world. Framed in this way, all empirical social sciences research involves theories and methods, whether they are stated explicitly or not. However, while theories and methods are often related, it is important that, as a researcher, you deliberately separate them in order to avoid your theories playing a disproportionate role in shaping what outcomes your chosen methods produce.
Introspectively engage in an ongoing dialectic between the application of theories and methods to help enable you to use the outcomes from your methods to interrogate and develop new theories, or ways of framing conceptually the research problem. This is how scholarship grows and branches out into new intellectual territory.
Reynolds, R. Larry. Ways of Knowing. Alternative Microeconomics . Part 1, Chapter 3. Boise State University; The Theory-Method Relationship. S-Cool Revision. United Kingdom.
Yet Another Writing Tip
Methods and the Methodology
Do not confuse the terms "methods" and "methodology." As Schneider notes, a method refers to the technical steps taken to do research . Descriptions of methods usually include defining and stating why you have chosen specific techniques to investigate a research problem, followed by an outline of the procedures you used to systematically select, gather, and process the data [remember to always save the interpretation of data for the discussion section of your paper].
The methodology refers to a discussion of the underlying reasoning why particular methods were used . This discussion includes describing the theoretical concepts that inform the choice of methods to be applied, placing the choice of methods within the more general nature of academic work, and reviewing its relevance to examining the research problem. The methodology section also includes a thorough review of the methods other scholars have used to study the topic.
Bryman, Alan. "Of Methods and Methodology." Qualitative Research in Organizations and Management: An International Journal 3 (2008): 159-168; Schneider, Florian. “What's in a Methodology: The Difference between Method, Methodology, and Theory…and How to Get the Balance Right?” PoliticsEastAsia.com. Chinese Department, University of Leiden, Netherlands.
- << Previous: Scholarly vs. Popular Publications
- Next: Qualitative Methods >>
- Last Updated: Jul 30, 2024 10:20 AM
- URL: https://libguides.usc.edu/writingguide
- How it works
"Christmas Offer"
Terms & conditions.
As the Christmas season is upon us, we find ourselves reflecting on the past year and those who we have helped to shape their future. It’s been quite a year for us all! The end of the year brings no greater joy than the opportunity to express to you Christmas greetings and good wishes.
At this special time of year, Research Prospect brings joyful discount of 10% on all its services. May your Christmas and New Year be filled with joy.
We are looking back with appreciation for your loyalty and looking forward to moving into the New Year together.
"Claim this offer"
In unfamiliar and hard times, we have stuck by you. This Christmas, Research Prospect brings you all the joy with exciting discount of 10% on all its services.
Offer valid till 5-1-2024
We love being your partner in success. We know you have been working hard lately, take a break this holiday season to spend time with your loved ones while we make sure you succeed in your academics
Discount code: RP23720
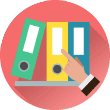
Published by Nicolas at March 21st, 2024 , Revised On March 12, 2024
The Ultimate Guide To Research Methodology
Research methodology is a crucial aspect of any investigative process, serving as the blueprint for the entire research journey. If you are stuck in the methodology section of your research paper , then this blog will guide you on what is a research methodology, its types and how to successfully conduct one.
Table of Contents
What Is Research Methodology?
Research methodology can be defined as the systematic framework that guides researchers in designing, conducting, and analyzing their investigations. It encompasses a structured set of processes, techniques, and tools employed to gather and interpret data, ensuring the reliability and validity of the research findings.
Research methodology is not confined to a singular approach; rather, it encapsulates a diverse range of methods tailored to the specific requirements of the research objectives.
Here is why Research methodology is important in academic and professional settings.
Facilitating Rigorous Inquiry
Research methodology forms the backbone of rigorous inquiry. It provides a structured approach that aids researchers in formulating precise thesis statements , selecting appropriate methodologies, and executing systematic investigations. This, in turn, enhances the quality and credibility of the research outcomes.
Ensuring Reproducibility And Reliability
In both academic and professional contexts, the ability to reproduce research outcomes is paramount. A well-defined research methodology establishes clear procedures, making it possible for others to replicate the study. This not only validates the findings but also contributes to the cumulative nature of knowledge.
Guiding Decision-Making Processes
In professional settings, decisions often hinge on reliable data and insights. Research methodology equips professionals with the tools to gather pertinent information, analyze it rigorously, and derive meaningful conclusions.
This informed decision-making is instrumental in achieving organizational goals and staying ahead in competitive environments.
Contributing To Academic Excellence
For academic researchers, adherence to robust research methodology is a hallmark of excellence. Institutions value research that adheres to high standards of methodology, fostering a culture of academic rigour and intellectual integrity. Furthermore, it prepares students with critical skills applicable beyond academia.
Enhancing Problem-Solving Abilities
Research methodology instills a problem-solving mindset by encouraging researchers to approach challenges systematically. It equips individuals with the skills to dissect complex issues, formulate hypotheses , and devise effective strategies for investigation.
Understanding Research Methodology
In the pursuit of knowledge and discovery, understanding the fundamentals of research methodology is paramount.
Basics Of Research
Research, in its essence, is a systematic and organized process of inquiry aimed at expanding our understanding of a particular subject or phenomenon. It involves the exploration of existing knowledge, the formulation of hypotheses, and the collection and analysis of data to draw meaningful conclusions.
Research is a dynamic and iterative process that contributes to the continuous evolution of knowledge in various disciplines.
Types of Research
Research takes on various forms, each tailored to the nature of the inquiry. Broadly classified, research can be categorized into two main types:
- Quantitative Research: This type involves the collection and analysis of numerical data to identify patterns, relationships, and statistical significance. It is particularly useful for testing hypotheses and making predictions.
- Qualitative Research: Qualitative research focuses on understanding the depth and details of a phenomenon through non-numerical data. It often involves methods such as interviews, focus groups, and content analysis, providing rich insights into complex issues.
Components Of Research Methodology
To conduct effective research, one must go through the different components of research methodology. These components form the scaffolding that supports the entire research process, ensuring its coherence and validity.
Research Design
Research design serves as the blueprint for the entire research project. It outlines the overall structure and strategy for conducting the study. The three primary types of research design are:
- Exploratory Research: Aimed at gaining insights and familiarity with the topic, often used in the early stages of research.
- Descriptive Research: Involves portraying an accurate profile of a situation or phenomenon, answering the ‘what,’ ‘who,’ ‘where,’ and ‘when’ questions.
- Explanatory Research: Seeks to identify the causes and effects of a phenomenon, explaining the ‘why’ and ‘how.’
Data Collection Methods
Choosing the right data collection methods is crucial for obtaining reliable and relevant information. Common methods include:
- Surveys and Questionnaires: Employed to gather information from a large number of respondents through standardized questions.
- Interviews: In-depth conversations with participants, offering qualitative insights.
- Observation: Systematic watching and recording of behaviour, events, or processes in their natural setting.
Data Analysis Techniques
Once data is collected, analysis becomes imperative to derive meaningful conclusions. Different methodologies exist for quantitative and qualitative data:
- Quantitative Data Analysis: Involves statistical techniques such as descriptive statistics, inferential statistics, and regression analysis to interpret numerical data.
- Qualitative Data Analysis: Methods like content analysis, thematic analysis, and grounded theory are employed to extract patterns, themes, and meanings from non-numerical data.
The research paper we write have:
- Precision and Clarity
- Zero Plagiarism
- High-level Encryption
- Authentic Sources
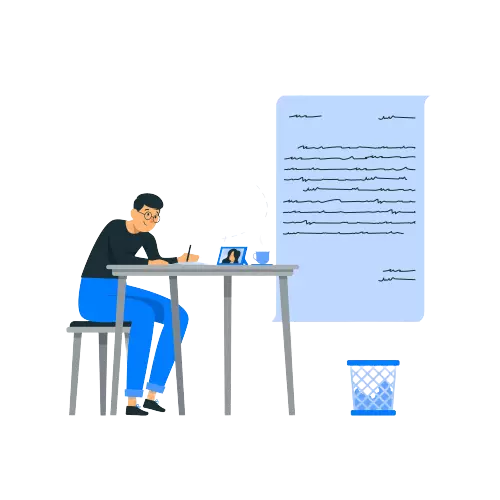
Choosing a Research Method
Selecting an appropriate research method is a critical decision in the research process. It determines the approach, tools, and techniques that will be used to answer the research questions.
Quantitative Research Methods
Quantitative research involves the collection and analysis of numerical data, providing a structured and objective approach to understanding and explaining phenomena.
Experimental Research
Experimental research involves manipulating variables to observe the effect on another variable under controlled conditions. It aims to establish cause-and-effect relationships.
Key Characteristics:
- Controlled Environment: Experiments are conducted in a controlled setting to minimize external influences.
- Random Assignment: Participants are randomly assigned to different experimental conditions.
- Quantitative Data: Data collected is numerical, allowing for statistical analysis.
Applications: Commonly used in scientific studies and psychology to test hypotheses and identify causal relationships.
Survey Research
Survey research gathers information from a sample of individuals through standardized questionnaires or interviews. It aims to collect data on opinions, attitudes, and behaviours.
- Structured Instruments: Surveys use structured instruments, such as questionnaires, to collect data.
- Large Sample Size: Surveys often target a large and diverse group of participants.
- Quantitative Data Analysis: Responses are quantified for statistical analysis.
Applications: Widely employed in social sciences, marketing, and public opinion research to understand trends and preferences.
Descriptive Research
Descriptive research seeks to portray an accurate profile of a situation or phenomenon. It focuses on answering the ‘what,’ ‘who,’ ‘where,’ and ‘when’ questions.
- Observation and Data Collection: This involves observing and documenting without manipulating variables.
- Objective Description: Aim to provide an unbiased and factual account of the subject.
- Quantitative or Qualitative Data: T his can include both types of data, depending on the research focus.
Applications: Useful in situations where researchers want to understand and describe a phenomenon without altering it, common in social sciences and education.
Qualitative Research Methods
Qualitative research emphasizes exploring and understanding the depth and complexity of phenomena through non-numerical data.
A case study is an in-depth exploration of a particular person, group, event, or situation. It involves detailed, context-rich analysis.
- Rich Data Collection: Uses various data sources, such as interviews, observations, and documents.
- Contextual Understanding: Aims to understand the context and unique characteristics of the case.
- Holistic Approach: Examines the case in its entirety.
Applications: Common in social sciences, psychology, and business to investigate complex and specific instances.
Ethnography
Ethnography involves immersing the researcher in the culture or community being studied to gain a deep understanding of their behaviours, beliefs, and practices.
- Participant Observation: Researchers actively participate in the community or setting.
- Holistic Perspective: Focuses on the interconnectedness of cultural elements.
- Qualitative Data: In-depth narratives and descriptions are central to ethnographic studies.
Applications: Widely used in anthropology, sociology, and cultural studies to explore and document cultural practices.
Grounded Theory
Grounded theory aims to develop theories grounded in the data itself. It involves systematic data collection and analysis to construct theories from the ground up.
- Constant Comparison: Data is continually compared and analyzed during the research process.
- Inductive Reasoning: Theories emerge from the data rather than being imposed on it.
- Iterative Process: The research design evolves as the study progresses.
Applications: Commonly applied in sociology, nursing, and management studies to generate theories from empirical data.
Research design is the structural framework that outlines the systematic process and plan for conducting a study. It serves as the blueprint, guiding researchers on how to collect, analyze, and interpret data.
Exploratory, Descriptive, And Explanatory Designs
Exploratory design.
Exploratory research design is employed when a researcher aims to explore a relatively unknown subject or gain insights into a complex phenomenon.
- Flexibility: Allows for flexibility in data collection and analysis.
- Open-Ended Questions: Uses open-ended questions to gather a broad range of information.
- Preliminary Nature: Often used in the initial stages of research to formulate hypotheses.
Applications: Valuable in the early stages of investigation, especially when the researcher seeks a deeper understanding of a subject before formalizing research questions.
Descriptive Design
Descriptive research design focuses on portraying an accurate profile of a situation, group, or phenomenon.
- Structured Data Collection: Involves systematic and structured data collection methods.
- Objective Presentation: Aims to provide an unbiased and factual account of the subject.
- Quantitative or Qualitative Data: Can incorporate both types of data, depending on the research objectives.
Applications: Widely used in social sciences, marketing, and educational research to provide detailed and objective descriptions.
Explanatory Design
Explanatory research design aims to identify the causes and effects of a phenomenon, explaining the ‘why’ and ‘how’ behind observed relationships.
- Causal Relationships: Seeks to establish causal relationships between variables.
- Controlled Variables : Often involves controlling certain variables to isolate causal factors.
- Quantitative Analysis: Primarily relies on quantitative data analysis techniques.
Applications: Commonly employed in scientific studies and social sciences to delve into the underlying reasons behind observed patterns.
Cross-Sectional Vs. Longitudinal Designs
Cross-sectional design.
Cross-sectional designs collect data from participants at a single point in time.
- Snapshot View: Provides a snapshot of a population at a specific moment.
- Efficiency: More efficient in terms of time and resources.
- Limited Temporal Insights: Offers limited insights into changes over time.
Applications: Suitable for studying characteristics or behaviours that are stable or not expected to change rapidly.
Longitudinal Design
Longitudinal designs involve the collection of data from the same participants over an extended period.
- Temporal Sequence: Allows for the examination of changes over time.
- Causality Assessment: Facilitates the assessment of cause-and-effect relationships.
- Resource-Intensive: Requires more time and resources compared to cross-sectional designs.
Applications: Ideal for studying developmental processes, trends, or the impact of interventions over time.
Experimental Vs Non-experimental Designs
Experimental design.
Experimental designs involve manipulating variables under controlled conditions to observe the effect on another variable.
- Causality Inference: Enables the inference of cause-and-effect relationships.
- Quantitative Data: Primarily involves the collection and analysis of numerical data.
Applications: Commonly used in scientific studies, psychology, and medical research to establish causal relationships.
Non-Experimental Design
Non-experimental designs observe and describe phenomena without manipulating variables.
- Natural Settings: Data is often collected in natural settings without intervention.
- Descriptive or Correlational: Focuses on describing relationships or correlations between variables.
- Quantitative or Qualitative Data: This can involve either type of data, depending on the research approach.
Applications: Suitable for studying complex phenomena in real-world settings where manipulation may not be ethical or feasible.
Effective data collection is fundamental to the success of any research endeavour.
Designing Effective Surveys
Objective Design:
- Clearly define the research objectives to guide the survey design.
- Craft questions that align with the study’s goals and avoid ambiguity.
Structured Format:
- Use a structured format with standardized questions for consistency.
- Include a mix of closed-ended and open-ended questions for detailed insights.
Pilot Testing:
- Conduct pilot tests to identify and rectify potential issues with survey design.
- Ensure clarity, relevance, and appropriateness of questions.
Sampling Strategy:
- Develop a robust sampling strategy to ensure a representative participant group.
- Consider random sampling or stratified sampling based on the research goals.
Conducting Interviews
Establishing Rapport:
- Build rapport with participants to create a comfortable and open environment.
- Clearly communicate the purpose of the interview and the value of participants’ input.
Open-Ended Questions:
- Frame open-ended questions to encourage detailed responses.
- Allow participants to express their thoughts and perspectives freely.
Active Listening:
- Practice active listening to understand areas and gather rich data.
- Avoid interrupting and maintain a non-judgmental stance during the interview.
Ethical Considerations:
- Obtain informed consent and assure participants of confidentiality.
- Be transparent about the study’s purpose and potential implications.
Observation
1. participant observation.
Immersive Participation:
- Actively immerse yourself in the setting or group being observed.
- Develop a deep understanding of behaviours, interactions, and context.
Field Notes:
- Maintain detailed and reflective field notes during observations.
- Document observed patterns, unexpected events, and participant reactions.
Ethical Awareness:
- Be conscious of ethical considerations, ensuring respect for participants.
- Balance the role of observer and participant to minimize bias.
2. Non-participant Observation
Objective Observation:
- Maintain a more detached and objective stance during non-participant observation.
- Focus on recording behaviours, events, and patterns without direct involvement.
Data Reliability:
- Enhance the reliability of data by reducing observer bias.
- Develop clear observation protocols and guidelines.
Contextual Understanding:
- Strive for a thorough understanding of the observed context.
- Consider combining non-participant observation with other methods for triangulation.
Archival Research
1. using existing data.
Identifying Relevant Archives:
- Locate and access archives relevant to the research topic.
- Collaborate with institutions or repositories holding valuable data.
Data Verification:
- Verify the accuracy and reliability of archived data.
- Cross-reference with other sources to ensure data integrity.
Ethical Use:
- Adhere to ethical guidelines when using existing data.
- Respect copyright and intellectual property rights.
2. Challenges and Considerations
Incomplete or Inaccurate Archives:
- Address the possibility of incomplete or inaccurate archival records.
- Acknowledge limitations and uncertainties in the data.
Temporal Bias:
- Recognize potential temporal biases in archived data.
- Consider the historical context and changes that may impact interpretation.
Access Limitations:
- Address potential limitations in accessing certain archives.
- Seek alternative sources or collaborate with institutions to overcome barriers.
Common Challenges in Research Methodology
Conducting research is a complex and dynamic process, often accompanied by a myriad of challenges. Addressing these challenges is crucial to ensure the reliability and validity of research findings.
Sampling Issues
Sampling bias:.
- The presence of sampling bias can lead to an unrepresentative sample, affecting the generalizability of findings.
- Employ random sampling methods and ensure the inclusion of diverse participants to reduce bias.
Sample Size Determination:
- Determining an appropriate sample size is a delicate balance. Too small a sample may lack statistical power, while an excessively large sample may strain resources.
- Conduct a power analysis to determine the optimal sample size based on the research objectives and expected effect size.
Data Quality And Validity
Measurement error:.
- Inaccuracies in measurement tools or data collection methods can introduce measurement errors, impacting the validity of results.
- Pilot test instruments, calibrate equipment, and use standardized measures to enhance the reliability of data.
Construct Validity:
- Ensuring that the chosen measures accurately capture the intended constructs is a persistent challenge.
- Use established measurement instruments and employ multiple measures to assess the same construct for triangulation.
Time And Resource Constraints
Timeline pressures:.
- Limited timeframes can compromise the depth and thoroughness of the research process.
- Develop a realistic timeline, prioritize tasks, and communicate expectations with stakeholders to manage time constraints effectively.
Resource Availability:
- Inadequate resources, whether financial or human, can impede the execution of research activities.
- Seek external funding, collaborate with other researchers, and explore alternative methods that require fewer resources.
Managing Bias in Research
Selection bias:.
- Selecting participants in a way that systematically skews the sample can introduce selection bias.
- Employ randomization techniques, use stratified sampling, and transparently report participant recruitment methods.
Confirmation Bias:
- Researchers may unintentionally favour information that confirms their preconceived beliefs or hypotheses.
- Adopt a systematic and open-minded approach, use blinded study designs, and engage in peer review to mitigate confirmation bias.
Tips On How To Write A Research Methodology
Conducting successful research relies not only on the application of sound methodologies but also on strategic planning and effective collaboration. Here are some tips to enhance the success of your research methodology:
Tip 1. Clear Research Objectives
Well-defined research objectives guide the entire research process. Clearly articulate the purpose of your study, outlining specific research questions or hypotheses.
Tip 2. Comprehensive Literature Review
A thorough literature review provides a foundation for understanding existing knowledge and identifying gaps. Invest time in reviewing relevant literature to inform your research design and methodology.
Tip 3. Detailed Research Plan
A detailed plan serves as a roadmap, ensuring all aspects of the research are systematically addressed. Develop a detailed research plan outlining timelines, milestones, and tasks.
Tip 4. Ethical Considerations
Ethical practices are fundamental to maintaining the integrity of research. Address ethical considerations early, obtain necessary approvals, and ensure participant rights are safeguarded.
Tip 5. Stay Updated On Methodologies
Research methodologies evolve, and staying updated is essential for employing the most effective techniques. Engage in continuous learning by attending workshops, conferences, and reading recent publications.
Tip 6. Adaptability In Methods
Unforeseen challenges may arise during research, necessitating adaptability in methods. Be flexible and willing to modify your approach when needed, ensuring the integrity of the study.
Tip 7. Iterative Approach
Research is often an iterative process, and refining methods based on ongoing findings enhance the study’s robustness. Regularly review and refine your research design and methods as the study progresses.
Frequently Asked Questions
What is the research methodology.
Research methodology is the systematic process of planning, executing, and evaluating scientific investigation. It encompasses the techniques, tools, and procedures used to collect, analyze, and interpret data, ensuring the reliability and validity of research findings.
What are the methodologies in research?
Research methodologies include qualitative and quantitative approaches. Qualitative methods involve in-depth exploration of non-numerical data, while quantitative methods use statistical analysis to examine numerical data. Mixed methods combine both approaches for a comprehensive understanding of research questions.
How to write research methodology?
To write a research methodology, clearly outline the study’s design, data collection, and analysis procedures. Specify research tools, participants, and sampling methods. Justify choices and discuss limitations. Ensure clarity, coherence, and alignment with research objectives for a robust methodology section.
How to write the methodology section of a research paper?
In the methodology section of a research paper, describe the study’s design, data collection, and analysis methods. Detail procedures, tools, participants, and sampling. Justify choices, address ethical considerations, and explain how the methodology aligns with research objectives, ensuring clarity and rigour.
What is mixed research methodology?
Mixed research methodology combines both qualitative and quantitative research approaches within a single study. This approach aims to enhance the details and depth of research findings by providing a more comprehensive understanding of the research problem or question.
You May Also Like
In Canada, scholarships are generally not taxable if they are for education in a qualifying program. Consult tax regulations or a professional for accurate information.
To cite a TED Talk in APA style, include speaker’s name, publication year, talk title, “TED Conferences,” and URL for clarity and accuracy.
Do you require captivating and feasible research subjects in the area of nursing and medicine? If so, then your search […]
Ready to place an order?
USEFUL LINKS
Learning resources.
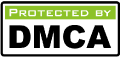
COMPANY DETAILS
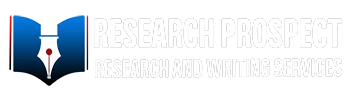
- How It Works
- University Libraries
- Research Guides
- Topic Guides
- Research Methods Guide
- Research Design & Method
Research Methods Guide: Research Design & Method
- Introduction
- Survey Research
- Interview Research
- Data Analysis
- Resources & Consultation
Tutorial Videos: Research Design & Method
Research Methods (sociology-focused)
Qualitative vs. Quantitative Methods (intro)
Qualitative vs. Quantitative Methods (advanced)
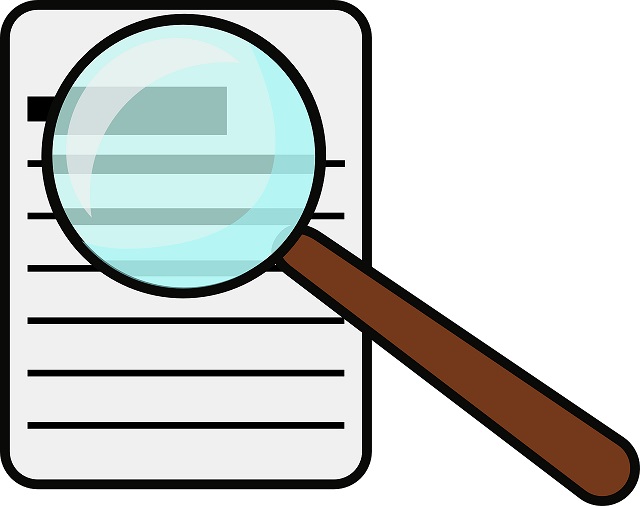
FAQ: Research Design & Method
What is the difference between Research Design and Research Method?
Research design is a plan to answer your research question. A research method is a strategy used to implement that plan. Research design and methods are different but closely related, because good research design ensures that the data you obtain will help you answer your research question more effectively.
Which research method should I choose ?
It depends on your research goal. It depends on what subjects (and who) you want to study. Let's say you are interested in studying what makes people happy, or why some students are more conscious about recycling on campus. To answer these questions, you need to make a decision about how to collect your data. Most frequently used methods include:
- Observation / Participant Observation
- Focus Groups
- Experiments
- Secondary Data Analysis / Archival Study
- Mixed Methods (combination of some of the above)
One particular method could be better suited to your research goal than others, because the data you collect from different methods will be different in quality and quantity. For instance, surveys are usually designed to produce relatively short answers, rather than the extensive responses expected in qualitative interviews.
What other factors should I consider when choosing one method over another?
Time for data collection and analysis is something you want to consider. An observation or interview method, so-called qualitative approach, helps you collect richer information, but it takes time. Using a survey helps you collect more data quickly, yet it may lack details. So, you will need to consider the time you have for research and the balance between strengths and weaknesses associated with each method (e.g., qualitative vs. quantitative).
- << Previous: Introduction
- Next: Survey Research >>
- Last Updated: Aug 21, 2023 10:42 AM
- Locations and Hours
- UCLA Library
- Research Guides
- Research Tips and Tools
Advanced Research Methods
Writing the research paper.
- What Is Research?
- Library Research
- Writing a Research Proposal
Before Writing the Paper
Methods, thesis and hypothesis, clarity, precision and academic expression, format your paper, typical problems, a few suggestions, avoid plagiarism.
- Presenting the Research Paper
Find a topic.
- Try to find a subject that really interests you.
- While you explore the topic, narrow or broaden your target and focus on something that gives the most promising results.
- Don't choose a huge subject if you have to write a 3 page long paper, and broaden your topic sufficiently if you have to submit at least 25 pages.
- Consult your class instructor (and your classmates) about the topic.
Explore the topic.
- Find primary and secondary sources in the library.
- Read and critically analyse them.
- Take notes.
- Compile surveys, collect data, gather materials for quantitative analysis (if these are good methods to investigate the topic more deeply).
- Come up with new ideas about the topic. Try to formulate your ideas in a few sentences.
- Review your notes and other materials and enrich the outline.
- Try to estimate how long the individual parts will be.
- Do others understand what you want to say?
- Do they accept it as new knowledge or relevant and important for a paper?
- Do they agree that your thoughts will result in a successful paper?
- Qualitative: gives answers on questions (how, why, when, who, what, etc.) by investigating an issue
- Quantitative:requires data and the analysis of data as well
- the essence, the point of the research paper in one or two sentences.
- a statement that can be proved or disproved.
- Be specific.
- Avoid ambiguity.
- Use predominantly the active voice, not the passive.
- Deal with one issue in one paragraph.
- Be accurate.
- Double-check your data, references, citations and statements.
Academic Expression
- Don't use familiar style or colloquial/slang expressions.
- Write in full sentences.
- Check the meaning of the words if you don't know exactly what they mean.
- Avoid metaphors.
- Almost the rough content of every paragraph.
- The order of the various topics in your paper.
- On the basis of the outline, start writing a part by planning the content, and then write it down.
- Put a visible mark (which you will later delete) where you need to quote a source, and write in the citation when you finish writing that part or a bigger part.
- Does the text make sense?
- Could you explain what you wanted?
- Did you write good sentences?
- Is there something missing?
- Check the spelling.
- Complete the citations, bring them in standard format.
Use the guidelines that your instructor requires (MLA, Chicago, APA, Turabian, etc.).
- Adjust margins, spacing, paragraph indentation, place of page numbers, etc.
- Standardize the bibliography or footnotes according to the guidelines.
- EndNote and EndNote Basic by UCLA Library Last Updated Jul 3, 2024 1921 views this year
- Zotero by UCLA Library Last Updated Jul 22, 2024 1232 views this year
(Based on English Composition 2 from Illinois Valley Community College):
- Weak organization
- Poor support and development of ideas
- Weak use of secondary sources
- Excessive errors
- Stylistic weakness
When collecting materials, selecting research topic, and writing the paper:
- Be systematic and organized (e.g. keep your bibliography neat and organized; write your notes in a neat way, so that you can find them later on.
- Use your critical thinking ability when you read.
- Write down your thoughts (so that you can reconstruct them later).
- Stop when you have a really good idea and think about whether you could enlarge it to a whole research paper. If yes, take much longer notes.
- When you write down a quotation or summarize somebody else's thoughts in your notes or in the paper, cite the source (i.e. write down the author, title, publication place, year, page number).
- If you quote or summarize a thought from the internet, cite the internet source.
- Write an outline that is detailed enough to remind you about the content.
- Read your paper for yourself or, preferably, somebody else.
- When you finish writing, check the spelling;
- Use the citation form (MLA, Chicago, or other) that your instructor requires and use it everywhere.
Plagiarism : somebody else's words or ideas presented without citation by an author
- Cite your source every time when you quote a part of somebody's work.
- Cite your source every time when you summarize a thought from somebody's work.
- Cite your source every time when you use a source (quote or summarize) from the Internet.
Consult the Citing Sources research guide for further details.
- << Previous: Writing a Research Proposal
- Next: Presenting the Research Paper >>
- Last Updated: Jul 24, 2024 1:23 PM
- URL: https://guides.library.ucla.edu/research-methods

Organizing Academic Research Papers: 6. The Methodology
- Purpose of Guide
- Design Flaws to Avoid
- Glossary of Research Terms
- Narrowing a Topic Idea
- Broadening a Topic Idea
- Extending the Timeliness of a Topic Idea
- Academic Writing Style
- Choosing a Title
- Making an Outline
- Paragraph Development
- Executive Summary
- Background Information
- The Research Problem/Question
- Theoretical Framework
- Citation Tracking
- Content Alert Services
- Evaluating Sources
- Primary Sources
- Secondary Sources
- Tertiary Sources
- What Is Scholarly vs. Popular?
- Qualitative Methods
- Quantitative Methods
- Using Non-Textual Elements
- Limitations of the Study
- Common Grammar Mistakes
- Avoiding Plagiarism
- Footnotes or Endnotes?
- Further Readings
- Annotated Bibliography
- Dealing with Nervousness
- Using Visual Aids
- Grading Someone Else's Paper
- How to Manage Group Projects
- Multiple Book Review Essay
- Reviewing Collected Essays
- About Informed Consent
- Writing Field Notes
- Writing a Policy Memo
- Writing a Research Proposal
- Acknowledgements
The methods section of a research paper provides the information by which a study’s validity is judged. The method section answers two main questions: 1) How was the data collected or generated? 2) How was it analyzed? The writing should be direct and precise and written in the past tense.
Importance of a Good Methodology Section
You must explain how you obtained and analyzed your results for the following reasons:
- Readers need to know how the data was obtained because the method you choose affects the results and, by extension, how you likely interpreted those results.
- Methodology is crucial for any branch of scholarship because an unreliable method produces unreliable results and it misappropriates interpretations of findings .
- In most cases, there are a variety of different methods you can choose to investigate a research problem. Your methodology section of your paper should make clear the reasons why you chose a particular method or procedure .
- The reader wants to know that the data was collected or generated in a way that is consistent with accepted practice in the field of study. For example, if you are using a questionnaire, readers need to know that it offered your respondents a reasonable range of answers to choose from.
- The research method must be appropriate to the objectives of the study . For example, be sure you have a large enough sample size to be able to generalize and make recommendations based upon the findings.
- The methodology should discuss the problems that were anticipated and the steps you took to prevent them from occurring . For any problems that did arise, you must describe the ways in which their impact was minimized or why these problems do not affect the findings in any way that impacts your interpretation of the data.
- Often in social science research, it is useful for other researchers to adapt or replicate your methodology. Therefore, it is important to always provide sufficient information to allow others to use or replicate the study . This information is particularly important when a new method had been developed or an innovative use of an existing method has been utilized.
Bem, Daryl J. Writing the Empirical Journal Article . Psychology Writing Center. University of Washington; Lunenburg, Frederick C. Writing a Successful Thesis or Dissertation: Tips and Strategies for Students in the Social and Behavioral Sciences . Thousand Oaks, CA: Corwin Press, 2008.
Structure and Writing Style
I. Groups of Research Methods
There are two main groups of research methods in the social sciences:
- The empirical-analytical group approaches the study of social sciences in a similar manner that researchers study the natural sciences. This type of research focuses on objective knowledge, research questions that can be answered yes or no, and operational definitions of variables to be measured. The empirical-analytical group employs deductive reasoning that uses existing theory as a foundation for hypotheses that need to be tested. This approach is focused on explanation .
- The interpretative group is focused on understanding phenomenon in a comprehensive, holistic way . This research method allows you to recognize your connection to the subject under study. Because the interpretative group focuses more on subjective knowledge, it requires careful interpretation of variables.
II. Content
An effectively written methodology section should:
- Introduce the overall methodological approach for investigating your research problem . Is your study qualitative or quantitative or a combination of both (mixed method)? Are you going to take a special approach, such as action research, or a more neutral stance?
- Indicate how the approach fits the overall research design . Your methods should have a clear connection with your research problem. In other words, make sure that your methods will actually address the problem. One of the most common deficiencies found in research papers is that the proposed methodology is unsuited to achieving the stated objective of your paper.
- Describe the specific methods of data collection you are going to use , such as, surveys, interviews, questionnaires, observation, archival research. If you are analyzing existing data, such as a data set or archival documents, describe how it was originally created or gathered and by whom.
- Explain how you intend to analyze your results . Will you use statistical analysis? Will you use specific theoretical perspectives to help you analyze a text or explain observed behaviors?
- Provide background and rationale for methodologies that are unfamiliar for your readers . Very often in the social sciences, research problems and the methods for investigating them require more explanation/rationale than widely accepted rules governing the natural and physical sciences. Be clear and concise in your explanation.
- Provide a rationale for subject selection and sampling procedure . For instance, if you propose to conduct interviews, how do you intend to select the sample population? If you are analyzing texts, which texts have you chosen, and why? If you are using statistics, why is this set of statisics being used? If other data sources exist, explain why the data you chose is most appropriate.
- Address potential limitations . Are there any practical limitations that could affect your data collection? How will you attempt to control for potential confounding variables and errors? If your methodology may lead to problems you can anticipate, state this openly and show why pursuing this methodology outweighs the risk of these problems cropping up.
NOTE : Once you have written all of the elements of the methods section, subsequent revisions should focus on how to present those elements as clearly and as logically as possibly. The description of how you prepared to study the research problem, how you gathered the data, and the protocol for analyzing the data should be organized chronologically. For clarity, when a large amount of detail must be presented, information should be presented in sub-sections according to topic.
III. Problems to Avoid
Irrelevant Detail The methodology section of your paper should be thorough but to the point. Don’t provide any background information that doesn’t directly help the reader to understand why a particular method was chosen, how the data was gathered or obtained, and how it was analyzed. Unnecessary Explanation of Basic Procedures Remember that you are not writing a how-to guide about a particular method. You should make the assumption that readers possess a basic understanding of how to investigate the research problem on their own and, therefore, you do not have to go into great detail about specific methodological procedures. The focus should be on how you applied a method , not on the mechanics of doing a method. NOTE: An exception to this rule is if you select an unconventional approach to doing the method; if this is the case, be sure to explain why this approach was chosen and how it enhances the overall research process. Problem Blindness It is almost a given that you will encounter problems when collecting or generating your data. Do not ignore these problems or pretend they did not occur. Often, documenting how you overcame obstacles can form an interesting part of the methodology. It demonstrates to the reader that you can provide a cogent rationale for the decisions you made to minimize the impact of any problems that arose. Literature Review Just as the literature review section of your paper provides an overview of sources you have examined while researching a particular topic, the methodology section should cite any sources that informed your choice and application of a particular method [i.e., the choice of a survey should include any citations to the works you used to help construct the survey].
It’s More than Sources of Information! A description of a research study's method should not be confused with a description of the sources of information. Such a list of sources is useful in itself, especially if it is accompanied by an explanation about the selection and use of the sources. The description of the project's methodology complements a list of sources in that it sets forth the organization and interpretation of information emanating from those sources.
Azevedo, L.F. et al. How to Write a Scientific Paper: Writing the Methods Section. Revista Portuguesa de Pneumologia 17 (2011): 232-238; Butin, Dan W. The Education Dissertation A Guide for Practitioner Scholars . Thousand Oaks, CA: Corwin, 2010; Carter, Susan. Structuring Your Research Thesis . New York: Palgrave Macmillan, 2012; Lunenburg, Frederick C. Writing a Successful Thesis or Dissertation: Tips and Strategies for Students in the Social and Behavioral Sciences . Thousand Oaks, CA: Corwin Press, 2008. Methods Section . The Writer’s Handbook. Writing Center. University of Wisconsin, Madison; Writing the Experimental Report: Methods, Results, and Discussion . The Writing Lab and The OWL. Purdue University; Methods and Materials . The Structure, Format, Content, and Style of a Journal-Style Scientific Paper. Department of Biology. Bates College.
Writing Tip
Statistical Designs and Tests? Do Not Fear Them!
Don't avoid using a quantitative approach to analyzing your research problem just because you fear the idea of applying statistical designs and tests. A qualitative approach, such as conducting interviews or content analysis of archival texts, can yield exciting new insights about a research problem, but it should not be undertaken simply because you have a disdain for running a simple regression. A well designed quantitative research study can often be accomplished in very clear and direct ways, whereas, a similar study of a qualitative nature usually requires considerable time to analyze large volumes of data and a tremendous burden to create new paths for analysis where previously no path associated with your research problem had existed.
Another Writing Tip
Knowing the Relationship Between Theories and Methods
There can be multiple meaning associated with the term "theories" and the term "methods" in social sciences research. A helpful way to delineate between them is to understand "theories" as representing different ways of characterizing the social world when you research it and "methods" as representing different ways of generating and analyzing data about that social world. Framed in this way, all empirical social sciences research involves theories and methods, whether they are stated explicitly or not. However, while theories and methods are often related, it is important that, as a researcher, you deliberately separate them in order to avoid your theories playing a disproportionate role in shaping what outcomes your chosen methods produce.
Introspectively engage in an ongoing dialectic between theories and methods to help enable you to use the outcomes from your methods to interrogate and develop new theories, or ways of framing conceptually the research problem. This is how scholarship grows and branches out into new intellectual territory.
Reynolds, R. Larry. Ways of Knowing. Alternative Microeconomics. Part 1, Chapter 3. Boise State University; The Theory-Method Relationship . S-Cool Revision. United Kingdom.
- << Previous: What Is Scholarly vs. Popular?
- Next: Qualitative Methods >>
- Last Updated: Jul 18, 2023 11:58 AM
- URL: https://library.sacredheart.edu/c.php?g=29803
- QuickSearch
- Library Catalog
- Databases A-Z
- Publication Finder
- Course Reserves
- Citation Linker
- Digital Commons
- Our Website
Research Support
- Ask a Librarian
- Appointments
- Interlibrary Loan (ILL)
- Research Guides
- Databases by Subject
- Citation Help
Using the Library
- Reserve a Group Study Room
- Renew Books
- Honors Study Rooms
- Off-Campus Access
- Library Policies
- Library Technology
User Information
- Grad Students
- Online Students
- COVID-19 Updates
- Staff Directory
- News & Announcements
- Library Newsletter
My Accounts
- Interlibrary Loan
- Staff Site Login

