- SCIENCE COMMUNICATION LAB
- IBIOLOGY LECTURES
- IBIOLOGY COURSES

Welcome back!
Please login to access your SCL Educator portal:
Forgot your password?
- Experimental Design
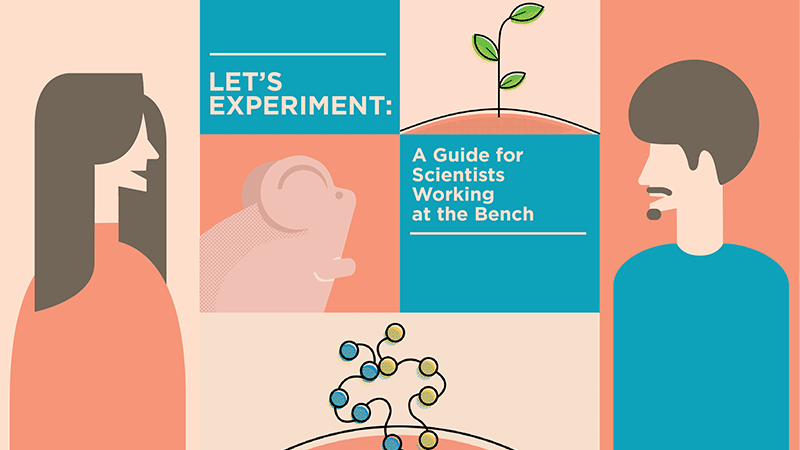
Let’s Experiment
How to Design Experiments in Biological Research
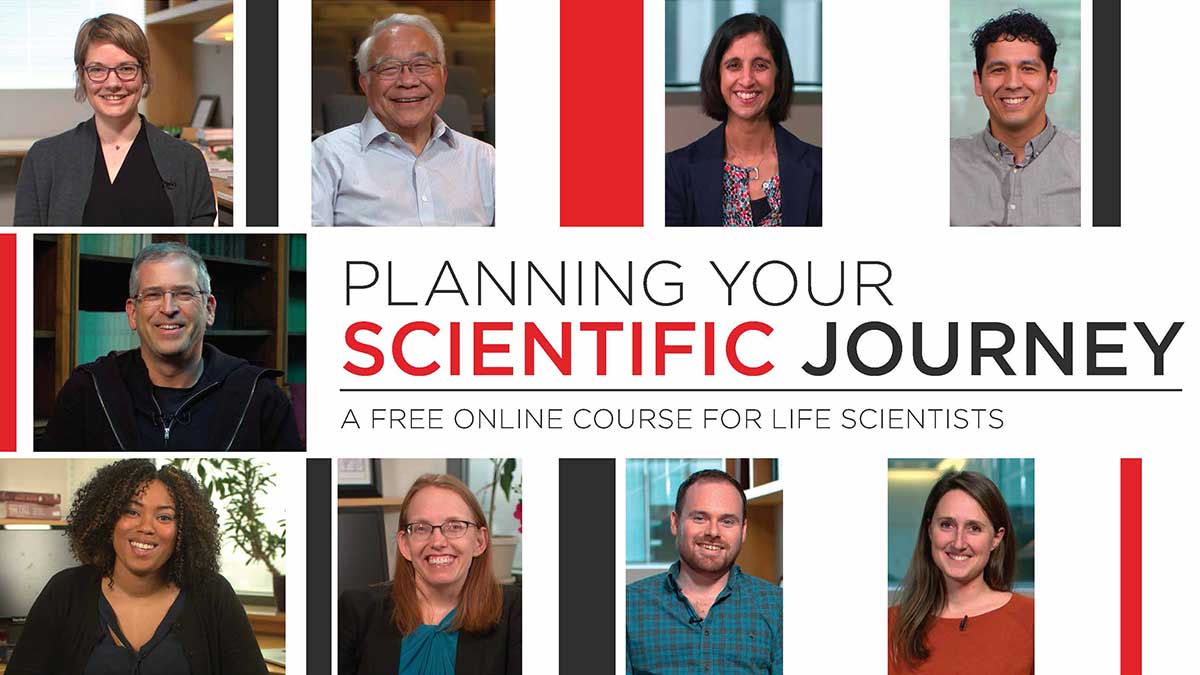
Planning Your Scientific Journey
How to Develop and Plan a Research Project
Sign Up for Updates
Connect with scicommlab, films by topic, award-winning films & videos.
- Career Planning
- Climate Change
- CRISPR + Genetics
- Famous Discoveries
- Health + Medicine
- Microbiology
- Science Communication
- Science Identity
- Science + Society
- With Educator Resources
Short Films
Scientists sharing their stories.
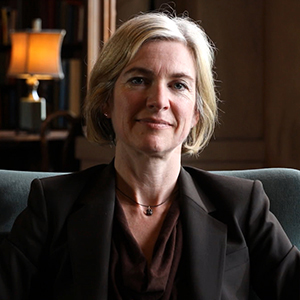
Feature Documentaries
Science on the big screen.
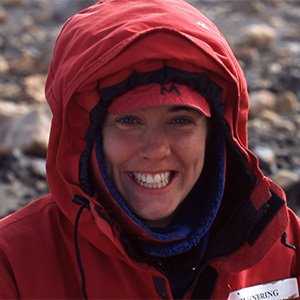
This material is based upon work supported by the National Science Foundation and the National Institute of General Medical Sciences under Grant No. 2122350 and 1 R25 GM139147. Any opinion, finding, conclusion, or recommendation expressed in these videos are solely those of the speakers and do not necessarily represent the views of the Science Communication Lab/iBiology, the National Science Foundation, the National Institutes of Health, or other Science Communication Lab funders.
- © 2024 by the Science Communication Lab (SCICOMMLAB)
- Privacy Policy
- Terms of Use
- Usage Policy
- DEI Statement
- Financial Conflict of Interest Policy
A Nature Research Service
- On-demand Courses
- Design research
Experiments: From Idea to Design
For researchers in the natural sciences who want to develop their experimental design skills
9 experts in experimental design, including experienced researchers and Nature Portfolio journal Editors
8.5 hours of learning
10-30-minute bite-sized lessons
4-module course with certificate
About this course
‘Experiments: From Idea to Design’ equips you with the right tools to help develop, plan and refine robust, impactful experiments. You will cover all the core concepts of experimental design and discover strategies to complete the full process of developing a research motivation, formulating hypotheses, assembling an experimental plan and utilising it.
What you’ll learn
- The benefits of honing your experimental design skills before embarking on full-scale experiments
- How to develop research motivations, identify assumptions and formulate hypotheses
- How to select the precise methods, tools, techniques and protocols you need to answer your research question
- How to refine and make use of your experimental design
Free Sample Foundations of experimental design
6 lessons 1h 30m
Free Sample Developing your motivation, assumptions and hypotheses
6 lessons 2h
Free Sample Assembling your experimental plan
7 lessons 3h
Free Sample Utilising your experimental design
Free sample experiments: from idea to design - sample.