FIND US ON

15 Types of Research Methods
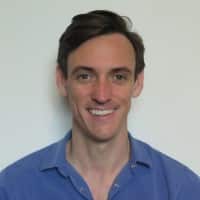
Chris Drew (PhD)
Dr. Chris Drew is the founder of the Helpful Professor. He holds a PhD in education and has published over 20 articles in scholarly journals. He is the former editor of the Journal of Learning Development in Higher Education. [Image Descriptor: Photo of Chris]
Learn about our Editorial Process
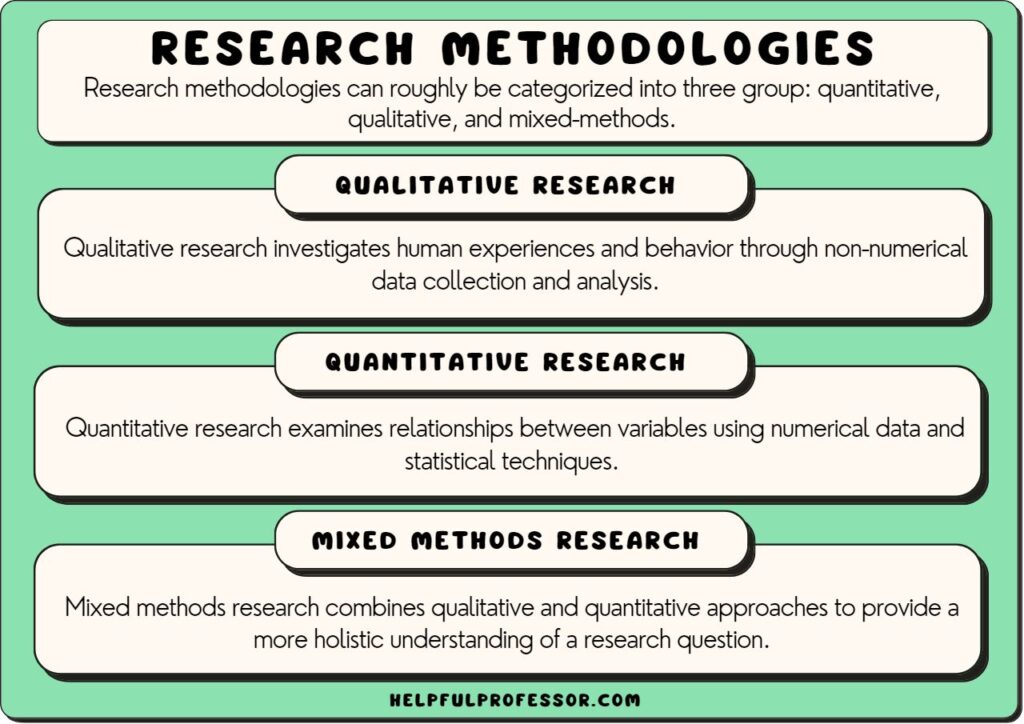
Research methods refer to the strategies, tools, and techniques used to gather and analyze data in a structured way in order to answer a research question or investigate a hypothesis (Hammond & Wellington, 2020).
Generally, we place research methods into two categories: quantitative and qualitative. Each has its own strengths and weaknesses, which we can summarize as:
- Quantitative research can achieve generalizability through scrupulous statistical analysis applied to large sample sizes.
- Qualitative research achieves deep, detailed, and nuance accounts of specific case studies, which are not generalizable.
Some researchers, with the aim of making the most of both quantitative and qualitative research, employ mixed methods, whereby they will apply both types of research methods in the one study, such as by conducting a statistical survey alongside in-depth interviews to add context to the quantitative findings.
Below, I’ll outline 15 common research methods, and include pros, cons, and examples of each .
Types of Research Methods
Research methods can be broadly categorized into two types: quantitative and qualitative.
- Quantitative methods involve systematic empirical investigation of observable phenomena via statistical, mathematical, or computational techniques, providing an in-depth understanding of a specific concept or phenomenon (Schweigert, 2021). The strengths of this approach include its ability to produce reliable results that can be generalized to a larger population, although it can lack depth and detail.
- Qualitative methods encompass techniques that are designed to provide a deep understanding of a complex issue, often in a specific context, through collection of non-numerical data (Tracy, 2019). This approach often provides rich, detailed insights but can be time-consuming and its findings may not be generalizable.
These can be further broken down into a range of specific research methods and designs:
Primarily Quantitative Methods | Primarily Qualitative methods |
---|---|
Experimental Research | Case Study |
Surveys and Questionnaires | Ethnography |
Longitudinal Studies | Phenomenology |
Cross-Sectional Studies | Historical research |
Correlational Research | Content analysis |
Causal-Comparative Research | Grounded theory |
Meta-Analysis | Action research |
Quasi-Experimental Design | Observational research |
Combining the two methods above, mixed methods research mixes elements of both qualitative and quantitative research methods, providing a comprehensive understanding of the research problem . We can further break these down into:
- Sequential Explanatory Design (QUAN→QUAL): This methodology involves conducting quantitative analysis first, then supplementing it with a qualitative study.
- Sequential Exploratory Design (QUAL→QUAN): This methodology goes in the other direction, starting with qualitative analysis and ending with quantitative analysis.
Let’s explore some methods and designs from both quantitative and qualitative traditions, starting with qualitative research methods.
Qualitative Research Methods
Qualitative research methods allow for the exploration of phenomena in their natural settings, providing detailed, descriptive responses and insights into individuals’ experiences and perceptions (Howitt, 2019).
These methods are useful when a detailed understanding of a phenomenon is sought.
1. Ethnographic Research
Ethnographic research emerged out of anthropological research, where anthropologists would enter into a setting for a sustained period of time, getting to know a cultural group and taking detailed observations.
Ethnographers would sometimes even act as participants in the group or culture, which many scholars argue is a weakness because it is a step away from achieving objectivity (Stokes & Wall, 2017).
In fact, at its most extreme version, ethnographers even conduct research on themselves, in a fascinating methodology call autoethnography .
The purpose is to understand the culture, social structure, and the behaviors of the group under study. It is often useful when researchers seek to understand shared cultural meanings and practices in their natural settings.
However, it can be time-consuming and may reflect researcher biases due to the immersion approach.
Pros of Ethnographic Research | Cons of Ethnographic Research |
---|---|
1. Provides deep cultural insights | 1. Time-consuming |
2. Contextually relevant findings | 2. Potential researcher bias |
3. Explores dynamic social processes | 3. May |
Example of Ethnography
Liquidated: An Ethnography of Wall Street by Karen Ho involves an anthropologist who embeds herself with Wall Street firms to study the culture of Wall Street bankers and how this culture affects the broader economy and world.
2. Phenomenological Research
Phenomenological research is a qualitative method focused on the study of individual experiences from the participant’s perspective (Tracy, 2019).
It focuses specifically on people’s experiences in relation to a specific social phenomenon ( see here for examples of social phenomena ).
This method is valuable when the goal is to understand how individuals perceive, experience, and make meaning of particular phenomena. However, because it is subjective and dependent on participants’ self-reports, findings may not be generalizable, and are highly reliant on self-reported ‘thoughts and feelings’.
Pros of Phenomenological Research | Cons of Phenomenological Research |
---|---|
1. Provides rich, detailed data | 1. Limited generalizability |
2. Highlights personal experience and perceptions | 2. Data collection can be time-consuming |
3. Allows exploration of complex phenomena | 3. Requires highly skilled researchers |
Example of Phenomenological Research
A phenomenological approach to experiences with technology by Sebnem Cilesiz represents a good starting-point for formulating a phenomenological study. With its focus on the ‘essence of experience’, this piece presents methodological, reliability, validity, and data analysis techniques that phenomenologists use to explain how people experience technology in their everyday lives.
3. Historical Research
Historical research is a qualitative method involving the examination of past events to draw conclusions about the present or make predictions about the future (Stokes & Wall, 2017).
As you might expect, it’s common in the research branches of history departments in universities.
This approach is useful in studies that seek to understand the past to interpret present events or trends. However, it relies heavily on the availability and reliability of source materials, which may be limited.
Common data sources include cultural artifacts from both material and non-material culture , which are then examined, compared, contrasted, and contextualized to test hypotheses and generate theories.
Pros of Historical Research | Cons of Historical Research |
---|---|
1. | 1. Dependent on available sources |
2. Can help understand current events or trends | 2. Potential bias in source materials |
3. Allows the study of change over time | 3. Difficult to replicate |
Example of Historical Research
A historical research example might be a study examining the evolution of gender roles over the last century. This research might involve the analysis of historical newspapers, advertisements, letters, and company documents, as well as sociocultural contexts.
4. Content Analysis
Content analysis is a research method that involves systematic and objective coding and interpreting of text or media to identify patterns, themes, ideologies, or biases (Schweigert, 2021).
A content analysis is useful in analyzing communication patterns, helping to reveal how texts such as newspapers, movies, films, political speeches, and other types of ‘content’ contain narratives and biases.
However, interpretations can be very subjective, which often requires scholars to engage in practices such as cross-comparing their coding with peers or external researchers.
Content analysis can be further broken down in to other specific methodologies such as semiotic analysis, multimodal analysis , and discourse analysis .
Pros of Content Analysis | Cons of Content Analysis |
---|---|
1. Unobtrusive data collection | 1. Lacks contextual information |
2. Allows for large sample analysis | 2. Potential coder bias |
3. Replicable and reliable if done properly | 3. May overlook nuances |
Example of Content Analysis
How is Islam Portrayed in Western Media? by Poorebrahim and Zarei (2013) employs a type of content analysis called critical discourse analysis (common in poststructuralist and critical theory research ). This study by Poorebrahum and Zarei combs through a corpus of western media texts to explore the language forms that are used in relation to Islam and Muslims, finding that they are overly stereotyped, which may represent anti-Islam bias or failure to understand the Islamic world.
5. Grounded Theory Research
Grounded theory involves developing a theory during and after data collection rather than beforehand.
This is in contrast to most academic research studies, which start with a hypothesis or theory and then testing of it through a study, where we might have a null hypothesis (disproving the theory) and an alternative hypothesis (supporting the theory).
Grounded Theory is useful because it keeps an open mind to what the data might reveal out of the research. It can be time-consuming and requires rigorous data analysis (Tracy, 2019).
Pros of Grounded Theory Research | Cons of Grounded Theory Research |
---|---|
1. Helps with theory development | 1. Time-consuming |
2. Rigorous data analysis | 2. Requires iterative data collection and analysis |
3. Can fill gaps in existing theories | 3. Requires skilled researchers |
Grounded Theory Example
Developing a Leadership Identity by Komives et al (2005) employs a grounded theory approach to develop a thesis based on the data rather than testing a hypothesis. The researchers studied the leadership identity of 13 college students taking on leadership roles. Based on their interviews, the researchers theorized that the students’ leadership identities shifted from a hierarchical view of leadership to one that embraced leadership as a collaborative concept.
6. Action Research
Action research is an approach which aims to solve real-world problems and bring about change within a setting. The study is designed to solve a specific problem – or in other words, to take action (Patten, 2017).
This approach can involve mixed methods, but is generally qualitative because it usually involves the study of a specific case study wherein the researcher works, e.g. a teacher studying their own classroom practice to seek ways they can improve.
Action research is very common in fields like education and nursing where practitioners identify areas for improvement then implement a study in order to find paths forward.
Pros of Action Research | Cons of Action Research |
---|---|
1. Addresses real-world problems and seeks to find solutions. | 1. It is time-consuming and often hard to implement into a practitioner’s already busy schedule |
2. Integrates research and action in an action-research cycle. | 2. Requires collaboration between researcher, practitioner, and research participants. |
3. Can bring about positive change in isolated instances, such as in a school or nursery setting. | 3. Complexity of managing dual roles (where the researcher is also often the practitioner) |
Action Research Example
Using Digital Sandbox Gaming to Improve Creativity Within Boys’ Writing by Ellison and Drew was a research study one of my research students completed in his own classroom under my supervision. He implemented a digital game-based approach to literacy teaching with boys and interviewed his students to see if the use of games as stimuli for storytelling helped draw them into the learning experience.
7. Natural Observational Research
Observational research can also be quantitative (see: experimental research), but in naturalistic settings for the social sciences, researchers tend to employ qualitative data collection methods like interviews and field notes to observe people in their day-to-day environments.
This approach involves the observation and detailed recording of behaviors in their natural settings (Howitt, 2019). It can provide rich, in-depth information, but the researcher’s presence might influence behavior.
While observational research has some overlaps with ethnography (especially in regard to data collection techniques), it tends not to be as sustained as ethnography, e.g. a researcher might do 5 observations, every second Monday, as opposed to being embedded in an environment.
Pros of Qualitative Observational Research | Cons of Qualitative Observational Research |
---|---|
1. Captures behavior in natural settings, allowing for interesting insights into authentic behaviors. | 1. Researcher’s presence may influence behavior |
2. Can provide rich, detailed data through the researcher’s vignettes. | 2. Can be time-consuming |
3. Non-invasive because researchers want to observe natural activities rather than interfering with research participants. | 3. Requires skilled and trained observers |
Observational Research Example
A researcher might use qualitative observational research to study the behaviors and interactions of children at a playground. The researcher would document the behaviors observed, such as the types of games played, levels of cooperation , and instances of conflict.
8. Case Study Research
Case study research is a qualitative method that involves a deep and thorough investigation of a single individual, group, or event in order to explore facets of that phenomenon that cannot be captured using other methods (Stokes & Wall, 2017).
Case study research is especially valuable in providing contextualized insights into specific issues, facilitating the application of abstract theories to real-world situations (Patten, 2017).
However, findings from a case study may not be generalizable due to the specific context and the limited number of cases studied (Walliman, 2021).
Pros of Case Study Research | Cons of Case Study Research |
---|---|
1. Provides detailed insights | 1. Limited generalizability |
2. Facilitates the study of complex phenomena | 2. Can be time-consuming |
3. Can test or generate theories | 3. Subject to observer bias |
See More: Case Study Advantages and Disadvantages
Example of a Case Study
Scholars conduct a detailed exploration of the implementation of a new teaching method within a classroom setting. The study focuses on how the teacher and students adapt to the new method, the challenges encountered, and the outcomes on student performance and engagement. While the study provides specific and detailed insights of the teaching method in that classroom, it cannot be generalized to other classrooms, as statistical significance has not been established through this qualitative approach.
Quantitative Research Methods
Quantitative research methods involve the systematic empirical investigation of observable phenomena via statistical, mathematical, or computational techniques (Pajo, 2022). The focus is on gathering numerical data and generalizing it across groups of people or to explain a particular phenomenon.
9. Experimental Research
Experimental research is a quantitative method where researchers manipulate one variable to determine its effect on another (Walliman, 2021).
This is common, for example, in high-school science labs, where students are asked to introduce a variable into a setting in order to examine its effect.
This type of research is useful in situations where researchers want to determine causal relationships between variables. However, experimental conditions may not reflect real-world conditions.
Pros of Experimental Research | Cons of Experimental Research |
---|---|
1. Allows for determination of causality | 1. Might not reflect real-world conditions |
2. Allows for the study of phenomena in highly controlled environments to minimize research contamination. | 2. Can be costly and time-consuming to create a controlled environment. |
3. Can be replicated so other researchers can test and verify the results. | 3. Ethical concerns need to be addressed as the research is directly manipulating variables. |
Example of Experimental Research
A researcher may conduct an experiment to determine the effects of a new educational approach on student learning outcomes. Students would be randomly assigned to either the control group (traditional teaching method) or the experimental group (new educational approach).
10. Surveys and Questionnaires
Surveys and questionnaires are quantitative methods that involve asking research participants structured and predefined questions to collect data about their attitudes, beliefs, behaviors, or characteristics (Patten, 2017).
Surveys are beneficial for collecting data from large samples, but they depend heavily on the honesty and accuracy of respondents.
They tend to be seen as more authoritative than their qualitative counterparts, semi-structured interviews, because the data is quantifiable (e.g. a questionnaire where information is presented on a scale from 1 to 10 can allow researchers to determine and compare statistical means, averages, and variations across sub-populations in the study).
Pros of Surveys and Questionnaires | Cons of Surveys and Questionnaires |
---|---|
1. Data can be gathered from larger samples than is possible in qualitative research. | 1. There is heavy dependence on respondent honesty |
2. The data is quantifiable, allowing for comparison across subpopulations | 2. There is limited depth of response as opposed to qualitative approaches. |
3. Can be cost-effective and time-efficient | 3. Static with no flexibility to explore responses (unlike semi- or unstrcutured interviewing) |
Example of a Survey Study
A company might use a survey to gather data about employee job satisfaction across its offices worldwide. Employees would be asked to rate various aspects of their job satisfaction on a Likert scale. While this method provides a broad overview, it may lack the depth of understanding possible with other methods (Stokes & Wall, 2017).
11. Longitudinal Studies
Longitudinal studies involve repeated observations of the same variables over extended periods (Howitt, 2019). These studies are valuable for tracking development and change but can be costly and time-consuming.
With multiple data points collected over extended periods, it’s possible to examine continuous changes within things like population dynamics or consumer behavior. This makes a detailed analysis of change possible.
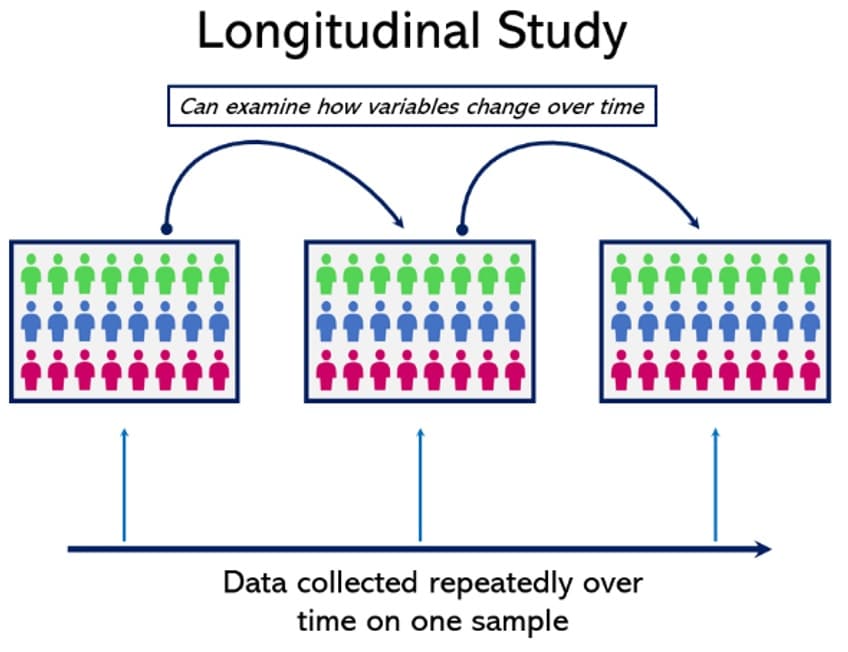
Perhaps the most relatable example of a longitudinal study is a national census, which is taken on the same day every few years, to gather comparative demographic data that can show how a nation is changing over time.
While longitudinal studies are commonly quantitative, there are also instances of qualitative ones as well, such as the famous 7 Up study from the UK, which studies 14 individuals every 7 years to explore their development over their lives.
Pros of Longitudinal Studies | Cons of Longitudinal Studies |
---|---|
1. Tracks changes over time allowing for comparison of past to present events. | 1. Is almost by definition time-consuming because time needs to pass between each data collection session. |
2. Can identify sequences of events, but causality is often harder to determine. | 2. There is high risk of participant dropout over time as participants move on with their lives. |
Example of a Longitudinal Study
A national census, taken every few years, uses surveys to develop longitudinal data, which is then compared and analyzed to present accurate trends over time. Trends a census can reveal include changes in religiosity, values and attitudes on social issues, and much more.
12. Cross-Sectional Studies
Cross-sectional studies are a quantitative research method that involves analyzing data from a population at a specific point in time (Patten, 2017). They provide a snapshot of a situation but cannot determine causality.
This design is used to measure and compare the prevalence of certain characteristics or outcomes in different groups within the sampled population.
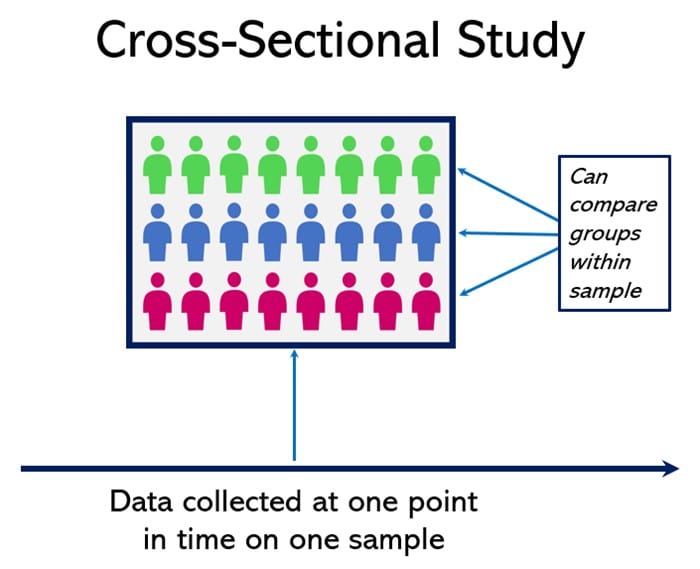
The major advantage of cross-sectional design is its ability to measure a wide range of variables simultaneously without needing to follow up with participants over time.
However, cross-sectional studies do have limitations . This design can only show if there are associations or correlations between different variables, but cannot prove cause and effect relationships, temporal sequence, changes, and trends over time.
Pros of Cross-Sectional Studies | Cons of Cross-Sectional Studies |
---|---|
1. Quick and inexpensive, with no long-term commitment required. | 1. Cannot determine causality because it is a simple snapshot, with no time delay between data collection points. |
2. Good for descriptive analyses. | 2. Does not allow researchers to follow up with research participants. |
Example of a Cross-Sectional Study
Our longitudinal study example of a national census also happens to contain cross-sectional design. One census is cross-sectional, displaying only data from one point in time. But when a census is taken once every few years, it becomes longitudinal, and so long as the data collection technique remains unchanged, identification of changes will be achievable, adding another time dimension on top of a basic cross-sectional study.
13. Correlational Research
Correlational research is a quantitative method that seeks to determine if and to what degree a relationship exists between two or more quantifiable variables (Schweigert, 2021).
This approach provides a fast and easy way to make initial hypotheses based on either positive or negative correlation trends that can be observed within dataset.
While correlational research can reveal relationships between variables, it cannot establish causality.
Methods used for data analysis may include statistical correlations such as Pearson’s or Spearman’s.
Pros of Correlational Research | Cons of Correlational Research |
---|---|
1. Reveals relationships between variables | 1. Cannot determine causality |
2. Can use existing data | 2. May be |
3. Can guide further experimental research | 3. Correlation may be coincidental |
Example of Correlational Research
A team of researchers is interested in studying the relationship between the amount of time students spend studying and their academic performance. They gather data from a high school, measuring the number of hours each student studies per week and their grade point averages (GPAs) at the end of the semester. Upon analyzing the data, they find a positive correlation, suggesting that students who spend more time studying tend to have higher GPAs.
14. Quasi-Experimental Design Research
Quasi-experimental design research is a quantitative research method that is similar to experimental design but lacks the element of random assignment to treatment or control.
Instead, quasi-experimental designs typically rely on certain other methods to control for extraneous variables.
The term ‘quasi-experimental’ implies that the experiment resembles a true experiment, but it is not exactly the same because it doesn’t meet all the criteria for a ‘true’ experiment, specifically in terms of control and random assignment.
Quasi-experimental design is useful when researchers want to study a causal hypothesis or relationship, but practical or ethical considerations prevent them from manipulating variables and randomly assigning participants to conditions.
Pros | Cons |
---|---|
1. It’s more feasible to implement than true experiments. | 1. Without random assignment, it’s harder to rule out confounding variables. |
2. It can be conducted in real-world settings, making the findings more applicable to the real world. | 2. The lack of random assignment may of the study. |
3. Useful when it’s unethical or impossible to manipulate the independent variable or randomly assign participants. | 3. It’s more difficult to establish a cause-effect relationship due to the potential for confounding variables. |
Example of Quasi-Experimental Design
A researcher wants to study the impact of a new math tutoring program on student performance. However, ethical and practical constraints prevent random assignment to the “tutoring” and “no tutoring” groups. Instead, the researcher compares students who chose to receive tutoring (experimental group) to similar students who did not choose to receive tutoring (control group), controlling for other variables like grade level and previous math performance.
Related: Examples and Types of Random Assignment in Research
15. Meta-Analysis Research
Meta-analysis statistically combines the results of multiple studies on a specific topic to yield a more precise estimate of the effect size. It’s the gold standard of secondary research .
Meta-analysis is particularly useful when there are numerous studies on a topic, and there is a need to integrate the findings to draw more reliable conclusions.
Some meta-analyses can identify flaws or gaps in a corpus of research, when can be highly influential in academic research, despite lack of primary data collection.
However, they tend only to be feasible when there is a sizable corpus of high-quality and reliable studies into a phenomenon.
Pros | Cons |
---|---|
Increased Statistical Power: By combining data from multiple studies, meta-analysis increases the statistical power to detect effects. | Publication Bias: Studies with null or negative findings are less likely to be published, leading to an overestimation of effect sizes. |
Greater Precision: It provides more precise estimates of effect sizes by reducing the influence of random error. | Quality of Studies: of a meta-analysis depends on the quality of the studies included. |
Resolving Discrepancies: Meta-analysis can help resolve disagreements between different studies on a topic. | Heterogeneity: Differences in study design, sample, or procedures can introduce heterogeneity, complicating interpretation of results. |
Example of a Meta-Analysis
The power of feedback revisited (Wisniewski, Zierer & Hattie, 2020) is a meta-analysis that examines 435 empirical studies research on the effects of feedback on student learning. They use a random-effects model to ascertain whether there is a clear effect size across the literature. The authors find that feedback tends to impact cognitive and motor skill outcomes but has less of an effect on motivational and behavioral outcomes.
Choosing a research method requires a lot of consideration regarding what you want to achieve, your research paradigm, and the methodology that is most valuable for what you are studying. There are multiple types of research methods, many of which I haven’t been able to present here. Generally, it’s recommended that you work with an experienced researcher or research supervisor to identify a suitable research method for your study at hand.
Hammond, M., & Wellington, J. (2020). Research methods: The key concepts . New York: Routledge.
Howitt, D. (2019). Introduction to qualitative research methods in psychology . London: Pearson UK.
Pajo, B. (2022). Introduction to research methods: A hands-on approach . New York: Sage Publications.
Patten, M. L. (2017). Understanding research methods: An overview of the essentials . New York: Sage
Schweigert, W. A. (2021). Research methods in psychology: A handbook . Los Angeles: Waveland Press.
Stokes, P., & Wall, T. (2017). Research methods . New York: Bloomsbury Publishing.
Tracy, S. J. (2019). Qualitative research methods: Collecting evidence, crafting analysis, communicating impact . London: John Wiley & Sons.
Walliman, N. (2021). Research methods: The basics. London: Routledge.
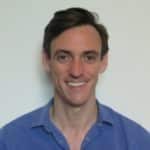
- Chris Drew (PhD) https://helpfulprofessor.com/author/chris-drew-phd-2/ 25 Number Games for Kids (Free and Easy)
- Chris Drew (PhD) https://helpfulprofessor.com/author/chris-drew-phd-2/ 25 Word Games for Kids (Free and Easy)
- Chris Drew (PhD) https://helpfulprofessor.com/author/chris-drew-phd-2/ 25 Outdoor Games for Kids
- Chris Drew (PhD) https://helpfulprofessor.com/author/chris-drew-phd-2/ 50 Incentives to Give to Students
Leave a Comment Cancel Reply
Your email address will not be published. Required fields are marked *
Educational resources and simple solutions for your research journey
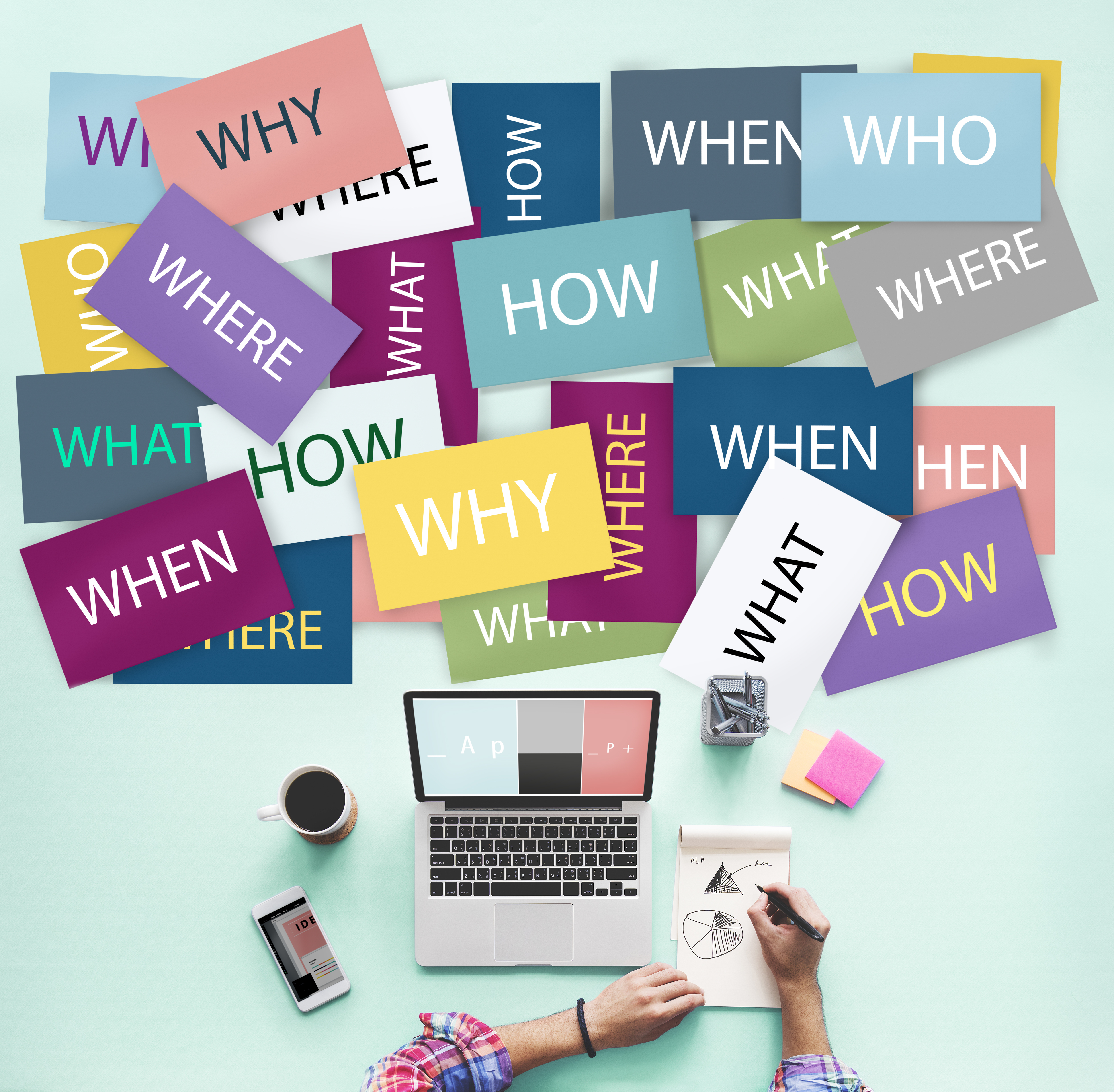
How to Write the Methods Section of a Research Paper
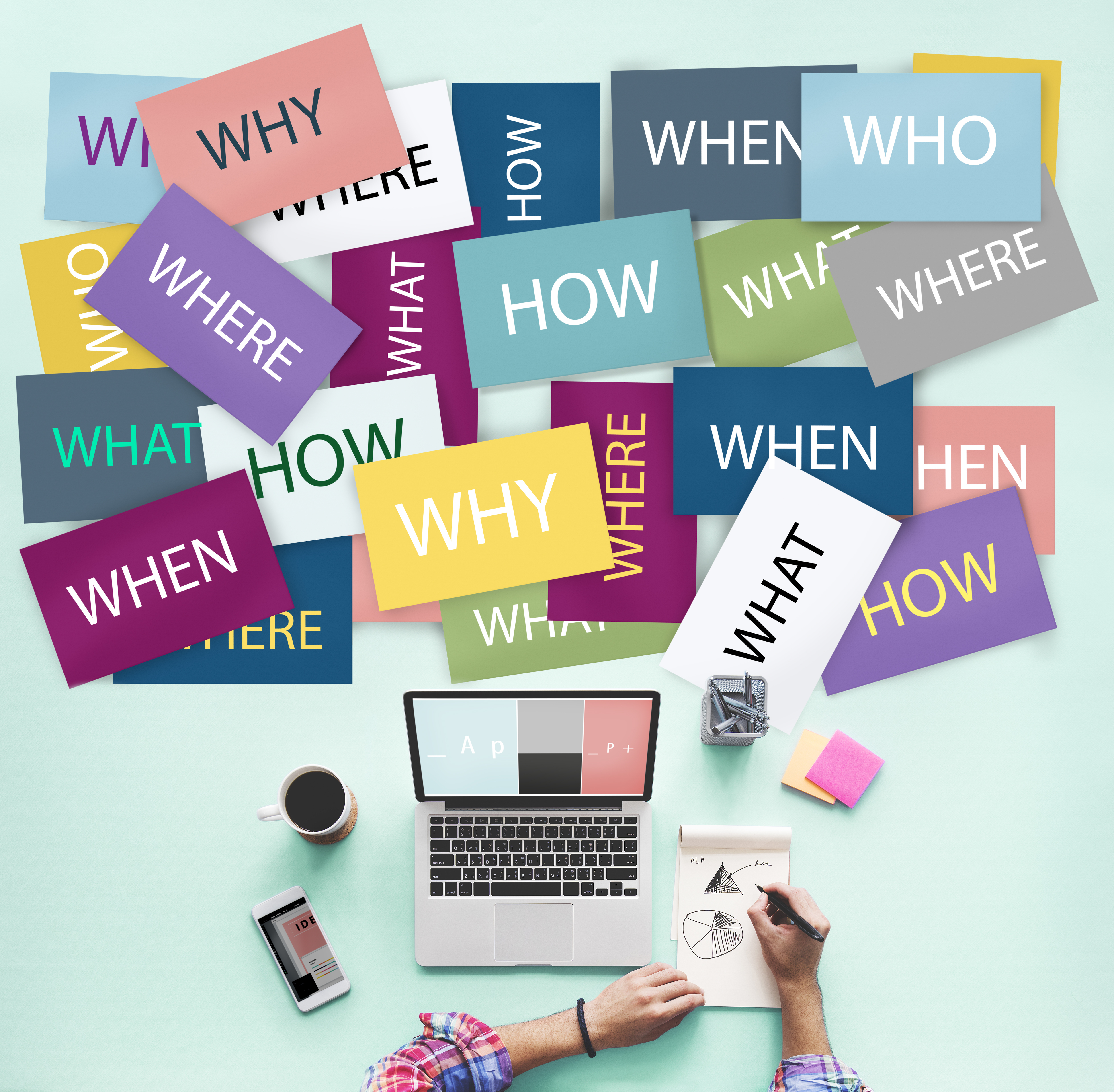
Writing a research paper is both an art and a skill, and knowing how to write the methods section of a research paper is the first crucial step in mastering scientific writing. If, like the majority of early career researchers, you believe that the methods section is the simplest to write and needs little in the way of careful consideration or thought, this article will help you understand it is not 1 .
We have all probably asked our supervisors, coworkers, or search engines “ how to write a methods section of a research paper ” at some point in our scientific careers, so you are not alone if that’s how you ended up here. Even for seasoned researchers, selecting what to include in the methods section from a wealth of experimental information can occasionally be a source of distress and perplexity.
Additionally, journal specifications, in some cases, may make it more of a requirement rather than a choice to provide a selective yet descriptive account of the experimental procedure. Hence, knowing these nuances of how to write the methods section of a research paper is critical to its success. The methods section of the research paper is not supposed to be a detailed heavy, dull section that some researchers tend to write; rather, it should be the central component of the study that justifies the validity and reliability of the research.
Are you still unsure of how the methods section of a research paper forms the basis of every investigation? Consider the last article you read but ignore the methods section and concentrate on the other parts of the paper . Now think whether you could repeat the study and be sure of the credibility of the findings despite knowing the literature review and even having the data in front of you. You have the answer!

Having established the importance of the methods section , the next question is how to write the methods section of a research paper that unifies the overall study. The purpose of the methods section , which was earlier called as Materials and Methods , is to describe how the authors went about answering the “research question” at hand. Here, the objective is to tell a coherent story that gives a detailed account of how the study was conducted, the rationale behind specific experimental procedures, the experimental setup, objects (variables) involved, the research protocol employed, tools utilized to measure, calculations and measurements, and the analysis of the collected data 2 .
In this article, we will take a deep dive into this topic and provide a detailed overview of how to write the methods section of a research paper . For the sake of clarity, we have separated the subject into various sections with corresponding subheadings.
Table of Contents
What is the methods section of a research paper ?
The methods section is a fundamental section of any paper since it typically discusses the ‘ what ’, ‘ how ’, ‘ which ’, and ‘ why ’ of the study, which is necessary to arrive at the final conclusions. In a research article, the introduction, which serves to set the foundation for comprehending the background and results is usually followed by the methods section, which precedes the result and discussion sections. The methods section must explicitly state what was done, how it was done, which equipment, tools and techniques were utilized, how were the measurements/calculations taken, and why specific research protocols, software, and analytical methods were employed.
Why is the methods section important?
The primary goal of the methods section is to provide pertinent details about the experimental approach so that the reader may put the results in perspective and, if necessary, replicate the findings 3 . This section offers readers the chance to evaluate the reliability and validity of any study. In short, it also serves as the study’s blueprint, assisting researchers who might be unsure about any other portion in establishing the study’s context and validity. The methods plays a rather crucial role in determining the fate of the article; an incomplete and unreliable methods section can frequently result in early rejections and may lead to numerous rounds of modifications during the publication process. This means that the reviewers also often use methods section to assess the reliability and validity of the research protocol and the data analysis employed to address the research topic. In other words, the purpose of the methods section is to demonstrate the research acumen and subject-matter expertise of the author(s) in their field.
Structure of methods section of a research paper
Similar to the research paper, the methods section also follows a defined structure; this may be dictated by the guidelines of a specific journal or can be presented in a chronological or thematic manner based on the study type. When writing the methods section , authors should keep in mind that they are telling a story about how the research was conducted. They should only report relevant information to avoid confusing the reader and include details that would aid in connecting various aspects of the entire research activity together. It is generally advisable to present experiments in the order in which they were conducted. This facilitates the logical flow of the research and allows readers to follow the progression of the study design.
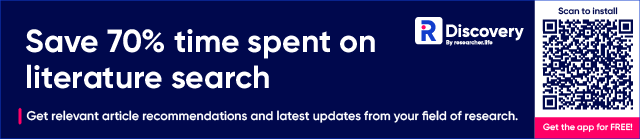
It is also essential to clearly state the rationale behind each experiment and how the findings of earlier experiments informed the design or interpretation of later experiments. This allows the readers to understand the overall purpose of the study design and the significance of each experiment within that context. However, depending on the particular research question and method, it may make sense to present information in a different order; therefore, authors must select the best structure and strategy for their individual studies.
In cases where there is a lot of information, divide the sections into subheadings to cover the pertinent details. If the journal guidelines pose restrictions on the word limit , additional important information can be supplied in the supplementary files. A simple rule of thumb for sectioning the method section is to begin by explaining the methodological approach ( what was done ), describing the data collection methods ( how it was done ), providing the analysis method ( how the data was analyzed ), and explaining the rationale for choosing the methodological strategy. This is described in detail in the upcoming sections.
How to write the methods section of a research paper
Contrary to widespread assumption, the methods section of a research paper should be prepared once the study is complete to prevent missing any key parameter. Hence, please make sure that all relevant experiments are done before you start writing a methods section . The next step for authors is to look up any applicable academic style manuals or journal-specific standards to ensure that the methods section is formatted correctly. The methods section of a research paper typically constitutes materials and methods; while writing this section, authors usually arrange the information under each category.
The materials category describes the samples, materials, treatments, and instruments, while experimental design, sample preparation, data collection, and data analysis are a part of the method category. According to the nature of the study, authors should include additional subsections within the methods section, such as ethical considerations like the declaration of Helsinki (for studies involving human subjects), demographic information of the participants, and any other crucial information that can affect the output of the study. Simply put, the methods section has two major components: content and format. Here is an easy checklist for you to consider if you are struggling with how to write the methods section of a research paper .
- Explain the research design, subjects, and sample details
- Include information on inclusion and exclusion criteria
- Mention ethical or any other permission required for the study
- Include information about materials, experimental setup, tools, and software
- Add details of data collection and analysis methods
- Incorporate how research biases were avoided or confounding variables were controlled
- Evaluate and justify the experimental procedure selected to address the research question
- Provide precise and clear details of each experiment
- Flowcharts, infographics, or tables can be used to present complex information
- Use past tense to show that the experiments have been done
- Follow academic style guides (such as APA or MLA ) to structure the content
- Citations should be included as per standard protocols in the field
Now that you know how to write the methods section of a research paper , let’s address another challenge researchers face while writing the methods section —what to include in the methods section . How much information is too much is not always obvious when it comes to trying to include data in the methods section of a paper. In the next section, we examine this issue and explore potential solutions.
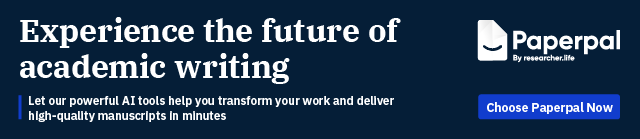
What to include in the methods section of a research paper
The technical nature of the methods section occasionally makes it harder to present the information clearly and concisely while staying within the study context. Many young researchers tend to veer off subject significantly, and they frequently commit the sin of becoming bogged down in itty bitty details, making the text harder to read and impairing its overall flow. However, the best way to write the methods section is to start with crucial components of the experiments. If you have trouble deciding which elements are essential, think about leaving out those that would make it more challenging to comprehend the context or replicate the results. The top-down approach helps to ensure all relevant information is incorporated and vital information is not lost in technicalities. Next, remember to add details that are significant to assess the validity and reliability of the study. Here is a simple checklist for you to follow ( bonus tip: you can also make a checklist for your own study to avoid missing any critical information while writing the methods section ).
- Structuring the methods section : Authors should diligently follow journal guidelines and adhere to the specific author instructions provided when writing the methods section . Journals typically have specific guidelines for formatting the methods section ; for example, Frontiers in Plant Sciences advises arranging the materials and methods section by subheading and citing relevant literature. There are several standardized checklists available for different study types in the biomedical field, including CONSORT (Consolidated Standards of Reporting Trials) for randomized clinical trials, PRISMA (Preferred Reporting Items for Systematic reviews and Meta-Analysis) for systematic reviews and meta-analysis, and STROBE (STrengthening the Reporting of OBservational studies in Epidemiology) for cohort, case-control, cross-sectional studies. Before starting the methods section , check the checklist available in your field that can function as a guide.
- Organizing different sections to tell a story : Once you are sure of the format required for structuring the methods section , the next is to present the sections in a logical manner; as mentioned earlier, the sections can be organized according to the chronology or themes. In the chronological arrangement, you should discuss the methods in accordance with how the experiments were carried out. An example of the method section of a research paper of an animal study should first ideally include information about the species, weight, sex, strain, and age. Next, the number of animals, their initial conditions, and their living and housing conditions should also be mentioned. Second, how the groups are assigned and the intervention (drug treatment, stress, or other) given to each group, and finally, the details of tools and techniques used to measure, collect, and analyze the data. Experiments involving animal or human subjects should additionally state an ethics approval statement. It is best to arrange the section using the thematic approach when discussing distinct experiments not following a sequential order.
- Define and explain the objects and procedure: Experimental procedure should clearly be stated in the methods section . Samples, necessary preparations (samples, treatment, and drug), and methods for manipulation need to be included. All variables (control, dependent, independent, and confounding) must be clearly defined, particularly if the confounding variables can affect the outcome of the study.
- Match the order of the methods section with the order of results: Though not mandatory, organizing the manuscript in a logical and coherent manner can improve the readability and clarity of the paper. This can be done by following a consistent structure throughout the manuscript; readers can easily navigate through the different sections and understand the methods and results in relation to each other. Using experiment names as headings for both the methods and results sections can also make it simpler for readers to locate specific information and corroborate it if needed.
- Relevant information must always be included: The methods section should have information on all experiments conducted and their details clearly mentioned. Ask the journal whether there is a way to offer more information in the supplemental files or external repositories if your target journal has strict word limitations. For example, Nature communications encourages authors to deposit their step-by-step protocols in an open-resource depository, Protocol Exchange which allows the protocols to be linked with the manuscript upon publication. Providing access to detailed protocols also helps to increase the transparency and reproducibility of the research.
- It’s all in the details: The methods section should meticulously list all the materials, tools, instruments, and software used for different experiments. Specify the testing equipment on which data was obtained, together with its manufacturer’s information, location, city, and state or any other stimuli used to manipulate the variables. Provide specifics on the research process you employed; if it was a standard protocol, cite previous studies that also used the protocol. Include any protocol modifications that were made, as well as any other factors that were taken into account when planning the study or gathering data. Any new or modified techniques should be explained by the authors. Typically, readers evaluate the reliability and validity of the procedures using the cited literature, and a widely accepted checklist helps to support the credibility of the methodology. Note: Authors should include a statement on sample size estimation (if applicable), which is often missed. It enables the reader to determine how many subjects will be required to detect the expected change in the outcome variables within a given confidence interval.
- Write for the audience: While explaining the details in the methods section , authors should be mindful of their target audience, as some of the rationale or assumptions on which specific procedures are based might not always be obvious to the audience, particularly for a general audience. Therefore, when in doubt, the objective of a procedure should be specified either in relation to the research question or to the entire protocol.
- Data interpretation and analysis : Information on data processing, statistical testing, levels of significance, and analysis tools and software should be added. Mention if the recommendations and expertise of an experienced statistician were followed. Also, evaluate and justify the preferred statistical method used in the study and its significance.
What NOT to include in the methods section of a research paper
To address “ how to write the methods section of a research paper ”, authors should not only pay careful attention to what to include but also what not to include in the methods section of a research paper . Here is a list of do not’s when writing the methods section :
- Do not elaborate on specifics of standard methods/procedures: You should refrain from adding unnecessary details of experiments and practices that are well established and cited previously. Instead, simply cite relevant literature or mention if the manufacturer’s protocol was followed.
- Do not add unnecessary details : Do not include minute details of the experimental procedure and materials/instruments used that are not significant for the outcome of the experiment. For example, there is no need to mention the brand name of the water bath used for incubation.
- Do not discuss the results: The methods section is not to discuss the results or refer to the tables and figures; save it for the results and discussion section. Also, focus on the methods selected to conduct the study and avoid diverting to other methods or commenting on their pros or cons.
- Do not make the section bulky : For extensive methods and protocols, provide the essential details and share the rest of the information in the supplemental files. The writing should be clear yet concise to maintain the flow of the section.
We hope that by this point, you understand how crucial it is to write a thoughtful and precise methods section and the ins and outs of how to write the methods section of a research paper . To restate, the entire purpose of the methods section is to enable others to reproduce the results or verify the research. We sincerely hope that this post has cleared up any confusion and given you a fresh perspective on the methods section .
As a parting gift, we’re leaving you with a handy checklist that will help you understand how to write the methods section of a research paper . Feel free to download this checklist and use or share this with those who you think may benefit from it.
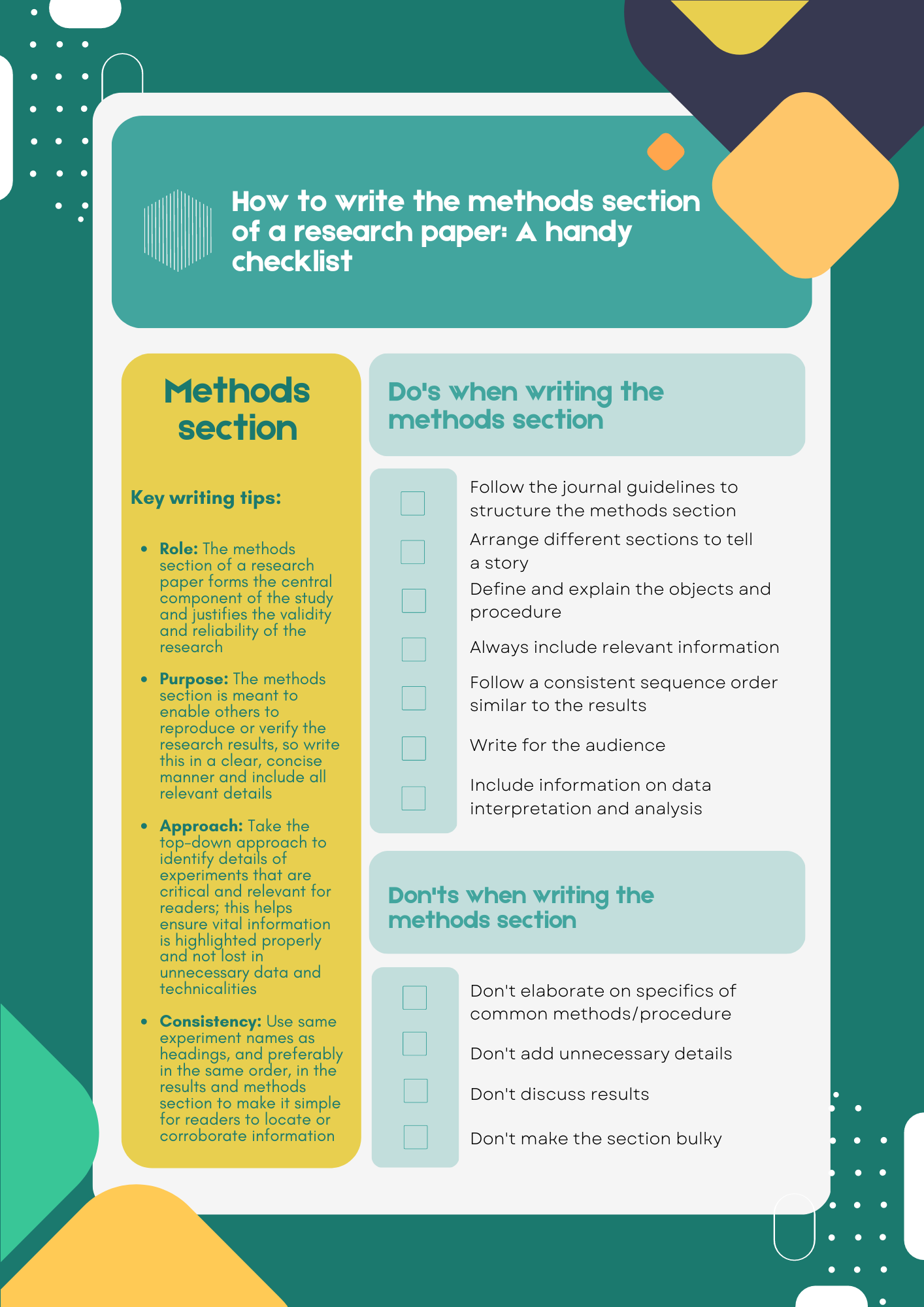
References
- Bhattacharya, D. How to write the Methods section of a research paper. Editage Insights, 2018. https://www.editage.com/insights/how-to-write-the-methods-section-of-a-research-paper (2018).
- Kallet, R. H. How to Write the Methods Section of a Research Paper. Respiratory Care 49, 1229–1232 (2004). https://pubmed.ncbi.nlm.nih.gov/15447808/
- Grindstaff, T. L. & Saliba, S. A. AVOIDING MANUSCRIPT MISTAKES. Int J Sports Phys Ther 7, 518–524 (2012). https://www.ncbi.nlm.nih.gov/pmc/articles/PMC3474299/
Editage All Access is a subscription-based platform that unifies the best AI tools and services designed to speed up, simplify, and streamline every step of a researcher’s journey. The Editage All Access Pack is a one-of-a-kind subscription that unlocks full access to an AI writing assistant, literature recommender, journal finder, scientific illustration tool, and exclusive discounts on professional publication services from Editage.
Based on 22+ years of experience in academia, Editage All Access empowers researchers to put their best research forward and move closer to success. Explore our top AI Tools pack, AI Tools + Publication Services pack, or Build Your Own Plan. Find everything a researcher needs to succeed, all in one place – Get All Access now starting at just $14 a month !
Related Posts

What is IMRaD Format in Research?

What is a Review Article? How to Write it?

Research Methodologies
- What are research designs?
- What are research methodologies?
What are research methods?
Quantitative research methods, qualitative research methods, mixed method approach, selecting the best research method.
- Additional Sources
Research methods are different from research methodologies because they are the ways in which you will collect the data for your research project. The best method for your project largely depends on your topic, the type of data you will need, and the people or items from which you will be collecting data. The following boxes below contain a list of quantitative, qualitative, and mixed research methods.
- Closed-ended questionnaires/survey: These types of questionnaires or surveys are like "multiple choice" tests, where participants must select from a list of premade answers. According to the content of the question, they must select the one that they agree with the most. This approach is the simplest form of quantitative research because the data is easy to combine and quantify.
- Structured interviews: These are a common research method in market research because the data can be quantified. They are strictly designed for little "wiggle room" in the interview process so that the data will not be skewed. You can conduct structured interviews in-person, online, or over the phone (Dawson, 2019).
Constructing Questionnaires
When constructing your questions for a survey or questionnaire, there are things you can do to ensure that your questions are accurate and easy to understand (Dawson, 2019):
- Keep the questions brief and simple.
- Eliminate any potential bias from your questions. Make sure that they do not word things in a way that favor one perspective over another.
- If your topic is very sensitive, you may want to ask indirect questions rather than direct ones. This prevents participants from being intimidated and becoming unwilling to share their true responses.
- If you are using a closed-ended question, try to offer every possible answer that a participant could give to that question.
- Do not ask questions that assume something of the participant. The question "How often do you exercise?" assumes that the participant exercises (when they may not), so you would want to include a question that asks if they exercise at all before asking them how often.
- Try and keep the questionnaire as short as possible. The longer a questionnaire takes, the more likely the participant will not complete it or get too tired to put truthful answers.
- Promise confidentiality to your participants at the beginning of the questionnaire.
Quantitative Research Measures
When you are considering a quantitative approach to your research, you need to identify why types of measures you will use in your study. This will determine what type of numbers you will be using to collect your data. There are four levels of measurement:
- Nominal: These are numbers where the order of the numbers do not matter. They aim to identify separate information. One example is collecting zip codes from research participants. The order of the numbers does not matter, but the series of numbers in each zip code indicate different information (Adamson and Prion, 2013).
- Ordinal: Also known as rankings because the order of these numbers matter. This is when items are given a specific rank according to specific criteria. A common example of ordinal measurements include ranking-based questionnaires, where participants are asked to rank items from least favorite to most favorite. Another common example is a pain scale, where a patient is asked to rank their pain on a scale from 1 to 10 (Adamson and Prion, 2013).
- Interval: This is when the data are ordered and the distance between the numbers matters to the researcher (Adamson and Prion, 2013). The distance between each number is the same. An example of interval data is test grades.
- Ratio: This is when the data are ordered and have a consistent distance between numbers, but has a "zero point." This means that there could be a measurement of zero of whatever you are measuring in your study (Adamson and Prion, 2013). An example of ratio data is measuring the height of something because the "zero point" remains constant in all measurements. The height of something could also be zero.
Focus Groups
This is when a select group of people gather to talk about a particular topic. They can also be called discussion groups or group interviews (Dawson, 2019). They are usually lead by a moderator to help guide the discussion and ask certain questions. It is critical that a moderator allows everyone in the group to get a chance to speak so that no one dominates the discussion. The data that are gathered from focus groups tend to be thoughts, opinions, and perspectives about an issue.
Advantages of Focus Groups
- Only requires one meeting to get different types of responses.
- Less researcher bias due to participants being able to speak openly.
- Helps participants overcome insecurities or fears about a topic.
- The researcher can also consider the impact of participant interaction.
Disadvantages of Focus Groups
- Participants may feel uncomfortable to speak in front of an audience, especially if the topic is sensitive or controversial.
- Since participation is voluntary, not every participant may contribute equally to the discussion.
- Participants may impact what others say or think.
- A researcher may feel intimidated by running a focus group on their own.
- A researcher may need extra funds/resources to provide a safe space to host the focus group.
- Because the data is collective, it may be difficult to determine a participant's individual thoughts about the research topic.
Observation
There are two ways to conduct research observations:
- Direct Observation: The researcher observes a participant in an environment. The researcher often takes notes or uses technology to gather data, such as a voice recorder or video camera. The researcher does not interact or interfere with the participants. This approach is often used in psychology and health studies (Dawson, 2019).
- Participant Observation: The researcher interacts directly with the participants to get a better understanding of the research topic. This is a common research method when trying to understand another culture or community. It is important to decide if you will conduct a covert (participants do not know they are part of the research) or overt (participants know the researcher is observing them) observation because it can be unethical in some situations (Dawson, 2019).
Open-Ended Questionnaires
These types of questionnaires are the opposite of "multiple choice" questionnaires because the answer boxes are left open for the participant to complete. This means that participants can write short or extended answers to the questions. Upon gathering the responses, researchers will often "quantify" the data by organizing the responses into different categories. This can be time consuming because the researcher needs to read all responses carefully.
Semi-structured Interviews
This is the most common type of interview where researchers aim to get specific information so they can compare it to other interview data. This requires asking the same questions for each interview, but keeping their responses flexible. This means including follow-up questions if a subject answers a certain way. Interview schedules are commonly used to aid the interviewers, which list topics or questions that will be discussed at each interview (Dawson, 2019).
Theoretical Analysis
Often used for nonhuman research, theoretical analysis is a qualitative approach where the researcher applies a theoretical framework to analyze something about their topic. A theoretical framework gives the researcher a specific "lens" to view the topic and think about it critically. it also serves as context to guide the entire study. This is a popular research method for analyzing works of literature, films, and other forms of media. You can implement more than one theoretical framework with this method, as many theories complement one another.
Common theoretical frameworks for qualitative research are (Grant and Osanloo, 2014):
- Behavioral theory
- Change theory
- Cognitive theory
- Content analysis
- Cross-sectional analysis
- Developmental theory
- Feminist theory
- Gender theory
- Marxist theory
- Queer theory
- Systems theory
- Transformational theory
Unstructured Interviews
These are in-depth interviews where the researcher tries to understand an interviewee's perspective on a situation or issue. They are sometimes called life history interviews. It is important not to bombard the interviewee with too many questions so they can freely disclose their thoughts (Dawson, 2019).
- Open-ended and closed-ended questionnaires: This approach means implementing elements of both questionnaire types into your data collection. Participants may answer some questions with premade answers and write their own answers to other questions. The advantage to this method is that you benefit from both types of data collection to get a broader understanding of you participants. However, you must think carefully about how you will analyze this data to arrive at a conclusion.
Other mixed method approaches that incorporate quantitative and qualitative research methods depend heavily on the research topic. It is strongly recommended that you collaborate with your academic advisor before finalizing a mixed method approach.
How do you determine which research method would be best for your proposal? This heavily depends on your research objective. According to Dawson (2019), there are several questions to ask yourself when determining the best research method for your project:
- Are you good with numbers and mathematics?
- Would you be interested in conducting interviews with human subjects?
- Would you enjoy creating a questionnaire for participants to complete?
- Do you prefer written communication or face-to-face interaction?
- What skills or experiences do you have that might help you with your research? Do you have any experiences from past research projects that can help with this one?
- How much time do you have to complete the research? Some methods take longer to collect data than others.
- What is your budget? Do you have adequate funding to conduct the research in the method you want?
- How much data do you need? Some research topics need only a small amount of data while others may need significantly larger amounts.
- What is the purpose of your research? This can provide a good indicator as to what research method will be most appropriate.
- << Previous: What are research methodologies?
- Next: Additional Sources >>
- Last Updated: Aug 2, 2022 2:36 PM
- URL: https://library.tiffin.edu/researchmethodologies
Generate accurate APA citations for free
- Knowledge Base
- APA Style 7th edition
- How to write an APA methods section
How to Write an APA Methods Section | With Examples
Published on February 5, 2021 by Pritha Bhandari . Revised on June 22, 2023.
The methods section of an APA style paper is where you report in detail how you performed your study. Research papers in the social and natural sciences often follow APA style. This article focuses on reporting quantitative research methods .
In your APA methods section, you should report enough information to understand and replicate your study, including detailed information on the sample , measures, and procedures used.
Instantly correct all language mistakes in your text
Upload your document to correct all your mistakes in minutes
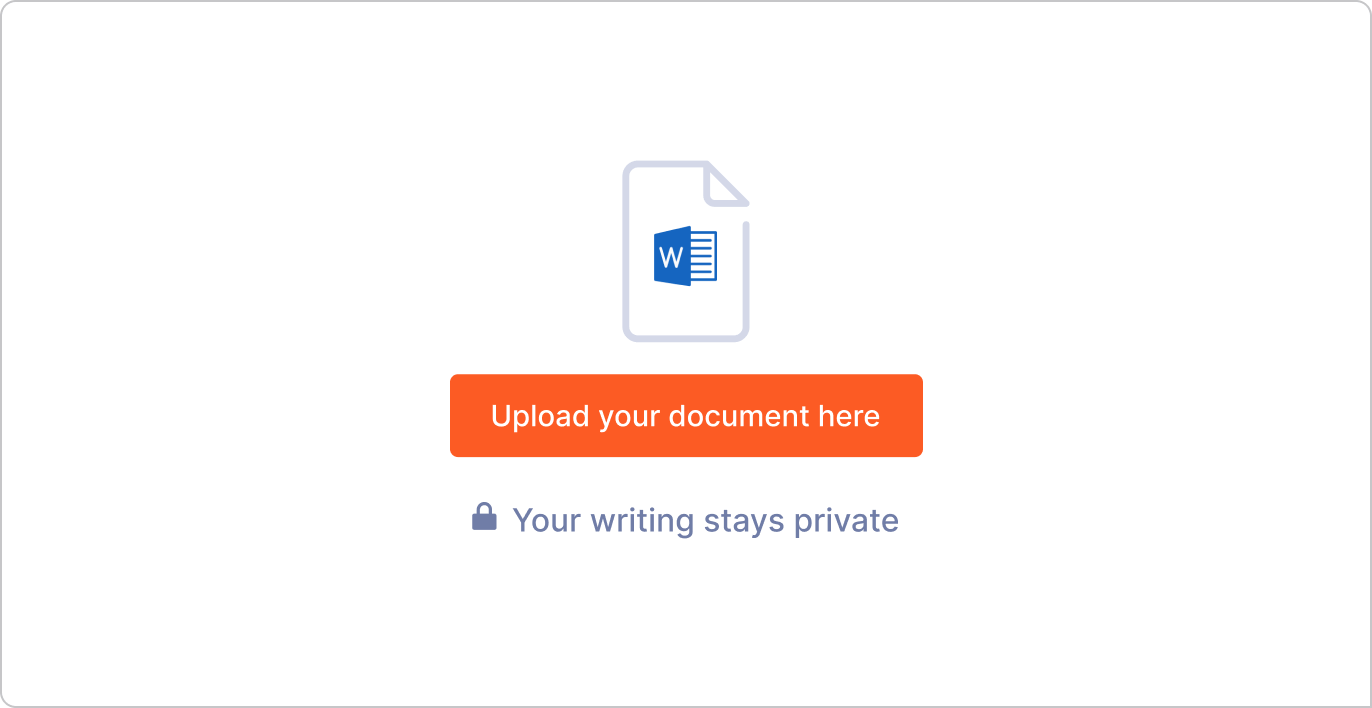
Table of contents
Structuring an apa methods section.
Participants
Example of an APA methods section
Other interesting articles, frequently asked questions about writing an apa methods section.
The main heading of “Methods” should be centered, boldfaced, and capitalized. Subheadings within this section are left-aligned, boldfaced, and in title case. You can also add lower level headings within these subsections, as long as they follow APA heading styles .
To structure your methods section, you can use the subheadings of “Participants,” “Materials,” and “Procedures.” These headings are not mandatory—aim to organize your methods section using subheadings that make sense for your specific study.
Heading | What to include |
---|---|
Participants | |
Materials | |
Procedure |
Note that not all of these topics will necessarily be relevant for your study. For example, if you didn’t need to consider outlier removal or ways of assigning participants to different conditions, you don’t have to report these steps.
The APA also provides specific reporting guidelines for different types of research design. These tell you exactly what you need to report for longitudinal designs , replication studies, experimental designs , and so on. If your study uses a combination design, consult APA guidelines for mixed methods studies.
Detailed descriptions of procedures that don’t fit into your main text can be placed in supplemental materials (for example, the exact instructions and tasks given to participants, the full analytical strategy including software code, or additional figures and tables).
Scribbr Citation Checker New
The AI-powered Citation Checker helps you avoid common mistakes such as:
- Missing commas and periods
- Incorrect usage of “et al.”
- Ampersands (&) in narrative citations
- Missing reference entries
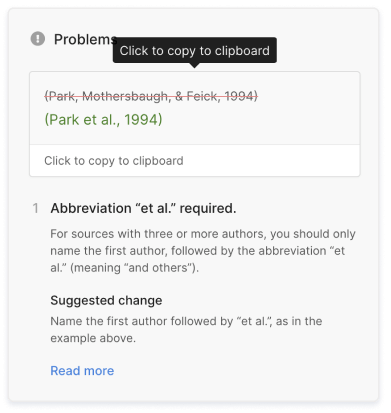
Begin the methods section by reporting sample characteristics, sampling procedures, and the sample size.

Participant or subject characteristics
When discussing people who participate in research, descriptive terms like “participants,” “subjects” and “respondents” can be used. For non-human animal research, “subjects” is more appropriate.
Specify all relevant demographic characteristics of your participants. This may include their age, sex, ethnic or racial group, gender identity, education level, and socioeconomic status. Depending on your study topic, other characteristics like educational or immigration status or language preference may also be relevant.
Be sure to report these characteristics as precisely as possible. This helps the reader understand how far your results may be generalized to other people.
The APA guidelines emphasize writing about participants using bias-free language , so it’s necessary to use inclusive and appropriate terms.
Sampling procedures
Outline how the participants were selected and all inclusion and exclusion criteria applied. Appropriately identify the sampling procedure used. For example, you should only label a sample as random if you had access to every member of the relevant population.
Of all the people invited to participate in your study, note the percentage that actually did (if you have this data). Additionally, report whether participants were self-selected, either by themselves or by their institutions (e.g., schools may submit student data for research purposes).
Identify any compensation (e.g., course credits or money) that was provided to participants, and mention any institutional review board approvals and ethical standards followed.
Sample size and power
Detail the sample size (per condition) and statistical power that you hoped to achieve, as well as any analyses you performed to determine these numbers.
It’s important to show that your study had enough statistical power to find effects if there were any to be found.
Additionally, state whether your final sample differed from the intended sample. Your interpretations of the study outcomes should be based only on your final sample rather than your intended sample.
Write up the tools and techniques that you used to measure relevant variables. Be as thorough as possible for a complete picture of your techniques.
Primary and secondary measures
Define the primary and secondary outcome measures that will help you answer your primary and secondary research questions.
Specify all instruments used in gathering these measurements and the construct that they measure. These instruments may include hardware, software, or tests, scales, and inventories.
- To cite hardware, indicate the model number and manufacturer.
- To cite common software (e.g., Qualtrics), state the full name along with the version number or the website URL .
- To cite tests, scales or inventories, reference its manual or the article it was published in. It’s also helpful to state the number of items and provide one or two example items.
Make sure to report the settings of (e.g., screen resolution) any specialized apparatus used.
For each instrument used, report measures of the following:
- Reliability : how consistently the method measures something, in terms of internal consistency or test-retest reliability.
- Validity : how precisely the method measures something, in terms of construct validity or criterion validity .
Giving an example item or two for tests, questionnaires , and interviews is also helpful.
Describe any covariates—these are any additional variables that may explain or predict the outcomes.
Quality of measurements
Review all methods you used to assure the quality of your measurements.
These may include:
- training researchers to collect data reliably,
- using multiple people to assess (e.g., observe or code) the data,
- translation and back-translation of research materials,
- using pilot studies to test your materials on unrelated samples.
For data that’s subjectively coded (for example, classifying open-ended responses), report interrater reliability scores. This tells the reader how similarly each response was rated by multiple raters.
Report all of the procedures applied for administering the study, processing the data, and for planned data analyses.
Data collection methods and research design
Data collection methods refers to the general mode of the instruments: surveys, interviews, observations, focus groups, neuroimaging, cognitive tests, and so on. Summarize exactly how you collected the necessary data.
Describe all procedures you applied in administering surveys, tests, physical recordings, or imaging devices, with enough detail so that someone else can replicate your techniques. If your procedures are very complicated and require long descriptions (e.g., in neuroimaging studies), place these details in supplementary materials.
To report research design, note your overall framework for data collection and analysis. State whether you used an experimental, quasi-experimental, descriptive (observational), correlational, and/or longitudinal design. Also note whether a between-subjects or a within-subjects design was used.
For multi-group studies, report the following design and procedural details as well:
- how participants were assigned to different conditions (e.g., randomization),
- instructions given to the participants in each group,
- interventions for each group,
- the setting and length of each session(s).
Describe whether any masking was used to hide the condition assignment (e.g., placebo or medication condition) from participants or research administrators. Using masking in a multi-group study ensures internal validity by reducing research bias . Explain how this masking was applied and whether its effectiveness was assessed.
Participants were randomly assigned to a control or experimental condition. The survey was administered using Qualtrics (https://www.qualtrics.com). To begin, all participants were given the AAI and a demographics questionnaire to complete, followed by an unrelated filler task. In the control condition , participants completed a short general knowledge test immediately after the filler task. In the experimental condition, participants were asked to visualize themselves taking the test for 3 minutes before they actually did. For more details on the exact instructions and tasks given, see supplementary materials.
Data diagnostics
Outline all steps taken to scrutinize or process the data after collection.
This includes the following:
- Procedures for identifying and removing outliers
- Data transformations to normalize distributions
- Compensation strategies for overcoming missing values
To ensure high validity, you should provide enough detail for your reader to understand how and why you processed or transformed your raw data in these specific ways.
Analytic strategies
The methods section is also where you describe your statistical analysis procedures, but not their outcomes. Their outcomes are reported in the results section.
These procedures should be stated for all primary, secondary, and exploratory hypotheses. While primary and secondary hypotheses are based on a theoretical framework or past studies, exploratory hypotheses are guided by the data you’ve just collected.
This annotated example reports methods for a descriptive correlational survey on the relationship between religiosity and trust in science in the US. Hover over each part for explanation of what is included.
The sample included 879 adults aged between 18 and 28. More than half of the participants were women (56%), and all participants had completed at least 12 years of education. Ethics approval was obtained from the university board before recruitment began. Participants were recruited online through Amazon Mechanical Turk (MTurk; www.mturk.com). We selected for a geographically diverse sample within the Midwest of the US through an initial screening survey. Participants were paid USD $5 upon completion of the study.
A sample size of at least 783 was deemed necessary for detecting a correlation coefficient of ±.1, with a power level of 80% and a significance level of .05, using a sample size calculator (www.sample-size.net/correlation-sample-size/).
The primary outcome measures were the levels of religiosity and trust in science. Religiosity refers to involvement and belief in religious traditions, while trust in science represents confidence in scientists and scientific research outcomes. The secondary outcome measures were gender and parental education levels of participants and whether these characteristics predicted religiosity levels.
Religiosity
Religiosity was measured using the Centrality of Religiosity scale (Huber, 2003). The Likert scale is made up of 15 questions with five subscales of ideology, experience, intellect, public practice, and private practice. An example item is “How often do you experience situations in which you have the feeling that God or something divine intervenes in your life?” Participants were asked to indicate frequency of occurrence by selecting a response ranging from 1 (very often) to 5 (never). The internal consistency of the instrument is .83 (Huber & Huber, 2012).
Trust in Science
Trust in science was assessed using the General Trust in Science index (McCright, Dentzman, Charters & Dietz, 2013). Four Likert scale items were assessed on a scale from 1 (completely distrust) to 5 (completely trust). An example question asks “How much do you distrust or trust scientists to create knowledge that is unbiased and accurate?” Internal consistency was .8.
Potential participants were invited to participate in the survey online using Qualtrics (www.qualtrics.com). The survey consisted of multiple choice questions regarding demographic characteristics, the Centrality of Religiosity scale, an unrelated filler anagram task, and finally the General Trust in Science index. The filler task was included to avoid priming or demand characteristics, and an attention check was embedded within the religiosity scale. For full instructions and details of tasks, see supplementary materials.
For this correlational study , we assessed our primary hypothesis of a relationship between religiosity and trust in science using Pearson moment correlation coefficient. The statistical significance of the correlation coefficient was assessed using a t test. To test our secondary hypothesis of parental education levels and gender as predictors of religiosity, multiple linear regression analysis was used.
If you want to know more about statistics , methodology , or research bias , make sure to check out some of our other articles with explanations and examples.
- Normal distribution
- Measures of central tendency
- Chi square tests
- Confidence interval
- Quartiles & Quantiles
Methodology
- Cluster sampling
- Stratified sampling
- Thematic analysis
- Cohort study
- Peer review
- Ethnography
Research bias
- Implicit bias
- Cognitive bias
- Conformity bias
- Hawthorne effect
- Availability heuristic
- Attrition bias
- Social desirability bias
In your APA methods section , you should report detailed information on the participants, materials, and procedures used.
- Describe all relevant participant or subject characteristics, the sampling procedures used and the sample size and power .
- Define all primary and secondary measures and discuss the quality of measurements.
- Specify the data collection methods, the research design and data analysis strategy, including any steps taken to transform the data and statistical analyses.
You should report methods using the past tense , even if you haven’t completed your study at the time of writing. That’s because the methods section is intended to describe completed actions or research.
In a scientific paper, the methodology always comes after the introduction and before the results , discussion and conclusion . The same basic structure also applies to a thesis, dissertation , or research proposal .
Depending on the length and type of document, you might also include a literature review or theoretical framework before the methodology.
Cite this Scribbr article
If you want to cite this source, you can copy and paste the citation or click the “Cite this Scribbr article” button to automatically add the citation to our free Citation Generator.
Bhandari, P. (2023, June 22). How to Write an APA Methods Section | With Examples. Scribbr. Retrieved August 8, 2024, from https://www.scribbr.com/apa-style/methods-section/
Is this article helpful?
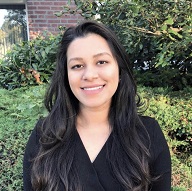
Pritha Bhandari
Other students also liked, how to write an apa results section, apa format for academic papers and essays, apa headings and subheadings, get unlimited documents corrected.
✔ Free APA citation check included ✔ Unlimited document corrections ✔ Specialized in correcting academic texts

An official website of the United States government
The .gov means it’s official. Federal government websites often end in .gov or .mil. Before sharing sensitive information, make sure you’re on a federal government site.
The site is secure. The https:// ensures that you are connecting to the official website and that any information you provide is encrypted and transmitted securely.
- Publications
- Account settings
Preview improvements coming to the PMC website in October 2024. Learn More or Try it out now .
- Advanced Search
- Journal List
- Neurol Res Pract

How to use and assess qualitative research methods
Loraine busetto.
1 Department of Neurology, Heidelberg University Hospital, Im Neuenheimer Feld 400, 69120 Heidelberg, Germany
Wolfgang Wick
2 Clinical Cooperation Unit Neuro-Oncology, German Cancer Research Center, Heidelberg, Germany
Christoph Gumbinger
Associated data.
Not applicable.
This paper aims to provide an overview of the use and assessment of qualitative research methods in the health sciences. Qualitative research can be defined as the study of the nature of phenomena and is especially appropriate for answering questions of why something is (not) observed, assessing complex multi-component interventions, and focussing on intervention improvement. The most common methods of data collection are document study, (non-) participant observations, semi-structured interviews and focus groups. For data analysis, field-notes and audio-recordings are transcribed into protocols and transcripts, and coded using qualitative data management software. Criteria such as checklists, reflexivity, sampling strategies, piloting, co-coding, member-checking and stakeholder involvement can be used to enhance and assess the quality of the research conducted. Using qualitative in addition to quantitative designs will equip us with better tools to address a greater range of research problems, and to fill in blind spots in current neurological research and practice.
The aim of this paper is to provide an overview of qualitative research methods, including hands-on information on how they can be used, reported and assessed. This article is intended for beginning qualitative researchers in the health sciences as well as experienced quantitative researchers who wish to broaden their understanding of qualitative research.
What is qualitative research?
Qualitative research is defined as “the study of the nature of phenomena”, including “their quality, different manifestations, the context in which they appear or the perspectives from which they can be perceived” , but excluding “their range, frequency and place in an objectively determined chain of cause and effect” [ 1 ]. This formal definition can be complemented with a more pragmatic rule of thumb: qualitative research generally includes data in form of words rather than numbers [ 2 ].
Why conduct qualitative research?
Because some research questions cannot be answered using (only) quantitative methods. For example, one Australian study addressed the issue of why patients from Aboriginal communities often present late or not at all to specialist services offered by tertiary care hospitals. Using qualitative interviews with patients and staff, it found one of the most significant access barriers to be transportation problems, including some towns and communities simply not having a bus service to the hospital [ 3 ]. A quantitative study could have measured the number of patients over time or even looked at possible explanatory factors – but only those previously known or suspected to be of relevance. To discover reasons for observed patterns, especially the invisible or surprising ones, qualitative designs are needed.
While qualitative research is common in other fields, it is still relatively underrepresented in health services research. The latter field is more traditionally rooted in the evidence-based-medicine paradigm, as seen in " research that involves testing the effectiveness of various strategies to achieve changes in clinical practice, preferably applying randomised controlled trial study designs (...) " [ 4 ]. This focus on quantitative research and specifically randomised controlled trials (RCT) is visible in the idea of a hierarchy of research evidence which assumes that some research designs are objectively better than others, and that choosing a "lesser" design is only acceptable when the better ones are not practically or ethically feasible [ 5 , 6 ]. Others, however, argue that an objective hierarchy does not exist, and that, instead, the research design and methods should be chosen to fit the specific research question at hand – "questions before methods" [ 2 , 7 – 9 ]. This means that even when an RCT is possible, some research problems require a different design that is better suited to addressing them. Arguing in JAMA, Berwick uses the example of rapid response teams in hospitals, which he describes as " a complex, multicomponent intervention – essentially a process of social change" susceptible to a range of different context factors including leadership or organisation history. According to him, "[in] such complex terrain, the RCT is an impoverished way to learn. Critics who use it as a truth standard in this context are incorrect" [ 8 ] . Instead of limiting oneself to RCTs, Berwick recommends embracing a wider range of methods , including qualitative ones, which for "these specific applications, (...) are not compromises in learning how to improve; they are superior" [ 8 ].
Research problems that can be approached particularly well using qualitative methods include assessing complex multi-component interventions or systems (of change), addressing questions beyond “what works”, towards “what works for whom when, how and why”, and focussing on intervention improvement rather than accreditation [ 7 , 9 – 12 ]. Using qualitative methods can also help shed light on the “softer” side of medical treatment. For example, while quantitative trials can measure the costs and benefits of neuro-oncological treatment in terms of survival rates or adverse effects, qualitative research can help provide a better understanding of patient or caregiver stress, visibility of illness or out-of-pocket expenses.
How to conduct qualitative research?
Given that qualitative research is characterised by flexibility, openness and responsivity to context, the steps of data collection and analysis are not as separate and consecutive as they tend to be in quantitative research [ 13 , 14 ]. As Fossey puts it : “sampling, data collection, analysis and interpretation are related to each other in a cyclical (iterative) manner, rather than following one after another in a stepwise approach” [ 15 ]. The researcher can make educated decisions with regard to the choice of method, how they are implemented, and to which and how many units they are applied [ 13 ]. As shown in Fig. 1 , this can involve several back-and-forth steps between data collection and analysis where new insights and experiences can lead to adaption and expansion of the original plan. Some insights may also necessitate a revision of the research question and/or the research design as a whole. The process ends when saturation is achieved, i.e. when no relevant new information can be found (see also below: sampling and saturation). For reasons of transparency, it is essential for all decisions as well as the underlying reasoning to be well-documented.

Iterative research process
While it is not always explicitly addressed, qualitative methods reflect a different underlying research paradigm than quantitative research (e.g. constructivism or interpretivism as opposed to positivism). The choice of methods can be based on the respective underlying substantive theory or theoretical framework used by the researcher [ 2 ].
Data collection
The methods of qualitative data collection most commonly used in health research are document study, observations, semi-structured interviews and focus groups [ 1 , 14 , 16 , 17 ].
Document study
Document study (also called document analysis) refers to the review by the researcher of written materials [ 14 ]. These can include personal and non-personal documents such as archives, annual reports, guidelines, policy documents, diaries or letters.
Observations
Observations are particularly useful to gain insights into a certain setting and actual behaviour – as opposed to reported behaviour or opinions [ 13 ]. Qualitative observations can be either participant or non-participant in nature. In participant observations, the observer is part of the observed setting, for example a nurse working in an intensive care unit [ 18 ]. In non-participant observations, the observer is “on the outside looking in”, i.e. present in but not part of the situation, trying not to influence the setting by their presence. Observations can be planned (e.g. for 3 h during the day or night shift) or ad hoc (e.g. as soon as a stroke patient arrives at the emergency room). During the observation, the observer takes notes on everything or certain pre-determined parts of what is happening around them, for example focusing on physician-patient interactions or communication between different professional groups. Written notes can be taken during or after the observations, depending on feasibility (which is usually lower during participant observations) and acceptability (e.g. when the observer is perceived to be judging the observed). Afterwards, these field notes are transcribed into observation protocols. If more than one observer was involved, field notes are taken independently, but notes can be consolidated into one protocol after discussions. Advantages of conducting observations include minimising the distance between the researcher and the researched, the potential discovery of topics that the researcher did not realise were relevant and gaining deeper insights into the real-world dimensions of the research problem at hand [ 18 ].
Semi-structured interviews
Hijmans & Kuyper describe qualitative interviews as “an exchange with an informal character, a conversation with a goal” [ 19 ]. Interviews are used to gain insights into a person’s subjective experiences, opinions and motivations – as opposed to facts or behaviours [ 13 ]. Interviews can be distinguished by the degree to which they are structured (i.e. a questionnaire), open (e.g. free conversation or autobiographical interviews) or semi-structured [ 2 , 13 ]. Semi-structured interviews are characterized by open-ended questions and the use of an interview guide (or topic guide/list) in which the broad areas of interest, sometimes including sub-questions, are defined [ 19 ]. The pre-defined topics in the interview guide can be derived from the literature, previous research or a preliminary method of data collection, e.g. document study or observations. The topic list is usually adapted and improved at the start of the data collection process as the interviewer learns more about the field [ 20 ]. Across interviews the focus on the different (blocks of) questions may differ and some questions may be skipped altogether (e.g. if the interviewee is not able or willing to answer the questions or for concerns about the total length of the interview) [ 20 ]. Qualitative interviews are usually not conducted in written format as it impedes on the interactive component of the method [ 20 ]. In comparison to written surveys, qualitative interviews have the advantage of being interactive and allowing for unexpected topics to emerge and to be taken up by the researcher. This can also help overcome a provider or researcher-centred bias often found in written surveys, which by nature, can only measure what is already known or expected to be of relevance to the researcher. Interviews can be audio- or video-taped; but sometimes it is only feasible or acceptable for the interviewer to take written notes [ 14 , 16 , 20 ].
Focus groups
Focus groups are group interviews to explore participants’ expertise and experiences, including explorations of how and why people behave in certain ways [ 1 ]. Focus groups usually consist of 6–8 people and are led by an experienced moderator following a topic guide or “script” [ 21 ]. They can involve an observer who takes note of the non-verbal aspects of the situation, possibly using an observation guide [ 21 ]. Depending on researchers’ and participants’ preferences, the discussions can be audio- or video-taped and transcribed afterwards [ 21 ]. Focus groups are useful for bringing together homogeneous (to a lesser extent heterogeneous) groups of participants with relevant expertise and experience on a given topic on which they can share detailed information [ 21 ]. Focus groups are a relatively easy, fast and inexpensive method to gain access to information on interactions in a given group, i.e. “the sharing and comparing” among participants [ 21 ]. Disadvantages include less control over the process and a lesser extent to which each individual may participate. Moreover, focus group moderators need experience, as do those tasked with the analysis of the resulting data. Focus groups can be less appropriate for discussing sensitive topics that participants might be reluctant to disclose in a group setting [ 13 ]. Moreover, attention must be paid to the emergence of “groupthink” as well as possible power dynamics within the group, e.g. when patients are awed or intimidated by health professionals.
Choosing the “right” method
As explained above, the school of thought underlying qualitative research assumes no objective hierarchy of evidence and methods. This means that each choice of single or combined methods has to be based on the research question that needs to be answered and a critical assessment with regard to whether or to what extent the chosen method can accomplish this – i.e. the “fit” between question and method [ 14 ]. It is necessary for these decisions to be documented when they are being made, and to be critically discussed when reporting methods and results.
Let us assume that our research aim is to examine the (clinical) processes around acute endovascular treatment (EVT), from the patient’s arrival at the emergency room to recanalization, with the aim to identify possible causes for delay and/or other causes for sub-optimal treatment outcome. As a first step, we could conduct a document study of the relevant standard operating procedures (SOPs) for this phase of care – are they up-to-date and in line with current guidelines? Do they contain any mistakes, irregularities or uncertainties that could cause delays or other problems? Regardless of the answers to these questions, the results have to be interpreted based on what they are: a written outline of what care processes in this hospital should look like. If we want to know what they actually look like in practice, we can conduct observations of the processes described in the SOPs. These results can (and should) be analysed in themselves, but also in comparison to the results of the document analysis, especially as regards relevant discrepancies. Do the SOPs outline specific tests for which no equipment can be observed or tasks to be performed by specialized nurses who are not present during the observation? It might also be possible that the written SOP is outdated, but the actual care provided is in line with current best practice. In order to find out why these discrepancies exist, it can be useful to conduct interviews. Are the physicians simply not aware of the SOPs (because their existence is limited to the hospital’s intranet) or do they actively disagree with them or does the infrastructure make it impossible to provide the care as described? Another rationale for adding interviews is that some situations (or all of their possible variations for different patient groups or the day, night or weekend shift) cannot practically or ethically be observed. In this case, it is possible to ask those involved to report on their actions – being aware that this is not the same as the actual observation. A senior physician’s or hospital manager’s description of certain situations might differ from a nurse’s or junior physician’s one, maybe because they intentionally misrepresent facts or maybe because different aspects of the process are visible or important to them. In some cases, it can also be relevant to consider to whom the interviewee is disclosing this information – someone they trust, someone they are otherwise not connected to, or someone they suspect or are aware of being in a potentially “dangerous” power relationship to them. Lastly, a focus group could be conducted with representatives of the relevant professional groups to explore how and why exactly they provide care around EVT. The discussion might reveal discrepancies (between SOPs and actual care or between different physicians) and motivations to the researchers as well as to the focus group members that they might not have been aware of themselves. For the focus group to deliver relevant information, attention has to be paid to its composition and conduct, for example, to make sure that all participants feel safe to disclose sensitive or potentially problematic information or that the discussion is not dominated by (senior) physicians only. The resulting combination of data collection methods is shown in Fig. 2 .

Possible combination of data collection methods
Attributions for icons: “Book” by Serhii Smirnov, “Interview” by Adrien Coquet, FR, “Magnifying Glass” by anggun, ID, “Business communication” by Vectors Market; all from the Noun Project
The combination of multiple data source as described for this example can be referred to as “triangulation”, in which multiple measurements are carried out from different angles to achieve a more comprehensive understanding of the phenomenon under study [ 22 , 23 ].
Data analysis
To analyse the data collected through observations, interviews and focus groups these need to be transcribed into protocols and transcripts (see Fig. 3 ). Interviews and focus groups can be transcribed verbatim , with or without annotations for behaviour (e.g. laughing, crying, pausing) and with or without phonetic transcription of dialects and filler words, depending on what is expected or known to be relevant for the analysis. In the next step, the protocols and transcripts are coded , that is, marked (or tagged, labelled) with one or more short descriptors of the content of a sentence or paragraph [ 2 , 15 , 23 ]. Jansen describes coding as “connecting the raw data with “theoretical” terms” [ 20 ]. In a more practical sense, coding makes raw data sortable. This makes it possible to extract and examine all segments describing, say, a tele-neurology consultation from multiple data sources (e.g. SOPs, emergency room observations, staff and patient interview). In a process of synthesis and abstraction, the codes are then grouped, summarised and/or categorised [ 15 , 20 ]. The end product of the coding or analysis process is a descriptive theory of the behavioural pattern under investigation [ 20 ]. The coding process is performed using qualitative data management software, the most common ones being InVivo, MaxQDA and Atlas.ti. It should be noted that these are data management tools which support the analysis performed by the researcher(s) [ 14 ].

From data collection to data analysis
Attributions for icons: see Fig. Fig.2, 2 , also “Speech to text” by Trevor Dsouza, “Field Notes” by Mike O’Brien, US, “Voice Record” by ProSymbols, US, “Inspection” by Made, AU, and “Cloud” by Graphic Tigers; all from the Noun Project
How to report qualitative research?
Protocols of qualitative research can be published separately and in advance of the study results. However, the aim is not the same as in RCT protocols, i.e. to pre-define and set in stone the research questions and primary or secondary endpoints. Rather, it is a way to describe the research methods in detail, which might not be possible in the results paper given journals’ word limits. Qualitative research papers are usually longer than their quantitative counterparts to allow for deep understanding and so-called “thick description”. In the methods section, the focus is on transparency of the methods used, including why, how and by whom they were implemented in the specific study setting, so as to enable a discussion of whether and how this may have influenced data collection, analysis and interpretation. The results section usually starts with a paragraph outlining the main findings, followed by more detailed descriptions of, for example, the commonalities, discrepancies or exceptions per category [ 20 ]. Here it is important to support main findings by relevant quotations, which may add information, context, emphasis or real-life examples [ 20 , 23 ]. It is subject to debate in the field whether it is relevant to state the exact number or percentage of respondents supporting a certain statement (e.g. “Five interviewees expressed negative feelings towards XYZ”) [ 21 ].
How to combine qualitative with quantitative research?
Qualitative methods can be combined with other methods in multi- or mixed methods designs, which “[employ] two or more different methods [ …] within the same study or research program rather than confining the research to one single method” [ 24 ]. Reasons for combining methods can be diverse, including triangulation for corroboration of findings, complementarity for illustration and clarification of results, expansion to extend the breadth and range of the study, explanation of (unexpected) results generated with one method with the help of another, or offsetting the weakness of one method with the strength of another [ 1 , 17 , 24 – 26 ]. The resulting designs can be classified according to when, why and how the different quantitative and/or qualitative data strands are combined. The three most common types of mixed method designs are the convergent parallel design , the explanatory sequential design and the exploratory sequential design. The designs with examples are shown in Fig. 4 .

Three common mixed methods designs
In the convergent parallel design, a qualitative study is conducted in parallel to and independently of a quantitative study, and the results of both studies are compared and combined at the stage of interpretation of results. Using the above example of EVT provision, this could entail setting up a quantitative EVT registry to measure process times and patient outcomes in parallel to conducting the qualitative research outlined above, and then comparing results. Amongst other things, this would make it possible to assess whether interview respondents’ subjective impressions of patients receiving good care match modified Rankin Scores at follow-up, or whether observed delays in care provision are exceptions or the rule when compared to door-to-needle times as documented in the registry. In the explanatory sequential design, a quantitative study is carried out first, followed by a qualitative study to help explain the results from the quantitative study. This would be an appropriate design if the registry alone had revealed relevant delays in door-to-needle times and the qualitative study would be used to understand where and why these occurred, and how they could be improved. In the exploratory design, the qualitative study is carried out first and its results help informing and building the quantitative study in the next step [ 26 ]. If the qualitative study around EVT provision had shown a high level of dissatisfaction among the staff members involved, a quantitative questionnaire investigating staff satisfaction could be set up in the next step, informed by the qualitative study on which topics dissatisfaction had been expressed. Amongst other things, the questionnaire design would make it possible to widen the reach of the research to more respondents from different (types of) hospitals, regions, countries or settings, and to conduct sub-group analyses for different professional groups.
How to assess qualitative research?
A variety of assessment criteria and lists have been developed for qualitative research, ranging in their focus and comprehensiveness [ 14 , 17 , 27 ]. However, none of these has been elevated to the “gold standard” in the field. In the following, we therefore focus on a set of commonly used assessment criteria that, from a practical standpoint, a researcher can look for when assessing a qualitative research report or paper.
Assessors should check the authors’ use of and adherence to the relevant reporting checklists (e.g. Standards for Reporting Qualitative Research (SRQR)) to make sure all items that are relevant for this type of research are addressed [ 23 , 28 ]. Discussions of quantitative measures in addition to or instead of these qualitative measures can be a sign of lower quality of the research (paper). Providing and adhering to a checklist for qualitative research contributes to an important quality criterion for qualitative research, namely transparency [ 15 , 17 , 23 ].
Reflexivity
While methodological transparency and complete reporting is relevant for all types of research, some additional criteria must be taken into account for qualitative research. This includes what is called reflexivity, i.e. sensitivity to the relationship between the researcher and the researched, including how contact was established and maintained, or the background and experience of the researcher(s) involved in data collection and analysis. Depending on the research question and population to be researched this can be limited to professional experience, but it may also include gender, age or ethnicity [ 17 , 27 ]. These details are relevant because in qualitative research, as opposed to quantitative research, the researcher as a person cannot be isolated from the research process [ 23 ]. It may influence the conversation when an interviewed patient speaks to an interviewer who is a physician, or when an interviewee is asked to discuss a gynaecological procedure with a male interviewer, and therefore the reader must be made aware of these details [ 19 ].
Sampling and saturation
The aim of qualitative sampling is for all variants of the objects of observation that are deemed relevant for the study to be present in the sample “ to see the issue and its meanings from as many angles as possible” [ 1 , 16 , 19 , 20 , 27 ] , and to ensure “information-richness [ 15 ]. An iterative sampling approach is advised, in which data collection (e.g. five interviews) is followed by data analysis, followed by more data collection to find variants that are lacking in the current sample. This process continues until no new (relevant) information can be found and further sampling becomes redundant – which is called saturation [ 1 , 15 ] . In other words: qualitative data collection finds its end point not a priori , but when the research team determines that saturation has been reached [ 29 , 30 ].
This is also the reason why most qualitative studies use deliberate instead of random sampling strategies. This is generally referred to as “ purposive sampling” , in which researchers pre-define which types of participants or cases they need to include so as to cover all variations that are expected to be of relevance, based on the literature, previous experience or theory (i.e. theoretical sampling) [ 14 , 20 ]. Other types of purposive sampling include (but are not limited to) maximum variation sampling, critical case sampling or extreme or deviant case sampling [ 2 ]. In the above EVT example, a purposive sample could include all relevant professional groups and/or all relevant stakeholders (patients, relatives) and/or all relevant times of observation (day, night and weekend shift).
Assessors of qualitative research should check whether the considerations underlying the sampling strategy were sound and whether or how researchers tried to adapt and improve their strategies in stepwise or cyclical approaches between data collection and analysis to achieve saturation [ 14 ].
Good qualitative research is iterative in nature, i.e. it goes back and forth between data collection and analysis, revising and improving the approach where necessary. One example of this are pilot interviews, where different aspects of the interview (especially the interview guide, but also, for example, the site of the interview or whether the interview can be audio-recorded) are tested with a small number of respondents, evaluated and revised [ 19 ]. In doing so, the interviewer learns which wording or types of questions work best, or which is the best length of an interview with patients who have trouble concentrating for an extended time. Of course, the same reasoning applies to observations or focus groups which can also be piloted.
Ideally, coding should be performed by at least two researchers, especially at the beginning of the coding process when a common approach must be defined, including the establishment of a useful coding list (or tree), and when a common meaning of individual codes must be established [ 23 ]. An initial sub-set or all transcripts can be coded independently by the coders and then compared and consolidated after regular discussions in the research team. This is to make sure that codes are applied consistently to the research data.
Member checking
Member checking, also called respondent validation , refers to the practice of checking back with study respondents to see if the research is in line with their views [ 14 , 27 ]. This can happen after data collection or analysis or when first results are available [ 23 ]. For example, interviewees can be provided with (summaries of) their transcripts and asked whether they believe this to be a complete representation of their views or whether they would like to clarify or elaborate on their responses [ 17 ]. Respondents’ feedback on these issues then becomes part of the data collection and analysis [ 27 ].
Stakeholder involvement
In those niches where qualitative approaches have been able to evolve and grow, a new trend has seen the inclusion of patients and their representatives not only as study participants (i.e. “members”, see above) but as consultants to and active participants in the broader research process [ 31 – 33 ]. The underlying assumption is that patients and other stakeholders hold unique perspectives and experiences that add value beyond their own single story, making the research more relevant and beneficial to researchers, study participants and (future) patients alike [ 34 , 35 ]. Using the example of patients on or nearing dialysis, a recent scoping review found that 80% of clinical research did not address the top 10 research priorities identified by patients and caregivers [ 32 , 36 ]. In this sense, the involvement of the relevant stakeholders, especially patients and relatives, is increasingly being seen as a quality indicator in and of itself.
How not to assess qualitative research
The above overview does not include certain items that are routine in assessments of quantitative research. What follows is a non-exhaustive, non-representative, experience-based list of the quantitative criteria often applied to the assessment of qualitative research, as well as an explanation of the limited usefulness of these endeavours.
Protocol adherence
Given the openness and flexibility of qualitative research, it should not be assessed by how well it adheres to pre-determined and fixed strategies – in other words: its rigidity. Instead, the assessor should look for signs of adaptation and refinement based on lessons learned from earlier steps in the research process.
Sample size
For the reasons explained above, qualitative research does not require specific sample sizes, nor does it require that the sample size be determined a priori [ 1 , 14 , 27 , 37 – 39 ]. Sample size can only be a useful quality indicator when related to the research purpose, the chosen methodology and the composition of the sample, i.e. who was included and why.
Randomisation
While some authors argue that randomisation can be used in qualitative research, this is not commonly the case, as neither its feasibility nor its necessity or usefulness has been convincingly established for qualitative research [ 13 , 27 ]. Relevant disadvantages include the negative impact of a too large sample size as well as the possibility (or probability) of selecting “ quiet, uncooperative or inarticulate individuals ” [ 17 ]. Qualitative studies do not use control groups, either.
Interrater reliability, variability and other “objectivity checks”
The concept of “interrater reliability” is sometimes used in qualitative research to assess to which extent the coding approach overlaps between the two co-coders. However, it is not clear what this measure tells us about the quality of the analysis [ 23 ]. This means that these scores can be included in qualitative research reports, preferably with some additional information on what the score means for the analysis, but it is not a requirement. Relatedly, it is not relevant for the quality or “objectivity” of qualitative research to separate those who recruited the study participants and collected and analysed the data. Experiences even show that it might be better to have the same person or team perform all of these tasks [ 20 ]. First, when researchers introduce themselves during recruitment this can enhance trust when the interview takes place days or weeks later with the same researcher. Second, when the audio-recording is transcribed for analysis, the researcher conducting the interviews will usually remember the interviewee and the specific interview situation during data analysis. This might be helpful in providing additional context information for interpretation of data, e.g. on whether something might have been meant as a joke [ 18 ].
Not being quantitative research
Being qualitative research instead of quantitative research should not be used as an assessment criterion if it is used irrespectively of the research problem at hand. Similarly, qualitative research should not be required to be combined with quantitative research per se – unless mixed methods research is judged as inherently better than single-method research. In this case, the same criterion should be applied for quantitative studies without a qualitative component.
The main take-away points of this paper are summarised in Table Table1. 1 . We aimed to show that, if conducted well, qualitative research can answer specific research questions that cannot to be adequately answered using (only) quantitative designs. Seeing qualitative and quantitative methods as equal will help us become more aware and critical of the “fit” between the research problem and our chosen methods: I can conduct an RCT to determine the reasons for transportation delays of acute stroke patients – but should I? It also provides us with a greater range of tools to tackle a greater range of research problems more appropriately and successfully, filling in the blind spots on one half of the methodological spectrum to better address the whole complexity of neurological research and practice.
Take-away-points
• Assessing complex multi-component interventions or systems (of change) • What works for whom when, how and why? • Focussing on intervention improvement | • Document study • Observations (participant or non-participant) • Interviews (especially semi-structured) • Focus groups | • Transcription of audio-recordings and field notes into transcripts and protocols • Coding of protocols • Using qualitative data management software |
• Combinations of quantitative and/or qualitative methods, e.g.: • : quali and quanti in parallel • : quanti followed by quali • : quali followed by quanti | • Checklists • Reflexivity • Sampling strategies • Piloting • Co-coding • Member checking • Stakeholder involvement | • Protocol adherence • Sample size • Randomization • Interrater reliability, variability and other “objectivity checks” • Not being quantitative research |
Acknowledgements
Abbreviations.
EVT | Endovascular treatment |
RCT | Randomised Controlled Trial |
SOP | Standard Operating Procedure |
SRQR | Standards for Reporting Qualitative Research |
Authors’ contributions
LB drafted the manuscript; WW and CG revised the manuscript; all authors approved the final versions.
no external funding.
Availability of data and materials
Ethics approval and consent to participate, consent for publication, competing interests.
The authors declare no competing interests.
Publisher’s Note
Springer Nature remains neutral with regard to jurisdictional claims in published maps and institutional affiliations.
Pardon Our Interruption
As you were browsing something about your browser made us think you were a bot. There are a few reasons this might happen:
- You've disabled JavaScript in your web browser.
- You're a power user moving through this website with super-human speed.
- You've disabled cookies in your web browser.
- A third-party browser plugin, such as Ghostery or NoScript, is preventing JavaScript from running. Additional information is available in this support article .
To regain access, please make sure that cookies and JavaScript are enabled before reloading the page.
9 Best Marketing Research Methods to Know Your Buyer Better [+ Examples]