No subscription yet? Try this free sample to preview lessons from the course
3 lessons 1h 30m
Start this module
Developed with expert academics and professionals
This course has been created with an international team of experts with a wide range of experience, including:
- Researchers with backgrounds in various disciplines such as plant biology and synthetic chemistry
- An expert on the theory of the scientific method
- Nature Portfolio journal Editors with extensive experience evaluating new methodologies and protocols
Distinguished Professor of Chemical and Biomolecular Engineering and Senior Vice Provost (Faculty and Institutional Development), National University of Singapore (NUS)
Massimiliano Di Ventra
Professor of Physics, University of California, San Diego
Allison Doerr
Chief Editor, Nature Methods
Oliver Graydon
Chief Editor, Nature Photonics
Ülo Niinemets
Professor of Plant Physiology and Head of the Chair, Estonian University of Life Sciences
Advice from experienced researchers
The course also has additional insights through interviews from:
Junior Fellow, Harvard University
Melanie Clyne
Chief Editor, Nature Protocols
David Lapola
Research Scientist, University of Campinas
Oliver Warr
Assistant Professor of Earth and Environmental Sciences, University of Ottawa
Discover related courses
Managing research data to unlock its full potential.
Learn how to manage your research data with this four-module course.
Interpreting Scientific Results
Explore the best techniques for interpreting your scientific results
Access options
For researchers.
- Register and complete our free course offering , or try a free sample of any of our paid-for courses
- Recommend our courses to your institution, so that we can contact them to discuss becoming a subscriber
For institutions, departments and labs
Find out which of our subscription plans best suits your needs See our subscription plans
Does my institution provide full course access?
When registering, you’ll be asked to select your institution first. If your institution is listed, it has subscribed and provides full access to our on-demand courses catalogue.
My institution isn’t listed!
Select „other“ and register with an individual account. This allows you to access all our free sample course modules, and our entirely free course on peer review. You might also want to recommend our courses to your institution.
I am in charge of purchasing training materials for our lab / department / institution. Buy a subscription
Start this course
Full course access via institutional subscription only. More info
Institutions, departments and labs: Give your research full access to our entire course catalogue
Image Credits
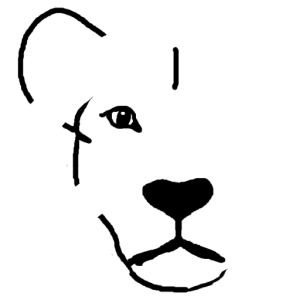
Developed by the Centre for Decision Research and Experimental Economics (CeDEx), University of Nottingham and the Chair of Economic Theory, University of Passau
Easy and fast development
Live web-based interaction.
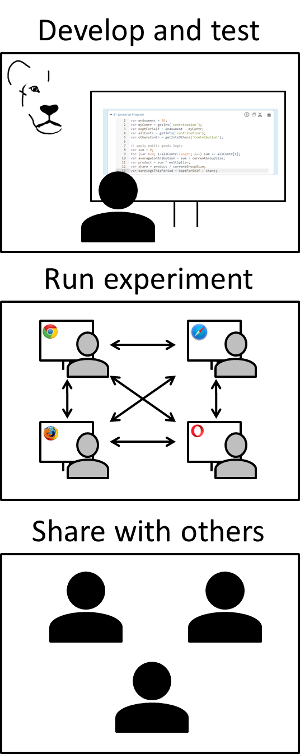
No installation needed
Share your experiments.
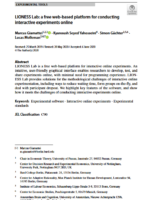
Giamattei, M., Yahosseini, K. S., Gächter, S. and Molleman, L. (2020): LIONESS Lab: a Free Web-Based Platform for Conducting Interactive Experiments Online. Journal of the Economic Science Association. DOI 10.1007/s40881-020-00087-0.
If you use lioness lab, please cite this paper in your publications..
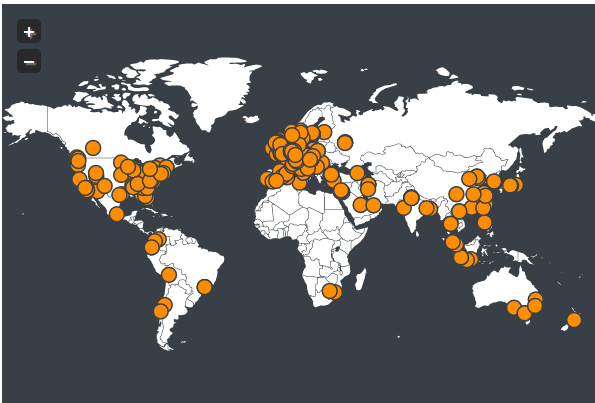

- My Storyboards
Exploring the Art of Experimental Design: A Step-by-Step Guide for Students and Educators
Experimental design for students.
Experimental design is a key method used in subjects like biology, chemistry, physics, psychology, and social sciences. It helps us figure out how different factors affect what we're studying, whether it's plants, chemicals, physical laws, human behavior, or how society works. Basically, it's a way to set up experiments so we can test ideas, see what happens, and make sense of our results. It's super important for students and researchers who want to answer big questions in science and understand the world better. Experimental design skills can be applied in situations ranging from problem solving to data analysis; they are wide reaching and can frequently be applied outside the classroom. The teaching of these skills is a very important part of science education, but is often overlooked when focused on teaching the content. As science educators, we have all seen the benefits practical work has for student engagement and understanding. However, with the time constraints placed on the curriculum, the time needed for students to develop these experimental research design and investigative skills can get squeezed out. Too often they get a ‘recipe’ to follow, which doesn’t allow them to take ownership of their practical work. From a very young age, they start to think about the world around them. They ask questions then use observations and evidence to answer them. Students tend to have intelligent, interesting, and testable questions that they love to ask. As educators, we should be working towards encouraging these questions and in turn, nurturing this natural curiosity in the world around them.
Teaching the design of experiments and letting students develop their own questions and hypotheses takes time. These materials have been created to scaffold and structure the process to allow teachers to focus on improving the key ideas in experimental design. Allowing students to ask their own questions, write their own hypotheses, and plan and carry out their own investigations is a valuable experience for them. This will lead to students having more ownership of their work. When students carry out the experimental method for their own questions, they reflect on how scientists have historically come to understand how the universe works.