Published: August 08, 2024
One of the most underrated skills you can have as a marketer is marketing research — which is great news for this unapologetic cyber sleuth.
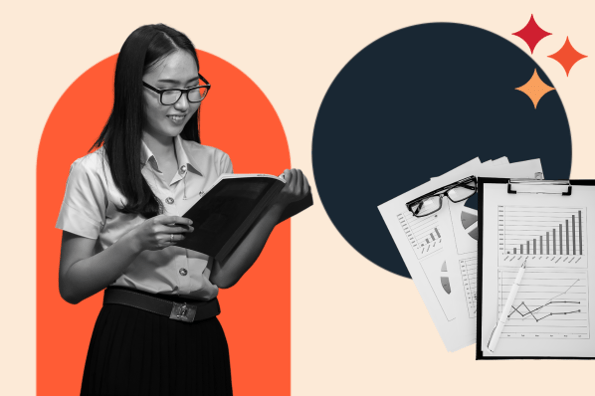
From brand design and product development to buyer personas and competitive analysis, I’ve researched a number of initiatives in my decade-long marketing career.
And let me tell you: having the right marketing research methods in your toolbox is a must.
Market research is the secret to crafting a strategy that will truly help you accomplish your goals. The good news is there is no shortage of options.
How to Choose a Marketing Research Method
Thanks to the Internet, we have more marketing research (or market research) methods at our fingertips than ever, but they’re not all created equal. Let’s quickly go over how to choose the right one.
.png)
Free Market Research Kit
5 Research and Planning Templates + a Free Guide on How to Use Them in Your Market Research
- SWOT Analysis Template
- Survey Template
- Focus Group Template
Download Free
All fields are required.
You're all set!
Click this link to access this resource at any time.
1. Identify your objective.
What are you researching? Do you need to understand your audience better? How about your competition? Or maybe you want to know more about your customer’s feelings about a specific product.
Before starting your research, take some time to identify precisely what you’re looking for. This could be a goal you want to reach, a problem you need to solve, or a question you need to answer.
For example, an objective may be as foundational as understanding your ideal customer better to create new buyer personas for your marketing agency (pause for flashbacks to my former life).
Or if you’re an organic sode company, it could be trying to learn what flavors people are craving.
2. Determine what type of data and research you need.
Next, determine what data type will best answer the problems or questions you identified. There are primarily two types: qualitative and quantitative. (Sound familiar, right?)
- Qualitative Data is non-numerical information, like subjective characteristics, opinions, and feelings. It’s pretty open to interpretation and descriptive, but it’s also harder to measure. This type of data can be collected through interviews, observations, and open-ended questions.
- Quantitative Data , on the other hand, is numerical information, such as quantities, sizes, amounts, or percentages. It’s measurable and usually pretty hard to argue with, coming from a reputable source. It can be derived through surveys, experiments, or statistical analysis.
Understanding the differences between qualitative and quantitative data will help you pinpoint which research methods will yield the desired results.
For instance, thinking of our earlier examples, qualitative data would usually be best suited for buyer personas, while quantitative data is more useful for the soda flavors.
However, truth be told, the two really work together.
Qualitative conclusions are usually drawn from quantitative, numerical data. So, you’ll likely need both to get the complete picture of your subject.
For example, if your quantitative data says 70% of people are Team Black and only 30% are Team Green — Shout out to my fellow House of the Dragon fans — your qualitative data will say people support Black more than Green.
(As they should.)
Primary Research vs Secondary Research
You’ll also want to understand the difference between primary and secondary research.
Primary research involves collecting new, original data directly from the source (say, your target market). In other words, it’s information gathered first-hand that wasn’t found elsewhere.
Some examples include conducting experiments, surveys, interviews, observations, or focus groups.
Meanwhile, secondary research is the analysis and interpretation of existing data collected from others. Think of this like what we used to do for school projects: We would read a book, scour the internet, or pull insights from others to work from.
So, which is better?
Personally, I say any research is good research, but if you have the time and resources, primary research is hard to top. With it, you don’t have to worry about your source's credibility or how relevant it is to your specific objective.
You are in full control and best equipped to get the reliable information you need.
3. Put it all together.
Once you know your objective and what kind of data you want, you’re ready to select your marketing research method.
For instance, let’s say you’re a restaurant trying to see how attendees felt about the Speed Dating event you hosted last week.
You shouldn’t run a field experiment or download a third-party report on speed dating events; those would be useless to you. You need to conduct a survey that allows you to ask pointed questions about the event.
This would yield both qualitative and quantitative data you can use to improve and bring together more love birds next time around.
Best Market Research Methods for 2024
Now that you know what you’re looking for in a marketing research method, let’s dive into the best options.
Note: According to HubSpot’s 2024 State of Marketing report, understanding customers and their needs is one of the biggest challenges facing marketers today. The options we discuss are great consumer research methodologies , but they can also be used for other areas.
Primary Research
1. interviews.
Interviews are a form of primary research where you ask people specific questions about a topic or theme. They typically deliver qualitative information.
I’ve conducted many interviews for marketing purposes, but I’ve also done many for journalistic purposes, like this profile on comedian Zarna Garg . There’s no better way to gather candid, open-ended insights in my book, but that doesn’t mean they’re a cure-all.
What I like: Real-time conversations allow you to ask different questions if you’re not getting the information you need. They also push interviewees to respond quickly, which can result in more authentic answers.
What I dislike: They can be time-consuming and harder to measure (read: get quantitative data) unless you ask pointed yes or no questions.
Best for: Creating buyer personas or getting feedback on customer experience, a product, or content.
2. Focus Groups
Focus groups are similar to conducting interviews but on a larger scale.
In marketing and business, this typically means getting a small group together in a room (or Zoom), asking them questions about various topics you are researching. You record and/or observe their responses to then take action.
They are ideal for collecting long-form, open-ended feedback, and subjective opinions.
One well-known focus group you may remember was run by Domino’s Pizza in 2009 .
After poor ratings and dropping over $100 million in revenue, the brand conducted focus groups with real customers to learn where they could have done better.
It was met with comments like “worst excuse for pizza I’ve ever had” and “the crust tastes like cardboard.” But rather than running from the tough love, it took the hit and completely overhauled its recipes.
The team admitted their missteps and returned to the market with better food and a campaign detailing their “Pizza Turn Around.”
The result? The brand won a ton of praise for its willingness to take feedback, efforts to do right by its consumers, and clever campaign. But, most importantly, revenue for Domino’s rose by 14.3% over the previous year.
The brand continues to conduct focus groups and share real footage from them in its promotion:
What I like: Similar to interviewing, you can dig deeper and pivot as needed due to the real-time nature. They’re personal and detailed.
What I dislike: Once again, they can be time-consuming and make it difficult to get quantitative data. There is also a chance some participants may overshadow others.
Best for: Product research or development
Pro tip: Need help planning your focus group? Our free Market Research Kit includes a handy template to start organizing your thoughts in addition to a SWOT Analysis Template, Survey Template, Focus Group Template, Presentation Template, Five Forces Industry Analysis Template, and an instructional guide for all of them. Download yours here now.
3. Surveys or Polls
Surveys are a form of primary research where individuals are asked a collection of questions. It can take many different forms.
They could be in person, over the phone or video call, by email, via an online form, or even on social media. Questions can be also open-ended or closed to deliver qualitative or quantitative information.
A great example of a close-ended survey is HubSpot’s annual State of Marketing .
In the State of Marketing, HubSpot asks marketing professionals from around the world a series of multiple-choice questions to gather data on the state of the marketing industry and to identify trends.
The survey covers various topics related to marketing strategies, tactics, tools, and challenges that marketers face. It aims to provide benchmarks to help you make informed decisions about your marketing.
It also helps us understand where our customers’ heads are so we can better evolve our products to meet their needs.
Apple is no stranger to surveys, either.
In 2011, the tech giant launched Apple Customer Pulse , which it described as “an online community of Apple product users who provide input on a variety of subjects and issues concerning Apple.”
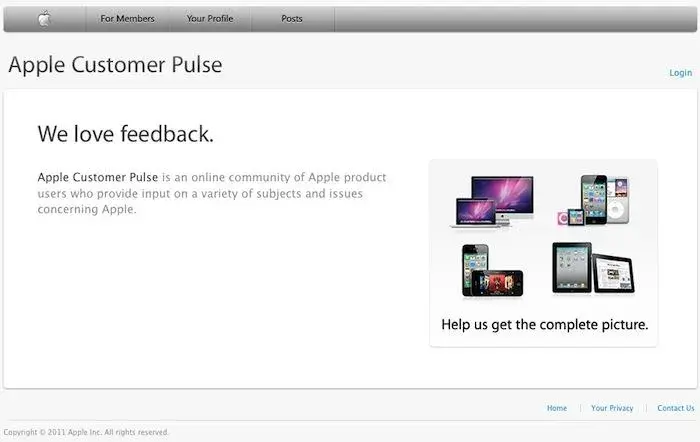
"For example, we did a large voluntary survey of email subscribers and top readers a few years back."
While these readers gave us a long list of topics, formats, or content types they wanted to see, they sometimes engaged more with content types they didn’t select or favor as much on the surveys when we ran follow-up ‘in the wild’ tests, like A/B testing.”
Pepsi saw similar results when it ran its iconic field experiment, “The Pepsi Challenge” for the first time in 1975.
The beverage brand set up tables at malls, beaches, and other public locations and ran a blindfolded taste test. Shoppers were given two cups of soda, one containing Pepsi, the other Coca-Cola (Pepsi’s biggest competitor). They were then asked to taste both and report which they preferred.
People overwhelmingly preferred Pepsi, and the brand has repeated the experiment multiple times over the years to the same results.
What I like: It yields qualitative and quantitative data and can make for engaging marketing content, especially in the digital age.
What I dislike: It can be very time-consuming. And, if you’re not careful, there is a high risk for scientific error.
Best for: Product testing and competitive analysis
Pro tip: " Don’t make critical business decisions off of just one data set," advises Pamela Bump. "Use the survey, competitive intelligence, external data, or even a focus group to give you one layer of ideas or a short-list for improvements or solutions to test. Then gather your own fresh data to test in an experiment or trial and better refine your data-backed strategy."
Secondary Research
8. public domain or third-party research.
While original data is always a plus, there are plenty of external resources you can access online and even at a library when you’re limited on time or resources.
Some reputable resources you can use include:
- Pew Research Center
- McKinley Global Institute
- Relevant Global or Government Organizations (i.e United Nations or NASA)
It’s also smart to turn to reputable organizations that are specific to your industry or field. For instance, if you’re a gardening or landscaping company, you may want to pull statistics from the Environmental Protection Agency (EPA).
If you’re a digital marketing agency, you could look to Google Research or HubSpot Research . (Hey, I know them!)
What I like: You can save time on gathering data and spend more time on analyzing. You can also rest assured the data is from a source you trust.
What I dislike: You may not find data specific to your needs.
Best for: Companies under a time or resource crunch, adding factual support to content
Pro tip: Fellow HubSpotter Iskiev suggests using third-party data to inspire your original research. “Sometimes, I use public third-party data for ideas and inspiration. Once I have written my survey and gotten all my ideas out, I read similar reports from other sources and usually end up with useful additions for my own research.”
9. Buy Research
If the data you need isn’t available publicly and you can’t do your own market research, you can also buy some. There are many reputable analytics companies that offer subscriptions to access their data. Statista is one of my favorites, but there’s also Euromonitor , Mintel , and BCC Research .
What I like: Same as public domain research
What I dislike: You may not find data specific to your needs. It also adds to your expenses.
Best for: Companies under a time or resource crunch or adding factual support to content
Which marketing research method should you use?
You’re not going to like my answer, but “it depends.” The best marketing research method for you will depend on your objective and data needs, but also your budget and timeline.
My advice? Aim for a mix of quantitative and qualitative data. If you can do your own original research, awesome. But if not, don’t beat yourself up. Lean into free or low-cost tools . You could do primary research for qualitative data, then tap public sources for quantitative data. Or perhaps the reverse is best for you.
Whatever your marketing research method mix, take the time to think it through and ensure you’re left with information that will truly help you achieve your goals.
Don't forget to share this post!
Related articles.
![research methods for research paper SWOT Analysis: How To Do One [With Template & Examples]](https://www.hubspot.com/hubfs/marketingplan_20.webp)
SWOT Analysis: How To Do One [With Template & Examples]
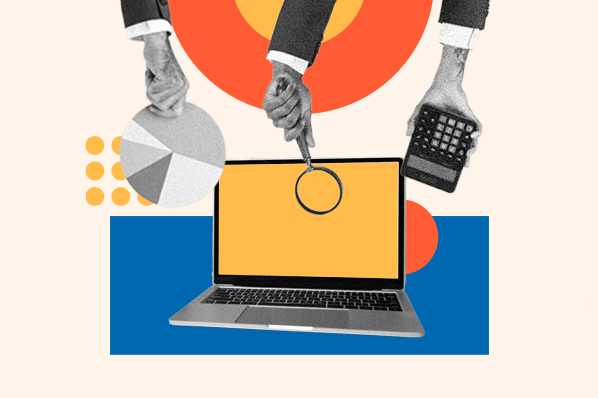
28 Tools & Resources for Conducting Market Research
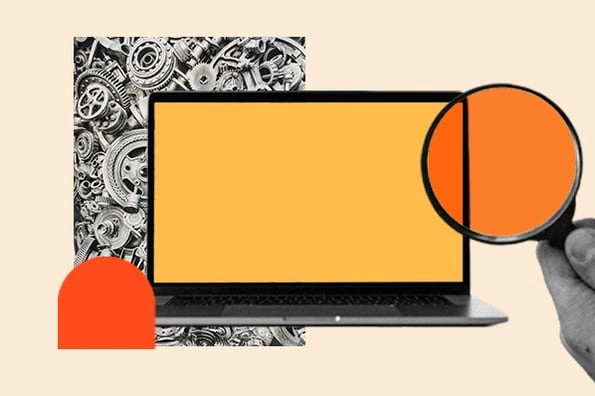
What is a Competitive Analysis — and How Do You Conduct One?
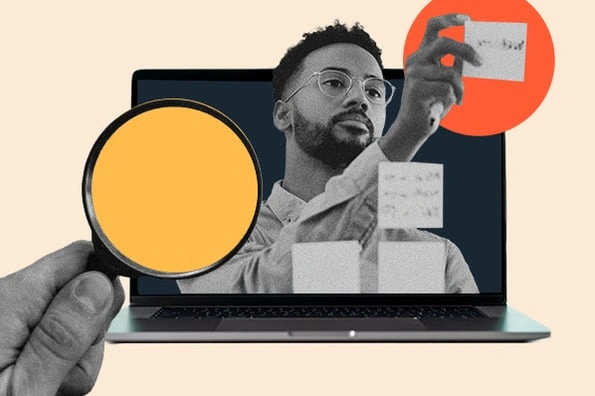
Market Research: A How-To Guide and Template
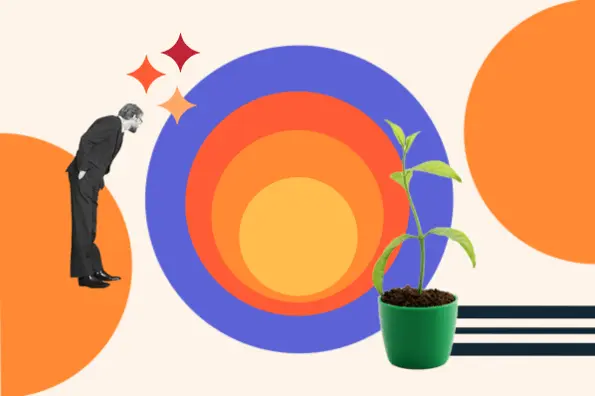
TAM, SAM & SOM: What Do They Mean & How Do You Calculate Them?
![research methods for research paper How to Run a Competitor Analysis [Free Guide]](https://www.hubspot.com/hubfs/Google%20Drive%20Integration/how%20to%20do%20a%20competitor%20analysis_122022.jpeg)
How to Run a Competitor Analysis [Free Guide]
![research methods for research paper 5 Challenges Marketers Face in Understanding Audiences [New Data + Market Researcher Tips]](https://www.hubspot.com/hubfs/challenges%20marketers%20face%20in%20understanding%20the%20customer%20.png)
5 Challenges Marketers Face in Understanding Audiences [New Data + Market Researcher Tips]
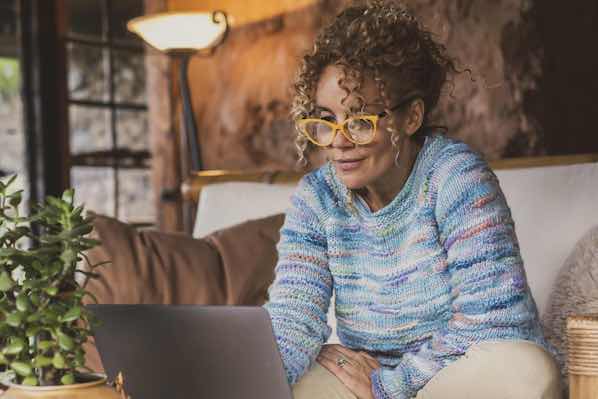
Causal Research: The Complete Guide
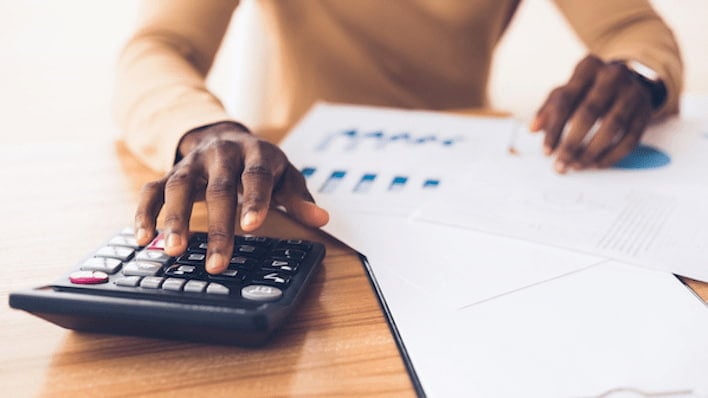
Total Addressable Market (TAM): What It Is & How You Can Calculate It
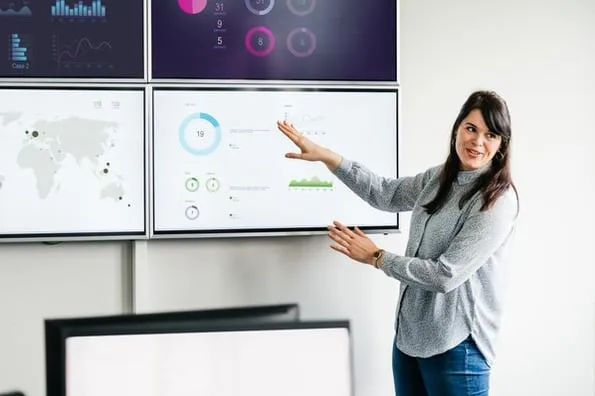
What Is Market Share & How Do You Calculate It?
Free Guide & Templates to Help Your Market Research
Marketing software that helps you drive revenue, save time and resources, and measure and optimize your investments — all on one easy-to-use platform
Click through the PLOS taxonomy to find articles in your field.
For more information about PLOS Subject Areas, click here .
Loading metrics
Open Access
Peer-reviewed
Research Article
Archaeology in space: The Sampling Quadrangle Assemblages Research Experiment (SQuARE) on the International Space Station. Report 1: Squares 03 and 05
Roles Conceptualization, Data curation, Formal analysis, Funding acquisition, Investigation, Methodology, Project administration, Resources, Supervision, Visualization, Writing – original draft, Writing – review & editing
* E-mail: [email protected]
Affiliations Department of Art, Chapman University, Orange, CA, United States of America, Space Engineering Research Center, University of Southern California, Marina del Rey, CA, United States of America

Roles Data curation, Formal analysis, Investigation, Methodology, Resources, Software, Supervision, Validation, Visualization, Writing – original draft, Writing – review & editing
Affiliation Department of History, Carleton University, Ottawa, ON, United States of America
Roles Conceptualization, Data curation, Methodology, Project administration, Supervision, Writing – review & editing
Affiliation College of Humanities, Arts and Social Sciences, Flinders University, Adelaide, Australia
Roles Software, Writing – original draft
Roles Investigation, Writing – original draft
Affiliation Archaeology Research Center, University of Southern California, Los Angeles, CA, United States of America
- Justin St. P. Walsh,
- Shawn Graham,
- Alice C. Gorman,
- Chantal Brousseau,
- Salma Abdullah
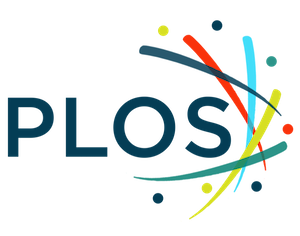
- Published: August 7, 2024
- https://doi.org/10.1371/journal.pone.0304229
- Reader Comments
Between January and March 2022, crew aboard the International Space Station (ISS) performed the first archaeological fieldwork in space, the Sampling Quadrangle Assemblages Research Experiment (SQuARE). The experiment aimed to: (1) develop a new understanding of how humans adapt to life in an environmental context for which we are not evolutionarily adapted, using evidence from the observation of material culture; (2) identify disjunctions between planned and actual usage of facilities on a space station; (3) develop and test techniques that enable archaeological research at a distance; and (4) demonstrate the relevance of social science methods and perspectives for improving life in space. In this article, we describe our methodology, which involves a creative re-imagining of a long-standing sampling practice for the characterization of a site, the shovel test pit. The ISS crew marked out six sample locations (“squares”) around the ISS and documented them through daily photography over a 60-day period. Here we present the results from two of the six squares: an equipment maintenance area, and an area near exercise equipment and the latrine. Using the photographs and an innovative webtool, we identified 5,438 instances of items, labeling them by type and function. We then performed chronological analyses to determine how the documented areas were actually used. Our results show differences between intended and actual use, with storage the most common function of the maintenance area, and personal hygiene activities most common in an undesignated area near locations for exercise and waste.
Citation: Walsh JSP, Graham S, Gorman AC, Brousseau C, Abdullah S (2024) Archaeology in space: The Sampling Quadrangle Assemblages Research Experiment (SQuARE) on the International Space Station. Report 1: Squares 03 and 05. PLoS ONE 19(8): e0304229. https://doi.org/10.1371/journal.pone.0304229
Editor: Peter F. Biehl, University of California Santa Cruz, UNITED STATES OF AMERICA
Received: March 9, 2024; Accepted: May 7, 2024; Published: August 7, 2024
Copyright: © 2024 Walsh et al. This is an open access article distributed under the terms of the Creative Commons Attribution License , which permits unrestricted use, distribution, and reproduction in any medium, provided the original author and source are credited.
Data Availability: All relevant data are within the paper and its Supporting Information files.
Funding: JW was the recipient of funding from Chapman University’s Office of Research and Sponsored Programs to support the activities of Axiom Space as implementation partner for the research presented in this article. There are no associated grant numbers for this financial support. Axiom Space served in the role of a contractor hired by Chapman University for the purpose of overseeing logistics relating to our research. In-kind support in the form of ISS crew time and access to the space station’s facilities, also awarded to JW from the ISS National Laboratory, resulted from an unsolicited proposal, and therefore there is no opportunity title or number associated with our work. No salary was received by any of the investigators as a result of the grant support. No additional external funding was received for this study.
Competing interests: The authors have declared that no competing interests exist.
Introduction
The International Space Station Archaeological Project (ISSAP) aims to fill a gap in social science investigation into the human experience of long-duration spaceflight [ 1 – 3 ]. As the largest, most intensively inhabited space station to date, with over 270 visitors from 23 countries during more than 23 years of continuous habitation, the International Space Station (ISS) is the ideal example of a new kind of spacefaring community—“a microsociety in a miniworld” [ 4 ]. While it is possible to interview crew members about their experiences, the value of an approach focused on material culture is that it allows identification of longer-term patterns of behaviors and associations that interlocutors are unable or even unwilling to articulate. In this respect, we are inspired by previous examples of contemporary archaeology such as the Tucson Garbage Project and the Undocumented Migration Project [ 5 – 7 ]. We also follow previous discussions of material culture in space contexts that highlight the social and cultural features of space technology [ 8 , 9 ].
Our primary goal is to identify how humans adapt to life in a new environment for which our species has not evolved, one characterized by isolation, confinement, and especially microgravity. Microgravity introduces opportunities, such as the ability to move and work in 360 degrees, and to carry out experiments impossible in full Earth gravity, but also limitations, as unrestrained objects float away. The most routine activities carried out on Earth become the focus of intense planning and technological intervention in microgravity. By extension, our project also seeks to develop archaeological techniques that permit the study of other habitats in remote, extreme, or dangerous environments [ 10 , 11 ]. Since it is too costly and difficult to visit our archaeological site in person, we have to creatively re-imagine traditional archaeological methods to answer key questions. To date, our team has studied crew-created visual displays [ 12 , 13 ], meanings and processes associated with items returned to Earth [ 14 ], distribution of different population groups around the various modules [ 15 ], and the development of machine learning (ML) computational techniques to extract data about people and places, all from historic photographs of life on the ISS [ 16 ].
From January to March 2022, we developed a new dataset through the first archaeological work conducted off-Earth. We documented material culture in six locations around the ISS habitat, using daily photography taken by the crew which we then annotated and studied as evidence for changes in archaeological assemblages of material culture over time. This was the first time such data had been captured in a way that allowed statistical analysis. Here, we present the data and results from Squares 03 and 05, the first two sample locations to be completed.
Materials and methods
Square concept and planning.
Gorman proposed the concept behind the investigation, deriving it from one of the most traditional terrestrial archaeological techniques, the shovel test pit. This method is used to understand the overall characteristics of a site quickly through sampling. A site is mapped with a grid of one-meter squares. Some of the squares are selected for initial excavation to understand the likely spatial and chronological distribution of features across the entire site. In effect, the technique is a way to sample a known percentage of the entire site systematically. In the ISS application of this method, we documented a notional stratigraphy through daily photography, rather than excavation.
Historic photography is a key dataset for the International Space Station Archaeological Project. Tens of thousands of images have been made available to us, either through publication [ 17 ], or through an arrangement with the ISS Research Integration Office, which supplied previously unpublished images from the first eight years of the station’s habitation. These photographs are informative about the relationships between people, places, and objects over time in the ISS. However, they were taken randomly (from an archaeological perspective) and released only according to NASA’s priorities and rules. Most significantly, they were not made with the purpose of answering archaeological questions. By contrast, the photographs taken during the present investigation were systematic, representative of a defined proportion of the habitat’s area, and targeted towards capturing archaeology’s primary evidence: material culture. We were interested in how objects move around individual spaces and the station, what these movements revealed about crew adherence to terrestrial planning, and the creative use of material culture to make the laboratory-like interior of the ISS more habitable.
Access to the field site was gained through approval of a proposal submitted to the Center for the Advancement of Science in Space (also known as the ISS National Laboratory [ISS NL]). Upon acceptance, Axiom Space was assigned as the Implementation Partner for carriage of the experiment according to standard procedure. No other permits were required for this work.
Experiment design
Since our work envisioned one-meter sample squares, and recognizing the use of acronyms as a persistent element of spacefaring culture, we named our payload the Sampling Quadrangle Assemblages Research Experiment (SQuARE). Permission from the ISS NL to conduct SQuARE was contingent on using equipment that was already on board the space station. SQuARE required only five items: a camera, a wide-angle lens, adhesive tape (for marking the boundaries of the sample locations), a ruler (for scale), and a color calibration card (for post-processing of the images). All of these were already present on the ISS.
Walsh performed tests on the walls of a terrestrial art gallery to assess the feasibility of creating perfect one-meter squares in microgravity. He worked on a vertical surface, using the Pythagorean theorem to determine where the corners should be located. The only additional items used for these tests were two metric measuring tapes and a pencil for marking the wall (these were also already on the ISS). While it was possible to make a square this way, it also became clear that at least two people were needed to manage holding the tape measures in position while marking the points for the corners. This was not possible in the ISS context.
Walsh and Gorman identified seven locations for the placement of squares. Five of these were in the US Orbital Segment (USOS, consisting of American, European, and Japanese modules) and two in the Russian Orbital Segment. Unfortunately, tense relations between the US and Russian governments meant we could only document areas in the USOS. The five locations were (with their SQuARE designations):
- 01—an experimental rack on the forward wall, starboard end, of the Japanese Experiment Module
- 02—an experimental rack on the forward wall, port end, of the European laboratory module Columbus
- 03—the starboard Maintenance Work Area (workstation) in the US Node 2 module
- 04—the wall area “above” (according to typical crew body orientation) the galley table in the US Node 1 module
- 05—the aft wall, center location, of the US Node 3 module
Our square selection encompassed different modules and activities, including work and leisure. We also asked the crew to select a sixth sample location based on their understanding of the experiment and what they thought would be interesting to document. They chose a workstation on the port wall of the US laboratory module, at the aft end, which they described in a debriefing following their return to Earth in June 2022 as “our central command post, like our shared office situation in the lab.” Results from the four squares not included here will appear in future publications.
Walsh worked with NASA staff to determine payload procedures, including precise locations for the placement of the tape that would mark the square boundaries. The squares could not obstruct other facilities or experiments, so (unlike in terrestrial excavations, where string is typically used to demarcate trench boundaries) only the corners of each square were marked, not the entire perimeter. We used Kapton tape due to its bright yellow-orange color, which aided visibility for the crew taking photographs and for us when cropping the images. In practice, due to space constraints, the procedures that could actually be performed by crew in the ISS context, and the need to avoid interfering with other ongoing experiments, none of the locations actually measured one square meter or had precise 90° corners like a trench on Earth.
On January 14, 2022, NASA astronaut Kayla Barron set up the sample locations, marking the beginning of archaeological work in space ( S1 Movie ). For 30 days, starting on January 21, a crew member took photos of the sample locations at approximately the same time each day; the process was repeated at a random time each day for a second 30-day period to eliminate biases. Photography ended on March 21, 2022. The crew were instructed not to move any items prior to taking the photographs. Walsh led image management, including color and barrel distortion correction, fixing the alignment of each image, and cropping them to the boundaries of the taped corners.
Data processing—Item tagging, statistics, visualizations
We refer to each day’s photo as a “context” by analogy with chronologically-linked assemblages of artifacts and installations at terrestrial archaeological sites ( S1 and S2 Datasets). As previously noted, each context represented a moment roughly 24 hours distant from the previous one, showing evidence of changes in that time. ISS mission planners attempted to schedule the activity at the same time in the first month, but there were inevitable changes due to contingencies. Remarkably, the average time between contexts in Phase 1 was an almost-perfect 24h 0m 13s. Most of the Phase 1 photos were taken between 1200 and 1300 GMT (the time zone in which life on the ISS is organized). In Phase 2, the times were much more variable, but the average time between contexts during this period was still 23h 31m 45s. The earliest Phase 2 photo was taken at 0815 GMT, and the latest at 2101. We did not identify any meaningful differences between results from the two phases.
Since the “test pits” were formed of images rather than soil matrices, we needed a tool to capture information about the identity, nature, and location of every object. An open-source image annotator platform [ 18 ] mostly suited our needs. Brousseau rebuilt the platform to work within the constraints of our access to the imagery (turning it into a desktop tool with secure access to our private server), to permit a greater range of metadata to be added to each item or be imported, to autosave, and to export the resulting annotations. The tool also had to respect privacy and security limitations required by NASA.
The platform Brousseau developed and iterated was rechristened “Rocket-Anno” ( S1 File ). For each context photograph, the user draws an outline around every object, creating a polygon; each polygon is assigned a unique ID and the user provides the relevant descriptive information, using a controlled vocabulary developed for ISS material culture by Walsh and Gorman. Walsh and Abdullah used Rocket-Anno to tag the items in each context for Squares 03 and 05. Once all the objects were outlined for every context’s photograph, the tool exported a JSON file with all of the metadata for both the images themselves and all of the annotations, including the coordinate points for every polygon ( S3 Dataset ). We then developed Python code using Jupyter “notebooks” (an interactive development environment) that ingests the JSON file and generates dataframes for various facets of the data. Graham created a “core” notebook that exports summary statistics, calculates Brainerd-Robinson coefficients of similarity, and visualizes the changing use of the square over time by indicating use-areas based on artifact types and subtypes ( S2 File ). Walsh and Abdullah also wrote detailed square notes with context-by-context discussions and interpretations of features and patterns.
We asked NASA for access to the ISS Crew Planner, a computer system that shows each astronaut’s tasks in five-minute increments, to aid with our interpretation of contexts, but were denied. As a proxy, we use another, less detailed source: the ISS Daily Summary Reports (DSRs), published on a semi-regular basis by NASA on its website [ 19 ]. Any activities mentioned in the DSRs often must be connected with a context by inference. Therefore, our conclusions are likely less precise than if we had seen the Crew Planner, but they also more clearly represent the result of simply observing and interpreting the material culture record.
The crew during our sample period formed ISS Expedition 66 (October 2021-March 2022). They were responsible for the movement of objects in the sample squares as they carried out their daily tasks. The group consisted of two Russians affiliated with Roscosmos (the Russian space agency, 26%), one German belonging to the European Space Agency (ESA, 14%), and four Americans employed by NASA (57%). There were six men (86%) and one woman (14%), approximately equivalent to the historic proportions in the ISS population (84% and 16%, respectively). The Russian crew had their sleeping quarters at the aft end of the station, in the Zvezda module. The ESA astronaut slept in the European Columbus laboratory module. The four NASA crew slept in the US Node 2 module (see below). These arrangements emphasize the national character of discrete spaces around the ISS, also evident in our previous study of population distributions [ 15 ]. Both of the sample areas in this study were located in US modules.
Square 03 was placed in the starboard Maintenance Work Area (MWA, Fig 1 ), one of a pair of workstations located opposite one another in the center of the Node 2 module, with four crew berths towards the aft and a series of five ports for the docking of visiting crew/cargo vehicles and two modules on the forward end ( Fig 2 ). Node 2 (sometimes called “Harmony”) is a connector that links the US, Japanese, and European lab modules. According to prevailing design standards when the workstation was developed, an MWA “shall serve as the primary location for servicing and repair of maximum sized replacement unit/system components” [ 20 ]. Historic images published by NASA showing its use suggested that its primary function was maintenance of equipment and also scientific work that did not require a specific facility such as a centrifuge or furnace.
- PPT PowerPoint slide
- PNG larger image
- TIFF original image
An open crew berth is visible at right. The yellow dotted line indicates the boundaries of the sample area. Credit: NASA/ISSAP.
https://doi.org/10.1371/journal.pone.0304229.g001
Credit: Tor Finseth, by permission, modified by Justin Walsh.
https://doi.org/10.1371/journal.pone.0304229.g002
Square 03 measured 90.3 cm (top) x 87.8 (left) x 89.4 (bottom) x 87.6 (right), for an area of approximately 0.79 m 2 . Its primary feature was a blue metal panel with 40 square loop-type Velcro patches arranged in four rows of ten. During daily photography, many items were attached to the Velcro patches (or held by a clip or in a resealable bag which had its own hook-type Velcro). Above and below the blue panel were additional Velcro patches placed directly on the white plastic wall surface. These patches were white, in different sizes and shapes and irregularly arranged, indicating that they had been placed on the wall in response to different needs. Some were dirty, indicating long use. The patches below the blue panel were rarely used during the sample period, but the patches above were used frequently to hold packages of wet wipes, as well as resealable bags with electrostatic dispersion kits and other items. Outside the sample area, the primary features were a crew berth to the right, and a blue metal table attached to the wall below. This table, the primary component of the MWA, “provides a rigid surface on which to perform maintenance tasks,” according to NASA [ 21 ]. It is modular and can be oriented in several configurations, from flat against the wall to horizontal ( i . e ., perpendicular to the wall). A laptop to the left of the square occasionally showed information about work happening in the area.
In the 60 context photos of Square 03, we recorded 3,608 instances of items, an average of 60.1 (median = 60.5) per context. The lowest count was 24 in context 2 (where most of the wall was hidden from view behind an opaque storage bag), and the highest was 75 in both contexts 20 and 21. For comparison between squares, we can also calculate the item densities per m 2 . The average count was 76.1/m 2 (minimum = 30, maximum = 95). The count per context ( Fig 3(A)) began much lower than average in the first three contexts because of a portable glovebag and a stowage bag that obscured much of the sample square. It rose to an above-average level which was sustained (with the exception of contexts 11 and 12, which involved the appearance of another portable glovebag) until about context 43, when the count dipped again and the area seemed to show less use. Contexts 42–59 showed below-average numbers, as much as 20% lower than previously.
(a) Count of artifacts in Square 03 over time. (b) Proportions of artifacts by function in Square 03. Credit: Rao Hamza Ali.
https://doi.org/10.1371/journal.pone.0304229.g003
74 types of items appeared at least once here, belonging to six categories: equipment (41%), office supplies (31%), electronic (17%), stowage (9%), media (1%), and food (<1%). To better understand the significance of various items in the archaeological record, we assigned them to functional categories ( Table 1 , Fig 3(B)) . 35% of artifacts were restraints, or items used for holding other things in place; 12% for tools; 9% for containers; 9% for writing items; 6% for audiovisual items; 6% for experimental items; 4% for lights; 4% for safety items; 4% for body maintenance; 4% for power items; 3% for computing items; 1% for labels; and less than 1% drinks. We could not identify a function for two percent of the items.
https://doi.org/10.1371/journal.pone.0304229.t001
One of the project goals is understanding cultural adaptations to the microgravity environment. We placed special attention on “gravity surrogates,” pieces of (often simple) technology that are used in space to replicate the terrestrial experience of things staying where they are placed. Gravity surrogates include restraints and containers. It is quite noticeable that gravity surrogates comprise close to half of all items (44%) in Square 03, while the tools category, which might have been expected to be most prominent in an area designated for maintenance, is less than one-third as large (12%). Adding other groups associated with work, such as “experiment” and “light,” only brings the total to 22%.
Square 05 (Figs 2 and 4 ) was placed in a central location on the aft wall of the multipurpose Node 3 (“Tranquility”) module. This module does not include any specific science facilities. Instead, there are two large pieces of exercise equipment, the TVIS (Treadmill with Vibration Isolation Stabilization System, on the forward wall at the starboard end), and the ARED (Advanced Resistive Exercise Device, on the overhead wall at the port end). Use of the machines forms a significant part of crew activities, as they are required to exercise for two hours each day to counteract loss of muscle mass and bone density, and enable readjustment to terrestrial gravity on their return. The Waste and Hygiene Compartment (WHC), which includes the USOS latrine, is also here, on the forward wall in the center of the module, opposite Square 05. Finally, three modules are docked at Node 3’s port end. Most notable is the Cupola, a kind of miniature module on the nadir side with a panoramic window looking at Earth. This is the most popular leisure space for the crew, who often describe the hours they spend there. The Permanent Multipurpose Module (PMM) is docked on the forward side, storing equipment, food, and trash. In previous expeditions, some crew described installing a curtain in the PMM to create a private space for changing clothes and performing body maintenance activities such as cleaning oneself [ 22 , 23 ], but it was unclear whether that continued to be its function during the expedition we observed. One crew member during our sample period posted a video on Instagram showing the PMM interior and their efforts to re-stow equipment in a bag [ 24 ]. The last space attached to Node 3 is an experimental inflatable module docked on the aft side, called the Bigelow Expandable Activity Module (BEAM), which is used for storage of equipment.
The yellow dotted line indicates the boundaries of the sample area. The ARED machine is at the far upper right, on the overhead wall. The TVIS treadmill is outside this image to the left, on the forward wall. The WHC is directly behind the photographer. Credit: NASA/ISSAP.
https://doi.org/10.1371/journal.pone.0304229.g004
Square 05 was on a mostly featureless wall, with a vertical handrail in the middle. Handrails are metal bars located throughout the ISS that are used by the crew to hold themselves in place or provide a point from which to propel oneself to another location. NASA’s most recent design standards acknowledge that “[t]hey also serve as convenient locations for temporary mounting, affixing, or restraint of loose equipment and as attachment points for equipment” [ 25 ]. The handrail in Square 05 was used as an impromptu object restraint when a resealable bag filled with other bags was squeezed between the handrail and the wall.
The Brine Processing Assembly (BPA), a white plastic box which separates water from other components of urine for treatment and re-introduction to the station’s drinkable water supply [ 26 ], was fixed to the wall outside the square boundaries at lower left. A bungee cord was attached to both sides of the box; the one on the right was connected at its other end to the handrail attachment bracket. Numerous items were attached to or wedged into this bungee cord during the survey, bringing “gravity” into being. A red plastic duct ran through the square from top center into the BPA. This duct led from the latrine via the overhead wall. About halfway through the survey period, in context 32, the duct was wrapped in Kapton tape. According to the DSR for that day, “the crew used duct tape [ sic ] to make a seal around the BPA exhaust to prevent odor permeation in the cabin” [ 27 ], revealing an aspect of the crew’s experience of this area that is captured only indirectly in the context photograph. Permanently attached to the wall were approximately 20 loop-type Velcro patches in many shapes and sizes, placed in a seemingly random pattern that likely indicates that they were put there at different times and for different reasons.
Other common items in Square 05 were a mirror, a laptop computer, and an experimental item belonging to the German space agency DLR called the Touch Array Assembly [ 28 ]. The laptop moved just three times, and only by a few centimeters each time, during the sample period. The Touch Array was a black frame enclosing three metal surfaces which were being tested for their bacterial resistance; members of the crew touched the surfaces at various moments during the sample period. Finally, and most prominent due to its size, frequency of appearance, and use (judged by its movement between context photos) was an unidentified crew member’s toiletry kit.
By contrast with Square 03, 05 was the most irregular sample location, roughly twice as wide as it was tall. Its dimensions were 111 cm (top) x 61.9 (left) x 111.4 (bottom) x 64.6 (right), for an area of approximately 0.7 m 2 , about 89% of Square 03. We identified 1,830 instances of items in the 60 contexts, an average of 30.5 (median = 32) per context. The minimum was 18 items in context 5, and the maximum was 39 in contexts 24, 51, and 52. The average item density was 43.6/m 2 (minimum = 26, maximum = 56), 57% of Square 03.
The number of items trended upward throughout the sample period ( Fig 5(A)) . The largest spike occurred in context 6 with the appearance of the toiletry kit, which stored (and revealed) a number of related items. The kit can also be linked to one of the largest dips in item count, seen from contexts 52 to 53, when it was closed (but remained in the square). Other major changes can often be attributed to the addition and removal of bungee cords, which had other items such as carabiners and brackets attached. For example, the dip seen in context 25 correlates with the removal of a bungee cord with four carabiners.
(a) Count of artifacts and average count in Square 05 over time. (b) Proportions of artifacts by function in Square 05. Credit: Rao Hamza Ali.
https://doi.org/10.1371/journal.pone.0304229.g005
41 different item types were found in Square 05, about 55% as many as in Square 03. These belonged to five different categories: equipment (63%), electronic (17%), stowage (10%), office supplies (5%), and food (2%). The distribution of function proportions was quite different in this sample location ( Table 2 and Fig 5(B)) . Even though restraints were still most prominent, making up 32% of all items, body maintenance was almost as high (30%), indicating how strongly this area was associated with the activity of cleaning and caring for oneself. Computing (8%, represented by the laptop, which seems not to have been used), power (8%, from various cables), container (7%, resealable bags and Cargo Transfer Bags), and hygiene (6%, primarily the BPA duct) were the next most common items. Experiment was the function of 4% of the items, mostly the Touch Array, which appeared in every context, followed by drink (2%) and life support (1%). Safety, audiovisual, food, and light each made up less than 1% of the functional categories.
https://doi.org/10.1371/journal.pone.0304229.t002
Tracking changes over time is critical to understanding the activity happening in each area. We now explore how the assemblages change by calculating the Brainerd-Robinson Coefficient of Similarity [ 29 , 30 ] as operationalized by Peeples [ 31 , 32 ]. This metric is used in archaeology for comparing all pairs of the contexts by the proportions of categorical artifact data, here functional type. Applying the coefficient to the SQuARE contexts enables identification of time periods for distinct activities using artifact function and frequency alone, independent of documentary or oral evidence.
Multiple phases of activities took place in the square. Moments of connected activity are visible as red clusters in contexts 0–2, 11–12, 28–32, and 41 ( Fig 6(A)) . Combining this visualization with close observation of the photos themselves, we argue that there are actually eight distinct chronological periods.
- Contexts 0–2: Period 1 (S1 Fig in S3 File ) is a three-day period of work involving a portable glovebag (contexts 0–1) and a large blue stowage bag (context 2). It is difficult to describe trends in functional types because the glovebag and stowage bag obstruct the view of many objects. Items which appear at the top of the sample area, such as audiovisual and body maintenance items, are overemphasized in the data as a result. It appears that some kind of science is happening here, perhaps medical sample collection due to the presence of several small resealable bags visible in the glovebag. The work appears particularly intense in context 1, with the positioning of the video camera and light to point into the glovebag. These items indicate observation and oversight of crew activities by ground control. A white cargo transfer bag for storage and the stowage bag for holding packing materials in the context 2 photo likely relate to the packing of a Cargo Dragon vehicle that was docked to Node 2. The Dragon departed from the ISS for Earth, full of scientific samples, equipment, and crew personal items, a little more than three hours after the context 2 photo was taken [ 33 ].
- Contexts 3–10: Period 2 (S2 Fig in S3 File ) was a “stable” eight-day period in the sample, when little activity is apparent, few objects were moved or transferred in or out the square, and the primary function of the area seems to be storage rather than work. In context 6, a large Post-It notepad appeared in the center of the metal panel with a phone number written on it. This number belonged to another astronaut, presumably indicating that someone on the ISS had been told to call that colleague on the ground (for reasons of privacy, and in accordance with NASA rules for disseminating imagery, we have blurred the number in the relevant images). In context 8, the same notepad sheet had new writing appear on it, this time reading “COL A1 L1,” the location of an experimental rack in the European lab module.
- Contexts 11–12: Period 3 (S3 Fig in S3 File ) involves a second appearance of a portable glovebag (a different one from that used in contexts 0–1, according to its serial number), this time for a known activity, a concrete hardening experiment belonging to the European Space Agency [ 34 , 35 ]. This two-day phase indicates how the MWA space can be shared with non-US agencies when required. It also demonstrates the utility of this flexible area for work beyond biology/medicine, such as material science. Oversight of the crew’s activities by ground staff is evident from the positioning of the video camera and LED light pointing into the glovebag.
- Contexts 13–27: Period 4 (S4 Fig in S3 File ) is another stable fifteen-day period, similar to Period 2. Many items continued to be stored on the aluminum panel. The LED light’s presence is a trace of the activity in Period 3 that persists throughout this phase. Only in context 25 can a movement of the lamp potentially be connected to an activity relating to one of the stored items on the wall: at least one nitrile glove was removed from a resealable bag behind the lamp. In general, the primary identifiable activity during Period 4 is storage.
- Contexts 28–32: Period 5 (S5 Fig in S3 File ), by contrast, represents a short period of five days of relatively high and diverse activity. In context 28, a Microsoft Hololens augmented reality headset appeared. According to the DSR for the previous day, a training activity called Sidekick was carried out using the headset [ 36 ]. The following day, a Saturday, showed no change in the quantity or type of objects, but many were moved around and grouped by function—adhesive tape rolls were placed together, tools were moved from Velcro patches into pouches or straightened, and writing implements were placed in a vertical orientation when previously they were tilted. Context 29 represents a cleaning and re-organization of the sample area, which is a common activity for the crew on Saturdays [ 37 ]. Finally, in context 32, an optical coherence tomography scanner—a large piece of equipment for medical research involving crew members’ eyes—appeared [ 38 ]. This device was used previously during the sample period, but on the same day as the ESA concrete experiment, so that earlier work seems to have happened elsewhere [ 39 ].
- Contexts 33–40: Period 6 (S6 Fig in S3 File ) is the third stable period, in which almost no changes are visible over eight days. The only sign of activity is a digital timer which was started six hours before the context 39 image was made and continued to run at least through context 42.
- Context 41: Period 7 (S7 Fig in S3 File ) is a single context in which medical sample collection may have occurred. Resealable bags (some holding others) appeared in the center of the image and at lower right. One of the bags at lower right had a printed label reading “Reservoir Containers.” We were not able to discern which type of reservoir containers the label refers to, although the DSR for the day mentions “[Human Research Facility] Generic Saliva Collection,” without stating the location for this work [ 40 ]. Evidence from photos of other squares shows that labeled bags could be re-used for other purposes, so our interpretation of medical activity for this context is not conclusive.
- Contexts 42–60: Period 8 (S8 Fig in S3 File ) is the last and longest period of stability and low activity—eighteen days in which no specific activity other than the storage of items can be detected. The most notable change is the appearance for the first time of a foil water pouch in the central part of the blue panel.
Visualization of Brainerd-Robinson similarity, compared context-by-context by item function, for (a) Square 03 and (b) Square 05. The more alike a pair of contexts is, the higher the coefficient value, with a context compared against itself where a value of 200 equals perfect similarity. The resulting matrix of coefficients is visualized on a scale from blue to red where blue is lowest and red is highest similarity. The dark red diagonal line indicates complete similarity, where each context is compared to itself. Dark blue represents a complete difference. Credit: Shawn Graham.
https://doi.org/10.1371/journal.pone.0304229.g006
In the standards used at the time of installation, “stowage space” was the sixth design requirement listed for the MWA after accessibility; equipment size capability; scratch-resistant surfaces; capabilities for electrical, mechanical, vacuum, and fluid support during maintenance; and the accommodation of diagnostic equipment [ 20 ]. Only capabilities for fabrication were listed lower than stowage. Yet 50 of the 60 contexts (83%) fell within stable periods where little or no activity is identifiable in Square 03. According to the sample results, therefore, this area seems to exist not for “maintenance,” but primarily for the storage and arrangement of items. The most recent update of the design standards does not mention the MWA, but states, “Stowage location of tool kits should be optimized for accessibility to workstations and/or maintenance workbenches” [ 25 ]. Our observation confirms the importance of this suggestion.
The MWA was also a flexible location for certain science work, like the concrete study or crew health monitoring. Actual maintenance of equipment was hardly in evidence in the sample (possibly contexts 25, 39, and 44), and may not even have happened at all in this location. Some training did happen here, such as review of procedures for the Electromagnetic Levitator camera (instructions for changing settings on a high-speed camera appeared on the laptop screen; the day’s DSR shows that this camera is part of the Electromagnetic Levitator facility, located in the Columbus module [ 41 ]. The training required the use of the Hololens system (context 28 DSR, cited above).
Although many item types were represented in Square 03, it became clear during data capture how many things were basically static, unmoving and therefore unused, especially certain tools, writing implements, and body maintenance items. The MWA was seen as an appropriate place to store these items. It may be the case that their presence here also indicates that their function was seen as an appropriate one for this space, but the function(s) may not be carried out—or perhaps not in this location. Actualization of object function was only visible to us when the state of the item changed—it appeared, it moved, it changed orientation, it disappeared, or, in the case of artifacts that were grouped in collections rather than found as singletons, its shape changed or it became visibly smaller/lesser. We therefore have the opportunity to explore not only actuality of object use, but also potentiality of use or function, and the meaning of that quality for archaeological interpretation [ 42 , 43 ]. This possibility is particularly intriguing in light of the archaeological turn towards recognizing the agency of objects to impact human activity [ 44 , 45 ]. We will explore these implications in a future publication.
We performed the same chronological analysis for Square 05. Fig 6(B) represents the analysis for both item types and for item functions. We identified three major phases of activity, corresponding to contexts 0–5, 6–52, and 53–59 (S9-S11 Figs in S3 File ). The primary characteristics of these phases relate to an early period of unclear associations (0–5) marked by the presence of rolls of adhesive tape and a few body maintenance items (toothpaste and toothbrush, wet wipes); the appearance of a toiletry kit on the right side of the sample area, fully open with clear views of many of the items contained within (6–52); and finally, the closure of the toiletry kit so that its contents can no longer be seen (53–59). We interpret the phases as follows:
- Contexts 0–5: In Period 1 (six days, S9 Fig in S3 File ), while items such as a mirror, dental floss picks, wet wipes, and a toothbrush held in the end of a toothpaste tube were visible, the presence of various other kinds of items confounds easy interpretation. Two rolls of duct tape were stored on the handrail in the center of the sample area, and the Touch Array and laptop appeared in the center. Little movement can be identified, apart from a blue nitrile glove that appeared in context 1 and moved left across the area until it was wedged into the bungee cord for contexts 3 and 4. The tape rolls were removed prior to context 5. A collection of resealable bags was wedged behind the handrail in context 3, remaining there until context 9. Overall, this appears to be a period characterized by eclectic associations, showing an area without a clear designated function.
- Contexts 6–52: Period 2 (S10 Fig in S3 File ) is clearly the most significant one for this location due to its duration (47 days). It was dominated by the number of body maintenance items located in and around the toiletry kit, especially a white hand towel (on which a brown stain was visible from context 11, allowing us to confirm that the same towel was present until context 46). A second towel appeared alongside the toiletry kit in context 47, and the first one was fixed at the same time to the handrail, where it remained through the end of the sample period. A third towel appeared in context 52, attached to the handrail together with the first one by a bungee cord, continuing to the end of the sample period. Individual body maintenance items moved frequently from one context to the next, showing the importance of this type of activity for this part of Node 3. For reasons that are unclear, the mirror shifted orientation from vertical to diagonal in context 22, and then was put back in a vertical orientation in context 31 (a Monday, a day which is not traditionally associated with cleaning and organization). Collections of resealable bags appeared at various times, including a large one labeled “KYNAR BAG OF ZIPLOCKS” in green marker at the upper left part of the sample area beginning of context 12 (Kynar is a non-flammable plastic material that NASA prefers for resealable bags to the generic commercial off-the-shelf variety because it is non-flammable; however, its resistance to heat makes it less desirable for creating custom sizes, so bags made from traditional but flammable low-density polyethylene still dominate on the ISS [ 14 ]). The Kynar bag contained varying numbers of bags within it over time; occasionally, it appeared to be empty. The Touch Array changed orientation on seven of 47 days in period 2, or 15% of the time (12% of all days in the survey), showing activity associated with scientific research in this area. In context 49, a life-support item, the Airborne Particulate Monitor (APM) was installed [ 46 ]. This device, which measures “real-time particulate data” to assess hazards to crew health [ 47 ], persisted through the end of the sample period.
- Contexts 53–59: Period 3 (S11 Fig in S3 File ) appears as a seven-day phase marked by low activity. Visually, the most notable feature is the closure of the toiletry kit, which led to much lower counts of body maintenance items. Hardly any of the items on the wall moved at all during this period.
While body maintenance in the form of cleaning and caring for oneself could be an expected function for an area with exercise and excretion facilities, it is worth noting that the ISS provides, at most, minimal accommodation for this activity. A description of the WHC stated, “To provide privacy…an enclosure was added to the front of the rack. This enclosure, referred to as the Cabin, is approximately the size of a typical bathroom stall and provides room for system consumables and hygiene item stowage. Space is available to also support limited hygiene functions such as hand and body washing” [ 48 ]. A diagram of the WHC in the same publication shows the Cabin without a scale but suggests that it measures roughly 2 m (h) x .75 (w) x .75 (d), a volume of approximately 1.125 m 3 . NASA’s current design standards state that the body volume of a 95th percentile male astronaut is 0.99 m 3 [ 20 ], meaning that a person of that size would take up 88% of the space of the Cabin, leaving little room for performing cleaning functions—especially if the Cabin is used as apparently intended, to also hold “system consumables and hygiene item[s]” that would further diminish the usable volume. This situation explains why crews try to adapt other spaces, such as storage areas like the PMM, for these activities instead. According to the crew debriefing statement, only one of them used the WHC for body maintenance purposes; it is not clear whether the toiletry kit belonged to that individual. But the appearance of the toiletry kit in Square 05—outside of the WHC, in a public space where others frequently pass by—may have been a response to the limitations of the WHC Cabin. It suggests a need for designers to re-evaluate affordances for body maintenance practices and storage for related items.
Although Square 03 and 05 were different sizes and shapes, comparing the density of items by function shows evidence of their usage ( Table 3 ). The typical context in Square 03 had twice as many restraints and containers, but less than one-quarter as many body maintenance items as Square 05. 03 also had many tools, lights, audiovisual equipment, and writing implements, while there were none of any of these types in 05. 05 had life support and hygiene items which were missing from 03. It appears that flexibility and multifunctionality were key elements for 03, while in 05 there was emphasis on one primary function (albeit an improvised one, designated by the crew rather than architects or ground control), cleaning and caring for one’s body, with a secondary function of housing static equipment for crew hygiene and life support.
https://doi.org/10.1371/journal.pone.0304229.t003
As this is the first time such an analysis has been performed, it is not yet possible to say how typical or unusual these squares are regarding the types of activities taking place; but they provide a baseline for eventual comparison with the other four squares and future work on ISS or other space habitats.
Some general characteristics are revealed by archaeological analysis of a space station’s material culture. First, even in a small, enclosed site, occupied by only a few people over a relatively short sample period, we can observe divergent patterns for different locations and activity phases. Second, while distinct functions are apparent for these two squares, they are not the functions that we expected prior to this research. As a result, our work fulfills the promise of the archaeological approach to understanding life in a space station by revealing new, previously unrecognized phenomena relating to life and work on the ISS. There is now systematically recorded archaeological data for a space habitat.
Squares 03 and 05 served quite different purposes. The reasons for this fact are their respective affordances and their locations relative to activity areas designated for science and exercise. Their national associations, especially the manifestation of the control wielded by NASA over its modules, also played a role in the use of certain materials, the placement of facilities, and the organization of work. How each area was used was also the result of an interplay between the original plans developed by mission planners and habitat designers (or the lack of such plans), the utility of the equipment and architecture in each location, and the contingent needs of the crew as they lived in the station. This interplay became visible in the station’s material culture, as certain areas were associated with particular behaviors, over time and through tradition—over the long duration across many crews (Node 2, location of Square 03, docked with the ISS in 2007, and Node 3, location of Square 05, docked in 2010), and during the specific period of this survey, from January to March 2022. During the crew debriefing, one astronaut said, “We were a pretty organized crew who was also pretty much on the same page about how to do things…. As time went on…we organized the lab and kind of got on the same page about where we put things and how we’re going to do things.” This statement shows how functional associations can become linked to different areas of the ISS through usage and mutual agreement. At the same time, the station is not frozen in time. Different people have divergent ideas about how and where to do things. It seems from the appearance of just one Russian item—a packet of generic wipes ( salfetky sukhiye ) stored in the toiletry kit throughout the sample period—that the people who used these spaces and carried out their functions did not typically include the ISS’s Russian crew. Enabling greater flexibility to define how spaces can be used could have a significant impact on improving crew autonomy over their lives, such as how and where to work. It could also lead to opening of all spaces within a habitat to the entire crew, which seems likely to improve general well-being.
An apparent disjunction between planned and actual usage appeared in Square 03. It is intended for maintenance as well as other kinds of work. But much of the time, there was nobody working here—a fact that is not captured by historic photos of the area, precisely because nothing is happening. The space has instead become the equivalent of a pegboard mounted on a wall in a home garage or shed, convenient for storage for all kinds of items—not necessarily items being used there—because it has an enormous number of attachment points. Storage has become its primary function. Designers of future workstations in space should consider that they might need to optimize for functions other than work, because most of the time, there might not be any work happening there. They could optimize for quick storage, considering whether to impose a system of organization, or allow users to organize as they want.
We expected from previous (though unsystematic) observation of historic photos and other research, that resealable plastic bags (combined with Velcro patches on the bags and walls) would be the primary means for creating gravity surrogates to control items in microgravity. They only comprise 7% of all items in Square 03 (256 instances). There are more than twice as many clips (572—more than 9 per context) in the sample. There were 193 instances of adhesive tape rolls, and more than 100 cable ties, but these were latent (not holding anything), representing potentiality of restraint rather than actualization. The squares showed different approaches to managing “gravity.” While Square 03 had a pre-existing structured array of Velcro patches, Square 05 showed a more expedient strategy with Velcro added in response to particular activities. Different needs require different affordances; creating “gravity” is a more nuanced endeavor than it initially appears. More work remains to be done to optimize gravity surrogates for future space habitats, because this is evidently one of the most critical adaptations that crews have to make in microgravity (44% of all items in Square 03, 39% in 05).
Square 05 is an empty space, seemingly just one side of a passageway for people going to use the lifting machine or the latrine, to look out of the Cupola, or get something out of deep storage in one of the ISS’s closets. In our survey, this square was a storage place for toiletries, resealable bags, and a computer that never (or almost never) gets used. It was associated with computing and hygiene simply by virtue of its location, rather than due to any particular facilities it possessed. It has no affordances for storage. There are no cabinets or drawers, as would be appropriate for organizing and holding crew personal items. A crew member decided that this was an appropriate place to leave their toiletry kit for almost two months. Whether this choice was appreciated or resented by fellow crew members cannot be discerned based on our evidence, but it seems to have been tolerated, given its long duration. The location of the other four USOS crew members’ toiletry kits during the sample period is unknown. A question raised by our observations is: how might a function be more clearly defined by designers for this area, perhaps by providing lockers for individual crew members to store their toiletries and towels? This would have a benefit not only for reducing clutter, but also for reducing exposure of toiletry kits and the items stored in them to flying sweat from the exercise equipment or other waste particles from the latrine. A larger compartment providing privacy for body maintenance and a greater range of motion would also be desirable.
As the first systematic collection of archaeological data from a space site outside Earth, this analysis of two areas on the ISS as part of the SQuARE payload has shown that novel insights into material culture use can be obtained, such as the use of wall areas as storage or staging posts between activities, the accretion of objects associated with different functions, and the complexity of using material replacements for gravity. These results enable better space station design and raise new questions that will be addressed through analysis of the remaining four squares.
Supporting information
S1 movie. nasa astronaut kayla barron installs the first square for the sampling quadrangle assemblages research experiment in the japanese experiment module (also known as kibo) on the international space station, january 14, 2022..
She places Kapton tape to mark the square’s upper right corner. Credit: NASA.
https://doi.org/10.1371/journal.pone.0304229.s001
S1 Dataset.
https://doi.org/10.1371/journal.pone.0304229.s002
S2 Dataset.
https://doi.org/10.1371/journal.pone.0304229.s003
S3 Dataset. The image annotations are represented according to sample square in json formatted text files.
The data is available in the ‘SQuARE-notebooks’ repository on Github.com in the ‘data’ subfolder at https://github.com/issarchaeologicalproject/SQuARE-notebooks/tree/main ; archived version of the repository is at Zenodo, DOI: 10.5281/zenodo.10654812 .
https://doi.org/10.1371/journal.pone.0304229.s004
S1 File. The ‘Rocket-Anno’ image annotation software is available on Github at https://github.com/issarchaeologicalproject/MRE-RocketAnno .
The archived version of the repository is at Zenodo, DOI: 10.5281/zenodo.10648399 .
https://doi.org/10.1371/journal.pone.0304229.s005
S2 File. The computational notebooks that process the data json files to reshape the data suitable for basic statistics as well as the computation of the Brainerd-Robinson coefficients of similarity are in the.ipynb notebook format.
The code is available in the ‘SQuARE-notebooks’ repository on Github.com in the ‘notebooks’ subfolder at https://github.com/issarchaeologicalproject/SQuARE-notebooks/tree/main ; archived version of the repository is at Zenodo, DOI: 10.5281/zenodo.10654812 . The software can be run online in the Google Colab environment ( https://colab.research.google.com ) or any system running Jupyter Notebooks ( https://jupyter.org/ ).
https://doi.org/10.1371/journal.pone.0304229.s006
https://doi.org/10.1371/journal.pone.0304229.s007
Acknowledgments
We thank Chapman University’s Office of Research and Sponsored Programs, and especially Dr. Thomas Piechota and Dr. Janeen Hill, for funding the Implementation Partner costs associated with the SQuARE payload. Chapman’s Leatherby Libraries’ Supporting Open Access Research and Scholarship (SOARS) program funded the article processing fee for this publication. Ken Savin and Ken Shields at the ISS National Laboratory gave major support by agreeing to sponsor SQuARE and providing access to ISS NL’s allocation of crew time. David Zuniga and Kryn Ambs at Axiom Space were key collaborators in managing payload logistics. NASA staff and contractors were critical to the experiment’s success, especially Kristen Fortson, Jay Weber, Crissy Canerday, Sierra Wolbert, and Jade Conway. We also gratefully acknowledge the help and resources provided by Dr. Erik Linstead, director of the Machine Learning and Affiliated Technology Lab at Chapman University. Aidan St. P. Walsh corrected the color and lens barrel distortion in all of the SQuARE imagery. Rao Hamza Ali produced charts using accessible color combinations for Figs 3 and 5 . And finally, of course, we are extremely appreciative of the efforts of the five USOS members of the Expedition 66 crew on the ISS—Kayla Barron, Raja Chari, Thomas Marshburn, Matthias Maurer, and Mark Vande Hei—who were the first archaeologists in space.
- 1. Buchli V. Extraterrestrial methods: Towards an ethnography of the ISS. In: Carroll T, Walford A, Walton S, editors. Lineages and advancements in material culture studies: Perspectives from UCL anthropology. London: Routledge; 2021, pp. 17–32.
- 2. Gorman A, Walsh J. Archaeology in a vacuum: obstacles to and solutions for developing a real space archaeology. In: Barnard H, editor. Archaeology outside the box: investigations at the edge of the discipline. Los Angeles, Cotsen Institute of Archaeology Press; 2023. pp. 131–123.
- 3. Walsh J. Adapting to space: The International Space Station Archaeological Project. In: Salazar Sutil JF, Gorman A, editors. Routledge handbook of social studies of outer space. London, Routledge; 2023. pp. 400–412. https://doi.org/10.4324/9781003280507-37
- View Article
- Google Scholar
- 6. Rathje W, Murphy C. Rubbish! The archaeology of garbage Tucson: University of Arizona Press; 2001.
- 7. De León J. The land of open graves: living and dying on the migrant trail. Berkeley, University of California Press; 2015.
- 8. Garrison Darrin A, O’Leary B, editors. Handbook of space engineering, archaeology, and heritage. Boca Raton, CRC Press; 2009.
- 9. Capelotti PJ. The human archaeology of space: Lunar, planetary, and interstellar relics of exploration. Jefferson, NC, McFarland Press; 2010.
- 11. Gorman A. Space and time through material culture: An account of space archaeology. In: Salazar Sutil JF, Gorman A, editors. Routledge handbook of social studies of outer space. London, Routledge; 2023. pp. 44–56. https://doi.org/10.4324/9781003280507-5
- 17. NASA. NASA Johnson. 2008 Aug [cited May 12 2024]. In: Flickr [Internet]. San Francisco. Available from https://www.flickr.com/photos/nasa2explore/
- 19. NASA. ISS Daily Status Reports. 2012 Mar 1 [Cited May 12 2024]. Available from: https://blogs.nasa.gov/stationreport/
- 20. NASA. Man-systems integration. STD-3000 Vol. 1. Houston, NASA Johnson; 1995, pp. 9–15, 78
- 21. NASA. Maintenance Work Area | Glenn Research Center. 2020 Mar 6 [cited May 12 2024]. Available from: https://www1.grc.nasa.gov/space/iss-research/mwa/
- 22. Cristoforetti S. Diario di un’apprendista astronauta. Milan, Le Polene; 2018. pp. 379.
- 23. Kelly S. Endurance: A year in space, a lifetime of discovery. New York, Knopf; 2017. pp. 175, 285–86.
- 24. Barron K. Instagram post, 2022 Feb 12 [cited 2024 May 12]. Available from: https://www.instagram.com/tv/CZ4pW9HJ2Wg/?igsh=ZDE1MWVjZGVmZQ==
- 25. NASA. NASA space flight human-system standard. STD-3001 Volume 1: Human integration design handbook. Rev. 1 Houston, NASA Johnson; 2014. pp. 814, 829–833.
- 27. Keeter B. ISS daily summary report– 2/21/2022. 2022 Feb 21 [cited May 12 2024]. In: NASA ISS On-Orbit Status Report blog [Internet]. Houston. Available from: https://blogs.nasa.gov/stationreport/2022/02/page/6/
- 28. DLR. Fingerprint research to combat harmful bacteria. 2022 Jan 18 [cited May 12 2024]. Available from: https://www.dlr.de/en/latest/news/2022/01/20220118_fingerprint-research-to-combat-harmful-bacteria
- 31. Peeples MA. R script for calculating the Brainerd-Robinson coefficient of similarity and assessing sampling error. 2011 [cited May 12 2024]. Available from: http://www.mattpeeples.net/br.html .
- 33. Garcia M. Cargo Dragon Splashes Down Ending SpaceX CRS-24 Mission. 2022 Jan 24 [cited May 12 2024]. NASA Space Station blog [Internet]. Available from: https://blogs.nasa.gov/spacestation/2022/01/24/cargo-dragon-splashes-down-ending-spacex-crs-24-mission/
- 34. ESA. Concrete Hardening | Cosmic Kiss 360°. 2022 Mar 5 [cited May 12 2024]. Available from: https://www.esa.int/ESA_Multimedia/Videos/2022/05/Concrete_Hardening_Cosmic_Kiss_360
- 35. Keeter B. ISS daily summary report– 2/01/2022. 2022 Feb 1 [cited May 12 2024]. In: NASA ISS On-Orbit Status Report blog [Internet]. Houston. Available from: https://blogs.nasa.gov/stationreport/2022/02/page/19/
- 36. Keeter B. ISS daily summary report– 2/17/2022. 2022 Feb 17 [cited May 12 2024]. In: NASA ISS On-Orbit Status Report blog [Internet]. Houston. Available from: https://blogs.nasa.gov/stationreport/2022/02/page/8/
- 37. T. Pultarova, How Do You Clean a Space Station? Astronaut Thomas Pesquet Shares Orbital Spring Cleaning Tips, Space.com, May 6, 2021. Online at https://www.space.com/space-station-cleaning-tips-astronaut-thomas-pesquet
- 38. Keeter B. ISS daily summary report– 2/22/2022. 2022 Feb 22 [cited May 12 2024]. In: NASA ISS On-Orbit Status Report blog [Internet]. Houston. Available from: https://blogs.nasa.gov/stationreport/2022/02/page/5/
- 39. Keeter B. ISS daily summary report– 2/02/2022. 2022 Feb 2 [cited May 12 2024]. NASA ISS On-Orbit Status Report blog [Internet]. Houston. Online at https://blogs.nasa.gov/stationreport/2022/02/page/18/
- 40. Keeter B. ISS daily summary report– 3/03/2022. 2022 Mar 3 [cited May 12 2024]. In: NASA ISS On-Orbit Status Report blog [Internet]. Houston. Available from: https://blogs.nasa.gov/stationreport/2022/03/page/21/
- 41. Keeter B. ISS daily summary report– 2/08/2022. 2022 Feb 8 [cited May 12 2024]. NASA ISS On-Orbit Status Report blog [Internet]. Houston. Available from: https://blogs.nasa.gov/stationreport/2022/02/page/15/
- 42. Aristotle of Stageira. Metaphysics, Volume I: Books 1–9, Tredennick H, translator. Loeb Classical Library 271. Cambridge, MA, Harvard University Press; 1933. pp. 429–473.
- 44. Hodder I. Entangled: An archaeology of the relationships between humans and things. Hoboken. NJ, Wiley-Blackwell; 2012.
- 45. Malafouris L., How Things Shape the Mind: A Theory of Material Engagement (MIT Press, 2016).
- 46. Keeter B. ISS daily summary report– 3/11/2022. 2022 Mar 11 [cited May 12 2024]. NASA ISS On-Orbit Status Report blog [Internet]. Houston. Available from: https://blogs.nasa.gov/stationreport/2022/03/page/15/
- Privacy Policy

Home » Research – Types, Methods and Examples
Research – Types, Methods and Examples
Table of Contents

Definition:
Research refers to the process of investigating a particular topic or question in order to discover new information , develop new insights, or confirm or refute existing knowledge. It involves a systematic and rigorous approach to collecting, analyzing, and interpreting data, and requires careful planning and attention to detail.
History of Research
The history of research can be traced back to ancient times when early humans observed and experimented with the natural world around them. Over time, research evolved and became more systematic as people sought to better understand the world and solve problems.
In ancient civilizations such as those in Greece, Egypt, and China, scholars pursued knowledge through observation, experimentation, and the development of theories. They explored various fields, including medicine, astronomy, and mathematics.
During the Middle Ages, research was often conducted by religious scholars who sought to reconcile scientific discoveries with their faith. The Renaissance brought about a renewed interest in science and the scientific method, and the Enlightenment period marked a major shift towards empirical observation and experimentation as the primary means of acquiring knowledge.
The 19th and 20th centuries saw significant advancements in research, with the development of new scientific disciplines and fields such as psychology, sociology, and computer science. Advances in technology and communication also greatly facilitated research efforts.
Today, research is conducted in a wide range of fields and is a critical component of many industries, including healthcare, technology, and academia. The process of research continues to evolve as new methods and technologies emerge, but the fundamental principles of observation, experimentation, and hypothesis testing remain at its core.
Types of Research
Types of Research are as follows:
- Applied Research : This type of research aims to solve practical problems or answer specific questions, often in a real-world context.
- Basic Research : This type of research aims to increase our understanding of a phenomenon or process, often without immediate practical applications.
- Experimental Research : This type of research involves manipulating one or more variables to determine their effects on another variable, while controlling all other variables.
- Descriptive Research : This type of research aims to describe and measure phenomena or characteristics, without attempting to manipulate or control any variables.
- Correlational Research: This type of research examines the relationships between two or more variables, without manipulating any variables.
- Qualitative Research : This type of research focuses on exploring and understanding the meaning and experience of individuals or groups, often through methods such as interviews, focus groups, and observation.
- Quantitative Research : This type of research uses numerical data and statistical analysis to draw conclusions about phenomena or populations.
- Action Research: This type of research is often used in education, healthcare, and other fields, and involves collaborating with practitioners or participants to identify and solve problems in real-world settings.
- Mixed Methods Research : This type of research combines both quantitative and qualitative research methods to gain a more comprehensive understanding of a phenomenon or problem.
- Case Study Research: This type of research involves in-depth examination of a specific individual, group, or situation, often using multiple data sources.
- Longitudinal Research: This type of research follows a group of individuals over an extended period of time, often to study changes in behavior, attitudes, or health outcomes.
- Cross-Sectional Research : This type of research examines a population at a single point in time, often to study differences or similarities among individuals or groups.
- Survey Research: This type of research uses questionnaires or interviews to gather information from a sample of individuals about their attitudes, beliefs, behaviors, or experiences.
- Ethnographic Research : This type of research involves immersion in a cultural group or community to understand their way of life, beliefs, values, and practices.
- Historical Research : This type of research investigates events or phenomena from the past using primary sources, such as archival records, newspapers, and diaries.
- Content Analysis Research : This type of research involves analyzing written, spoken, or visual material to identify patterns, themes, or messages.
- Participatory Research : This type of research involves collaboration between researchers and participants throughout the research process, often to promote empowerment, social justice, or community development.
- Comparative Research: This type of research compares two or more groups or phenomena to identify similarities and differences, often across different countries or cultures.
- Exploratory Research : This type of research is used to gain a preliminary understanding of a topic or phenomenon, often in the absence of prior research or theories.
- Explanatory Research: This type of research aims to identify the causes or reasons behind a particular phenomenon, often through the testing of theories or hypotheses.
- Evaluative Research: This type of research assesses the effectiveness or impact of an intervention, program, or policy, often through the use of outcome measures.
- Simulation Research : This type of research involves creating a model or simulation of a phenomenon or process, often to predict outcomes or test theories.
Data Collection Methods
- Surveys : Surveys are used to collect data from a sample of individuals using questionnaires or interviews. Surveys can be conducted face-to-face, by phone, mail, email, or online.
- Experiments : Experiments involve manipulating one or more variables to measure their effects on another variable, while controlling for other factors. Experiments can be conducted in a laboratory or in a natural setting.
- Case studies : Case studies involve in-depth analysis of a single case, such as an individual, group, organization, or event. Case studies can use a variety of data collection methods, including interviews, observation, and document analysis.
- Observational research : Observational research involves observing and recording the behavior of individuals or groups in a natural setting. Observational research can be conducted covertly or overtly.
- Content analysis : Content analysis involves analyzing written, spoken, or visual material to identify patterns, themes, or messages. Content analysis can be used to study media, social media, or other forms of communication.
- Ethnography : Ethnography involves immersion in a cultural group or community to understand their way of life, beliefs, values, and practices. Ethnographic research can use a range of data collection methods, including observation, interviews, and document analysis.
- Secondary data analysis : Secondary data analysis involves using existing data from sources such as government agencies, research institutions, or commercial organizations. Secondary data can be used to answer research questions, without collecting new data.
- Focus groups: Focus groups involve gathering a small group of people together to discuss a topic or issue. The discussions are usually guided by a moderator who asks questions and encourages discussion.
- Interviews : Interviews involve one-on-one conversations between a researcher and a participant. Interviews can be structured, semi-structured, or unstructured, and can be conducted in person, by phone, or online.
- Document analysis : Document analysis involves collecting and analyzing written documents, such as reports, memos, and emails. Document analysis can be used to study organizational communication, policy documents, and other forms of written material.
Data Analysis Methods
Data Analysis Methods in Research are as follows:
- Descriptive statistics : Descriptive statistics involve summarizing and describing the characteristics of a dataset, such as mean, median, mode, standard deviation, and frequency distributions.
- Inferential statistics: Inferential statistics involve making inferences or predictions about a population based on a sample of data, using methods such as hypothesis testing, confidence intervals, and regression analysis.
- Qualitative analysis: Qualitative analysis involves analyzing non-numerical data, such as text, images, or audio, to identify patterns, themes, or meanings. Qualitative analysis can be used to study subjective experiences, social norms, and cultural practices.
- Content analysis: Content analysis involves analyzing written, spoken, or visual material to identify patterns, themes, or messages. Content analysis can be used to study media, social media, or other forms of communication.
- Grounded theory: Grounded theory involves developing a theory or model based on empirical data, using methods such as constant comparison, memo writing, and theoretical sampling.
- Discourse analysis : Discourse analysis involves analyzing language use, including the structure, function, and meaning of words and phrases, to understand how language reflects and shapes social relationships and power dynamics.
- Network analysis: Network analysis involves analyzing the structure and dynamics of social networks, including the relationships between individuals and groups, to understand social processes and outcomes.
Research Methodology
Research methodology refers to the overall approach and strategy used to conduct a research study. It involves the systematic planning, design, and execution of research to answer specific research questions or test hypotheses. The main components of research methodology include:
- Research design : Research design refers to the overall plan and structure of the study, including the type of study (e.g., observational, experimental), the sampling strategy, and the data collection and analysis methods.
- Sampling strategy: Sampling strategy refers to the method used to select a representative sample of participants or units from the population of interest. The choice of sampling strategy will depend on the research question and the nature of the population being studied.
- Data collection methods : Data collection methods refer to the techniques used to collect data from study participants or sources, such as surveys, interviews, observations, or secondary data sources.
- Data analysis methods: Data analysis methods refer to the techniques used to analyze and interpret the data collected in the study, such as descriptive statistics, inferential statistics, qualitative analysis, or content analysis.
- Ethical considerations: Ethical considerations refer to the principles and guidelines that govern the treatment of human participants or the use of sensitive data in the research study.
- Validity and reliability : Validity and reliability refer to the extent to which the study measures what it is intended to measure and the degree to which the study produces consistent and accurate results.
Applications of Research
Research has a wide range of applications across various fields and industries. Some of the key applications of research include:
- Advancing scientific knowledge : Research plays a critical role in advancing our understanding of the world around us. Through research, scientists are able to discover new knowledge, uncover patterns and relationships, and develop new theories and models.
- Improving healthcare: Research is instrumental in advancing medical knowledge and developing new treatments and therapies. Clinical trials and studies help to identify the effectiveness and safety of new drugs and medical devices, while basic research helps to uncover the underlying causes of diseases and conditions.
- Enhancing education: Research helps to improve the quality of education by identifying effective teaching methods, developing new educational tools and technologies, and assessing the impact of various educational interventions.
- Driving innovation: Research is a key driver of innovation, helping to develop new products, services, and technologies. By conducting research, businesses and organizations can identify new market opportunities, gain a competitive advantage, and improve their operations.
- Informing public policy : Research plays an important role in informing public policy decisions. Policy makers rely on research to develop evidence-based policies that address societal challenges, such as healthcare, education, and environmental issues.
- Understanding human behavior : Research helps us to better understand human behavior, including social, cognitive, and emotional processes. This understanding can be applied in a variety of settings, such as marketing, organizational management, and public policy.
Importance of Research
Research plays a crucial role in advancing human knowledge and understanding in various fields of study. It is the foundation upon which new discoveries, innovations, and technologies are built. Here are some of the key reasons why research is essential:
- Advancing knowledge: Research helps to expand our understanding of the world around us, including the natural world, social structures, and human behavior.
- Problem-solving: Research can help to identify problems, develop solutions, and assess the effectiveness of interventions in various fields, including medicine, engineering, and social sciences.
- Innovation : Research is the driving force behind the development of new technologies, products, and processes. It helps to identify new possibilities and opportunities for improvement.
- Evidence-based decision making: Research provides the evidence needed to make informed decisions in various fields, including policy making, business, and healthcare.
- Education and training : Research provides the foundation for education and training in various fields, helping to prepare individuals for careers and advancing their knowledge.
- Economic growth: Research can drive economic growth by facilitating the development of new technologies and innovations, creating new markets and job opportunities.
When to use Research
Research is typically used when seeking to answer questions or solve problems that require a systematic approach to gathering and analyzing information. Here are some examples of when research may be appropriate:
- To explore a new area of knowledge : Research can be used to investigate a new area of knowledge and gain a better understanding of a topic.
- To identify problems and find solutions: Research can be used to identify problems and develop solutions to address them.
- To evaluate the effectiveness of programs or interventions : Research can be used to evaluate the effectiveness of programs or interventions in various fields, such as healthcare, education, and social services.
- To inform policy decisions: Research can be used to provide evidence to inform policy decisions in areas such as economics, politics, and environmental issues.
- To develop new products or technologies : Research can be used to develop new products or technologies and improve existing ones.
- To understand human behavior : Research can be used to better understand human behavior and social structures, such as in psychology, sociology, and anthropology.
Characteristics of Research
The following are some of the characteristics of research:
- Purpose : Research is conducted to address a specific problem or question and to generate new knowledge or insights.
- Systematic : Research is conducted in a systematic and organized manner, following a set of procedures and guidelines.
- Empirical : Research is based on evidence and data, rather than personal opinion or intuition.
- Objective: Research is conducted with an objective and impartial perspective, avoiding biases and personal beliefs.
- Rigorous : Research involves a rigorous and critical examination of the evidence and data, using reliable and valid methods of data collection and analysis.
- Logical : Research is based on logical and rational thinking, following a well-defined and logical structure.
- Generalizable : Research findings are often generalized to broader populations or contexts, based on a representative sample of the population.
- Replicable : Research is conducted in a way that allows others to replicate the study and obtain similar results.
- Ethical : Research is conducted in an ethical manner, following established ethical guidelines and principles, to ensure the protection of participants’ rights and well-being.
- Cumulative : Research builds on previous studies and contributes to the overall body of knowledge in a particular field.
Advantages of Research
Research has several advantages, including:
- Generates new knowledge: Research is conducted to generate new knowledge and understanding of a particular topic or phenomenon, which can be used to inform policy, practice, and decision-making.
- Provides evidence-based solutions : Research provides evidence-based solutions to problems and issues, which can be used to develop effective interventions and strategies.
- Improves quality : Research can improve the quality of products, services, and programs by identifying areas for improvement and developing solutions to address them.
- Enhances credibility : Research enhances the credibility of an organization or individual by providing evidence to support claims and assertions.
- Enables innovation: Research can lead to innovation by identifying new ideas, approaches, and technologies.
- Informs decision-making : Research provides information that can inform decision-making, helping individuals and organizations make more informed and effective choices.
- Facilitates progress: Research can facilitate progress by identifying challenges and opportunities and developing solutions to address them.
- Enhances understanding: Research can enhance understanding of complex issues and phenomena, helping individuals and organizations navigate challenges and opportunities more effectively.
- Promotes accountability : Research promotes accountability by providing a basis for evaluating the effectiveness of policies, programs, and interventions.
- Fosters collaboration: Research can foster collaboration by bringing together individuals and organizations with diverse perspectives and expertise to address complex issues and problems.
Limitations of Research
Some Limitations of Research are as follows:
- Cost : Research can be expensive, particularly when large-scale studies are required. This can limit the number of studies that can be conducted and the amount of data that can be collected.
- Time : Research can be time-consuming, particularly when longitudinal studies are required. This can limit the speed at which research findings can be generated and disseminated.
- Sample size: The size of the sample used in research can limit the generalizability of the findings to larger populations.
- Bias : Research can be affected by bias, both in the design and implementation of the study, as well as in the analysis and interpretation of the data.
- Ethics : Research can present ethical challenges, particularly when human or animal subjects are involved. This can limit the types of research that can be conducted and the methods that can be used.
- Data quality: The quality of the data collected in research can be affected by a range of factors, including the reliability and validity of the measures used, as well as the accuracy of the data entry and analysis.
- Subjectivity : Research can be subjective, particularly when qualitative methods are used. This can limit the objectivity and reliability of the findings.
- Accessibility : Research findings may not be accessible to all stakeholders, particularly those who are not part of the academic or research community.
- Interpretation : Research findings can be open to interpretation, particularly when the data is complex or contradictory. This can limit the ability of researchers to draw firm conclusions.
- Unforeseen events : Unexpected events, such as changes in the environment or the emergence of new technologies, can limit the relevance and applicability of research findings.
About the author
Muhammad Hassan
Researcher, Academic Writer, Web developer
You may also like