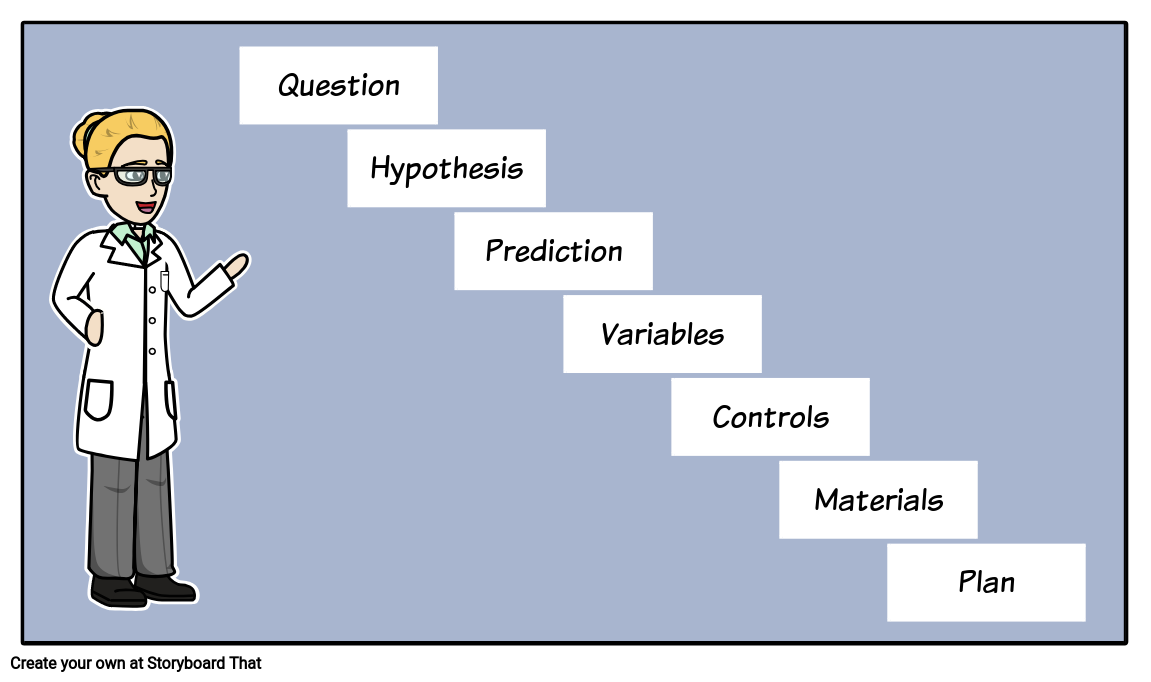
Take a look at the printer-friendly pages and worksheet templates below!
What are the Steps of Experimental Design?
Embarking on the journey of scientific discovery begins with mastering experimental design steps. This foundational process is essential for formulating experiments that yield reliable and insightful results, guiding researchers and students alike through the detailed planning, experimental research design, and execution of their studies. By leveraging an experimental design template, participants can ensure the integrity and validity of their findings. Whether it's through designing a scientific experiment or engaging in experimental design activities, the aim is to foster a deep understanding of the fundamentals: How should experiments be designed? What are the 7 experimental design steps? How can you design your own experiment?
This is an exploration of the seven key experimental method steps, experimental design ideas, and ways to integrate design of experiments. Student projects can benefit greatly from supplemental worksheets and we will also provide resources such as worksheets aimed at teaching experimental design effectively. Let’s dive into the essential stages that underpin the process of designing an experiment, equipping learners with the tools to explore their scientific curiosity.
1. Question
This is a key part of the scientific method and the experimental design process. Students enjoy coming up with questions. Formulating questions is a deep and meaningful activity that can give students ownership over their work. A great way of getting students to think of how to visualize their research question is using a mind map storyboard.
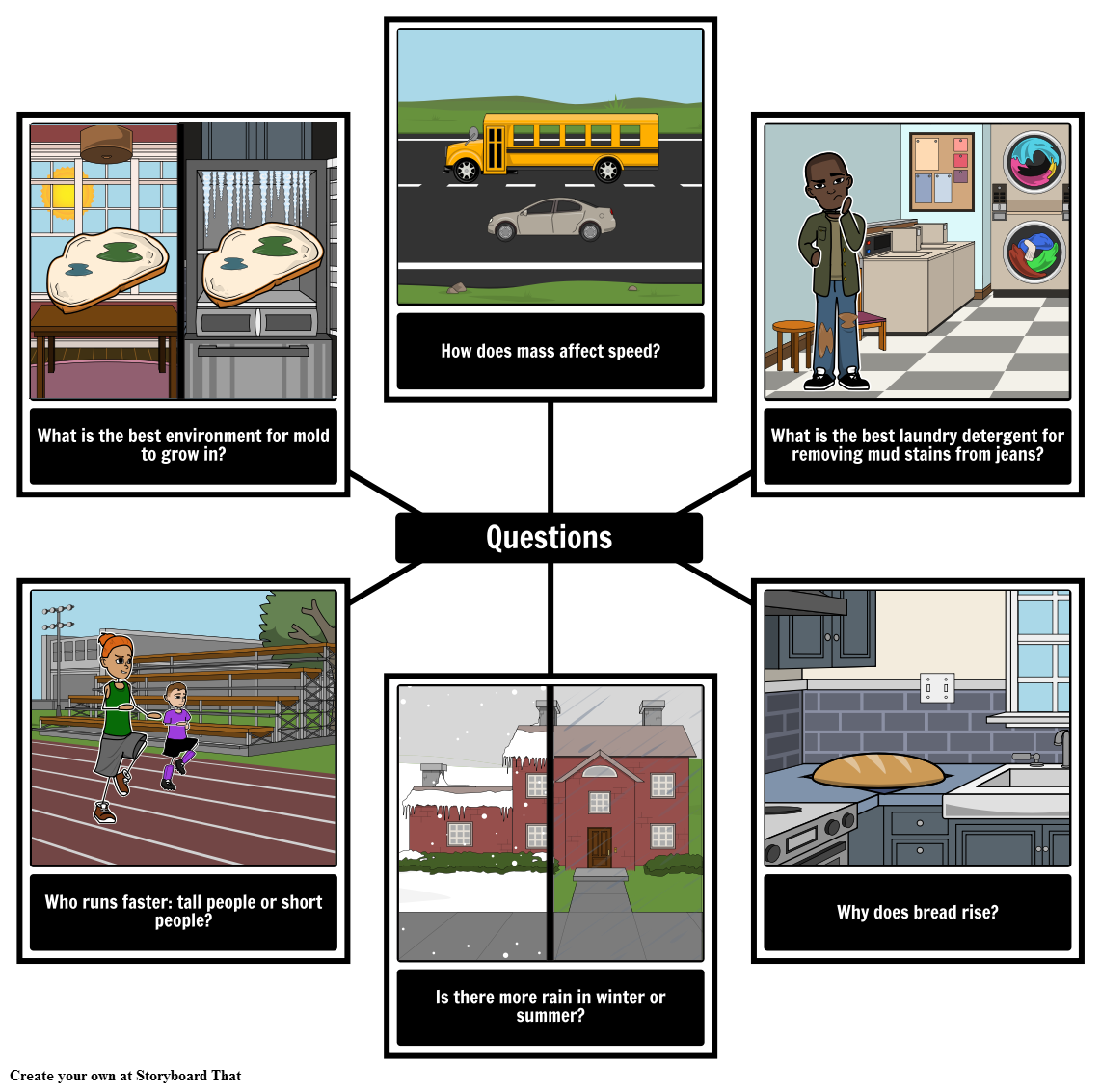
Ask students to think of any questions they want to answer about the universe or get them to think about questions they have about a particular topic. All questions are good questions, but some are easier to test than others.