Chemist – Definition, Types and Work Area

What is Archaeology – Definition and Overview

Concept – Definition, Types and Examples

Physicist – Definition, Types and Work Area

What is Science – Definition, Methods, Types

Ethos Pathos Logos – Definition, Meanings and...
Information
- Author Services
Initiatives
You are accessing a machine-readable page. In order to be human-readable, please install an RSS reader.
All articles published by MDPI are made immediately available worldwide under an open access license. No special permission is required to reuse all or part of the article published by MDPI, including figures and tables. For articles published under an open access Creative Common CC BY license, any part of the article may be reused without permission provided that the original article is clearly cited. For more information, please refer to https://www.mdpi.com/openaccess .
Feature papers represent the most advanced research with significant potential for high impact in the field. A Feature Paper should be a substantial original Article that involves several techniques or approaches, provides an outlook for future research directions and describes possible research applications.
Feature papers are submitted upon individual invitation or recommendation by the scientific editors and must receive positive feedback from the reviewers.
Editor’s Choice articles are based on recommendations by the scientific editors of MDPI journals from around the world. Editors select a small number of articles recently published in the journal that they believe will be particularly interesting to readers, or important in the respective research area. The aim is to provide a snapshot of some of the most exciting work published in the various research areas of the journal.
Original Submission Date Received: .
- Active Journals
- Find a Journal
- Proceedings Series
- For Authors
- For Reviewers
- For Editors
- For Librarians
- For Publishers
- For Societies
- For Conference Organizers
- Open Access Policy
- Institutional Open Access Program
- Special Issues Guidelines
- Editorial Process
- Research and Publication Ethics
- Article Processing Charges
- Testimonials
- Preprints.org
- SciProfiles
- Encyclopedia
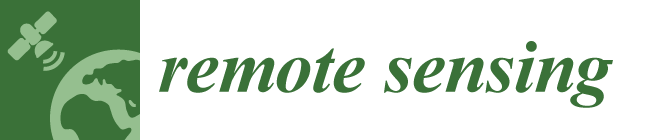
Article Menu
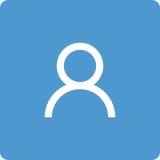
- Subscribe SciFeed
- Recommended Articles
- Google Scholar
- on Google Scholar
- Table of Contents
Find support for a specific problem in the support section of our website.
Please let us know what you think of our products and services.
Visit our dedicated information section to learn more about MDPI.
JSmol Viewer
A review of computer vision-based crack detection methods in civil infrastructure: progress and challenges.
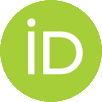
1. Introduction
2. crack detection combining traditional image processing methods and deep learning, 2.1. crack detection based on image edge detection and deep learning, 2.2. crack detection based on threshold segmentation and deep learning, 2.3. crack detection based on morphological operations and deep learning, 3. crack detection based on multimodal data fusion, 3.1. multi-sensor fusion, 3.2. multi-source data fusion, 4. crack detection based on image semantic understanding, 4.1. crack detection based on classification networks, 4.2. crack detection based on object detection networks, 4.3. crack detection based on segmentation networks.
Model | Improvement/Innovation | Backbone/Feature Extraction Architecture | Efficiency | Results |
---|---|---|---|---|
FCS-Net [ ] | Integrating ResNet-50, ASPP, and BN | ResNet-50 | - | MIoU = 74.08% |
FCN-SFW [ ] | Combining fully convolutional network (FCN) and structural forests with wavelet transform (SFW) for detecting tiny cracks | FCN | Computing time = 1.5826 s | Precision = 64.1% Recall = 87.22% F1 score = 68.28% |
AFFNet [ ] | Using ResNet101 as the backbone network, and incorporating two attention mechanism modules, namely VH-CAM and ECAUM | ResNet101 | Execution time = 52 ms | MIoU = 84.49% FWIoU = 97.07% PA = 98.36% MPA = 92.01% |
DeepLabv3+ [ ] | Replacing ordinary convolution with separable convolution; improved SE_ASSP module | Xception-65 | - | AP = 97.63% MAP = 95.58% MIoU = 81.87% |
U-Net [ ] | The parameters were optimized (the depths of the network, the choice of activation functions, the selection of loss functions, and the data augmentation) | Encoder and decoder | Analysis speed (1024 × 1024 pixels) = 0.022 s | Precision = 84.6% Recall = 72.5% F1 score = 78.1% IoU = 64% |
KTCAM-Net [ ] | Combined CAM and RCM; integrating classification network and segmentation network | DeepLabv3 | FPS = 28 | Accuracy = 97.26% Precision = 68.9% Recall = 83.7% F1 score = 75.4% MIoU = 74.3% |
ADDU-Net [ ] | Featuring asymmetric dual decoders and dual attention mechanisms | Encoder and decoder | FPS = 35 | Precision = 68.9% Recall = 83.7% F1 score = 75.4% MIoU = 74.3% |
CGTr-Net [ ] | Optimized CG-Trans, TCFF, and hybrid loss functions | CG-Trans | - | Precision = 88.8% Recall = 88.3% F1 score = 88.6% MIoU = 89.4% |
PCSN [ ] | Using Adadelta as the optimizer and categorical cross-entropy as the loss function for the network | SegNet | Inference time = 0.12 s | mAP = 83% Accuracy = 90% Recall = 50% |
DEHF-Net [ ] | Introducing dual-branch encoder unit, feature fusion scheme, edge refinement module, and multi-scale feature fusion module | Dual-branch encoder unit | - | Precision = 86.3% Recall = 92.4% Dice score = 78.7% mIoU = 81.6% |
Student model + teacher model [ ] | Proposed a semi-supervised semantic segmentation network | EfficientUNet | - | Precision = 84.98% Recall = 84.38% F1 score = 83.15% |
5. Datasets
6. evaluation index, 7. discussion, 8. conclusions, author contributions, data availability statement, acknowledgments, conflicts of interest.
Aspect | Combining Traditional Image Processing Methods and Deep Learning | Multimodal Data Fusion |
---|---|---|
Processing speed | Moderate—traditional methods are usually fast, but deep learning models may be slower, and the overall speed depends on the complexity of the deep learning model | Slower—data fusion and processing speed can be slow, especially with large-scale multimodal data, involving significant computational and data transfer overhead |
Accuracy | High—combines the interpretability of traditional methods with the complex pattern handling of deep learning, generally resulting in high detection accuracy | Typically higher—combining different data sources (e.g., images, text, audio) provides comprehensive information, improving overall detection accuracy |
Robustness | Strong—traditional methods provide background knowledge, enhancing robustness, but deep learning’s risk of overfitting may reduce robustness | Very strong—fusion of multiple data sources enhances the model’s adaptability to different environments and conditions, better handling noise and anomalies |
Complexity | High—integrating traditional methods and deep learning involves complex design and balancing, with challenges in tuning and interpreting deep learning models | High—involves complex data preprocessing, alignment, and fusion, handling inconsistencies and complexities from multiple data sources |
Adaptability | Strong—can adapt to different types of cracks and background variations, with deep learning models learning features from data, though it requires substantial labeled data | Very strong—combines diverse data sources, adapting well to various environments and conditions, and handling complex backgrounds and variations effectively |
Interpretability | Higher—traditional methods provide clear explanations, while deep learning models often lack interpretability; combining them can improve overall interpretability | Lower—fusion models generally have lower interpretability, making it difficult to intuitively explain how different data sources influence the final results |
Data requirements | High—deep learning models require a lot of labeled data, while traditional methods are more lenient, though deep learning still demands substantial data | Very high—requires large amounts of data from various modalities, and these data need to be processed and aligned effectively for successful fusion |
Flexibility | Moderate—combining traditional methods and deep learning handles various types of cracks, but may be limited in very complex scenarios | High—handles multiple data sources and different crack information, improving performance in diverse conditions through multimodal fusion |
Real-time capability | Poor—deep learning models are often slow to train and infer, making them less suitable for real-time detection, though combining with traditional methods can help | Poor—multimodal data fusion processing is generally slow, making it less suitable for real-time applications |
Maintenance cost | Moderate to high—deep learning models require regular updates and maintenance, while traditional methods have lower maintenance costs | High—involves ongoing maintenance and updates for multiple data sources, with complex data preprocessing and fusion processes |
Noise handling | Good—traditional methods effectively handle noise under certain conditions, and deep learning models can mitigate noise effects through training | Strong—multimodal fusion can complement information from different sources, improving robustness to noise and enhancing detection accuracy |
- Azimi, M.; Eslamlou, A.D.; Pekcan, G. Data-driven structural health monitoring and damage detection through deep learning: State-of-the-art review. Sensors 2020 , 20 , 2778. [ Google Scholar ] [ CrossRef ] [ PubMed ]
- Han, X.; Zhao, Z. Structural surface crack detection method based on computer vision technology. J. Build. Struct. 2018 , 39 , 418–427. [ Google Scholar ]
- Kruachottikul, P.; Cooharojananone, N.; Phanomchoeng, G.; Chavarnakul, T.; Kovitanggoon, K.; Trakulwaranont, D. Deep learning-based visual defect-inspection system for reinforced concrete bridge substructure: A case of thailand’s department of highways. J. Civ. Struct. Health Monit. 2021 , 11 , 949–965. [ Google Scholar ] [ CrossRef ]
- Gehri, N.; Mata-Falcón, J.; Kaufmann, W. Automated crack detection and measurement based on digital image correlation. Constr. Build. Mater. 2020 , 256 , 119383. [ Google Scholar ] [ CrossRef ]
- Mohan, A.; Poobal, S. Crack detection using image processing: A critical review and analysis. Alex. Eng. J. 2018 , 57 , 787–798. [ Google Scholar ] [ CrossRef ]
- Liu, Y.; Fan, J.; Nie, J.; Kong, S.; Qi, Y. Review and prospect of digital-image-based crack detection of structure surface. China Civ. Eng. J. 2021 , 54 , 79–98. [ Google Scholar ]
- Hsieh, Y.-A.; Tsai, Y.J. Machine learning for crack detection: Review and model performance comparison. J. Comput. Civ. Eng. 2020 , 34 , 04020038. [ Google Scholar ] [ CrossRef ]
- Xu, Y.; Bao, Y.; Chen, J.; Zuo, W.; Li, H. Surface fatigue crack identification in steel box girder of bridges by a deep fusion convolutional neural network based on consumer-grade camera images. Struct. Health Monit. 2019 , 18 , 653–674. [ Google Scholar ] [ CrossRef ]
- Wang, W.; Deng, L.; Shao, X. Fatigue design of steel bridges considering the effect of dynamic vehicle loading and overloaded trucks. J. Bridge Eng. 2016 , 21 , 04016048. [ Google Scholar ] [ CrossRef ]
- Zheng, K.; Zhou, S.; Zhang, Y.; Wei, Y.; Wang, J.; Wang, Y.; Qin, X. Simplified evaluation of shear stiffness degradation of diagonally cracked reinforced concrete beams. Materials 2023 , 16 , 4752. [ Google Scholar ] [ CrossRef ]
- Canny, J. A computational approach to edge detection. IEEE Trans. Pattern Anal. Mach. Intell. 1986 , PAMI-8 , 679–698. [ Google Scholar ] [ CrossRef ]
- Otsu, N. A threshold selection method from gray-level histograms. Automatica 1975 , 11 , 23–27. [ Google Scholar ] [ CrossRef ]
- Sohn, H.G.; Lim, Y.M.; Yun, K.H.; Kim, G.H. Monitoring crack changes in concrete structures. Comput.-Aided Civ. Infrastruct. Eng. 2005 , 20 , 52–61. [ Google Scholar ] [ CrossRef ]
- Wang, P.; Qiao, H.; Feng, Q.; Xue, C. Internal corrosion cracks evolution in reinforced magnesium oxychloride cement concrete. Adv. Cem. Res. 2023 , 36 , 15–30. [ Google Scholar ] [ CrossRef ]
- Loutridis, S.; Douka, E.; Trochidis, A. Crack identification in double-cracked beams using wavelet analysis. J. Sound Vib. 2004 , 277 , 1025–1039. [ Google Scholar ] [ CrossRef ]
- Fan, C.L. Detection of multidamage to reinforced concrete using support vector machine-based clustering from digital images. Struct. Control Health Monit. 2021 , 28 , e2841. [ Google Scholar ] [ CrossRef ]
- Kyal, C.; Reza, M.; Varu, B.; Shreya, S. Image-based concrete crack detection using random forest and convolution neural network. In Computational Intelligence in Pattern Recognition: Proceedings of the International Conference on Computational Intelligence in Pattern Recognition (CIPR 2021), Held at the Institute of Engineering and Management, Kolkata, West Bengal, India, on 24–25 April 2021 ; Springer: Singapore, 2022; pp. 471–481. [ Google Scholar ]
- Jia, H.; Lin, J.; Liu, J. Bridge seismic damage assessment model applying artificial neural networks and the random forest algorithm. Adv. Civ. Eng. 2020 , 2020 , 6548682. [ Google Scholar ] [ CrossRef ]
- Park, M.J.; Kim, J.; Jeong, S.; Jang, A.; Bae, J.; Ju, Y.K. Machine learning-based concrete crack depth prediction using thermal images taken under daylight conditions. Remote Sens. 2022 , 14 , 2151. [ Google Scholar ] [ CrossRef ]
- LeCun, Y.; Bengio, Y.; Hinton, G. Deep learning. Nature 2015 , 521 , 436–444. [ Google Scholar ] [ CrossRef ]
- Liu, Z.; Cao, Y.; Wang, Y.; Wang, W. Computer vision-based concrete crack detection using u-net fully convolutional networks. Autom. Constr. 2019 , 104 , 129–139. [ Google Scholar ] [ CrossRef ]
- Li, G.; Ma, B.; He, S.; Ren, X.; Liu, Q. Automatic tunnel crack detection based on u-net and a convolutional neural network with alternately updated clique. Sensors 2020 , 20 , 717. [ Google Scholar ] [ CrossRef ] [ PubMed ]
- Chaiyasarn, K.; Buatik, A.; Mohamad, H.; Zhou, M.; Kongsilp, S.; Poovarodom, N. Integrated pixel-level cnn-fcn crack detection via photogrammetric 3d texture mapping of concrete structures. Autom. Constr. 2022 , 140 , 104388. [ Google Scholar ] [ CrossRef ]
- Li, S.; Zhao, X.; Zhou, G. Automatic pixel-level multiple damage detection of concrete structure using fully convolutional network. Comput.-Aided Civ. Infrastruct. Eng. 2019 , 34 , 616–634. [ Google Scholar ] [ CrossRef ]
- Zheng, X.; Zhang, S.; Li, X.; Li, G.; Li, X. Lightweight bridge crack detection method based on segnet and bottleneck depth-separable convolution with residuals. IEEE Access 2021 , 9 , 161649–161668. [ Google Scholar ] [ CrossRef ]
- Azouz, Z.; Honarvar Shakibaei Asli, B.; Khan, M. Evolution of crack analysis in structures using image processing technique: A review. Electronics 2023 , 12 , 3862. [ Google Scholar ] [ CrossRef ]
- Hamishebahar, Y.; Guan, H.; So, S.; Jo, J. A comprehensive review of deep learning-based crack detection approaches. Appl. Sci. 2022 , 12 , 1374. [ Google Scholar ] [ CrossRef ]
- Meng, S.; Gao, Z.; Zhou, Y.; He, B.; Djerrad, A. Real-time automatic crack detection method based on drone. Comput.-Aided Civ. Infrastruct. Eng. 2023 , 38 , 849–872. [ Google Scholar ] [ CrossRef ]
- Humpe, A. Bridge inspection with an off-the-shelf 360 camera drone. Drones 2020 , 4 , 67. [ Google Scholar ] [ CrossRef ]
- Truong-Hong, L.; Lindenbergh, R. Automatically extracting surfaces of reinforced concrete bridges from terrestrial laser scanning point clouds. Autom. Constr. 2022 , 135 , 104127. [ Google Scholar ] [ CrossRef ]
- Cusson, D.; Rossi, C.; Ozkan, I.F. Early warning system for the detection of unexpected bridge displacements from radar satellite data. J. Civ. Struct. Health Monit. 2021 , 11 , 189–204. [ Google Scholar ] [ CrossRef ]
- Bonaldo, G.; Caprino, A.; Lorenzoni, F.; da Porto, F. Monitoring displacements and damage detection through satellite MT-INSAR techniques: A new methodology and application to a case study in rome (Italy). Remote Sens. 2023 , 15 , 1177. [ Google Scholar ] [ CrossRef ]
- Zheng, Z.; Zhong, Y.; Wang, J.; Ma, A.; Zhang, L. Building damage assessment for rapid disaster response with a deep object-based semantic change detection framework: From natural disasters to man-made disasters. Remote Sens. Environ. 2021 , 265 , 112636. [ Google Scholar ] [ CrossRef ]
- Chen, X.; Zhang, X.; Ren, M.; Zhou, B.; Sun, M.; Feng, Z.; Chen, B.; Zhi, X. A multiscale enhanced pavement crack segmentation network coupling spectral and spatial information of UAV hyperspectral imagery. Int. J. Appl. Earth Obs. Geoinf. 2024 , 128 , 103772. [ Google Scholar ] [ CrossRef ]
- Liu, F.; Liu, J.; Wang, L. Deep learning and infrared thermography for asphalt pavement crack severity classification. Autom. Constr. 2022 , 140 , 104383. [ Google Scholar ] [ CrossRef ]
- Liu, S.; Han, Y.; Xu, L. Recognition of road cracks based on multi-scale retinex fused with wavelet transform. Array 2022 , 15 , 100193. [ Google Scholar ] [ CrossRef ]
- Zhang, H.; Qian, Z.; Tan, Y.; Xie, Y.; Li, M. Investigation of pavement crack detection based on deep learning method using weakly supervised instance segmentation framework. Constr. Build. Mater. 2022 , 358 , 129117. [ Google Scholar ] [ CrossRef ]
- Dorafshan, S.; Thomas, R.J.; Maguire, M. Comparison of deep convolutional neural networks and edge detectors for image-based crack detection in concrete. Constr. Build. Mater. 2018 , 186 , 1031–1045. [ Google Scholar ] [ CrossRef ]
- Munawar, H.S.; Hammad, A.W.; Haddad, A.; Soares, C.A.P.; Waller, S.T. Image-based crack detection methods: A review. Infrastructures 2021 , 6 , 115. [ Google Scholar ] [ CrossRef ]
- Chen, D.; Li, X.; Hu, F.; Mathiopoulos, P.T.; Di, S.; Sui, M.; Peethambaran, J. Edpnet: An encoding–decoding network with pyramidal representation for semantic image segmentation. Sensors 2023 , 23 , 3205. [ Google Scholar ] [ CrossRef ]
- Mo, S.; Shi, Y.; Yuan, Q.; Li, M. A survey of deep learning road extraction algorithms using high-resolution remote sensing images. Sensors 2024 , 24 , 1708. [ Google Scholar ] [ CrossRef ]
- Chen, D.; Li, J.; Di, S.; Peethambaran, J.; Xiang, G.; Wan, L.; Li, X. Critical points extraction from building façades by analyzing gradient structure tensor. Remote Sens. 2021 , 13 , 3146. [ Google Scholar ] [ CrossRef ]
- Liu, Y.; Yeoh, J.K.; Chua, D.K. Deep learning-based enhancement of motion blurred UAV concrete crack images. J. Comput. Civ. Eng. 2020 , 34 , 04020028. [ Google Scholar ] [ CrossRef ]
- Flah, M.; Nunez, I.; Ben Chaabene, W.; Nehdi, M.L. Machine learning algorithms in civil structural health monitoring: A systematic review. Arch. Comput. Methods Eng. 2021 , 28 , 2621–2643. [ Google Scholar ] [ CrossRef ]
- Li, G.; Li, X.; Zhou, J.; Liu, D.; Ren, W. Pixel-level bridge crack detection using a deep fusion about recurrent residual convolution and context encoder network. Measurement 2021 , 176 , 109171. [ Google Scholar ] [ CrossRef ]
- Ali, R.; Chuah, J.H.; Talip, M.S.A.; Mokhtar, N.; Shoaib, M.A. Structural crack detection using deep convolutional neural networks. Autom. Constr. 2022 , 133 , 103989. [ Google Scholar ] [ CrossRef ]
- Wang, H.; Li, Y.; Dang, L.M.; Lee, S.; Moon, H. Pixel-level tunnel crack segmentation using a weakly supervised annotation approach. Comput. Ind. 2021 , 133 , 103545. [ Google Scholar ] [ CrossRef ]
- Zhu, J.; Song, J. Weakly supervised network based intelligent identification of cracks in asphalt concrete bridge deck. Alex. Eng. J. 2020 , 59 , 1307–1317. [ Google Scholar ] [ CrossRef ]
- Li, Y.; Bao, T.; Xu, B.; Shu, X.; Zhou, Y.; Du, Y.; Wang, R.; Zhang, K. A deep residual neural network framework with transfer learning for concrete dams patch-level crack classification and weakly-supervised localization. Measurement 2022 , 188 , 110641. [ Google Scholar ] [ CrossRef ]
- Yang, Q.; Shi, W.; Chen, J.; Lin, W. Deep convolution neural network-based transfer learning method for civil infrastructure crack detection. Autom. Constr. 2020 , 116 , 103199. [ Google Scholar ] [ CrossRef ]
- Dais, D.; Bal, I.E.; Smyrou, E.; Sarhosis, V. Automatic crack classification and segmentation on masonry surfaces using convolutional neural networks and transfer learning. Autom. Constr. 2021 , 125 , 103606. [ Google Scholar ] [ CrossRef ]
- Abdellatif, M.; Peel, H.; Cohn, A.G.; Fuentes, R. Combining block-based and pixel-based approaches to improve crack detection and localisation. Autom. Constr. 2021 , 122 , 103492. [ Google Scholar ] [ CrossRef ]
- Dan, D.; Dan, Q. Automatic recognition of surface cracks in bridges based on 2D-APES and mobile machine vision. Measurement 2021 , 168 , 108429. [ Google Scholar ] [ CrossRef ]
- Weng, X.; Huang, Y.; Wang, W. Segment-based pavement crack quantification. Autom. Constr. 2019 , 105 , 102819. [ Google Scholar ] [ CrossRef ]
- Kao, S.-P.; Chang, Y.-C.; Wang, F.-L. Combining the YOLOv4 deep learning model with UAV imagery processing technology in the extraction and quantization of cracks in bridges. Sensors 2023 , 23 , 2572. [ Google Scholar ] [ CrossRef ] [ PubMed ]
- Li, X.; Xu, X.; He, X.; Wei, X.; Yang, H. Intelligent crack detection method based on GM-ResNet. Sensors 2023 , 23 , 8369. [ Google Scholar ] [ CrossRef ] [ PubMed ]
- Choi, Y.; Park, H.W.; Mi, Y.; Song, S. Crack detection and analysis of concrete structures based on neural network and clustering. Sensors 2024 , 24 , 1725. [ Google Scholar ] [ CrossRef ] [ PubMed ]
- Guo, J.-M.; Markoni, H.; Lee, J.-D. BARNet: Boundary aware refinement network for crack detection. IEEE Trans. Intell. Transp. Syst. 2021 , 23 , 7343–7358. [ Google Scholar ] [ CrossRef ]
- Luo, J.; Lin, H.; Wei, X.; Wang, Y. Adaptive canny and semantic segmentation networks based on feature fusion for road crack detection. IEEE Access 2023 , 11 , 51740–51753. [ Google Scholar ] [ CrossRef ]
- Ranyal, E.; Sadhu, A.; Jain, K. Enhancing pavement health assessment: An attention-based approach for accurate crack detection, measurement, and mapping. Expert Syst. Appl. 2024 , 247 , 123314. [ Google Scholar ] [ CrossRef ]
- Liu, K.; Chen, B.M. Industrial UAV-based unsupervised domain adaptive crack recognitions: From database towards real-site infrastructural inspections. IEEE Trans. Ind. Electron. 2022 , 70 , 9410–9420. [ Google Scholar ] [ CrossRef ]
- Wang, W.; Hu, W.; Wang, W.; Xu, X.; Wang, M.; Shi, Y.; Qiu, S.; Tutumluer, E. Automated crack severity level detection and classification for ballastless track slab using deep convolutional neural network. Autom. Constr. 2021 , 124 , 103484. [ Google Scholar ] [ CrossRef ]
- Xu, Z.; Zhang, X.; Chen, W.; Liu, J.; Xu, T.; Wang, Z. Muraldiff: Diffusion for ancient murals restoration on large-scale pre-training. IEEE Trans. Emerg. Top. Comput. Intell. 2024 , 8 , 2169–2181. [ Google Scholar ] [ CrossRef ]
- Bradley, D.; Roth, G. Adaptive thresholding using the integral image. J. Graph. Tools 2007 , 12 , 13–21. [ Google Scholar ] [ CrossRef ]
- Sezgin, M.; Sankur, B.l. Survey over image thresholding techniques and quantitative performance evaluation. J. Electron. Imaging 2004 , 13 , 146–168. [ Google Scholar ]
- Kapur, J.N.; Sahoo, P.K.; Wong, A.K. A new method for gray-level picture thresholding using the entropy of the histogram. Comput. Vis. Graph. Image Process. 1985 , 29 , 273–285. [ Google Scholar ] [ CrossRef ]
- Pal, N.R.; Pal, S.K. A review on image segmentation techniques. Pattern Recognit. 1993 , 26 , 1277–1294. [ Google Scholar ] [ CrossRef ]
- Flah, M.; Suleiman, A.R.; Nehdi, M.L. Classification and quantification of cracks in concrete structures using deep learning image-based techniques. Cem. Concr. Compos. 2020 , 114 , 103781. [ Google Scholar ] [ CrossRef ]
- Mazni, M.; Husain, A.R.; Shapiai, M.I.; Ibrahim, I.S.; Anggara, D.W.; Zulkifli, R. An investigation into real-time surface crack classification and measurement for structural health monitoring using transfer learning convolutional neural networks and otsu method. Alex. Eng. J. 2024 , 92 , 310–320. [ Google Scholar ] [ CrossRef ]
- He, Z.; Xu, W. Deep learning and image preprocessing-based crack repair trace and secondary crack classification detection method for concrete bridges. Struct. Infrastruct. Eng. 2024 , 20 , 1–17. [ Google Scholar ] [ CrossRef ]
- He, T.; Li, H.; Qian, Z.; Niu, C.; Huang, R. Research on weakly supervised pavement crack segmentation based on defect location by generative adversarial network and target re-optimization. Constr. Build. Mater. 2024 , 411 , 134668. [ Google Scholar ] [ CrossRef ]
- Su, H.; Wang, X.; Han, T.; Wang, Z.; Zhao, Z.; Zhang, P. Research on a U-Net bridge crack identification and feature-calculation methods based on a CBAM attention mechanism. Buildings 2022 , 12 , 1561. [ Google Scholar ] [ CrossRef ]
- Kang, D.; Benipal, S.S.; Gopal, D.L.; Cha, Y.-J. Hybrid pixel-level concrete crack segmentation and quantification across complex backgrounds using deep learning. Autom. Constr. 2020 , 118 , 103291. [ Google Scholar ] [ CrossRef ]
- Lei, Q.; Zhong, J.; Wang, C. Joint optimization of crack segmentation with an adaptive dynamic threshold module. IEEE Trans. Intell. Transp. Syst. 2024 , 25 , 6902–6916. [ Google Scholar ] [ CrossRef ]
- Lei, Q.; Zhong, J.; Wang, C.; Xia, Y.; Zhou, Y. Dynamic thresholding for accurate crack segmentation using multi-objective optimization. In Joint European Conference on Machine Learning and Knowledge Discovery in Databases, Turin, Italy, 18 September 2023 ; Springer: Cham, Switzerland, 2023; pp. 389–404. [ Google Scholar ]
- Vincent, L.; Soille, P. Watersheds in digital spaces: An efficient algorithm based on immersion simulations. IEEE Trans. Pattern Anal. Mach. Intell. 1991 , 13 , 583–598. [ Google Scholar ] [ CrossRef ]
- Huang, H.; Zhao, S.; Zhang, D.; Chen, J. Deep learning-based instance segmentation of cracks from shield tunnel lining images. Struct. Infrastruct. Eng. 2022 , 18 , 183–196. [ Google Scholar ] [ CrossRef ]
- Fan, Z.; Lin, H.; Li, C.; Su, J.; Bruno, S.; Loprencipe, G. Use of parallel resnet for high-performance pavement crack detection and measurement. Sustainability 2022 , 14 , 1825. [ Google Scholar ] [ CrossRef ]
- Kong, S.Y.; Fan, J.S.; Liu, Y.F.; Wei, X.C.; Ma, X.W. Automated crack assessment and quantitative growth monitoring. Comput.-Aided Civ. Infrastruct. Eng. 2021 , 36 , 656–674. [ Google Scholar ] [ CrossRef ]
- Dang, L.M.; Wang, H.; Li, Y.; Park, Y.; Oh, C.; Nguyen, T.N.; Moon, H. Automatic tunnel lining crack evaluation and measurement using deep learning. Tunn. Undergr. Space Technol. 2022 , 124 , 104472. [ Google Scholar ] [ CrossRef ]
- Andrushia, A.D.; Anand, N.; Lubloy, E. Deep learning based thermal crack detection on structural concrete exposed to elevated temperature. Adv. Struct. Eng. 2021 , 24 , 1896–1909. [ Google Scholar ] [ CrossRef ]
- Dang, L.M.; Wang, H.; Li, Y.; Nguyen, L.Q.; Nguyen, T.N.; Song, H.-K.; Moon, H. Deep learning-based masonry crack segmentation and real-life crack length measurement. Constr. Build. Mater. 2022 , 359 , 129438. [ Google Scholar ] [ CrossRef ]
- Nguyen, A.; Gharehbaghi, V.; Le, N.T.; Sterling, L.; Chaudhry, U.I.; Crawford, S. ASR crack identification in bridges using deep learning and texture analysis. Structures 2023 , 50 , 494–507. [ Google Scholar ] [ CrossRef ]
- Dong, C.; Li, L.; Yan, J.; Zhang, Z.; Pan, H.; Catbas, F.N. Pixel-level fatigue crack segmentation in large-scale images of steel structures using an encoder–decoder network. Sensors 2021 , 21 , 4135. [ Google Scholar ] [ CrossRef ] [ PubMed ]
- Jian, L.; Chengshun, L.; Guanhong, L.; Zhiyuan, Z.; Bo, H.; Feng, G.; Quanyi, X. Lightweight defect detection equipment for road tunnels. IEEE Sens. J. 2023 , 24 , 5107–5121. [ Google Scholar ]
- Liang, H.; Qiu, D.; Ding, K.-L.; Zhang, Y.; Wang, Y.; Wang, X.; Liu, T.; Wan, S. Automatic pavement crack detection in multisource fusion images using similarity and difference features. IEEE Sens. J. 2023 , 24 , 5449–5465. [ Google Scholar ] [ CrossRef ]
- Alamdari, A.G.; Ebrahimkhanlou, A. A multi-scale robotic approach for precise crack measurement in concrete structures. Autom. Constr. 2024 , 158 , 105215. [ Google Scholar ] [ CrossRef ]
- Liu, H.; Kollosche, M.; Laflamme, S.; Clarke, D.R. Multifunctional soft stretchable strain sensor for complementary optical and electrical sensing of fatigue cracks. Smart Mater. Struct. 2023 , 32 , 045010. [ Google Scholar ] [ CrossRef ]
- Dang, D.-Z.; Wang, Y.-W.; Ni, Y.-Q. Nonlinear autoregression-based non-destructive evaluation approach for railway tracks using an ultrasonic fiber bragg grating array. Constr. Build. Mater. 2024 , 411 , 134728. [ Google Scholar ] [ CrossRef ]
- Yan, M.; Tan, X.; Mahjoubi, S.; Bao, Y. Strain transfer effect on measurements with distributed fiber optic sensors. Autom. Constr. 2022 , 139 , 104262. [ Google Scholar ] [ CrossRef ]
- Shukla, H.; Piratla, K. Leakage detection in water pipelines using supervised classification of acceleration signals. Autom. Constr. 2020 , 117 , 103256. [ Google Scholar ] [ CrossRef ]
- Chen, X.; Zhang, X.; Li, J.; Ren, M.; Zhou, B. A new method for automated monitoring of road pavement aging conditions based on recurrent neural network. IEEE Trans. Intell. Transp. Syst. 2022 , 23 , 24510–24523. [ Google Scholar ] [ CrossRef ]
- Zhang, S.; He, X.; Xue, B.; Wu, T.; Ren, K.; Zhao, T. Segment-anything embedding for pixel-level road damage extraction using high-resolution satellite images. Int. J. Appl. Earth Obs. Geoinf. 2024 , 131 , 103985. [ Google Scholar ] [ CrossRef ]
- Park, S.E.; Eem, S.-H.; Jeon, H. Concrete crack detection and quantification using deep learning and structured light. Constr. Build. Mater. 2020 , 252 , 119096. [ Google Scholar ] [ CrossRef ]
- Yan, Y.; Mao, Z.; Wu, J.; Padir, T.; Hajjar, J.F. Towards automated detection and quantification of concrete cracks using integrated images and lidar data from unmanned aerial vehicles. Struct. Control Health Monit. 2021 , 28 , e2757. [ Google Scholar ] [ CrossRef ]
- Dong, Q.; Wang, S.; Chen, X.; Jiang, W.; Li, R.; Gu, X. Pavement crack detection based on point cloud data and data fusion. Philos. Trans. R. Soc. A 2023 , 381 , 20220165. [ Google Scholar ] [ CrossRef ] [ PubMed ]
- Kim, H.; Lee, S.; Ahn, E.; Shin, M.; Sim, S.-H. Crack identification method for concrete structures considering angle of view using RGB-D camera-based sensor fusion. Struct. Health Monit. 2021 , 20 , 500–512. [ Google Scholar ] [ CrossRef ]
- Chen, J.; Lu, W.; Lou, J. Automatic concrete defect detection and reconstruction by aligning aerial images onto semantic-rich building information model. Comput.-Aided Civ. Infrastruct. Eng. 2023 , 38 , 1079–1098. [ Google Scholar ] [ CrossRef ]
- Pozzer, S.; Rezazadeh Azar, E.; Dalla Rosa, F.; Chamberlain Pravia, Z.M. Semantic segmentation of defects in infrared thermographic images of highly damaged concrete structures. J. Perform. Constr. Facil. 2021 , 35 , 04020131. [ Google Scholar ] [ CrossRef ]
- Kaur, R.; Singh, S. A comprehensive review of object detection with deep learning. Digit. Signal Process. 2023 , 132 , 103812. [ Google Scholar ] [ CrossRef ]
- Sharma, V.K.; Mir, R.N. A comprehensive and systematic look up into deep learning based object detection techniques: A review. Comput. Sci. Rev. 2020 , 38 , 100301. [ Google Scholar ] [ CrossRef ]
- Zhang, L.; Yang, F.; Zhang, Y.D.; Zhu, Y.J. Road crack detection using deep convolutional neural network. In Proceedings of the 2016 IEEE International Conference on Image Processing (ICIP), Phoenix, AZ, USA, 25–28 September 2016; IEEE: Piscataway, NJ, USA, 2016; pp. 3708–3712. [ Google Scholar ]
- Yang, C.; Chen, J.; Li, Z.; Huang, Y. Structural crack detection and recognition based on deep learning. Appl. Sci. 2021 , 11 , 2868. [ Google Scholar ] [ CrossRef ]
- Rajadurai, R.-S.; Kang, S.-T. Automated vision-based crack detection on concrete surfaces using deep learning. Appl. Sci. 2021 , 11 , 5229. [ Google Scholar ] [ CrossRef ]
- Kim, B.; Yuvaraj, N.; Sri Preethaa, K.; Arun Pandian, R. Surface crack detection using deep learning with shallow CNN architecture for enhanced computation. Neural Comput. Appl. 2021 , 33 , 9289–9305. [ Google Scholar ] [ CrossRef ]
- O’Brien, D.; Osborne, J.A.; Perez-Duenas, E.; Cunningham, R.; Li, Z. Automated crack classification for the CERN underground tunnel infrastructure using deep learning. Tunn. Undergr. Space Technol. 2023 , 131 , 104668. [ Google Scholar ]
- Chen, K.; Reichard, G.; Xu, X.; Akanmu, A. Automated crack segmentation in close-range building façade inspection images using deep learning techniques. J. Build. Eng. 2021 , 43 , 102913. [ Google Scholar ] [ CrossRef ]
- Dong, Z.; Wang, J.; Cui, B.; Wang, D.; Wang, X. Patch-based weakly supervised semantic segmentation network for crack detection. Constr. Build. Mater. 2020 , 258 , 120291. [ Google Scholar ] [ CrossRef ]
- Buatik, A.; Thansirichaisree, P.; Kalpiyapun, P.; Khademi, N.; Pasityothin, I.; Poovarodom, N. Mosaic crack mapping of footings by convolutional neural networks. Sci. Rep. 2024 , 14 , 7851. [ Google Scholar ] [ CrossRef ] [ PubMed ]
- Zhang, Y.; Zhang, L. Detection of pavement cracks by deep learning models of transformer and UNet. arXiv 2023 , arXiv:2304.12596. [ Google Scholar ] [ CrossRef ]
- Al-Huda, Z.; Peng, B.; Algburi, R.N.A.; Al-antari, M.A.; Rabea, A.-J.; Zhai, D. A hybrid deep learning pavement crack semantic segmentation. Eng. Appl. Artif. Intell. 2023 , 122 , 106142. [ Google Scholar ] [ CrossRef ]
- Shamsabadi, E.A.; Xu, C.; Rao, A.S.; Nguyen, T.; Ngo, T.; Dias-da-Costa, D. Vision transformer-based autonomous crack detection on asphalt and concrete surfaces. Autom. Constr. 2022 , 140 , 104316. [ Google Scholar ] [ CrossRef ]
- Huang, S.; Tang, W.; Huang, G.; Huangfu, L.; Yang, D. Weakly supervised patch label inference networks for efficient pavement distress detection and recognition in the wild. IEEE Trans. Intell. Transp. Syst. 2023 , 24 , 5216–5228. [ Google Scholar ] [ CrossRef ]
- Huang, G.; Huang, S.; Huangfu, L.; Yang, D. Weakly supervised patch label inference network with image pyramid for pavement diseases recognition in the wild. In Proceedings of the ICASSP 2021—2021 IEEE International Conference on Acoustics, Speech and Signal Processing (ICASSP), Toronto, ON, Canada, 6–11 June 2021; IEEE: Piscataway, NJ, USA, 2021; pp. 7978–7982. [ Google Scholar ]
- Guo, J.-M.; Markoni, H. Efficient and adaptable patch-based crack detection. IEEE Trans. Intell. Transp. Syst. 2022 , 23 , 21885–21896. [ Google Scholar ] [ CrossRef ]
- König, J.; Jenkins, M.D.; Mannion, M.; Barrie, P.; Morison, G. Weakly-supervised surface crack segmentation by generating pseudo-labels using localization with a classifier and thresholding. IEEE Trans. Intell. Transp. Syst. 2022 , 23 , 24083–24094. [ Google Scholar ] [ CrossRef ]
- Al-Huda, Z.; Peng, B.; Algburi, R.N.A.; Al-antari, M.A.; Rabea, A.-J.; Al-maqtari, O.; Zhai, D. Asymmetric dual-decoder-U-Net for pavement crack semantic segmentation. Autom. Constr. 2023 , 156 , 105138. [ Google Scholar ] [ CrossRef ]
- Wen, T.; Lang, H.; Ding, S.; Lu, J.J.; Xing, Y. PCDNet: Seed operation-based deep learning model for pavement crack detection on 3d asphalt surface. J. Transp. Eng. Part B Pavements 2022 , 148 , 04022023. [ Google Scholar ] [ CrossRef ]
- Mishra, A.; Gangisetti, G.; Eftekhar Azam, Y.; Khazanchi, D. Weakly supervised crack segmentation using crack attention networks on concrete structures. Struct. Health Monit. 2024 , 23 , 14759217241228150. [ Google Scholar ] [ CrossRef ]
- Kompanets, A.; Pai, G.; Duits, R.; Leonetti, D.; Snijder, B. Deep learning for segmentation of cracks in high-resolution images of steel bridges. arXiv 2024 , arXiv:2403.17725. [ Google Scholar ]
- Liu, Y.; Yeoh, J.K. Robust pixel-wise concrete crack segmentation and properties retrieval using image patches. Autom. Constr. 2021 , 123 , 103535. [ Google Scholar ] [ CrossRef ]
- Girshick, R.; Donahue, J.; Darrell, T.; Malik, J. Rich feature hierarchies for accurate object detection and semantic segmentation. In Proceedings of the IEEE Conference on Computer Vision and Pattern Recognition, Columbus, OH, USA, 23–28 June 2014; pp. 580–587. [ Google Scholar ]
- Girshick, R. Fast R-CNN. In Proceedings of the IEEE International Conference on Computer Vision, Santiago, Chile, 7–13 December 2015; pp. 1440–1448. [ Google Scholar ]
- Ren, S.; He, K.; Girshick, R.; Sun, J. Faster R-CNN: Towards real-time object detection with region proposal networks. In Proceedings of the Advances in Neural Information Processing Systems, Montreal, QC, Canada, 7–12 December 2015; Volume 28. [ Google Scholar ]
- He, K.; Gkioxari, G.; Dollár, P.; Girshick, R. Mask R-CNN. In Proceedings of the IEEE International Conference on Computer Vision, Venice, Italy, 22–29 October 2017; pp. 2961–2969. [ Google Scholar ]
- Redmon, J.; Divvala, S.; Girshick, R.; Farhadi, A. You only look once: Unified, real-time object detection. In Proceedings of the IEEE Conference on Computer Vision and Pattern Recognition, Las Vegas, NV, USA, 27–30 June 2016; pp. 779–788. [ Google Scholar ]
- Redmon, J.; Farhadi, A. YOLO9000: Better, faster, stronger. In Proceedings of the IEEE Conference on Computer Vision and Pattern Recognition, Honolulu, HI, USA, 21–26 July 2017; pp. 7263–7271. [ Google Scholar ]
- Redmon, J.; Farhadi, A. Yolov3: An incremental improvement. arXiv 2018 , arXiv:1804.02767. [ Google Scholar ]
- Bochkovskiy, A.; Wang, C.-Y.; Liao, H.-Y.M. Yolov4: Optimal speed and accuracy of object detection. arXiv 2020 , arXiv:2004.10934. [ Google Scholar ]
- Wang, C.-Y.; Bochkovskiy, A.; Liao, H.-Y.M. Yolov7: Trainable bag-of-freebies sets new state-of-the-art for real-time object detectors. In Proceedings of the IEEE/CVF Conference on Computer Vision and Pattern Recognition, Vancouver, BC, Canada, 18–22 June 2023; pp. 7464–7475. [ Google Scholar ]
- Liu, W.; Anguelov, D.; Erhan, D.; Szegedy, C.; Reed, S.; Fu, C.-Y.; Berg, A.C. SSD: Single shot multibox detector. In Proceedings of the Computer Vision–ECCV 2016: 14th European Conference, Amsterdam, The Netherlands, 11–14 October 2016; Part I 14. Springer: Berlin/Heidelberg, Germany, 2016; pp. 21–37. [ Google Scholar ]
- Lin, T.-Y.; Goyal, P.; Girshick, R.; He, K.; Dollár, P. Focal loss for dense object detection. In Proceedings of the IEEE International Conference on Computer Vision, Venice, Italy, 22–29 October 2017; pp. 2980–2988. [ Google Scholar ]
- Xu, Y.; Li, D.; Xie, Q.; Wu, Q.; Wang, J. Automatic defect detection and segmentation of tunnel surface using modified mask R-CNN. Measurement 2021 , 178 , 109316. [ Google Scholar ] [ CrossRef ]
- Zhao, W.; Liu, Y.; Zhang, J.; Shao, Y.; Shu, J. Automatic pixel-level crack detection and evaluation of concrete structures using deep learning. Struct. Control Health Monit. 2022 , 29 , e2981. [ Google Scholar ] [ CrossRef ]
- Li, R.; Yu, J.; Li, F.; Yang, R.; Wang, Y.; Peng, Z. Automatic bridge crack detection using unmanned aerial vehicle and faster R-CNN. Constr. Build. Mater. 2023 , 362 , 129659. [ Google Scholar ] [ CrossRef ]
- Tran, T.S.; Nguyen, S.D.; Lee, H.J.; Tran, V.P. Advanced crack detection and segmentation on bridge decks using deep learning. Constr. Build. Mater. 2023 , 400 , 132839. [ Google Scholar ] [ CrossRef ]
- Zhang, J.; Qian, S.; Tan, C. Automated bridge crack detection method based on lightweight vision models. Complex Intell. Syst. 2023 , 9 , 1639–1652. [ Google Scholar ] [ CrossRef ]
- Ren, R.; Liu, F.; Shi, P.; Wang, H.; Huang, Y. Preprocessing of crack recognition: Automatic crack-location method based on deep learning. J. Mater. Civ. Eng. 2023 , 35 , 04022452. [ Google Scholar ] [ CrossRef ]
- Liu, Z.; Yeoh, J.K.; Gu, X.; Dong, Q.; Chen, Y.; Wu, W.; Wang, L.; Wang, D. Automatic pixel-level detection of vertical cracks in asphalt pavement based on gpr investigation and improved mask R-CNN. Autom. Constr. 2023 , 146 , 104689. [ Google Scholar ] [ CrossRef ]
- Li, Z.; Zhu, H.; Huang, M. A deep learning-based fine crack segmentation network on full-scale steel bridge images with complicated backgrounds. IEEE Access 2021 , 9 , 114989–114997. [ Google Scholar ] [ CrossRef ]
- Alipour, M.; Harris, D.K.; Miller, G.R. Robust pixel-level crack detection using deep fully convolutional neural networks. J. Comput. Civ. Eng. 2019 , 33 , 04019040. [ Google Scholar ] [ CrossRef ]
- Wang, S.; Pan, Y.; Chen, M.; Zhang, Y.; Wu, X. FCN-SFW: Steel structure crack segmentation using a fully convolutional network and structured forests. IEEE Access 2020 , 8 , 214358–214373. [ Google Scholar ] [ CrossRef ]
- Hang, J.; Wu, Y.; Li, Y.; Lai, T.; Zhang, J.; Li, Y. A deep learning semantic segmentation network with attention mechanism for concrete crack detection. Struct. Health Monit. 2023 , 22 , 3006–3026. [ Google Scholar ] [ CrossRef ]
- Sun, Y.; Yang, Y.; Yao, G.; Wei, F.; Wong, M. Autonomous crack and bughole detection for concrete surface image based on deep learning. IEEE Access 2021 , 9 , 85709–85720. [ Google Scholar ] [ CrossRef ]
- Wang, Z.; Leng, Z.; Zhang, Z. A weakly-supervised transformer-based hybrid network with multi-attention for pavement crack detection. Constr. Build. Mater. 2024 , 411 , 134134. [ Google Scholar ] [ CrossRef ]
- Chen, T.; Cai, Z.; Zhao, X.; Chen, C.; Liang, X.; Zou, T.; Wang, P. Pavement crack detection and recognition using the architecture of segNet. J. Ind. Inf. Integr. 2020 , 18 , 100144. [ Google Scholar ] [ CrossRef ]
- Bai, S.; Ma, M.; Yang, L.; Liu, Y. Pixel-wise crack defect segmentation with dual-encoder fusion network. Constr. Build. Mater. 2024 , 426 , 136179. [ Google Scholar ] [ CrossRef ]
- Wang, W.; Su, C. Semi-supervised semantic segmentation network for surface crack detection. Autom. Constr. 2021 , 128 , 103786. [ Google Scholar ] [ CrossRef ]
- Tabernik, D.; Šela, S.; Skvarč, J.; Skočaj, D. Segmentation-based deep-learning approach for surface-defect detection. J. Intell. Manuf. 2020 , 31 , 759–776. [ Google Scholar ] [ CrossRef ]
- König, J.; Jenkins, M.D.; Mannion, M.; Barrie, P.; Morison, G. Optimized deep encoder-decoder methods for crack segmentation. Digit. Signal Process. 2021 , 108 , 102907. [ Google Scholar ] [ CrossRef ]
- Wang, C.; Liu, H.; An, X.; Gong, Z.; Deng, F. Swincrack: Pavement crack detection using convolutional swin-transformer network. Digit. Signal Process. 2024 , 145 , 104297. [ Google Scholar ] [ CrossRef ]
- Lan, Z.-X.; Dong, X.-M. Minicrack: A simple but efficient convolutional neural network for pixel-level narrow crack detection. Comput. Ind. 2022 , 141 , 103698. [ Google Scholar ] [ CrossRef ]
- Salton, G. Introduction to Modern Information Retrieval ; McGraw-Hill: New York, NY, USA, 1983. [ Google Scholar ]
- Jenkins, M.D.; Carr, T.A.; Iglesias, M.I.; Buggy, T.; Morison, G. A deep convolutional neural network for semantic pixel-wise segmentation of road and pavement surface cracks. In Proceedings of the 2018 26th European Signal Processing Conference (EUSIPCO), Rome, Italy, 3–7 September 2018; IEEE: Piscataway, NJ, USA; pp. 2120–2124. [ Google Scholar ]
- Tsai, Y.-C.; Chatterjee, A. Comprehensive, quantitative crack detection algorithm performance evaluation system. J. Comput. Civ. Eng. 2017 , 31 , 04017047. [ Google Scholar ] [ CrossRef ]
- Li, H.; Wang, J.; Zhang, Y.; Wang, Z.; Wang, T. A study on evaluation standard for automatic crack detection regard the random fractal. arXiv 2020 , arXiv:2007.12082. [ Google Scholar ]
Click here to enlarge figure
Method | Features | Domain | Dataset | Image Device/Source | Results | Limitations |
---|---|---|---|---|---|---|
Canny and YOLOv4 [ ] | Crack detection and measurement | Bridges | 1463 images 256 × 256 pixels | Smartphone and DJI UAV | Accuracy = 92% mAP = 92% | The Canny edge detector is affected by the threshold |
Canny and GM-ResNet [ ] | Crack detection, measurement, and classification | Road | 522 images 224 × 224 pixels | Concrete crack sub-dataset | Precision = 97.9% Recall = 98.9% F1 measure = 98.0% Accuracy in shadow conditions = 99.3% Accuracy in shadow-free conditions = 99.9% | Its detection performance for complex cracks is not yet perfect |
Sobel and ResNet50 [ ] | Crack detection | Concrete | 4500 images 100 × 100 pixels | FLIR E8 | Precision = 98.4% Recall = 88.7% F1 measure = 93.2% | - |
Sobel and BARNet [ ] | Crack detection and localization | Road | 206 images 800 × 600 pixels | CrackTree200 dataset | AIU = 19.85% ODS = 79.9% OIS = 81.4% | Hyperparameter tuning is needed to balance the penalty weights for different types of cracks |
Canny and DeepLabV3+ [ ] | Crack detection | Road | 2000 × 1500 pixels | Crack500 dataset | MIoU = 77.64% MAE = 1.55 PA = 97.38% F1 score = 63% | Detection performance deteriorating in dark environments or when interfering objects are present |
Canny and RetinaNet [ ] | Crack detection and measurement | Road | 850 images 256 × 256 pixels | SDNET 2018 dataset | Precision = 85.96% Recall = 84.48% F1 score = 85.21% | - |
Canny and Transformer [ ] | Crack detection and segmentation | Buildings | 11298 images 450 × 450 pixels | UAVs | GA = 83.5% MIoU = 76.2% Precision = 74.3% Recall = 75.2% F1 score = 74.7% | Resulting in a marginal increment in computational costs for various network backbones |
Canny and Inception-ResNet-v2 [ ] | Crack detection, measurement, and classification | High-speed railway | 4650 images 400 × 400 pixels | The track inspection vehicle | High severity level: Precision = 98.37% Recall = 93.82% F1 score = 95.99% Low severity level: Precision = 94.25% Recall = 98.39% F1 score = 96.23% | Only the average width was used to define the severity of the crack, and the influence of the length on the detection result was not considered |
Canny and Unet [ ] | Crack detection | Buildings | 165 images | - | SSIM = 14.5392 PSNR = 0.3206 RMSE = 0.0747 | Relies on a large amount of mural data for training and enhancement |
Method | Features | Domain | Dataset | Image Device/Source | Results | Limitations |
---|---|---|---|---|---|---|
Otsu and Keras classifier [ ] | Crack detection, measurement, and classification | Concrete | 4000 images 227 × 227 pixels | Open dataset available | Classifiers accuracy = 98.25%, 97.18%, 96.17% Length error = 1.5% Width error = 5% Angle of orientation error = 2% | Only accurately quantify one single crack per image |
Otsu and TL MobileNetV2 [ ] | Crack detection, measurement, and classification | Concrete | 11435 images 224 × 224 pixels | Mendeley data—crack detection | Accuracy = 99.87% Recall = 99.74% Precision = 100% F1 score = 99.87% | Dependency on image quality |
Otsu, YOLOv7, Poisson noise, and bilateral filtering [ ] | Crack detection and classification | Bridges | 500 images 640 × 640 pixels | Dataset | Training time = 35 min Inference time = 8.9 s Target correct rate = 85.97% Negative sample misclassification rate = 42.86% | It does not provide quantified information such as length and area |
Adaptive threshold and WSIS [ ] | Crack detection | Road | 320 images 3024 × 4032 pixels | Photos of cracks | Recall = 90% Precision = 52% IoU = 50% F1 score = 66% Accuracy = 98% | For some small cracks (with a width of less than 3 pixels), model can only identify the existence of small cracks, but it is difficult to depict the cracks in detail |
Adaptive threshold and U-GAT-IT [ ] | Crack detection | Road | 300 training images and237 test images | DeepCrack dataset | Recall = 79.3% Precision = 82.2% F1 score = 80.7% | Further research is needed to address the interference caused by factors such as small cracks, road shadows, and water stains |
Local thresholding and DCNN [ ] | Crack detection | Concrete | 125 images 227 × 227 pixels | Cameras | Accuracy = 93% Recall = 91% Precision = 92% F1 score = 91% | - |
Otsu and Faster R-CNN [ ] | Crack detection, localization, and quantification | Concrete | 100 images 1920 × 1080 pixels | Nikon d7200 camera and Galaxy s9 camera | AP = 95% mIoU = 83% RMSE = 2.6 pixels Length accuracy = 93% | The proposed method is useful for concrete cracks only; its applicability for the detection of other crack materials might be limited |
Adaptive Dynamic Thresholding Module (ADTM) and Mask DINO [ ] | Crack detection and segmentation | Road | 395 images 2000 × 1500 pixels | Crack500 | mIoU = 81.3% mAcc = 96.4% gAcc = 85.0% | ADTM module can only handle binary classification problems |
Dynamic Thresholding Branch and DeepCrack [ ] | Crack detection and classification | Bridges | 3648 × 5472 pixels | Crack500 | mIoU = 79.3% mAcc = 98.5% gAcc = 86.6% | Image-level thresholds lead to misclassification of the background |
Method | Features | Domain | Dataset | Image Device/Source | Results | Limitations |
---|---|---|---|---|---|---|
Morphological closing operations and Mask R-CNN [ ] | Crack detection | Tunnel | 761 images 227 × 227 pixels | MTI-200a | Balanced accuracy = 81.94% F1 score = 68.68% IoU = 52.72% | Relatively small compared to the needs of the required sample size for universal conditions |
Morphological operations and Parallel ResNet [ ] | Crack detection and measurement | Road | 206 images (CrackTree200) 800 × 600 pixels and 118 images (CFD) 320 × 480 pixels | CrackTree200 dataset and CFD dataset | CrackTree200: Precision = 94.27% Recall = 92.52% F1 = 93.08% CFD: Precision = 96.21% Recall = 95.12% F1 = 95.63% | The method was only performed on accurate static images |
Closing and CNN [ ] | Crack detection, measurement, and classification | Concrete | 3208 images 256 × 256 pixels or 128 × 128 pixels | Hand-held DSLR cameras | Relative error = 5% Accuracy > 95% Loss < 0.1 | The extraction of the cracks’ edge will have a larger influence on the results |
Dilation and TunnelURes [ ] | Crack detection, measurement, and classification | Tunnel | 6810 images image sizes vary 10441 × 2910 to 50739 × 3140 | Night 4K line-scan cameras | AUC = 0.97 PA = 0.928 IoU = 0.847 | The medial-axis skeletonization algorithm created many errors because it was susceptible to the crack intersection and the image edges where the crack’s representation changed |
Opening, closing, and U-Net [ ] | Crack detection, measurement, and classification | Concrete | 200 images 512 × 512 pixels | Canon SX510 HS camera | Precision = 96.52% Recall = 93.73% F measure = 96.12% Accuracy = 99.74% IoU = 78.12% | It can only detect the other type of cracks which have the same crack geometry as that of thermal cracks |
Morphological operations and DeepLabV3+ [ ] | Crack detection and measurement | Masonry structure | 200 images 780 × 355 pixels and 2880 × 1920 pixels | Internet, drones, and smartphones | IoU = 0.97 F1 score = 98% Accuracy = 98% | The model will not detect crack features that do not appear in the dataset (complicated cracks, tiny cracks, etc.) |
Erosion, texture analysis techniques, and InceptionV3 [ ] | Crack detection and classification | Bridges | 1706 images 256 × 256 pixels | Cameras | F1 score = 93.7% Accuracy = 94.07% | - |
U-Net, opening, and closing operations [ ] | Crack detection and segmentation | Bridges | 244 images 512 × 512 pixels | Cameras | mP = 44.57% mR = 53.13% Mf1 = 42.79% mIoU = 64.79% | The model lacks generality, and there are cases of false detection |
Sensor Type | Fusion Method | Advantages | Disadvantages | Application Scenarios |
---|---|---|---|---|
Optical sensor [ ] | Data-level fusion | High resolution, rich in details | Susceptible to light and occlusion | Surface crack detection, general environments |
Thermal sensor [ ] | Feature level fusion | Suitable for nighttime or low-light environments, detects temperature changes | Low resolution, lack of detail | Nighttime detection, heat-sensitive areas, large-area surface crack detection |
Laser sensor [ ] | Data-level fusion and feature level fusion | High-precision 3D point cloud data, accurately measures crack morphology | High equipment cost, complex data processing | Complex structures, precise measurements |
Strain sensor [ ] | Feature level fusion and decision-level fusion | High sensitivity to structural changes; durable | Requires contact with the material; installation complexity | Monitoring structural health in bridges and buildings; detecting early-stage crack development |
Ultrasonic sensor [ ] | Data-level fusion and feature level fusion | Detects internal cracks in materials, strong penetration | Affected by material and geometric shape, limited resolution | Internal cracks, metal material detection |
Optical fiber sensor [ ] | Feature level fusion | High sensitivity to changes in material properties, non-contact measurement | Affected by environmental conditions, requires calibration | Surface crack detection, structural health monitoring |
Vibration sensor [ ] | Data-level fusion | Detects structural vibration characteristics, strong adaptability | Affected by environmental vibrations, requires complex signal processing | Dynamic crack monitoring, bridges and other structures |
Multispectral satellite sensor [ ] | Data-level fusion | Rich spectral information | Limited spectral resolution, weather- and lighting-dependent, high cost | Pavement crack detection, bridge and infrastructure monitoring, building facade inspection |
High-resolution satellite sensors [ ] | Data-level fusion and feature level fusion | High spatial resolution, wide coverage, frequent revisit times, rich information content | Weather dependency, high cost, data processing complexity, limited temporal resolution | Road and pavement crack detection, bridge and infrastructure monitoring, urban building facade inspection, railway and highway crack monitoring |
Scale | Dataset/(Pixels × Pixels) | References |
---|---|---|
Image-based | 227 × 227 | [ , , , ] |
224 × 224 | [ ] | |
256 × 256 | [ ] | |
416 × 416 | [ ] | |
512 × 512 | [ ] | |
Patch-based | 128 × 128 | [ , ] |
200 × 200 | [ ] | |
224 × 224 | [ , , , , ] | |
227 × 227 | [ ] | |
256 × 256 | [ , ] | |
300 × 300 | [ , ] | |
320 × 480 | [ , ] | |
544 × 384 | [ ] | |
512 × 512 | [ , , , ] | |
584 × 384 | [ ] |
Model | Improvement/Innovation | Dataset | Backbone | Results |
---|---|---|---|---|
Faster R-CNN [ ] | Combined with drones for crack detection | 2000 images 5280 × 2970 pixels | VGG-16 | Precision = 92.03% Recall = 96.26% F1 score = 94.10% |
Faster R-CNN [ ] | Double-head structure is introduced, including an independent fully connected head and a convolution head | 1622 images 1612 × 1947 pixels | ResNet50 | AP = 47.2% |
Mask R-CNN [ ] | The morphological closing operation was incorporated into the M-R-101-FPN model to form an integrated model | 761 images 227 × 227 pixels | ResNets and VGG | Balanced accuracy = 81.94% F1 score = 68.68% IoU = 52.72% |
Mask R-CNN [ ] | PAFPN module and edge detection branch was introduced | 9680 images 1500 × 1500 pixels | ResNet-FPN | Precision = 92.03% Recall = 96.26% AP = 94.10% mAP = 90.57% Error rate = 0.57% |
Mask R-CNN [ ] | FPN structure introduces side join method and combines FPN with ResNet-101 to change RoI-Pooling layer to RoI-Align layer | 3430 images 1024 × 1024 pixels | ResNet101 | AP = 83.3% F1 score = 82.4% Average error = 2.33% mIoU = 70.1% |
YOLOv3-tiny [ ] | A structural crack detection and quantification method combined with structured light is proposed | 500 images 640 × 640 pixels | Darknet-53 | Accuracy = 94% Precision = 98% |
YOLOv4 [ ] | Some lightweight networks were used instead of the original backbone feature extraction network, and DenseNet, MobileNet, and GhostNet were selected for the lightweight networks | 800 images 416 × 416 pixels | DenseNet, MobileNet v1, MobileNet v2, MobileNet v3, and GhostNet | Precision = 93.96% Recall = 90.12% F1 score = 92% |
YOLOv4 [ ] | - | 1463 images 256 × 256 pixels | Darknet-53 | Accuracy = 92% mAP = 92% |
Datasets Name | Number of Images | Image Resolution | Manual Annotation | Scope of Applicability | Limitations |
---|---|---|---|---|---|
CrackTree200 [ ] | 206 images | 800 × 600 pixels | Pixel-level annotations for cracks | Crack classification and segmentation | With only 200 images, the dataset’s relatively small size can hinder the model’s ability to generalize across diverse conditions, potentially leading to overfitting on the specific examples provided |
Crack500 [ ] | 500 images | 2000 × 1500 pixels | Pixel-level annotations for cracks | Crack classification and segmentation | Limited number of images compared to larger datasets, which might affect the generalization of models trained on this dataset |
SDNET 2018 [ ] | 56000 images | 256 × 256 pixels | Pixel-level annotations for cracks | Crack classification and segmentation | The dataset’s focus on concrete surfaces may limit the model’s performance when applied to different types of surfaces or structures |
Mendeley data—crack detection [ ] | 40000 images | 227 × 227 pixels | Pixel-level annotations for cracks | Crack classification | The dataset might not cover all types of cracks or surface conditions, which can limit its applicability to a wide range of real-world scenarios |
DeepCrack [ ] | 2500 images | 512 × 512 pixels | Annotations for cracks | Crack segmentation | The resolution might limit the ability of models to capture very small or subtle crack features |
CFD [ ] | 118 images | 320 × 480 pixels | Pixel-level annotations for cracks | Crack segmentation | The dataset contains a limited number of data samples, which may limit the generalization ability of the model |
CrackTree260 [ ] | 260 images | 800 × 600 pixels and 960 × 720 pixels | Pixel-level labeling, bounding boxes, or other crack markers | Object detection and segmentation | Because the dataset is small, it can be easy for the model to overfit the training data, especially if you’re using a complex model |
CrackLS315 [ ] | 315 images | 512 × 512 pixels | Pixel-level segmentation mask or bounding box | Object detection and segmentation | The small size of the dataset may make the model perform poorly in complex scenarios, especially when encountering different types of cracks or uncommon crack features |
Stone331 [ ] | 331 images | 512 × 512 pixels | Pixel-level segmentation mask or bounding box | Object detection and segmentation | The relatively small number of images limits the generalization ability of the model, especially in deep learning tasks where smaller datasets tend to lead to overfitting |
Index | Index Value and Calculation Formula | Curve |
---|---|---|
True positive | - | |
False positive | - | |
True negative | - | |
False negative | - | |
Precision | PRC | |
Recall | PRC, ROC curve | |
F1 score | F1 score curve | |
Accuracy | Accuracy vs. threshold curve | |
Average precision | PRC | |
Mean average precision | - | |
IoU | IoU distribution curve, precision-recall curve with IoU thresholds |
The statements, opinions and data contained in all publications are solely those of the individual author(s) and contributor(s) and not of MDPI and/or the editor(s). MDPI and/or the editor(s) disclaim responsibility for any injury to people or property resulting from any ideas, methods, instructions or products referred to in the content. |
Share and Cite
Yuan, Q.; Shi, Y.; Li, M. A Review of Computer Vision-Based Crack Detection Methods in Civil Infrastructure: Progress and Challenges. Remote Sens. 2024 , 16 , 2910. https://doi.org/10.3390/rs16162910
Yuan Q, Shi Y, Li M. A Review of Computer Vision-Based Crack Detection Methods in Civil Infrastructure: Progress and Challenges. Remote Sensing . 2024; 16(16):2910. https://doi.org/10.3390/rs16162910
Yuan, Qi, Yufeng Shi, and Mingyue Li. 2024. "A Review of Computer Vision-Based Crack Detection Methods in Civil Infrastructure: Progress and Challenges" Remote Sensing 16, no. 16: 2910. https://doi.org/10.3390/rs16162910
Article Metrics
Article access statistics, further information, mdpi initiatives, follow mdpi.
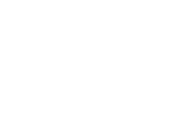
Subscribe to receive issue release notifications and newsletters from MDPI journals

Energy & Environmental Science
Health prognostics for lithium-ion batteries: mechanisms, methods, and prospects.

* Corresponding authors
a Department of Energy, Aalborg University, Aalborg, Denmark
b College of Mechanical and Vehicle Engineering, Chongqing University, Chongqing, China E-mail: [email protected]
c Department of Automotive and Mechatronics Engineering, Ontario Tech University, Oshawa, Canada
Lithium-ion battery aging mechanism analysis and health prognostics are of great significance for a smart battery management system to ensure safe and optimal use of the battery system. This paper provides a comprehensive review of aging mechanisms and the state-of-the-art health prognostic methods and summarizes the main challenges and research prospects for battery health prognostics. First, the complex relationships among aging mechanisms, aging modes, influencing factors, and aging types are reviewed and summarized. Then, the battery health prognostic methods are divided according to different time scales and objectives, which include the short-term state of health estimation, long-term end-of-life prediction, and degradation trajectory prediction, followed by a detailed review of each prognostic task and method. For consistency, we first provide a clear and concise description of each method, showing the similarities and peculiarities of these methods, and then review several representative works. After that, comparative evaluations are conducted. The main advantages and disadvantages of each prognostic task and prognostic method are analyzed in detail. Next, key challenges are presented by considering the specific characteristics of each prognostic task. Moreover, for each challenge, potential solutions are presented and discussed. These proposed potential solutions to the main challenges are beneficial and can be considered by researchers in their further studies. Finally, the future trends of battery health prognostics are discussed, and several new ideas for battery health prognostics are proposed.
Article information

Download Citation
Permissions.

Y. Che, X. Hu, X. Lin, J. Guo and R. Teodorescu, Energy Environ. Sci. , 2023, 16 , 338 DOI: 10.1039/D2EE03019E
This article is licensed under a Creative Commons Attribution-NonCommercial 3.0 Unported Licence . You can use material from this article in other publications, without requesting further permission from the RSC, provided that the correct acknowledgement is given and it is not used for commercial purposes.
To request permission to reproduce material from this article in a commercial publication , please go to the Copyright Clearance Center request page .
If you are an author contributing to an RSC publication, you do not need to request permission provided correct acknowledgement is given.
If you are the author of this article, you do not need to request permission to reproduce figures and diagrams provided correct acknowledgement is given. If you want to reproduce the whole article in a third-party commercial publication (excluding your thesis/dissertation for which permission is not required) please go to the Copyright Clearance Center request page .
Read more about how to correctly acknowledge RSC content .
Social activity
Search articles by author, advertisements.
Research on data analysis method of oscillating water column wave energy converters
- Zhang, Yaqun
- Li, Xianhao
- Sheng, Songwei
- Fan, Zhaohui
- Liu, Jingfeng
Taking the floating oscillating water column central tube wave energy converters (OWC WECs) as the research goal, the Fourier transform (FFT), and the ensemble empirical mode decomposition method (EEMD) were used to statistically analyze the wave height, motion acceleration, velocity and displacement of the device in the physical model test from the perspective of a frequency domain. Through the Fourier transform, the energy spectrum of the incident wave and the motion of the WEC is obtained, and it is found that the peak frequency of the two is the same, that is, the period is 1.138s, but the peak energy of the former is slightly greater than that of the latter. Through the ensemble empirical mode decomposition method, the 1-8 intrinsic mode functions of the incident wave and the displacement of the device are obtained, which shows that the device efficiently captures the dominant frequency wave in the incident wave, that is, the wave height is 0.0628m, the period is 1.1s, and the motion displacement of the vertical direction obtained by the device is 0.0585mm, and the period is 1.1119s. At the same time, it absorbs low-frequency, long-period reflected waves in the pool. The analysis results of the two methods are highly consistent, and the OWC WEC shows good hydrodynamic performance, with efficient wave trapping ability and fast wave responsability. This paper's research methods and results provide a basis for the later optimization of the device and a new idea for the data processing method of the WECs.
Thank you for visiting nature.com. You are using a browser version with limited support for CSS. To obtain the best experience, we recommend you use a more up to date browser (or turn off compatibility mode in Internet Explorer). In the meantime, to ensure continued support, we are displaying the site without styles and JavaScript.
- View all journals
- Explore content
- About the journal
- Publish with us
- Sign up for alerts
- Open access
- Published: 07 August 2024
Highest ocean heat in four centuries places Great Barrier Reef in danger
- Benjamin J. Henley ORCID: orcid.org/0000-0003-3940-1963 1 , 2 , 3 ,
- Helen V. McGregor ORCID: orcid.org/0000-0002-4031-2282 1 , 2 ,
- Andrew D. King ORCID: orcid.org/0000-0001-9006-5745 4 , 5 ,
- Ove Hoegh-Guldberg ORCID: orcid.org/0000-0001-7510-6713 6 ,
- Ariella K. Arzey 1 , 2 ,
- David J. Karoly 4 ,
- Janice M. Lough 7 ,
- Thomas M. DeCarlo ORCID: orcid.org/0000-0003-3269-1320 8 , 9 &
- Braddock K. Linsley ORCID: orcid.org/0000-0003-2085-0662 10
Nature volume 632 , pages 320–326 ( 2024 ) Cite this article
11k Accesses
1 Citations
2751 Altmetric
Metrics details
- Climate change
- Environmental impact
- Palaeoclimate
Mass coral bleaching on the Great Barrier Reef (GBR) in Australia between 2016 and 2024 was driven by high sea surface temperatures (SST) 1 . The likelihood of temperature-induced bleaching is a key determinant for the future threat status of the GBR 2 , but the long-term context of recent temperatures in the region is unclear. Here we show that the January–March Coral Sea heat extremes in 2024, 2017 and 2020 (in order of descending mean SST anomalies) were the warmest in 400 years, exceeding the 95th-percentile uncertainty limit of our reconstructed pre-1900 maximum. The 2016, 2004 and 2022 events were the next warmest, exceeding the 90th-percentile limit. Climate model analysis confirms that human influence on the climate system is responsible for the rapid warming in recent decades. This attribution, together with the recent ocean temperature extremes, post-1900 warming trend and observed mass coral bleaching, shows that the existential threat to the GBR ecosystem from anthropogenic climate change is now realized. Without urgent intervention, the iconic GBR is at risk of experiencing temperatures conducive to near-annual coral bleaching 3 , with negative consequences for biodiversity and ecosystems services. A continuation on the current trajectory would further threaten the ecological function 4 and outstanding universal value 5 of one of Earth’s greatest natural wonders.
Similar content being viewed by others
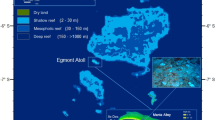
Mesophotic coral bleaching associated with changes in thermocline depth