2. Hypothesis
A hypothesis is known as an educated guess. A hypothesis should be a statement that can be tested scientifically. At the end of the experiment, look back to see whether the conclusion supports the hypothesis or not.
Forming good hypotheses can be challenging for students to grasp. It is important to remember that the hypothesis is not a research question, it is a testable statement . One way of forming a hypothesis is to form it as an “if... then...” statement. This certainly isn't the only or best way to form a hypothesis, but can be a very easy formula for students to use when first starting out.
An “if... then...” statement requires students to identify the variables first, and that may change the order in which they complete the stages of the visual organizer. After identifying the dependent and independent variables, the hypothesis then takes the form if [change in independent variable], then [change in dependent variable].
For example, if an experiment were looking for the effect of caffeine on reaction time, the independent variable would be amount of caffeine and the dependent variable would be reaction time. The “if, then” hypothesis could be: If you increase the amount of caffeine taken, then the reaction time will decrease.
3. Explanation of Hypothesis
What led you to this hypothesis? What is the scientific background behind your hypothesis? Depending on age and ability, students use their prior knowledge to explain why they have chosen their hypotheses, or alternatively, research using books or the internet. This could also be a good time to discuss with students what a reliable source is.
For example, students may reference previous studies showing the alertness effects of caffeine to explain why they hypothesize caffeine intake will reduce reaction time.
4. Prediction
The prediction is slightly different to the hypothesis. A hypothesis is a testable statement, whereas the prediction is more specific to the experiment. In the discovery of the structure of DNA, the hypothesis proposed that DNA has a helical structure. The prediction was that the X-ray diffraction pattern of DNA would be an X shape.
Students should formulate a prediction that is a specific, measurable outcome based on their hypothesis. Rather than just stating "caffeine will decrease reaction time," students could predict that "drinking 2 cans of soda (90mg caffeine) will reduce average reaction time by 50 milliseconds compared to drinking no caffeine."
5. Identification of Variables
Below is an example of a Discussion Storyboard that can be used to get your students talking about variables in experimental design.
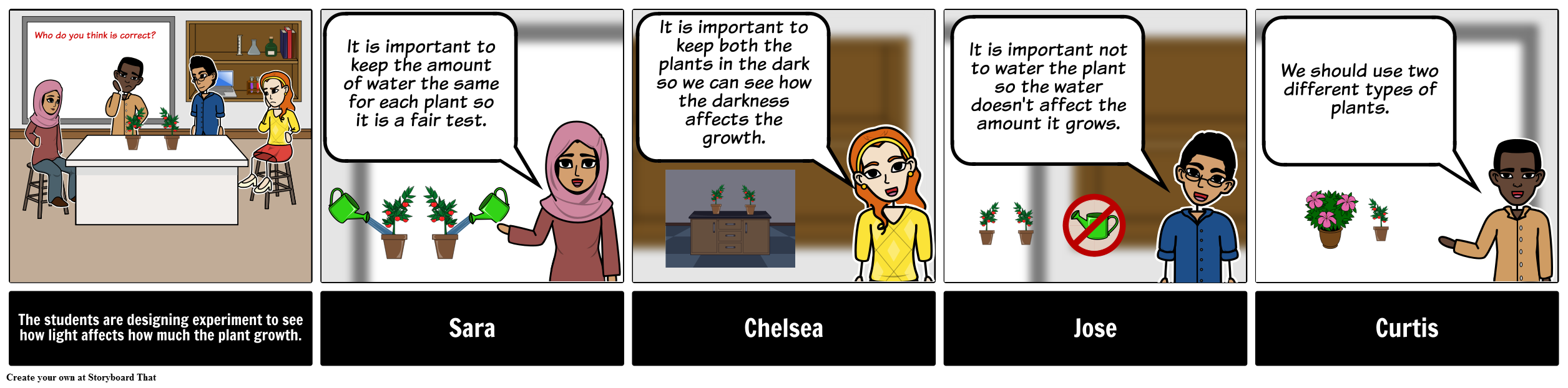
The three types of variables you will need to discuss with your students are dependent, independent, and controlled variables. To keep this simple, refer to these as "what you are going to measure", "what you are going to change", and "what you are going to keep the same". With more advanced students, you should encourage them to use the correct vocabulary.
Dependent variables are what is measured or observed by the scientist. These measurements will often be repeated because repeated measurements makes your data more reliable.
The independent variables are variables that scientists decide to change to see what effect it has on the dependent variable. Only one is chosen because it would be difficult to figure out which variable is causing any change you observe.
Controlled variables are quantities or factors that scientists want to remain the same throughout the experiment. They are controlled to remain constant, so as to not affect the dependent variable. Controlling these allows scientists to see how the independent variable affects the dependent variable within the experimental group.
Use this example below in your lessons, or delete the answers and set it as an activity for students to complete on Storyboard That.
How temperature affects the amount of sugar able to be dissolved in water | |
---|---|
Independent Variable | Water Temperature (Range 5 different samples at 10°C, 20°C, 30°C, 40°C and 50°C) |
Dependent Variable | The amount of sugar that can be dissolved in the water, measured in teaspoons. |
Controlled Variables |
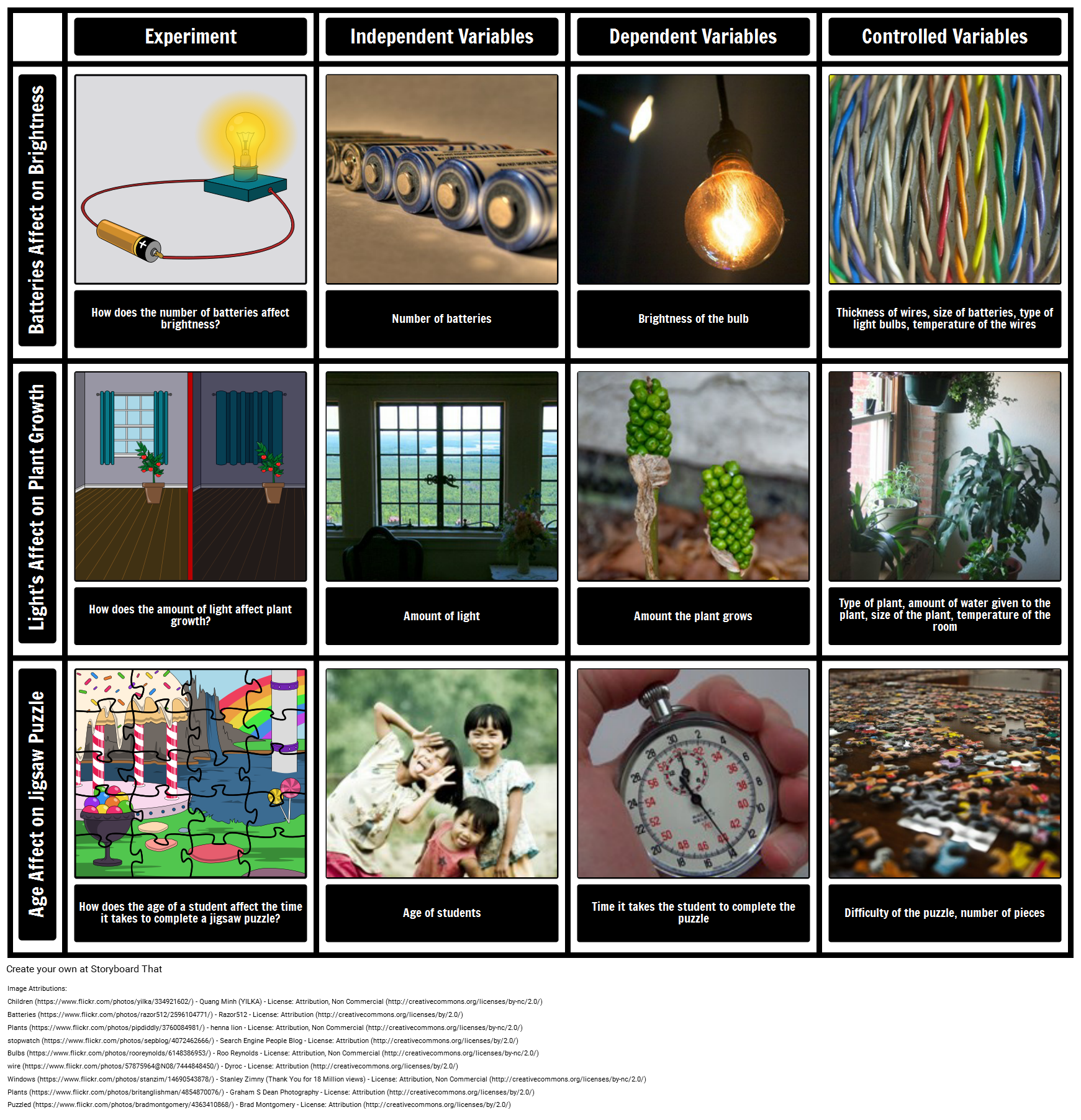
6. Risk Assessment
Ultimately this must be signed off on by a responsible adult, but it is important to get students to think about how they will keep themselves safe. In this part, students should identify potential risks and then explain how they are going to minimize risk. An activity to help students develop these skills is to get them to identify and manage risks in different situations. Using the storyboard below, get students to complete the second column of the T-chart by saying, "What is risk?", then explaining how they could manage that risk. This storyboard could also be projected for a class discussion.
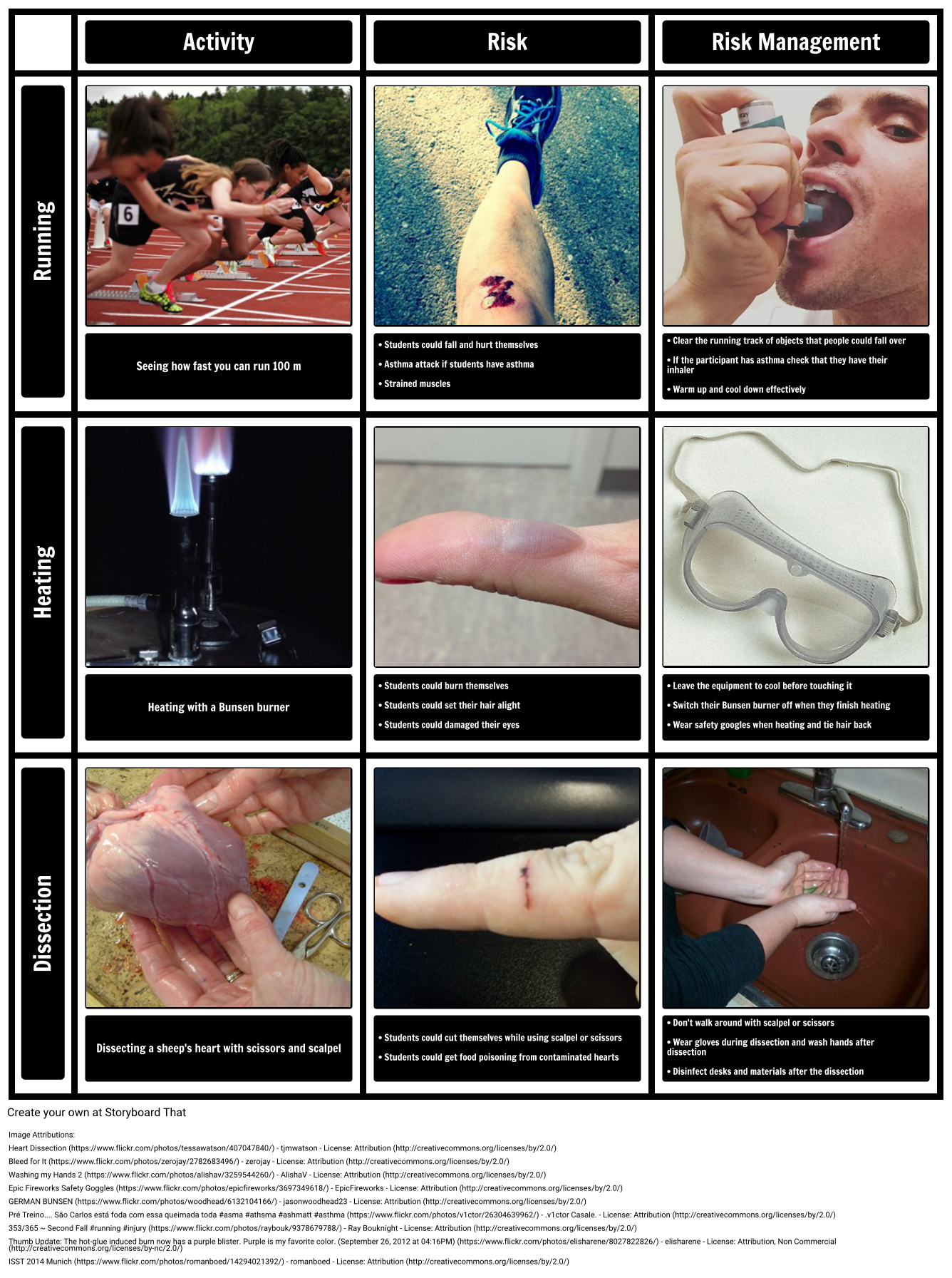
7. Materials
In this section, students will list the materials they need for the experiments, including any safety equipment that they have highlighted as needing in the risk assessment section. This is a great time to talk to students about choosing tools that are suitable for the job. You are going to use a different tool to measure the width of a hair than to measure the width of a football field!