Atypical weather patterns cause coral bleaching on the Great Barrier Reef, Australia during the 2021–2022 La Niña
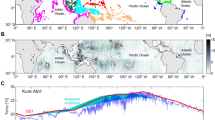
Internal tides can provide thermal refugia that will buffer some coral reefs from future global warming
Like many coral reefs globally, the World Heritage-listed GBR in Australia is under threat 4 , 6 . Mass coral bleaching, declining calcification rates 5 , 7 , outbreaks of crown-of-thorns starfish ( Acanthaster spp.) 8 , severe tropical cyclones 9 and overfishing 10 have placed compounding detrimental pressures on the reef ecosystem. Coral bleaching typically occurs when heat stress triggers the breakdown of the symbiosis between corals and their symbiotic dinoflagellates 11 . Although coral bleaching can occur locally as a result of low salinity, cold waters or pollution, regional and global mass bleaching events, in which the majority of corals in one or more regions bleach at once, are strongly associated with increasing SST linked to global warming 2 .
The first modern observations of mass coral bleaching on the GBR occurred in the 1980s, but these events were less widespread and generally less severe 3 than the bleaching events in the twenty-first century 4 . Stress bands in coral skeletal cores have provided potential evidence for pre-1980s bleaching in the GBR and Coral Sea, such as during the 1877–78 El Niño 12 . However, stress bands are evident in relatively few cores before 1980 (ref. 12 ), suggesting that severe mass bleaching did not occur in the 1800s and most of the 1900s.
As the oceans have warmed, however, mass coral bleaching events have become increasingly lethal to corals 4 . Coral bleaching on the GBR 1 in 1998 coincided with a strong eastern-Pacific El Niño, and in 2002 with a weak El Niño. El Niño events can induce lower cloud cover and increased solar irradiance over the GBR 13 , increasing the risk of thermal stress and mass bleaching events 14 . In 2004, water temperatures were anomalously warm, and although bleaching occurred in the Coral Sea 15 , it was not widespread in the GBR, probably because there was reduced upwelling and an associated reduced influence of nutrients on symbiotic dinoflagellate expulsion 16 .
However, in the nine January–March periods from 2016 to 2024 (inclusive) there were five mass coral bleaching events on the GBR. Each was associated with high SSTs and affected large sections of the reef. GBR mass bleaching occurred in both 2016 and 2017, influenced by the presence of an El Niño event in 2016, and led to the death of at least 50% of shallow-water (depths of 5–10 m) reef-building corals 4 . Major bleaching events occurred again in quick succession in 2020 and 2022, with the accumulated heat stress for large sections of the GBR reaching levels conducive to widespread bleaching but lower levels of coral mortality 1 . The bleaching event in 2022 occurred, unusually, during a La Niña event, which is typically associated with cooler summer SSTs, higher than average rainfall and higher cloud cover on the GBR 1 . At the time of writing, researchers are assessing the impacts of the 2024 mass bleaching event.
The frequency of recent mass coral bleaching and mortality on the GBR is cause for concern. In 2021, the World Heritage Committee of the United Nations Educational, Scientific and Cultural Organization (UNESCO) drafted 17 a decision to inscribe the GBR on the List of World Heritage in Danger, stating that the reef is “facing ascertained danger”, citing recent mass coral bleaching events and insufficient progress by the State Party (Australia) in countering climate change, improving water quality and land management issues. The committee’s adopted decisions 18 have not included inscription of the ‘in danger’ status, but the draft inscription highlights the seriousness of the recent mass coral bleaching events. Authorities in Australia 5 have noted that climate change and coral bleaching have deteriorated the integrity of the outstanding universal value of the GBR, a defining feature of its World Heritage status.
Although rapidly rising SSTs are attributed to human activities with virtual certainty 19 , understanding the multi-century SST history of the GBR is critical to understanding the influence of SST on mass coral bleaching and mortality in recent decades. Putting aside a problematic attempt to do this 20 , which was discredited 21 , 22 , knowledge of the long-term context for GBR SSTs comes primarily from two multi-century reconstructions based on the geochemistry of coral cores collected from the inner shelf 23 and outer shelf 24 (Flinders Reef) in the central GBR. These reconstructions showed that SSTs in the early 2000s were not unusually high relative to levels in the past three centuries, with five-year mean SSTs (and salinities) estimated to be higher in the 1700s than in the 1900s. However, these records were limited by their relatively coarse five-year sampling resolution and their most recent data point being from the early 2000s. After these studies were published, SSTs in the GBR have continued to rise. Updated analysis of coral data from Flinders Reef provides valuable improved temporal resolution 25 , but interpretations of these records remain limited spatially.
Here, we investigate the recent high SST events in the GBR region in the context of the past four centuries. We combine a network of 22 coral Sr/Ca and δ 18 O palaeothermometer series (Supplementary Tables 1 and 2 ) located in and near to the Coral Sea region to infer spatial mean SST anomalies (SSTAs) for January–March, the months when maximum SST and thermal bleaching are most likely to occur in the Coral Sea 16 , 26 , each year from 1618 to 1995 ( Methods and Supplementary Information ). Anthropogenic climate change began and proceeded entirely within the multi-century lives of some of these massive coral colonies, offering a continuous multi-century record covering the industrial era. We use this 1618–1995 reconstruction and the available 1900–2024 instrumental data to contextualize the modern trend and rank four centuries of January–March SSTAs with greater precision than was previously possible. We then assess the degree of human influence on ocean temperatures in the region using climate model simulations run both with and without anthropogenic forcing.
The instrumental period (1900–present)
Mass coral bleaching on the GBR in 2016, 2017, 2020, 2022 and 2024 during January–March coincided with widespread warm SSTAs in the surrounding seas 1 , including the Coral Sea (Fig. 1a–e , using ERSSTv5 data 27 ). The Coral Sea and GBR have experienced a strong warming trend since 1900 (Fig. 1f ). January–March SSTAs averaged over the GBR are strongly correlated ( ρ = 0.84, P ≪ 0.01) with those in the broader Coral Sea (Fig. 1f ), including when the long-term warming trend is removed from both time series ( ρ = 0.69, P < 0.01; Supplementary Fig. 4 ). Based on the strength of this correlation, we associate high January–March area-averaged Coral Sea SSTAs with increased thermal bleaching risk in the GBR.
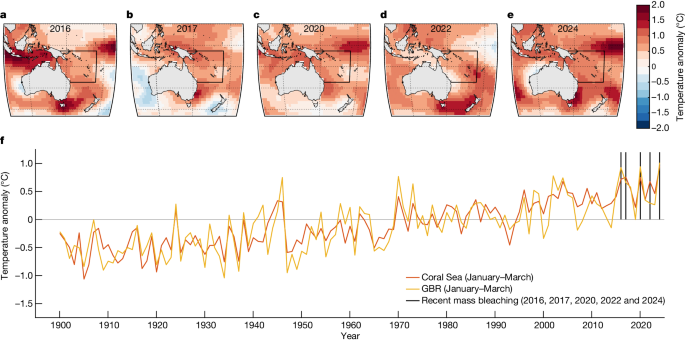
a – e , SSTAs (using ERSSTv5 data) for January–March in the Australasian region relative to the 1961–90 average for the five recent GBR mass coral bleaching years: 2016, 2017, 2020, 2022 and 2024. The black box shows the Coral Sea region (4° S–26° S, 142° E–174° E). f , Coral Sea and GBR mean SSTAs for 1900–2024 in January–March relative to the 1961–90 average. The black vertical lines indicate the five recent GBR mass coral bleaching years.
Record temperatures were set in 2016 and 2017 in the Coral Sea, and in 2020 they peaked fractionally below the record high of 2017. The January–March of 2022 was another warm event, the fifth warmest on record at the time. Recent data (ERSSTv5) indicate that 2024 set a new record by a margin of more than 0.19 °C above the previous record for the region. The January–March mean SSTs averaged over the five mass bleaching years during the period 2016–2024 are 0.77 °C higher than the 1961–90 January–March averages in both the Coral Sea and the GBR. The multidecadal warming trend, extreme years and association between GBR and Coral Sea SSTs are similar for the HadISST 28 gridded SST dataset, with some notable differences in the 1900–40 period (Supplementary Fig. 3 ). Furthermore, analysis of modern temperature-sensitive Sr/Ca series from GBR corals for 1900–2017 provides coherent independent evidence of statistically significant multi-decadal warming trends in January–March SSTs in the central and southern GBR (Supplementary Information section 4.2 ).
A multi-century context (1618–present)
Reconstructing Coral Sea January–March SSTs from 1618 to 1995 extends the century-long instrumental record back in time by an additional three centuries (Fig. 2a and Methods ). The reconstruction (calibrated to ERSSTv5) shows that multi-decadal SST variability was a persistent feature in the past. At the centennial timescale, there is relative stability before 1900, with the exception that cooler temperatures prevailed in the 1600s. Warming during the industrial era has been evident since the early 1900s (Fig. 2a ). There is a warming trend for January–March of 0.09 °C per decade for 1900–2024 and 0.12 °C per decade for 1960–2024 (Fig. 1f ) using ERSSTv5 data. Calibrating our reconstruction to HadISST1.1 yields similar results, with some differences in the degree of pre-1900 variability at both multi-decadal and centennial timescales (Supplementary Information section 5.2.6 ).
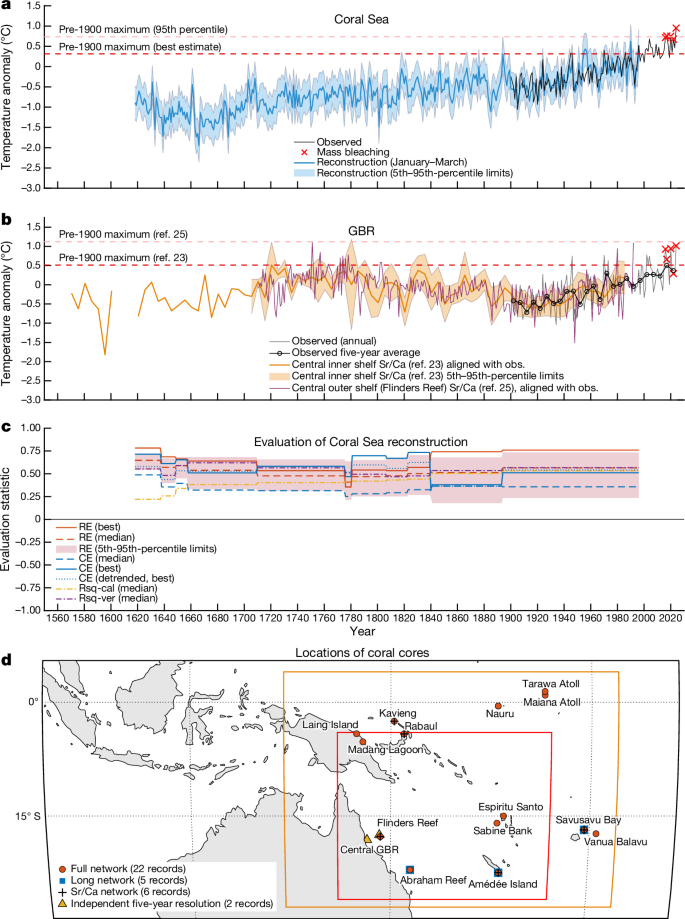
a , Reconstructed and observed mean January–March SSTAs in the Coral Sea for 1618–2024 relative to 1961–90. Dark blue, highest skill (maximum coefficient of efficiency) reconstruction with the full proxy network; light blue, 5th–95th-percentile reconstruction uncertainty; black, observed (ERSSTv5) data. Red crosses indicate the five recent mass bleaching events. Dashed lines indicate the best estimate (highest skill, red) and 95th-percentile (pink) uncertainty bound for the maximum pre-1900 January–March SSTA. b , Central GBR SSTA for the inner shelf 23 in thick orange and outer shelf 25 (Flinders Reef) in thin orange lines; these series are aligned here (see Methods ) with modern observations of mean GBR SSTAs for January–March relative to 1961–90. Observed data are shown at annual (grey line) and five-year (black line with open circles, plotted at the centre of each five-year period and temporally aligned with the five-year coral series 23 ) resolution. Dashed lines indicate best-estimate pre-1900 January–March maxima for refs. 23 (red) and 25 (pink). Orange shading indicates 5th–95th-percentile uncertainty bounds. Red crosses indicate the five recent mass bleaching events. c , Evaluation metrics for the Coral Sea reconstruction (Supplementary Information section 3.1 ); RE, reduction of error; CE, coefficient of efficiency; Rsq-cal, R-squared in the calibration period; Rsq-ver, R-squared in the verification (evaluation) period. d , Coral data locations relative to source data region (orange box) and Coral Sea region (red box). Coral proxy metadata are given in Supplementary Tables 1 and 2 .
Our best-estimate (highest skill; Methods ) annual-resolution Coral Sea reconstruction (Fig. 2a ), using the full coral network calibrated to the ERSSTv5 instrumental data, indicates that the January–March mean SSTAs in 2016, 2017, 2020, 2022 and 2024 were, respectively, 1.50 °C, 1.54 °C, 1.53 °C, 1.46 °C and 1.73 °C above the 1618–1899 (hereafter ‘pre-1900’) reconstructed average. Using the same best-estimate reconstruction, Coral Sea January–March SSTs during these GBR mass bleaching years were five of the six warmest years the region has experienced in the past 400 years (Fig. 2a ).
By comparing the recent warm events to the reconstruction’s uncertainty range ( Methods ), we quantify, using likelihood terminology consistent with recent reports from the Intergovernmental Panel on Climate Change 19 , that the recent heat extremes in 2017, 2020 and 2024 are ‘extremely likely’ (>95th percentile; Fig. 2a ) to be higher than any January–March in the period 1618–1899. Furthermore, the heat extremes in 2016 and 2022 are (at least) ‘very likely’ (>90th percentile) to be above the pre-1900 maximum. We perform a series of tests that verify that our findings are not simply an artefact of the nature of the coral network itself (Supplementary Information section 5.2 ). In a network perturbation test, we generate 22 subsets of the reconstruction by adding proxy records incrementally in order from the highest to the lowest correlation with the target (Supplementary Information section 5.2.5 ). We confirm that 2017, 2020 and 2024 were ‘extremely likely’ (>95th percentile) to have been warmer than any year pre-1900 (using ERSSTv5 data) for all of these proxy subsets. Furthermore, in 20 of the 22 subsets, 2016 was also ‘extremely likely’ (>95th percentile), rather than ‘very likely’, to be warmer (2022 was ‘extremely likely’ in 14 of the 22 subsets). All our additional tests, including a reconstruction with only Sr/Ca coral data (thereby omitting the possibility of any non-temperature signal in δ 18 O coral on the reconstruction), achieve high reconstruction skill and confirm the extraordinary nature of recent extreme temperatures in the multi-century context (Supplementary Information section 5.2 ). Analyses using HadISST1.1 generally show lower correlations with the coral data and reconstructions with slightly warmer regional SSTs before 1900, along with more-muted centennial and multi-decadal variability in the pre-instrumental period. Nevertheless, the HadISST1.1-calibrated reconstructions show that the recent thermal extremes are well above the best estimate (highest skill) of the pre-1900 maximum of reconstructed January–March SSTAs (Supplementary Fig. 42 ). Furthermore, lower SSTAs (in the HadISST1.1 data) relative to the previous three centuries (as in our reconstructions calibrated to HadISST1.1), coupled with the recently observed mass coral bleaching events, could indicate that long-lived corals have a greater sensitivity to warming than is currently recognized.
Reconstructed regional GBR SSTAs based on a five-year-resolution, multi-century coral δ 18 O record from the central inshore GBR 23 (Fig. 2b ) show similarly strong warming since 1900 but more multi-decadal-to-centennial variability than the Coral Sea reconstruction. Recent five-year mean January–March GBR SSTAs narrowly exceed the best estimate of the maximum pre-1900 five-year mean since the early 1600s (Fig. 2b ). The averages for the five-year periods centred on 2018 and 2022 exceed the pre-1900 maximum by 0.11 °C and 0.06 °C, respectively. Results are similar using the five-year-resolution Flinders Reef (central outer shelf) 24 record (Supplementary Fig. 24 ), although its interpretation is limited by the lack of uncertainty estimates available for that record. Our Coral Sea reconstruction incorporates an updated (annual resolution) record from Flinders Reef 25 , which indicates similar centennial trends (thin orange line in Fig. 2b ) and shows that the recent high January–March SSTA events have approached the estimated local pre-1900 maximum SSTA. Although contiguous multi-century cores from within the GBR are limited in their spatial extent, twentieth-century warming is evident in these records.
The extraordinary nature of the recent Coral Sea January–March SSTs in the context of the past 400 years is further illustrated by comparing the ranked temperature anomalies (Fig. 3 ) for the combined reconstructed and instrumental period from 1618–2024, incorporating reconstruction uncertainty ( Methods ). The mass coral bleaching years of 2016, 2017, 2020, 2022 and 2024, and the heat event of 2004, stand out as the warmest events across the whole 407-year record. The warmest three years (2024, 2017 and 2020) exceed the upper uncertainty bound (95th percentile) of the warmest reconstructed January–March in the pre-1900 period (pink (upper) dashed line in Fig. 3 ); 2016, 2004 and 2022 exceed the 90th percentile bound (red (lower) dashed line in Fig. 3 ). The warming trend is clear in the association between the ascending rank of the temperature anomalies and the year (shown as the colour of the filled circles in Fig. 3 ). Despite high interannual variability, 78 of the warmest 100 January–March periods between 1618 and 2024 occurred after 1900, and the 23 warmest all occur after 1900. The warmest 20 January–March periods all occur after 1950, coinciding with accelerated global warming.
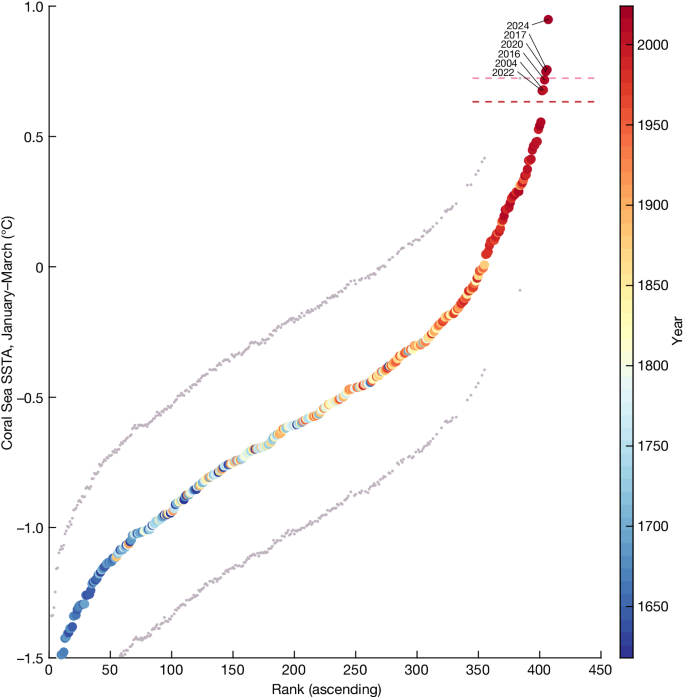
Ranked January–March SSTAs for 1618–2024 relative to 1961–90 (coloured circles) from the best-estimate (highest skill, full coral network) reconstruction (1618–1899) and instrumental (ERSSTv5) data (1900–2024). The year is indicated by the colour of the filled circles. The 5th–95th-percentile uncertainty bounds of the pre-1900 reconstructed SSTAs are shown by small grey dots. The year labels indicate the warmest six years on record, five of which were mass coral bleaching years on the GBR. The pink (upper) dashed line indicates the 95th-percentile uncertainty bound of the maximum pre-1900 reconstructed SSTA; the red (lower) dashed line indicates the 90th-percentile limit.
Assessing anthropogenic influence
Using climate model simulations from the most recent (sixth) phase of the Coupled Model Intercomparison Project 29 (CMIP6), we assess the human influence on January–March SSTAs in the Coral Sea. The model simulations are from two experiments in the Detection and Attribution Model Intercomparison Project (DAMIP) 30 . The first set of simulations represents historical climate conditions, including both the natural and human influences on the climate system over the 1850–2014 period (‘historical’; red in Fig. 4 ). The second experiment is a counterfactual climate that spans the same period and uses the same models but includes only natural influences on the climate, omitting all human influences (‘historical-natural’; blue in Fig. 4 ). The historical experiment includes anthropogenic emissions of greenhouse gases and aerosols, stratospheric ozone changes and anthropogenic land-use changes; the historical-natural experiment does not. Variations in natural climate forcings, such as from volcanic eruptions and solar variability, are incorporated in both experiments. We include models that have a transient climate response (the global mean surface-temperature anomaly at the time of a doubling of atmospheric CO 2 concentration) in the range 1.4–2.2 °C, which is deemed ‘likely’ by the science community 31 ( Methods and Supplementary Information ).
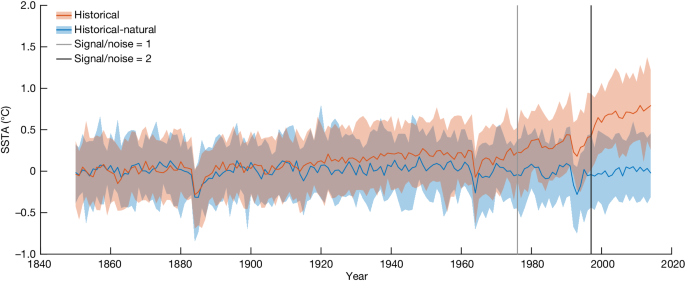
Climate-model simulations of Coral Sea January–March SSTAs relative to the 1850–1900 average for the period 1850–2014, for models within the ‘likely’ range for their transient climate response 31 . The blue line (median) and light blue shading (5th–95th-percentile limits) are from the ‘historical-natural’ climate model simulations (no anthropogenic climate forcing); the red line and light red shading are from the ‘historical’ simulations (anthropogenic influences on the climate included) using the same set of climate models. The climate-model-derived time of emergence of anthropogenic climate change, shown by the grey and black vertical lines (1976 and 1997), is when the ratio of the climate change signal to the standard deviation of noise/variability 32 across model ensemble members first rises above 1 and 2, respectively. All models are represented equally in the model ensemble.
It is only with the incorporation of anthropogenic influences on the climate that the model simulations capture the modern-era warming of the Coral Sea January–March SSTA (Fig. 4 ). The median of the historical simulations has statistically significant warming trends of 0.05 °C, 0.10 °C and 0.15 °C per decade for the periods from 1900, 1950 and 1970 to 2014, respectively; the equivalent historical-natural trends are smaller in magnitude than ±0.01 °C per decade. To further explore the centennial-scale trends, we use a bootstrap ensemble ( Methods ) of the two sets of 165-year simulations from 1850–2014. We found that 100% of the historical bootstrap ensemble has statistically significant positive trends ( Methods ) for 1900–2014, but this value is 0% for the historical-natural ensemble. The observed (ERSSTv5) mean SSTA for 2016–2024 of 0.60 °C relative to 1961–90 is warmer than any nine-year sequence in the 7,095 simulated years in the historical-natural experiments from models with transient climate responses in the ‘likely’ range 31 .
We also use the simulations to estimate the time of emergence of the anthropogenic influence on January–March Coral Sea SSTAs above the natural background variability. The anthropogenic warming signal 32 increases from near zero in 1900 to around 0.5 standard deviations of the variability (‘noise’) in 1960. The climate change signal-to-noise ratio then increases rapidly from 1960 to 2014, exceeding 1.0 in 1976, 2.0 in 1997 and around 2.8 by 2014, the end of these simulations (Fig. 4 , Methods and Supplementary Fig. 50 ). Anthropogenic impacts on the climate are virtually certain to be the primary driver of this long-term warming in the Coral Sea.
Previously, our knowledge of the SST history of the GBR and the Coral Sea region has been highly dependent on instrumental observations, with the exception of the five-year-resolution multi-century coral Sr/Ca and U/Ca SST reconstructions from the two point locations in the central GBR 23 , 24 , an update at one of these locations 25 , seasonal resolution ‘floating’ (in time) chronologies from the GBR in the Holocene 33 , 34 and point SST estimates further back in time 35 . Thus, the context of recent warming trends in the Coral Sea and GBR and their relation to natural variability on decadal to centennial timescales is largely unknown without reconstructions such as the one we developed here.
Our coral proxy network is located mostly beyond the GBR, in the Coral Sea, and some series are located outside the Coral Sea region (Fig. 2d ). The selection of the Coral Sea as a study region allowed for a larger sample of contributing coral proxy data than exists for the GBR. However, coral bleaching on the GBR can be influenced by factors other than large-scale SST, including local oceanic and atmospheric dynamics that can modulate the occurrence and severity of thermal bleaching and mortality events 13 . Nonetheless, warming of seasonal SSTs over the larger Coral Sea region is likely to prime the background state and increase the likelihood of smaller spatio-temporal-scale heat anomalies. Furthermore, where we use only the five-year resolution series directly from the GBR to reconstruct GBR SSTAs, we draw similar conclusions about the long-term trajectory of SSTAs as for our full coral network (Fig. 2b and Supplementary Fig. 24 ). Furthermore, short modern coral series from within the GBR, analysed in this study, document a multi-decadal warming signal that is coherent with instrumental data (Supplementary Figs. 29 and 30 ). Nonetheless, additional high-resolution, multi-century, temperature-sensitive coral geochemical series from within the GBR would help unravel the local and remote ocean–atmosphere contributions to past bleaching events and reduce uncertainties.
The focus on the larger Coral Sea study region also takes advantage of the global modelling efforts of CMIP6. The large number of ensemble members available for CMIP6 means that greater climate model diversity, and therefore greater certainty in our attribution analysis, is possible compared with most single model analyses. There is also a methodological benefit in having high replication of the same experiments run with multiple climate models. However, coarse-resolution global-scale models do not accurately simulate smaller-scale processes, such as inshore currents and mesoscale eddies in the Coral Sea or the Gulf of Carpentaria, which probably affect local surface temperatures and variations in nutrient upwelling in the GBR 36 , 37 . Upwelling on the GBR is linked to the strength of the East Australian Current 16 , the southward branch of the South Pacific subtropical gyre. The CMIP-scale models we use do capture these gyre dynamics. The models show that the East Australian Current is expected to increase in strength as the climate continues to warm through this century 38 , and this may lead to more nutrient inputs that can exacerbate coral sensitivity to rising heat stress 39 , 40 . As well as focusing our model analysis on the larger Coral Sea region, we use a three-month time step. In doing so, we minimize the impact of model spatio-temporal resolution on our inferences about the role of anthropogenic greenhouse-gas emissions on the SST conditions that give rise to GBR mass bleaching.
Remaining uncertainties
We present analyses and interpretations that are as robust as possible given currently available data and methods. However, several sources of remaining uncertainty mean that future reconstructions of past Coral Sea and GBR SSTs could differ from those presented here. Although bias corrections are applied to observational SST datasets such as ERSST and HadISST, these datasets probably retain biases, especially for the period during and before 1945 (ref. 41 ), and these may not be fully accounted for in the uncertainty estimates 42 . Because our reconstructions are calibrated directly to these datasets, future observational-bias corrections are likely to improve proxy-based reconstructions.
Reconstructions of SST that use coral δ 18 O records may be susceptible to the influence of changes in the coral δ 18 O–SST relationship on time periods longer than the instrumental training period, along with non-SST changes in the δ 18 O of seawater, which can covary with salinity. As such, new coral records of temperature-sensitive trace-element ratios such as Sr/Ca, Li/Mg or U/Ca may prove influential in future efforts to distinguish between changes in past temperature and hydroclimate. Owing to the limited availability of multi-century coral data from within the GBR itself, the reconstructed low-frequency variability of GBR SSTs in recent centuries is likely to change as more temperature proxy data become available. It is also likely that new sub-annual resolution records would aid in removing potential signal damping or bias from our use of some annual-resolution records to reconstruct seasonal SSTAs.
Ecological consequences
With global warming of 0.8–1.1 °C above pre-industrial levels 19 there has been a marked increase in mass coral bleaching globally 43 . Even limiting global warming to the Paris Agreement’s ambitious 1.5 °C level would be likely to lead to the loss of 70–90% of corals that are on reefs today 44 . If all current international mitigation commitments are implemented, global mean surface temperature is still estimated to increase in the coming decades, with estimates varying between 1.9 °C (ref. 45 ) and 3.2 °C (ref. 46 ) above pre-industrial levels by the end of this century. Global warming above 2 °C would have disastrous consequences for coral ecosystems 19 , 44 and the hundreds of millions of people who currently depend on them.
Coral reefs of the future, if they can persist, are likely to have a different community structure to those in the recent past, probably one with much less diversity in coral species 4 . This is because mass bleaching events have a differential impact on different coral species. For example, fast-growing branching and tabulate corals are affected more than slower-growing massive species because they have different thermal tolerance 4 . The simplification of reef structures will have adverse impacts on the many thousands of species that rely on the complex three-dimensional structure of reefs 4 . Therefore, even with an ambitious long-term international mitigation goal, the ecological function 4 of the GBR is likely to deteriorate further 5 before it stabilizes.
Coral adaptation and acclimatization may be the only realistic prospect for the conservation of some parts of the GBR this century. However, although adaptation opportunities may be plausible to some extent 47 , they are no panacea because evolutionary changes to fundamental variables such as temperature take decades, if not centuries, to occur, especially in long-lived species such as reef-building corals 48 . There is currently no clear evidence of the real-time evolution of thermally tolerant corals 48 . Most rapid changes depend on a history of exposure to key genetic types and extremes, and there are limitations to genetic adaptation that prevent species-level adaptation to environments outside of their ecological and evolutionary history 19 . Model projections also indicate that rates of coral adaptation are too slow to keep pace with global warming 49 . In a rapidly warming world, the temperature conditions that give rise to mass coral bleaching events are likely to soon become commonplace. So, although we may see some resilience of coral to future marine heat events through acclimatization, thermal refugia are likely to be overwhelmed 50 . Global warming of more than 1.5 °C above pre-industrial levels will probably be catastrophic for coral reefs 44 .
Our new multi-century reconstruction illustrates the exceptional nature of ocean surface warming in the Coral Sea today and the resulting existential risk for the reef-building corals that are the backbone of the GBR. The reconstruction shows that SSTs were relatively cool and stable for hundreds of years, and that recent January–March ocean surface heat in the Coral Sea is unprecedented in at least the past 400 years. The coral colonies and reefs that have lived through the past several centuries, and that yielded the valuable Sr/Ca and δ 18 O data on which our reconstruction is based, are themselves under serious threat. Our analysis of climate-model simulations confirms that human influence is the driver of recent January–March Coral Sea surface warming. Together, the evidence presented in our study indicates that the GBR is in danger. Given this, it is conceivable that UNESCO may in the future reconsider its determination that the iconic GBR is not in danger. In the absence of rapid, coordinated and ambitious global action to combat climate change, we will likely be witness to the demise of one of Earth’s great natural wonders.
Instrumental observations
The Coral Sea and GBR area-averaged monthly SSTAs relative to 1961–90 for January–March are obtained from version 5 of the Extended Reconstructed Sea Surface Temperature dataset (ERSSTv5) 27 . We compare our results using ERSSTv5 with those generated using the Hadley Centre Sea Ice and Sea Surface Temperature dataset (HadISST1.1) 28 . We use only post-1900 instrumental SST observations here. Although gridded datasets have some coverage before 1900, ship-derived temperature data in the region for that period are too sparse to be reliable for calibrating our reconstruction (Supplementary Information section 1.2 ). The regional mean for the GBR is computed using the seven grid-cell locations used by the Australian Bureau of Meteorology (Supplementary Information section 1.1 ). We define the Coral Sea region as the ocean areas inside 4° S–26° S, 142° E–174° E.
Coral-derived temperature proxy data
We use a network of 22 published and publicly available sub-annual and annual resolution temperature-sensitive coral geochemical series (proxies; Fig. 2d , Supplementary Tables 1 and 2 , and Supplementary Fig. 5a–v ) from the western tropical Pacific in our source data region (4° N–27° S, 134° E–184° E) that cover at least the period from 1900 to 1995. Of these 22 series, 16 are δ 18 O, which are in per mil (‰) notation relative to Vienna PeeDee Belemnite (VPDB) 51 ; the remaining six are Sr/Ca series. The coral data are used as predictors in the reconstruction of January–March mean SSTAs in the Coral Sea region. We apply the inverse Rosenblatt transformation 52 , 53 to the coral data to ensure that our reconstruction predictors are normally distributed. Sub-annually resolved series are converted to the annual time step by averaging across the November–April window. This maximizes the detection of the summer peak values, allowing for some inaccuracy in sub-annual dating and the timing of coral skeleton deposition 54 , 55 . A small fraction (less than 0.8%) of missing data is infilled using the regularized expectation maximization (RegEM) algorithm 56 (Supplementary Information section 2.3 ), after which the proxy series are standardized such that each has a mean of zero and a standard deviation of one over their common 1900–1995 period.
Reconstruction method
To produce our Coral Sea reconstruction, we use nested principal component regression 57 (PCR), in which the principal components of the network of 22 coral proxies are used as regressors against the target-region January–March SSTA relative to the 1961–90 average. We perform the reconstructions separately for each nest of proxies, where a nest is a set of proxies that cover the same time period. The longest nest dates back to 1618, when at least two series are available. The nests allow for the use of all coral proxies over the full time period of their coverage. The 96-year portion of the instrumental period (1900–1995) that overlaps with the reconstruction period is used for calibration and evaluation (or equivalently, verification) against observations. We reconstruct regional SSTAs from the principal components of the coral network of δ 18 O and Sr/Ca data, rather than their local SST calibrations, to minimize the number of computational steps and to aid in representing the full reconstruction uncertainty.
Principal component analysis (PCA) is used to reduce the dimensionality of the proxy matrix, as follows. Let P ( t , r ) denote the palaeoclimate-data matrix during the time period t = 1,..., n at an annual time step for proxy series r = 1,..., p . PCA is undertaken on this matrix during the calibration period, P cal . We obtain the principal component coefficients matrix P coeff ( r , e ) for principal components e = 1,..., n PC and principal component scores P score ( t , e ), which are representations of the input matrix P cal in the principal component space. P score is truncated to include n PC,use principal components to form \({P}_{{\rm{score}}}^{{\prime} }\) such that the variance of the proxy network explained by the n PC,use principal components is greater than \({\sigma }_{{\rm{expl}}}^{2}\) (which we set to 95%). Reconstruction tests in which \({\sigma }_{{\rm{expl}}}^{2}\) is varied from 70% to 95% show that our results are not strongly sensitive to this choice, and tests based on lag-one autoregressive noise for \({\sigma }_{{\rm{expl}}}^{2}\) from 50% to 99% further support this choice (Supplementary Information section 3.2 ). These principal components are used as predictors against which the Coral Sea January–March instrumental SSTAs are regressed. We regress the standardized SSTA target data during the calibration period, I cal , against the retained principal components of the predictor data, \({P}_{{\rm{score}}}^{{\prime} }\) :
Thus, we obtain n PC,use estimates of the regression coefficients γ e with gaussian error term ε t ~ N (0, \({\sigma }_{N}^{2}\) ). The principal components are extended back into the pre-instrumental period by multiplying the entire proxy matrix P ( t , p ) with the truncated principal component coefficient matrix \({P}_{{\rm{coeff}}}^{{\prime} }\) ( t , e ) to obtain \({Q}_{{\rm{coeff}}}^{{\prime} }\) :
The reconstruction proceeds with the fitted regression coefficients γ e and extended coefficient matrix \({Q}_{{\rm{coeff}}}^{{\prime} }\) to obtain a reconstruction time series R m ( t ) for a given nest of proxy series
The standardized reconstruction R m ( t ) is then calibrated to the instrumental data such that the standard deviation and mean of the reconstruction and target during the calibration interval are equal. As well as obtaining reconstructions for each nest of available proxies, we compute stitched reconstructions S c ( t ) for each calibration period c , which include at each time step the reconstructed data for the proxy nest with maximum coefficient of efficiency 58 , 59 (Supplementary Information section 3.1 ). This procedure is performed for contiguous calibration intervals between 60 and 80 years duration between 1900 and 1995, with interval width and location increments of two years, reserving the remaining data in the overlapping period for independent evaluation, and for all proxy nests. The reconstruction error is modelled with a lag-one autoregressive process fitted to the residuals. We evaluate the capacity of our reconstruction method to achieve spurious skill from overfitting by performing a test in which we replace the coral data with synthetic noise (Supplementary Information section 3.2i ). We find that reconstructions based on synthetic noise achieve extremely low or zero skill and as more noise principal components are included in the regression, the evaluation metrics indicate declining skill. Our reconstruction and evaluation methods therefore guard against the potential for spurious skill.
Pseudo-proxy reconstructions
Our reconstruction method is further evaluated by using a pseudo-proxy modelling approach based on the Community Earth System Model (CESM) Last Millennium Experiment (LME) 60 , for which there are 13 full-forcing ensemble members covering the period 850–2005. We use the pseudo-proxy reconstructions to evaluate our reconstruction method and coral network in a fully coupled climate-model environment. We form pseudo-proxies by extracting from each LME ensemble member the SST and sea surface salinity (SSS) from the 1.5° × 1.5° grid cell located nearest to our coral data. We then apply proxy system models in the form of linear regression models, basing δ 18 O on both SST and SSS, and Sr/Ca on SST only (Supplementary Information section 3.3 ). We set the spatial and temporal availability of the pseudo-coral network to match that of the coral network. We then apply our PCR reconstruction and evaluation procedure to the pseudo-proxy network, taking advantage of the availability of the modelled Coral Sea SSTA data across the multi-century period of 1618–2005, which allows for the evaluation of the pseudo-proxy reconstruction over this entire time period. We first test our method using a ‘perfect proxy’ approach (with no proxy measurement error) before superimposing synthetic noise on the pseudo-proxy time series, evaluating our methodology at two separate levels of measurement error, quantified by signal-to-noise ratios of 1.0 and 4.0. The evaluation metrics for these tests indicate that our coral network and reconstruction method obtain skilful reconstructions of Coral Sea SSTAs in the climate-model environment (Supplementary Figs. 17b , 18 , 20b , 21 , 22b and 23 ).
Comparison with independent coral datasets
We use two multi-century five-year-resolution coral series from the central GBR 23 , 24 (Fig. 2b and Supplementary Fig. 24 ) and a network of sub-annual and annual resolution modern coral series (dated from 1900 onwards but not covering the full 1900–1995 period) from 44 sites in the GBR (Supplementary Information section 4.2 ) for independent evaluation of coral-derived evidence for warming in the region. We estimate five-year GBR SSTAs (Fig. 2b ) by aligning the post-1900 mean and variance of the proxy and instrumental (ERSSTv5) data.
Reconstruction sensitivity to non-SST influences
Of the 22 available coral series, 16 are records of δ 18 O, a widely used measure of the ratio of the stable isotopes 18 O and 16 O. In the tropical Pacific Ocean, δ 18 O is significantly correlated with SST 61 , 62 , 63 , 64 . Coral δ 18 O is also sensitive to the δ 18 O of seawater 65 , which can reflect advection of different water masses and/or changes in freshwater input, such as from riverine sources or precipitation, which in turn co-vary with SSS. Thus, it is generally considered that the main non-SST contributions to coral δ 18 O are processes that co-vary with SSS 62 , 66 . Our methodology minimizes the influence of non-temperature impacts on the reconstruction by exploiting the contrast in spatial heterogeneity between SST and SSS in January–March (Supplementary Information section 5.1 ). SSS is spatially inhomogeneous in the tropical Pacific 66 , 67 , leading to low coherence in SSS signals across our coral network. By contrast, the strong and coherent SST signal across our coral network locations and the Coral Sea region leads to principal components that are strongly representative of SST variations. This produces a skilful reconstruction of SST, as determined by evaluation against independent observations, and low correlations with SSS across the Coral Sea region (Supplementary Fig. 31 ).
Although the likelihood of non-SST influences on our SST reconstruction is low, we nonetheless test the sensitivity of our reconstruction and its associated interpretations to the possibility of these influences on the coral data. The tests compute the correlations between our best-estimate SSTA reconstruction (highest coefficient of efficiency) and observations of SSS, along with a series of additional reconstructions based on subsets of our coral network. The correlations between our highest coefficient of efficiency January–March Coral Sea SSTA reconstruction and January–March SSS are mapped for the Coral Sea and its neighbouring domain using three instrumental SSS datasets (Supplementary Fig. 31 ). Correlations are not statistically significant over most of the domain. Noting differing spatial correlation patterns between the instrumental SSS datasets 68 , which also cover different time periods (Supplementary Information section 5.1 ), we undertake six sensitivity tests using subsets of the coral network (Supplementary Information section 5.2 ). We use the following combinations of coral series: (1) the full network of 22 δ 18 O and Sr/Ca series (Figs. 2a and 3 ); (2) a subset of the six available Sr/Ca series (Supplementary Figs. 32 – 33 ), to test how the reconstruction is influenced by the inclusion of coral δ 18 O records; (3) a fixed nest subset of the five longest coral series, extending back to at least 1700 (Supplementary Figs. 34 – 35 ), to test for the potential influence of combining series of differing lengths (from our splicing of portions of the best reconstructions from each nest); (4) a subset of the ten coral series that are most strongly correlated with the target (Supplementary Figs. 36 and 37 ), to test how our reconstruction is influenced by the inclusion of coral series that are less strongly correlated with our target; (5) a subset of coral series that excludes the six records that are reported to potentially include biological mediation or non-climatic effects, or have low correlation with the target (Supplementary Figs. 38 and 39 ), to test their influence on the reconstruction; and (6) a network perturbation test comprising 22 separate subsets of proxies, in which proxy records are added incrementally in order of highest to lowest correlation with the target, starting with a single coral series and increasing the number of included proxies to all 22 series in our network (Supplementary Information section 5.2.5 ), to systematically quantify the influence of gradually including more coral datasets on our reconstruction and its interpretations.
The evaluation metrics (Fig. 2c and Supplementary Figs. 32b , 34b , 36b and 38b ) indicate a skilful reconstruction back to 1618 for the reconstructions based on the Full, Sr/Ca only, Long, Best-10 and OmitBioMed networks. These reconstructions explain 82.7%, 80.6%, 77.6%, 79.8% and 80.4% (R-squared values) of the variance in January–March SSTAs, respectively, in the independent evaluation periods (using ERSSTv5b). All coral subsets in the network perturbation test produce skilful reconstructions (Supplementary Fig. 40 ). The highest-skill reconstructions for all subsets in the network perturbation test align with our key interpretations (Supplementary Figs. 41 and 42 ). Together, our sensitivity tests show that the coral network, observational data and reconstruction methodology are a sound basis for reconstructing Coral Sea January–March SSTAs in past centuries and contextualizing recent high-SST events ( Supplementary Information ).
Climate-model attribution ensembles and experiments
The multi-model attribution analysis used here is based on simulations from CMIP6. We analyse simulations from the historical experiment (including natural and anthropogenic influences for 1850–2014) and the historical-natural experiment (natural-only forcings for 1850–2014). We select climate models for which monthly surface temperature is available in at least three historical and historical-natural simulations (Supplementary Table 5 ). All model simulations are interpolated to a common regular 1.5° × 1.5° latitude–longitude grid. January–March SSTAs relative to 1961–90 are calculated for each simulation. The full historical all-forcings ensemble is composed of 14 models with 268 simulations for 1850–2014. The natural-only ensemble is composed of the same 14 models with 95 individual simulations. A subset of climate models in the CMIP6 ensemble are considered by the science community to be ‘too hot’, simulating warming in response to increased atmospheric carbon dioxide concentrations that is larger than that supported by independent evidence 31 . We omit these models from our analysis by including only models with a transient climate response in the ‘likely’ range 31 of 1.4–2.2 °C. Our results are not strongly sensitive to this selection (Supplementary Information section 6.3 ). The ten remaining models yield a total of 25,410 years from 154 historical ensemble members and 7,095 years from 43 historical-natural ensemble members. We weight the models equally in our analysis using bootstrap sampling. We report linear trends based on simple linear regression models fitted with ordinary least squares. The statistical significance of linear trends is assessed using the Spearman’s rank correlation test 69 .
Time of emergence of the anthropogenic impact
We assess the anthropogenic influence on SSTAs in the Coral Sea region by starting with the assumption that any anthropogenic influence on SSTAs in the Coral Sea is indistinguishable from natural variability at the commencement of the model experiments. We measure the impact of anthropogenic influence on the climate in the region using a signal-to-noise approach 32 , 70 . We calculate the anthropogenic ‘signal’ as the mean of the difference between the smoothed (using a 41-year Lowess filter) modelled historical Coral Sea SSTA and the mean smoothed modelled historical-natural SSTA. Our ‘noise’ is the standard deviation of the difference between the modelled historical SSTA and its smoothed time series (Supplementary Information section 6 ).
Methods additionally rely on Supplementary Information and refs. 71 , 72 , 73 , 74 , 75 , 76 , 77 , 78 , 79 , 80 , 81 , 82 , 83 , 84 , 85 , 86 , 87 , 88 , 89 , 90 , 91 , 92 , 93 , 94 , 95 , 96 , 97 , 98 , 99 , 100 , 101 , 102 , 103 , 104 .
Data availability
The ERSSTv5 instrumental SST data are available from the US National Oceanic and Atmospheric Administration at https://psl.noaa.gov/data/gridded/data.noaa.ersst.v5.html . The HadISST1.1 data are available from the UK Met Office at https://www.metoffice.gov.uk/hadobs/hadisst/ . The original coral palaeoclimate data are available at the links provided in Supplementary Table 2 . Land areas for maps are obtained from the Mapping Toolbox v.23.2 in Matlab v.2023b and the Global Self-consistent, Hierarchical, High-resolution Geography (GSHHS) Database at https://www.soest.hawaii.edu/pwessel/gshhg/ through the m_map toolbox by R. Pawlowicz, available at https://www.eoas.ubc.ca/%7Erich/map.html . Prepared data from the coral geochemical series, reconstructions and climate models that support the findings of this study are available at: https://doi.org/10.24433/CO.4883292.v1 .
Code availability
The code that supports the findings of this study is available and can be run at : https://doi.org/10.24433/CO.4883292.v1 .
Australian Institute of Marine Science. Long-Term Monitoring Program. https://www.aims.gov.au/research-topics/monitoring-and-discovery/monitoring-great-barrier-reef/long-term-monitoring-program (2024).
Hughes, T. P. et al. Global warming and recurrent mass bleaching of corals. Nature 543 , 373–377 (2017).
Article ADS CAS PubMed Google Scholar
Hoegh-Guldberg, O. Climate change, coral bleaching and the future of the world’s coral reefs. Mar. Freshw. Res. 50 , 839–866 (1999).
Google Scholar
Hughes, T. P. et al. Global warming transforms coral reef assemblages. Nature 556 , 492–496 (2018).
Great Barrier Reef Marine Park Authority. Great Barrier Reef Outlook Report 2019 (Great Barrier Reef Marine Park Authority, 2019).
Hughes, T. P. et al. Coral reefs in the Anthropocene. Nature 546 , 82–90 (2017).
Davis, K. L., Colefax, A. P., Tucker, J. P., Kelaher, B. P. & Santos, I. R. Global coral reef ecosystems exhibit declining calcification and increasing primary productivity. Commun. Earth Environ. 2 , 105 (2021).
Article ADS Google Scholar
Westcott, D. A. et al. Relative efficacy of three approaches to mitigate Crown-of-Thorns Starfish outbreaks on Australia’s Great Barrier Reef. Sci. Rep. 10 , 12594 (2020).
Article ADS CAS PubMed PubMed Central Google Scholar
Mellin, C. et al. Spatial resilience of the Great Barrier Reef under cumulative disturbance impacts. Glob. Chang. Biol. 25 , 2431–2445 (2019).
Article ADS PubMed Google Scholar
Jackson, J. B. C. et al. Historical overfishing and the recent collapse of coastal ecosystems. Science 293 , 629–637 (2001).
Article CAS PubMed Google Scholar
Hoegh-Guldberg, O. & Smith, G. J. The effect of sudden changes in temperature, light and salinity on the population density and export of zooxanthellae from the reef corals Stylophora pistillata Esper and Seriatopora hystrix Dana. J. Exp. Mar. Biol. Ecol. 129 , 279–303 (1989).
Article Google Scholar
DeCarlo, T. M. et al. Acclimatization of massive reef-building corals to consecutive heatwaves. Proc. Biol. Sci. 286 , 20190235 (2019).
PubMed PubMed Central Google Scholar
McGowan, H. & Theobald, A. ENSO weather and coral bleaching on the Great Barrier Reef, Australia. Geophys. Res. Lett. 44 , 10,601–10,607 (2017).
Zhao, W., Huang, Y., Siems, S. & Manton, M. The role of clouds in coral bleaching events over the Great Barrier Reef. Geophys. Res. Lett. 48 , e2021GL093936 (2021).
Oxley, W. G., Emslie, M., Muir, P. & Thompson, A. Marine Surveys Undertaken in the Lihou Reef National Nature Reserve (Australian Institute of Marine Science, 2004).
DeCarlo, T. M. & Harrison, H. B. An enigmatic decoupling between heat stress and coral bleaching on the Great Barrier Reef. PeerJ 7 , e7473 (2019).
Article PubMed PubMed Central Google Scholar
UNESCO World Heritage Committee. Extended 44th Session of the World Heritage Committee, Fuzhou (China) 16–31 July 2021 . Draft decision 44 COM 7B.90. https://whc.unesco.org/document/188005 (UNESCO, 2021).
UNESCO World Heritage Committee. Extended 45th Session of the World Heritage Committee, Riyadh (Saudi Arabia) 10–25 September 2023 . Decision 45 COM 7B.13. https://whc.unesco.org/document/199654 (UNESCO, 2023).
IPCC. Summary for Policymakers. In Climate Change 2021: The Physical Science Basis. Contribution of Working Group I to the Sixth Assessment Report of the Intergovernmental Panel on Climate Change (eds Masson-Delmotte, V. et al.) (Cambridge Univ. Press, 2021).
Kamenos, N. A. & Hennige, S. J. Reconstructing four centuries of temperature-induced coral bleaching on the Great Barrier Reef. Front. Mar. Sci. 5 , 283 (2018).
Hoegh-Guldberg, O. et al. Commentary: reconstructing four centuries of temperature-induced coral bleaching on the Great Barrier Reef. Front. Mar. Sci. 6 , 86 (2019).
DeCarlo, T. M. Commentary: reconstructing four centuries of temperature-induced coral bleaching on the Great Barrier Reef. Front. Mar. Sci. 7 , 30 (2020).
Hendy, E. J. et al. Abrupt decrease in tropical Pacific sea surface salinity at end of Little Ice Age. Science 295 , 1511–1514 (2002).
Calvo, E. et al. Interdecadal climate variability in the Coral Sea since 1708 A.D. Palaeogeogr. Palaeoclimatol. Palaeoecol. 248 , 190–201 (2007).
Zinke, J. et al. North Flinders Reef (Coral Sea, Australia) Porites sp. corals as a candidate global boundary stratotype section and point for the Anthropocene series. Anthropocene Rev. 10 , 201–224 (2023).
Spady, B. L. et al. Global Coral Bleaching Database (NCEI Accession 0228498) (NOAA National Centers for Environmental Information, 2022); https://www.ncei.noaa.gov/archive/accession/0228498 .
Huang, B. et al. Extended reconstructed sea surface temperature, Version 5 (ERSSTv5): Upgrades, validations, and intercomparisons. J. Clim. 30 , 8179–8205 (2017).
Rayner, N. A. et al. Global analyses of sea surface temperature, sea ice, and night marine air temperature since the late nineteenth century. J. Geophys. Res. Atmos. 108 , 4407 (2003).
Eyring, V. et al. Overview of the Coupled Model Intercomparison Project Phase 6 (CMIP6) experimental design and organization. Geosci. Model Dev. 9 , 1937–1958 (2016).
Gillett, N. P. et al. The Detection and Attribution Model Intercomparison Project (DAMIP v1.0) contribution to CMIP6. Geosci. Model Dev. 9 , 3685–3697 (2016).
Hausfather, Z., Marvel, K., Schmidt, G. A., Nielsen-Gammon, J. W. & Zelinka, M. Climate simulations: recognize the ‘hot model’ problem. Nature 605 , 26–29 (2022).
Hawkins, E. et al. Observed emergence of the climate change signal: from the familiar to the unknown. Geophys. Res. Lett. 47 , e2019GL086259 (2020).
Gagan, M. K. et al. Temperature and surface-ocean water balance of the mid-Holocene tropical western Pacific. Science 279 , 1014–1018 (1998).
Arzey, A. K. et al. Coral skeletal proxy records database for the Great Barrier Reef, Australia. Preprint at Earth Syst. Sci. Data https://doi.org/10.5194/essd-2024-159 (2024).
Brenner, L. D. et al. Coral record of Younger Dryas Chronozone warmth on the Great Barrier Reef. Paleoceanogr. Paleoclimatol. 35 , e2020PA003962 (2020).
Furnas, M. J. & Mitchell, A. W. Nutrient inputs into the central Great Barrier Reef (Australia) from subsurface intrusions of Coral Sea waters: a two-dimensional displacement model. Cont. Shelf Res. 16 , 1127–1148 (1996).
Wolanski, E., Andutta, F., Deleersnijder, E., Li, Y. & Thomas, C. J. The Gulf of Carpentaria heated Torres Strait and the Northern Great Barrier Reef during the 2016 mass coral bleaching event. Estuar. Coast. Shelf Sci. 194 , 172–181 (2017).
Oliver, E. C. J. & Holbrook, N. J. Extending our understanding of South Pacific gyre ‘spin-up’: modeling the East Australian Current in a future climate. J. Geophys. Res. Oceans 119 , 2788–2805 (2014).
DeCarlo, T. M. et al. Nutrient-supplying ocean currents modulate coral bleaching susceptibility. Sci. Adv. 6 , eabc5493 (2020).
Article ADS PubMed PubMed Central Google Scholar
Wiedenmann, J. et al. Nutrient enrichment can increase the susceptibility of reef corals to bleaching. Nat. Clim. Chang. 3 , 160–164 (2013).
Article ADS CAS Google Scholar
Chan, D. & Huybers, P. Correcting observational biases in sea surface temperature observations removes anomalous warmth during World War II. J. Clim. 34 , 4585–4602 (2021).
Chan, D., Kent, E. C., Berry, D. I. & Huybers, P. Correcting datasets leads to more homogeneous early-twentieth-century sea surface warming. Nature 571 , 393–397 (2019).
Hughes, T. P. et al. Spatial and temporal patterns of mass bleaching of corals in the Anthropocene. Science 359 , 80–83 (2018).
Hoegh-Guldberg, O. et al. Chapter 3: Impacts of 1.5°C global warming on natural and human systems. In Global Warming of 1.5°C (eds Masson-Delmotte, V. et al.) (IPCC, 2018).
Meinshausen, M. et al. Realization of Paris Agreement pledges may limit warming just below 2 °C. Nature 604 , 304–309 (2022).
Matthews, H. D. & Wynes, S. Current global efforts are insufficient to limit warming to 1.5 °C. Science 376 , 1404–1409 (2022).
Coles, S. L. et al. Evidence of acclimatization or adaptation in Hawaiian corals to higher ocean temperatures. PeerJ 6 , e5347 (2018).
Hughes, T. P., Baird, A. H., Morrison, T. H. & Torda, G. Principles for coral reef restoration in the anthropocene. One Earth 6 , 656–665 (2023).
Logan, C. A., Dunne, J. P., Ryan, J. S., Baskett, M. L. & Donner, S. D. Quantifying global potential for coral evolutionary response to climate change. Nat. Clim. Chang. 11 , 537–542 (2021).
Dixon, A. M., Forster, P. M., Heron, S. F., Stoner, A. M. K. & Beger, M. Future loss of local-scale thermal refugia in coral reef ecosystems. PLOS Clim. 1 , e0000004 (2022).
Coplen, T. B. Discontinuance of SMOW and PDB. Nature 375 , 285 (1995).
van Albada, S. J. & Robinson, P. A. Transformation of arbitrary distributions to the normal distribution with application to EEG test-retest reliability. J. Neurosci. Methods 161 , 205–211 (2007).
Article PubMed Google Scholar
Emile-Geay, J. & Tingley, M. Inferring climate variability from nonlinear proxies: application to palaeo-ENSO studies. Clim. Past 12 , 31–50 (2016).
Barnes, D. J., Taylor, R. B. & Lough, J. M. On the inclusion of trace materials into massive coral skeletons. Part II: distortions in skeletal records of annual climate cycles due to growth processes. J. Exp. Mar. Biol. Ecol. 194 , 251–275 (1995).
Article CAS Google Scholar
Gagan, M. K., Dunbar, G. B. & Suzuki, A. The effect of skeletal mass accumulation in Porites on coral Sr/Ca and δ 18 O paleothermometry. Paleoceanogr. Paleoclimatol. 27 , PA1203 (2012).
ADS Google Scholar
Schneider, T. Analysis of incomplete climate data: estimation of mean values and covariance matrices and imputation of missing values. J. Clim. 14 , 853–871 (2001).
PAGES 2k Consortium. Consistent multidecadal variability in global temperature reconstructions and simulations over the Common Era. Nat. Geosci. 12 , 643–649 (2019).
Cook, E. R., Briffa, K. R. & Jones, P. D. Spatial regression methods in dendroclimatology: a review and comparison of two techniques. Int. J. Climatol. 14 , 379–402 (1994).
Nash, J. E. & Sutcliffe, J. V. River flow forecasting through conceptual models part I − a discussion of principles. J. Hydrol. 10 , 282–290 (1970).
Otto-Bliesner, B. L. et al. Climate variability and change since 850 CE: an ensemble approach with the Community Earth System Model. Bull. Am. Meteorol. Soc. 97 , 735–754 (2016).
Evans, M. N., Kaplan, A. & Cane, M. A. Optimal sites for coral-based reconstruction of global sea surface temperature. Paleoceanogr. Paleoclimatol. 13 , 502–516 (1998).
Russon, T., Tudhope, A. W., Hegerl, G. C., Collins, M. & Tindall, J. Inter-annual tropical Pacific climate variability in an isotope-enabled CGCM: Implications for interpreting coral stable oxygen isotope records of ENSO. Clim. Past 9 , 1543–1557 (2013).
PAGES Hydro2k Consortium. Comparing proxy and model estimates of hydroclimate variability and change over the Common Era. Clim. Past 13 , 1851–1900 (2017).
Freund, M. B. et al. Higher frequency of Central Pacific El Niño events in recent decades relative to past centuries. Nat. Geosci. 12 , 450–455 (2019).
Gagan, M. K. et al. New views of tropical paleoclimates from corals. Quat. Sci. Rev. 19 , 45–64 (2000).
Thompson, D. M., Ault, T. R., Evans, M. N., Cole, J. E. & Emile-Geay, J. Comparison of observed and simulated tropical climate trends using a forward model of coral δ 18 O. Geophys. Res. Lett. 38 , L14706 (2011).
LeGrande, A. N. & Schmidt, G. A. Global gridded data set of the oxygen isotopic composition in seawater. Geophys. Res. Lett. 33 , L12604 (2006).
Reed, E. V., Thompson, D. M. & Anchukaitis, K. J. Coral-based sea surface salinity reconstructions and the role of observational uncertainties in inferred variability and trends. Paleoceanogr. Paleoclimatol. 37 , e2021PA004371 (2022).
Khaliq, M. N., Ouarda, T. B. M. J., Gachon, P., Sushama, L. & St-Hilaire, A. Identification of hydrological trends in the presence of serial and cross correlations: A review of selected methods and their application to annual flow regimes of Canadian rivers. J. Hydrol. 368 , 117–130 (2009).
Mahlstein, I., Hegerl, G. & Solomon, S. Emerging local warming signals in observational data. Geophys. Res. Lett. 39 , L21711 (2012).
Freeman, E. et al. ICOADS Release 3.0: a major update to the historical marine climate record. Int. J. Climatol. 37 , 2211–2232 (2017).
Huang, B. et al. Uncertainty estimates for sea surface temperature and land surface air temperature in NOAAGlobalTemp version 5. J. Clim. 33 , 1351–1379 (2020).
Druffel, E. R. M. & Griffin, S. Variability of surface ocean radiocarbon and stable isotopes in the southwestern Pacific. J. Geophys. Res. 104 , 23607–23613 (1999).
DeLong, K. L., Quinn, T. M., Taylor, F. W., Lin, K. & Shen, C.-C. Sea surface temperature variability in the southwest tropical Pacific since AD 1649. Nat. Clim. Change 2 , 799–804 (2012).
Quinn, T. et al. A multicentury stable isotope record from a New Caledonia coral: Interannual and decadal SST variability in the southwest Pacific since 1657. Paleoceanography 13 , 412–426 (1998).
Quinn, T. M., Crowley, T. J. & Taylor, F. W. New stable isotope results from a 173-year coral from Espiritu Santo, Vanuatu. Geophys. Res. Lett. 23 , 3413–3416 (1996).
Alibert, C. & Kinsley, L. A 170-year Sr/Ca and Ba/Ca coral record from the western Pacific warm pool: 1. What can we learn from an unusual coral record? J. Geophys. Res. Oceans 113 , C04008 (2008).
Tudhope, A. W. et al. Variability in the El Niño-Southern Oscillation through a glacial-interglacial cycle. Science 291 , 1511–1517 (2001).
Urban, F. E., Cole, J. E. & Overpeck, J. T. Influence of mean climate change on climate variability from a 155-year tropical Pacific coral record. Nature 407 , 989–993 (2000).
Guilderson, T. P. & Schrag, D. P. Reliability of coral isotope records from the western Pacific warm pool: A comparison using age-optimized records. Paleoceanography 14 , 457–464 (1999).
Quinn, T. M., Taylor, F. W. & Crowley, T. J. Coral-based climate variability in the Western Pacific Warm Pool since 1867. J. Geophys. Res. 111 , C11006 (2006).
Gorman, M. K. et al. A coral-based reconstruction of sea surface salinity at Sabine Bank, Vanuatu from 1842 to 2007 CE. Paleoceanography 27 , PA3226 (2012).
Bagnato, S., Linsley, B. K., Howe, S. S., Wellington, G. M. & Salinger, J. Coral oxygen isotope records of interdecadal climate variations in the South Pacific Convergence Zone region. Geochem. Geophys. Geosyst. 6 , Q06001 (2005).
Linsley, B. K. et al. Tracking the extent of the South Pacific Convergence Zone since the early 1600s. Geochem. Geophys. Geosyst. 7 , Q05003 (2006).
Cole, J. E., Fairbanks, R. G. & Shen, G. T. Recent variability in the Southern Oscillation: Isotopic results from a Tarawa Atoll coral. Science 260 , 1790–1793 (1993).
Dassié, E. P. et al. A Fiji multi-coral δ 18 O composite approach to obtaining a more accurate reconstruction of the last two-centuries of the ocean-climate variability in the South Pacific Convergence Zone region. Paleoceanography 29 , 1196–1213 (2014).
Carton, J. A., Chepurin, G. A. & Chen, L. SODA3: A new ocean climate reanalysis. J. Clim. 31 , 6967–6983 (2018).
Zuo, H., Balmaseda, M. A., Tietsche, S., Mogensen, K. & Mayer, M. The ECMWF operational ensemble reanalysis-analysis system for ocean and sea ice: A description of the system and assessment. Ocean Sci. 15 , 779–808 (2019).
Cheng, L. et al. Improved estimates of changes in upper ocean salinity and the hydrological cycle. J. Clim. 33 , 10357–10381 (2020).
Thompson, D. M. et al. Identifying hydro‐sensitive coral δ18O records for improved high‐resolution temperature and salinity reconstructions. Geophys. Res. Lett. 49 , e2021GL096153 (2022).
Wu, Y., Fallon, S. J., Cantin, N. E. & Lough, J. M. Assessing multiproxy approaches (Sr/Ca, U/Ca, Li/Mg, and B/Mg) to reconstruct sea surface temperature from coral skeletons throughout the Great Barrier Reef. Sci. Total Environ. 786 , 147393 (2021).
Sadler, J., Webb, G. E., Leonard, N. D., Nothdurft, L. D. & Clark, T. R. Reef core insights into mid-Holocene water temperatures of the southern Great Barrier Reef. Paleoceanography 31 , 1395–1408 (2016).
Roche, R. C. et al. Mid-Holocene sea surface conditions and riverine influence on the inshore Great Barrier Reef. Holocene 24 , 885–897 (2014).
Reed, E. V., Cole, J. E., Lough, J. M., Thompson, D. & Cantin, N. E. Linking climate variability and growth in coral skeletal records from the Great Barrier Reef. Coral Reefs 38 , 29–43 (2019).
Razak, T. B. et al. Use of skeletal Sr/Ca ratios to determine growth patterns in a branching coral Isopora palifera. Mar. Biol. 164 , 96 (2017).
Marshall, J. F. Decadal-scale, High Resolution Records of Sea Surface Temperature in the Eastern Indian and South Western Pacific Oceans from Proxy Records of the Strontium/calcium Ratio of Massive Porites Corals PhD thesis, Australian National Univ. (2000).
Marshall, J. F. & McCulloch, M. T. An assessment of the Sr/Ca ratio in shallow water hermatypic corals as a proxy for sea surface temperature. Geochim. Cosmochim. Acta 66 , 3263–3280 (2002).
Gagan, M. K. et al. Coral oxygen isotope evidence for recent groundwater fluxes to the Australian Great Barrier Reef. Geophys. Res. Lett. 29 , 43-1–43-4 (2002).
D’Olivo, J. P., Sinclair, D. J., Rankenburg, K. & McCulloch, M. T. A universal multi-trace element calibration for reconstructing sea surface temperatures from long-lived Porites corals: Removing ‘vital-effects’. Geochim. Cosmochim. Acta 239 , 109–135 (2018).
Fallon, S. J., McCulloch, M. T. & Alibert, C. Examining water temperature proxies in Porites corals from the Great Barrier Reef: a cross-shelf comparison. Coral Reefs 22 , 389–404 (2003).
Brenner, L. D., Linsley, B. K. & Potts, D. C. A modern Sr/Ca-δ 18 O-sea surface temperature calibration for Isopora corals on the Great Barrier Reef. Paleoceanography 32 , 182–194 (2017).
Alibert, C. et al. Source of trace element variability in Great Barrier Reef corals affected by the Burdekin flood plumes. Geochim. Cosmochim. Acta 67 , 231–246 (2003).
Murty, S. A. et al. Spatial and temporal robustness of Sr/Ca-SST calibrations in Red Sea corals: Evidence for influence of mean annual temperature on calibration slopes. Paleoceanogr. Paleoclimatol. 33 , 443–456 (2018).
Sayani, H. R., Cobb, K. M., DeLong, K., Hitt, N. T. & Druffel, E. R. M. Intercolony δ 18 O and Sr/Ca variability among Porites spp. corals at Palmyra Atoll: Toward more robust coral-based estimates of climate. Geochem. Geophys. Geosyst. 20 , 5270–5284 (2019).
Otto, F. E. L. Geert Jan van Oldenborgh 1961–2021. Nat. Clim. Chang. 11 , 1017 (2021).
Download references
Acknowledgements
We acknowledge the originators of the coral data cited in Supplementary Tables 1 and 2 ; S. E. Perkins-Kirkpatrick and the deceased G. J. van Oldenborgh 105 for contributions to an earlier version of this manuscript; E. P. Dassié and J. Zinke for discussions and data; R. Neukom for advice on an earlier version of the reconstruction code; and B. Trewin and K. Braganza for advice about the Bureau of Meteorology GBR SST time series. B.J.H. and H.V.M. acknowledge support from an Australian Research Council (ARC) SRIEAS grant, Securing Antarctica’s Environmental Future (SR200100005), and ARC Discovery Project DP200100206. A.D.K. acknowledges support from an ARC DECRA (DE180100638) and the Australian government’s National Environmental Science Program. B.J.H. and A.D.K. acknowledge an affiliation with the ARC Centre of Excellence for Climate Extremes (CE170100023). H.V.M. acknowledges support from an ARC Future Fellowship (FT140100286). A.K.A. acknowledges support from an Australian government research training program scholarship and an AINSE postgraduate research award. Funding was provided to B.K.L. by the Vetlesen Foundation through a gift to the Lamont-Doherty Earth Observatory. Grants to B.K.L. enabled the generation of coral oxygen isotope and Sr/Ca data from Fiji that were used in our reconstruction (US National Science Foundation OCE-0318296 and ATM-9901649 and US National Oceanic and Atmospheric Administration NA96GP0406). We acknowledge the support of the NCI facility in Australia and the World Climate Research Programme’s working group on coupled modelling, which is responsible for CMIP. We thank the climate-modelling groups for producing and making available their model output. For CMIP, the US Department of Energy’s Program for Climate Model Diagnosis and Intercomparison provided coordinating support and led the development of software infrastructure in partnership with the Global Organisation for Earth System Science Portals.
Author information
Authors and affiliations.
Environmental Futures, School of Earth, Atmospheric and Life Sciences, University of Wollongong, Wollongong, New South Wales, Australia
Benjamin J. Henley, Helen V. McGregor & Ariella K. Arzey
Securing Antarctica’s Environmental Future, University of Wollongong, Wollongong, New South Wales, Australia
School of Agriculture, Food and Ecosystem Sciences, University of Melbourne, Parkville, Victoria, Australia
Benjamin J. Henley
School of Geography, Earth and Atmospheric Sciences, University of Melbourne, Parkville, Victoria, Australia
Andrew D. King & David J. Karoly
ARC Centre of Excellence for Climate Extremes, University of Melbourne, Parkville, Victoria, Australia
Andrew D. King
School of the Environment, The University of Queensland, Brisbane, Queensland, Australia
Ove Hoegh-Guldberg
Australian Institute of Marine Science, Townsville, Queensland, Australia
Janice M. Lough
ARC Centre of Excellence for Coral Reef Studies and School of Earth Sciences, University of Western Australia, Crawley, Western Australia, Australia
Thomas M. DeCarlo
Department of Earth and Environmental Sciences, Tulane University, New Orleans, LA, USA
Lamont-Doherty Earth Observatory of Columbia University, Palisades, NY, USA
Braddock K. Linsley
You can also search for this author in PubMed Google Scholar
Contributions
B.J.H., H.V.M. and A.D.K. conceived the study and developed the methodology. B.J.H. did most of the analysis. A.K.A. contributed analysis of modern coral data (Supplementary Information section 4.2 ). T.M.D. contributed analysis of instrumental data coverage (Supplementary Information section 1.2 ). B.K.L. contributed sub-annual coral data. B.J.H. and H.V.M. led the preparation of the manuscript, with contributions from A.D.K., O.H.-G., A.K.A., D.J.K., J.M.L., T.M.D. and B.K.L. Generative artificial intelligence was not used in any aspect of this study or manuscript.
Corresponding author
Correspondence to Benjamin J. Henley .
Ethics declarations
Competing interests.
The authors declare no competing interests.
Peer review
Peer review information.
Nature thanks Simon Michel, Miriam Pfeiffer, Claudia Tebaldi and the other, anonymous, reviewer(s) for their contribution to the peer review of this work.
Additional information
Publisher’s note Springer Nature remains neutral with regard to jurisdictional claims in published maps and institutional affiliations.
Supplementary information
Supplementary information ., rights and permissions.
Open Access This article is licensed under a Creative Commons Attribution 4.0 International License, which permits use, sharing, adaptation, distribution and reproduction in any medium or format, as long as you give appropriate credit to the original author(s) and the source, provide a link to the Creative Commons licence, and indicate if changes were made. The images or other third party material in this article are included in the article’s Creative Commons licence, unless indicated otherwise in a credit line to the material. If material is not included in the article’s Creative Commons licence and your intended use is not permitted by statutory regulation or exceeds the permitted use, you will need to obtain permission directly from the copyright holder. To view a copy of this licence, visit http://creativecommons.org/licenses/by/4.0/ .
Reprints and permissions
About this article
Cite this article.
Henley, B.J., McGregor, H.V., King, A.D. et al. Highest ocean heat in four centuries places Great Barrier Reef in danger. Nature 632 , 320–326 (2024). https://doi.org/10.1038/s41586-024-07672-x
Download citation
Received : 02 November 2022
Accepted : 04 June 2024
Published : 07 August 2024
Issue Date : 08 August 2024
DOI : https://doi.org/10.1038/s41586-024-07672-x
Share this article
Anyone you share the following link with will be able to read this content:
Sorry, a shareable link is not currently available for this article.
Provided by the Springer Nature SharedIt content-sharing initiative
This article is cited by
Great barrier reefâs temperature soars to 400-year high.
- Jeff Tollefson
Nature (2024)
By submitting a comment you agree to abide by our Terms and Community Guidelines . If you find something abusive or that does not comply with our terms or guidelines please flag it as inappropriate.
Quick links
- Explore articles by subject
- Guide to authors
- Editorial policies
Sign up for the Nature Briefing: Anthropocene newsletter — what matters in anthropocene research, free to your inbox weekly.


COMMENTS
Research methods are specific procedures for collecting and analyzing data. Developing your research methods is an integral part of your research design. When planning your methods, there are two key decisions you will make. First, decide how you will collect data. Your methods depend on what type of data you need to answer your research question:
The research methodology is an important section of any research paper or thesis, as it describes the methods and procedures that will be used to conduct the research. It should include details about the research design, data collection methods, data analysis techniques, and any ethical considerations.
Quantitative research methods are used to collect and analyze numerical data. This type of research is useful when the objective is to test a hypothesis, determine cause-and-effect relationships, and measure the prevalence of certain phenomena. Quantitative research methods include surveys, experiments, and secondary data analysis.
Your research methodology discusses and explains the data collection and analysis methods you used in your research. A key part of your thesis, dissertation, or research paper, the methodology chapter explains what you did and how you did it, allowing readers to evaluate the reliability and validity of your research and your dissertation topic.
Choosing an optimal research methodology is crucial for the success of any research project. The methodology you select will determine the type of data you collect, how you collect it, and how you analyse it. Understanding the different types of research methods available along with their strengths and weaknesses, is thus imperative to make an ...
Compare your paper to billions of pages and articles with Scribbr's Turnitin-powered plagiarism checker. Run a free check ... Research methods are specific procedures for collecting and analysing data. Developing your research methods is an integral part of your research design. When planning your methods, there are two key decisions you will ...
I. Groups of Research Methods. There are two main groups of research methods in the social sciences: The empirical-analytical group approaches the study of social sciences in a similar manner that researchers study the natural sciences.This type of research focuses on objective knowledge, research questions that can be answered yes or no, and operational definitions of variables to be measured.
Definition: Research Paper is a written document that presents the author's original research, analysis, and interpretation of a specific topic or issue. It is typically based on Empirical Evidence, and may involve qualitative or quantitative research methods, or a combination of both. The purpose of a research paper is to contribute new ...
Selecting an appropriate research method is a critical decision in the research process. It determines the approach, tools, and techniques that will be used to answer the research questions. ... In the methodology section of a research paper, describe the study's design, data collection, and analysis methods. Detail procedures, tools ...
Other interesting articles. If you want to know more about statistics, methodology, or research bias, make sure to check out some of our other articles with explanations and examples. Statistics. Normal distribution. Skewness. Kurtosis. Degrees of freedom. Variance. Null hypothesis.
Most frequently used methods include: Observation / Participant Observation. Surveys. Interviews. Focus Groups. Experiments. Secondary Data Analysis / Archival Study. Mixed Methods (combination of some of the above) One particular method could be better suited to your research goal than others, because the data you collect from different ...
Writing the Research Paper. Write a detailed outline. Almost the rough content of every paragraph. The order of the various topics in your paper. On the basis of the outline, start writing a part by planning the content, and then write it down. Put a visible mark (which you will later delete) where you need to quote a source, and write in the ...
I. Groups of Research Methods. There are two main groups of research methods in the social sciences: The empirical-analytical group approaches the study of social sciences in a similar manner that researchers study the natural sciences. This type of research focuses on objective knowledge, research questions that can be answered yes or no, and operational definitions of variables to be measured.
These methods are useful when a detailed understanding of a phenomenon is sought. 1. Ethnographic Research. Ethnographic research emerged out of anthropological research, where anthropologists would enter into a setting for a sustained period of time, getting to know a cultural group and taking detailed observations.
The methods section is a fundamental section of any paper since it typically discusses the 'what', 'how', 'which', and 'why' of the study, which is necessary to arrive at the final conclusions. In a research article, the introduction, which serves to set the foundation for comprehending the background and results is usually ...
INTRODUCTION. Scientific research is usually initiated by posing evidenced-based research questions which are then explicitly restated as hypotheses.1,2 The hypotheses provide directions to guide the study, solutions, explanations, and expected results.3,4 Both research questions and hypotheses are essentially formulated based on conventional theories and real-world processes, which allow the ...
4. Use subheadings: Dividing the Methods section in terms of the experiments helps the reader to follow the section better. You may write the specific objective of each experiment as a subheading. Alternatively, if applicable, the name of each experiment can also be used as subheading. 5.
The methods section should describe what was done to answer the research question, describe how it was done, justify the experimental design, and explain how the results were analyzed. Scientific writing is direct and orderly. Therefore, the methods section structure should: describe the materials used in the study, explain how the materials ...
Research methods are different from research methodologies because they are the ways in which you will collect the data for your research project. The best method for your project largely depends on your topic, the type of data you will need, and the people or items from which you will be collecting data. The following boxes below contain a ...
Research papers in the social and natural sciences often follow APA style. This article focuses on reporting quantitative research methods. In your APA methods section, you should report enough information to understand and replicate your study, including detailed information on the sample, measures, and procedures used.
Abstract. This paper aims to provide an overview of the use and assessment of qualitative research methods in the health sciences. Qualitative research can be defined as the study of the nature of phenomena and is especially appropriate for answering questions of why something is (not) observed, assessing complex multi-component interventions ...
Research methods are processes used to collect data. You can use this data to analyze current methods or procedures and to find additional information on a topic. ... You might ask participants to complete tests verbally, on paper or on a computer. Some experiments could involve multiple simulations. For example, a researcher might give a ...
2 Throughout this paper, I will formulate a quantitative research question. Secondly, identify relevant qualitative methodologies and strategies that would support answering my research question. And lastly, explain the importance of targeted data collection to a research plan as a whole. Quantitative Research Question Does the implementation of proper timeout protocols and safety procedures ...
Best Market Research Methods for 2024. Now that you know what you're looking for in a marketing research method, let's dive into the best options. Note: According to HubSpot's 2024 State of Marketing report, understanding customers and their needs is one of the biggest challenges facing marketers today.
Between January and March 2022, crew aboard the International Space Station (ISS) performed the first archaeological fieldwork in space, the Sampling Quadrangle Assemblages Research Experiment (SQuARE). The experiment aimed to: (1) develop a new understanding of how humans adapt to life in an environmental context for which we are not evolutionarily adapted, using evidence from the observation ...
Research design: Research design refers to the overall plan and structure of the study, including the type of study (e.g., observational, experimental), the sampling strategy, and the data collection and analysis methods. Sampling strategy: Sampling strategy refers to the method used to select a representative sample of participants or units ...
A total of 325 documents were searched again and matched with the keywords, and 120 documents were selected for analysis and research. Based on the main research methods of the 120 documents, we classify them into three crack detection methods: fusion of traditional methods and deep learning, multimodal data fusion, and semantic image ...
This paper provides a comprehensive review of aging mechanisms and the state-of-the-art health prognostic methods and summarizes the main challenges and research prospects for battery health prognostics. First, the complex relationships among aging mechanisms, aging modes, influencing factors, and aging types are reviewed and summarized.
The analysis results of the two methods are highly consistent, and the OWC WEC shows good hydrodynamic performance, with efficient wave trapping ability and fast wave responsability. This paper's research methods and results provide a basis for the later optimization of the device and a new idea for the data processing method of the WECs.
Methods 161, 205-211 (2007). ... A.K.A. acknowledges support from an Australian government research training program scholarship and an AINSE postgraduate research award. Funding was provided to ...