8. General Plan and Diagram
It is important to talk to students about reproducibility. They should write a procedure that would allow their experimental method to be reproduced easily by another scientist. The easiest and most concise way for students to do this is by making a numbered list of instructions. A useful activity here could be getting students to explain how to make a cup of tea or a sandwich. Act out the process, pointing out any steps they’ve missed.
For English Language Learners and students who struggle with written English, students can describe the steps in their experiment visually using Storyboard That.
Not every experiment will need a diagram, but some plans will be greatly improved by including one. Have students focus on producing clear and easy-to-understand diagrams that illustrate the experimental group.
For example, a procedure to test the effect of sunlight on plant growth utilizing completely randomized design could detail:
- Select 10 similar seedlings of the same age and variety
- Prepare 2 identical trays with the same soil mixture
- Place 5 plants in each tray; label one set "sunlight" and one set "shade"
- Position sunlight tray by a south-facing window, and shade tray in a dark closet
- Water both trays with 50 mL water every 2 days
- After 3 weeks, remove plants and measure heights in cm
9. Carry Out Experiment
Once their procedure is approved, students should carefully carry out their planned experiment, following their written instructions. As data is collected, students should organize the raw results in tables, graphs, photos or drawings. This creates clear documentation for analyzing trends.
Some best practices for data collection include:
- Record quantitative data numerically with units
- Note qualitative observations with detailed descriptions
- Capture set up through illustrations or photos
- Write observations of unexpected events
- Identify data outliers and sources of error
For example, in the plant growth experiment, students could record:
Group | Sunlight | Sunlight | Sunlight | Shade | Shade |
---|---|---|---|---|---|
Plant ID | 1 | 2 | 3 | 1 | 2 |
Start Height | 5 cm | 4 cm | 5 cm | 6 cm | 4 cm |
End Height | 18 cm | 17 cm | 19 cm | 9 cm | 8 cm |
They would also describe observations like leaf color change or directional bending visually or in writing.
It is crucial that students practice safe science procedures. Adult supervision is required for experimentation, along with proper risk assessment.
Well-documented data collection allows for deeper analysis after experiment completion to determine whether hypotheses and predictions were supported.
Completed Examples
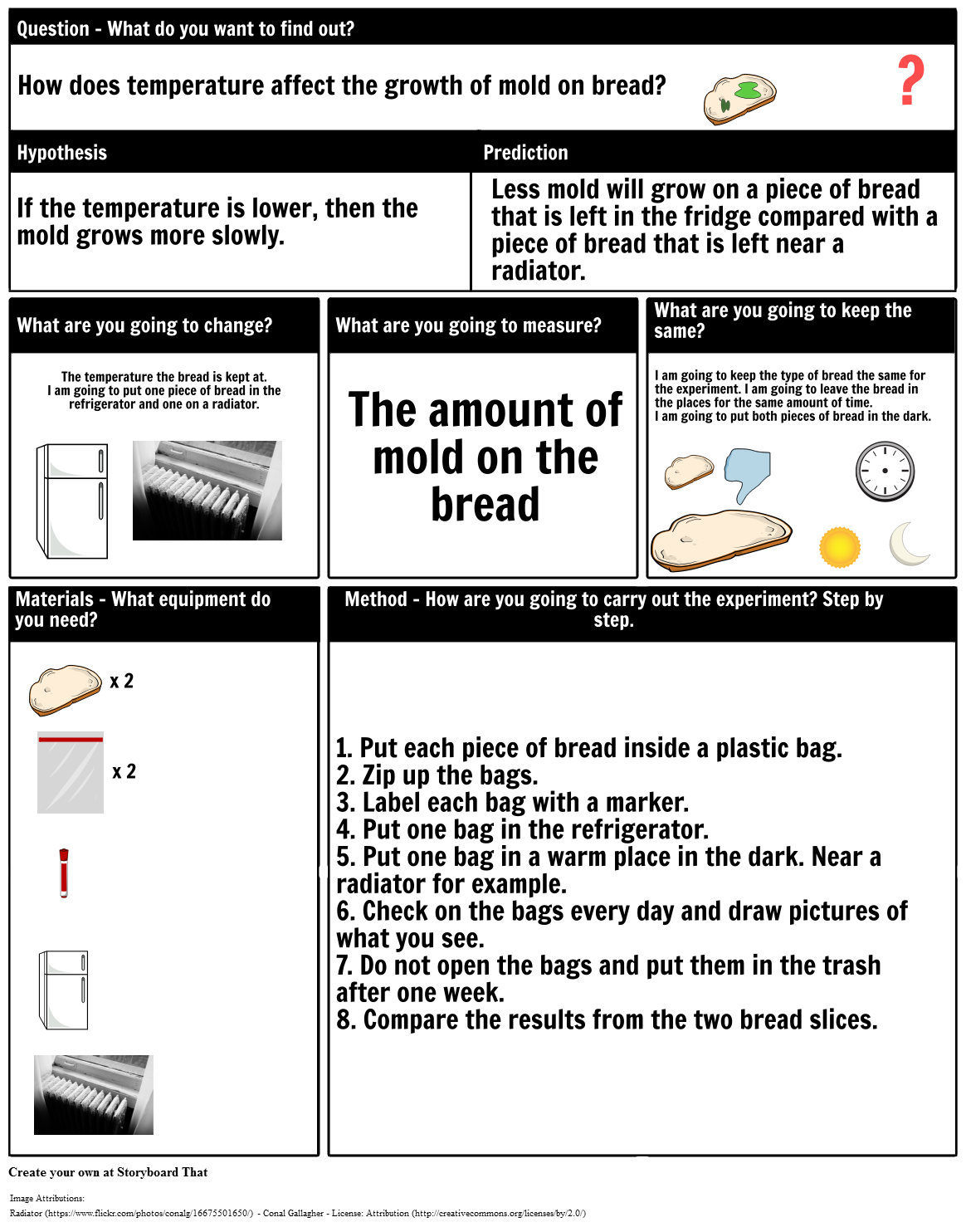
Resources and Experimental Design Examples
Using visual organizers is an effective way to get your students working as scientists in the classroom.
There are many ways to use these investigation planning tools to scaffold and structure students' work while they are working as scientists. Students can complete the planning stage on Storyboard That using the text boxes and diagrams, or you could print them off and have students complete them by hand. Another great way to use them is to project the planning sheet onto an interactive whiteboard and work through how to complete the planning materials as a group. Project it onto a screen and have students write their answers on sticky notes and put their ideas in the correct section of the planning document.
Very young learners can still start to think as scientists! They have loads of questions about the world around them and you can start to make a note of these in a mind map. Sometimes you can even start to ‘investigate’ these questions through play.
The foundation resource is intended for elementary students or students who need more support. It is designed to follow exactly the same process as the higher resources, but made slightly easier. The key difference between the two resources are the details that students are required to think about and the technical vocabulary used. For example, it is important that students identify variables when they are designing their investigations. In the higher version, students not only have to identify the variables, but make other comments, such as how they are going to measure the dependent variable or utilizing completely randomized design. As well as the difference in scaffolding between the two levels of resources, you may want to further differentiate by how the learners are supported by teachers and assistants in the room.
Students could also be encouraged to make their experimental plan easier to understand by using graphics, and this could also be used to support ELLs.
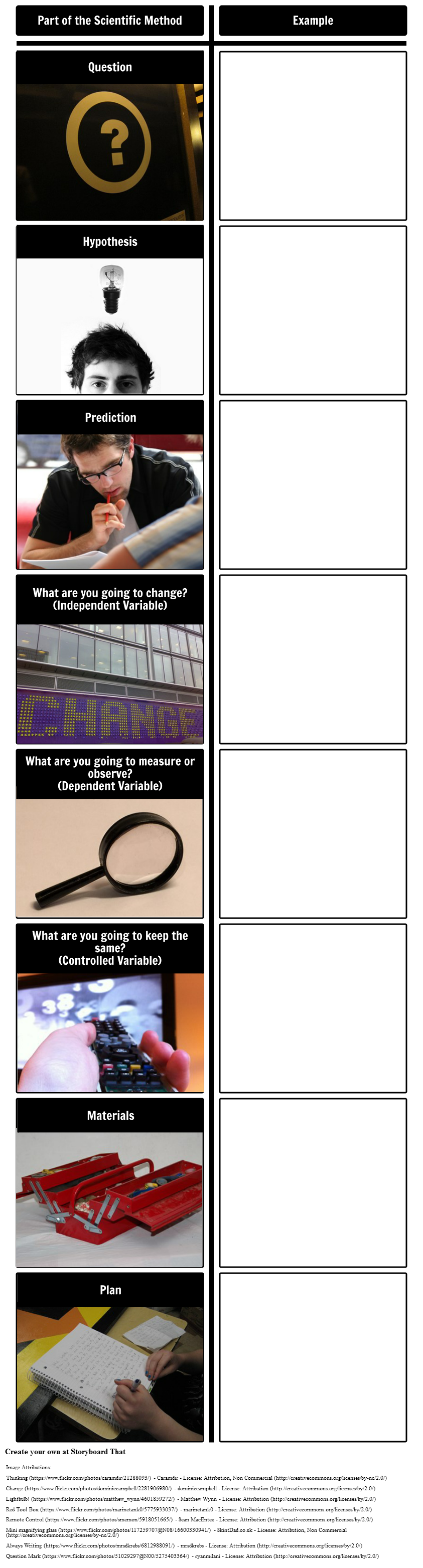
Students need to be assessed on their science inquiry skills alongside the assessment of their knowledge. Not only will that let students focus on developing their skills, but will also allow them to use their assessment information in a way that will help them improve their science skills. Using Quick Rubric , you can create a quick and easy assessment framework and share it with students so they know how to succeed at every stage. As well as providing formative assessment which will drive learning, this can also be used to assess student work at the end of an investigation and set targets for when they next attempt to plan their own investigation. The rubrics have been written in a way to allow students to access them easily. This way they can be shared with students as they are working through the planning process so students know what a good experimental design looks like.
Proficient 13 Points | Emerging 7 Points | Beginning 0 Points | |
---|---|---|---|
Proficient 11 Points | Emerging 5 Points | Beginning 0 Points | |
---|---|---|---|
Printable Resources
Return to top
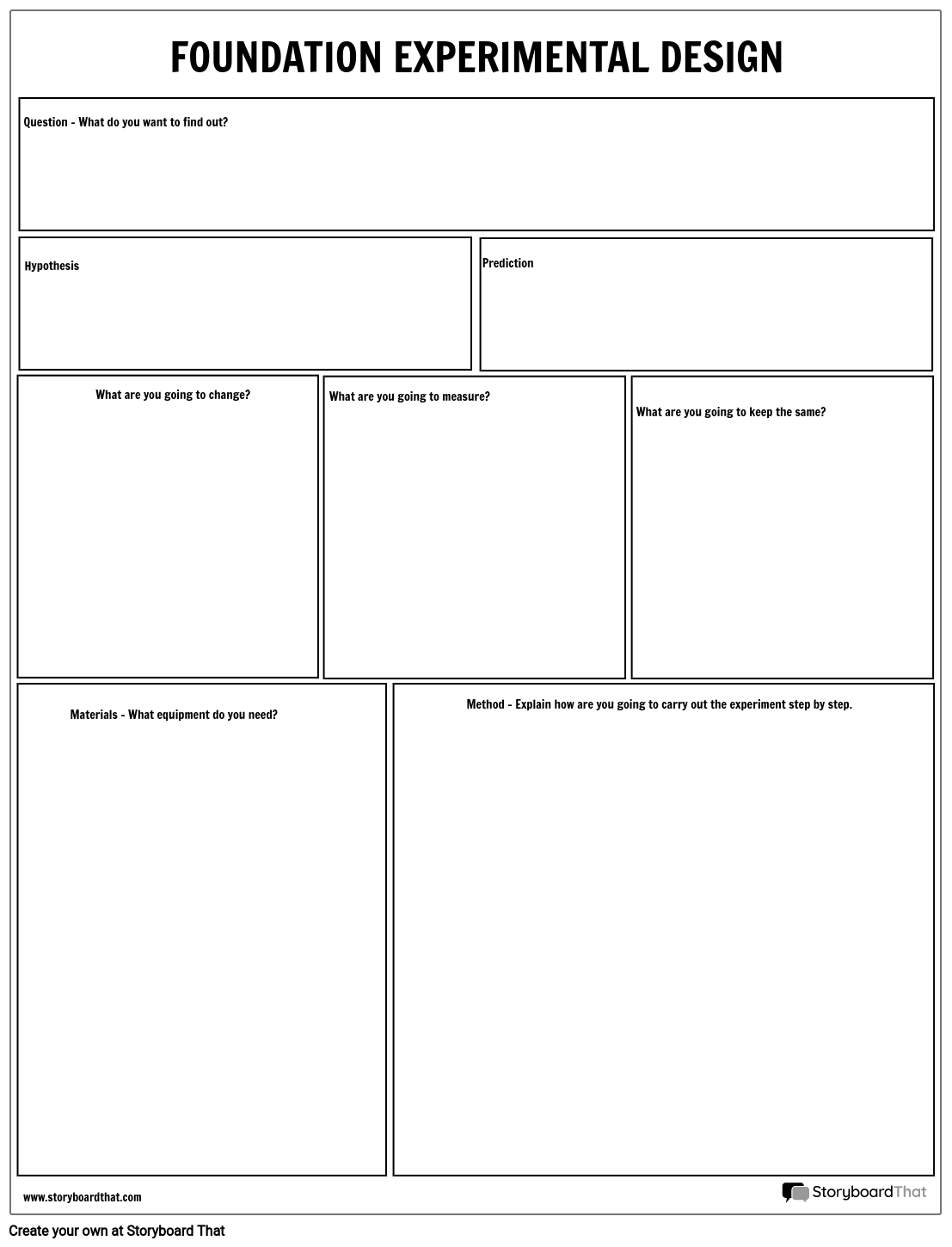
Related Activities
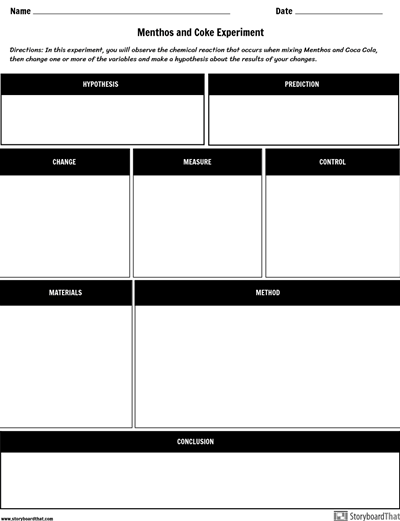
Additional Worksheets
If you're looking to add additional projects or continue to customize worksheets, take a look at several template pages we've compiled for you below. Each worksheet can be copied and tailored to your projects or students! Students can also be encouraged to create their own if they want to try organizing information in an easy to understand way.
- Lab Worksheets
- Discussion Worksheets
- Checklist Worksheets
Related Resources
- Scientific Method Steps
- Science Discussion Storyboards
- Developing Critical Thinking Skills
How to Teach Students the Design of Experiments
Encourage questioning and curiosity.
Foster a culture of inquiry by encouraging students to ask questions about the world around them.
Formulate testable hypotheses
Teach students how to develop hypotheses that can be scientifically tested. Help them understand the difference between a hypothesis and a question.
Provide scientific background
Help students understand the scientific principles and concepts relevant to their hypotheses. Encourage them to draw on prior knowledge or conduct research to support their hypotheses.
Identify variables
Teach students about the three types of variables (dependent, independent, and controlled) and how they relate to experimental design. Emphasize the importance of controlling variables and measuring the dependent variable accurately.
Plan and diagram the experiment
Guide students in developing a clear and reproducible experimental procedure. Encourage them to create a step-by-step plan or use visual diagrams to illustrate the process.
Carry out the experiment and analyze data
Support students as they conduct the experiment according to their plan. Guide them in collecting data in a meaningful and organized manner. Assist them in analyzing the data and drawing conclusions based on their findings.
Frequently Asked Questions about Experimental Design for Students
What are some common experimental design tools and techniques that students can use.
Common experimental design tools and techniques that students can use include random assignment, control groups, blinding, replication, and statistical analysis. Students can also use observational studies, surveys, and experiments with natural or quasi-experimental designs. They can also use data visualization tools to analyze and present their results.
How can experimental design help students develop critical thinking skills?
Experimental design helps students develop critical thinking skills by encouraging them to think systematically and logically about scientific problems. It requires students to analyze data, identify patterns, and draw conclusions based on evidence. It also helps students to develop problem-solving skills by providing opportunities to design and conduct experiments to test hypotheses.
How can experimental design be used to address real-world problems?
Experimental design can be used to address real-world problems by identifying variables that contribute to a particular problem and testing interventions to see if they are effective in addressing the problem. For example, experimental design can be used to test the effectiveness of new medical treatments or to evaluate the impact of social interventions on reducing poverty or improving educational outcomes.
What are some common experimental design pitfalls that students should avoid?
Common experimental design pitfalls that students should avoid include failing to control variables, using biased samples, relying on anecdotal evidence, and failing to measure dependent variables accurately. Students should also be aware of ethical considerations when conducting experiments, such as obtaining informed consent and protecting the privacy of research subjects.
- 353/365 ~ Second Fall #running #injury • Ray Bouknight • License Attribution (http://creativecommons.org/licenses/by/2.0/)
- Always Writing • mrsdkrebs • License Attribution (http://creativecommons.org/licenses/by/2.0/)
- Batteries • Razor512 • License Attribution (http://creativecommons.org/licenses/by/2.0/)
- Bleed for It • zerojay • License Attribution (http://creativecommons.org/licenses/by/2.0/)
- Bulbs • Roo Reynolds • License Attribution, Non Commercial (http://creativecommons.org/licenses/by-nc/2.0/)
- Change • dominiccampbell • License Attribution (http://creativecommons.org/licenses/by/2.0/)
- Children • Quang Minh (YILKA) • License Attribution, Non Commercial (http://creativecommons.org/licenses/by-nc/2.0/)
- Danger • KatJaTo • License Attribution (http://creativecommons.org/licenses/by/2.0/)
- draw • Asja. • License Attribution (http://creativecommons.org/licenses/by/2.0/)
- Epic Fireworks Safety Goggles • EpicFireworks • License Attribution (http://creativecommons.org/licenses/by/2.0/)
- GERMAN BUNSEN • jasonwoodhead23 • License Attribution (http://creativecommons.org/licenses/by/2.0/)
- Heart Dissection • tjmwatson • License Attribution (http://creativecommons.org/licenses/by/2.0/)
- ISST 2014 Munich • romanboed • License Attribution (http://creativecommons.org/licenses/by/2.0/)
- Lightbulb! • Matthew Wynn • License Attribution (http://creativecommons.org/licenses/by/2.0/)
- Mini magnifying glass • SkintDad.co.uk • License Attribution, Non Commercial (http://creativecommons.org/licenses/by-nc/2.0/)
- Plants • henna lion • License Attribution, Non Commercial (http://creativecommons.org/licenses/by-nc/2.0/)
- Plants • Graham S Dean Photography • License Attribution (http://creativecommons.org/licenses/by/2.0/)
- Pré Treino.... São Carlos está foda com essa queimada toda #asma #athsma #ashmatt #asthma • .v1ctor Casale. • License Attribution (http://creativecommons.org/licenses/by/2.0/)
- puzzle • olgaberrios • License Attribution (http://creativecommons.org/licenses/by/2.0/)
- Puzzled • Brad Montgomery • License Attribution (http://creativecommons.org/licenses/by/2.0/)
- Question Mark • ryanmilani • License Attribution (http://creativecommons.org/licenses/by/2.0/)
- Radiator • Conal Gallagher • License Attribution (http://creativecommons.org/licenses/by/2.0/)
- Red Tool Box • marinetank0 • License Attribution (http://creativecommons.org/licenses/by/2.0/)
- Remote Control • Sean MacEntee • License Attribution (http://creativecommons.org/licenses/by/2.0/)
- stopwatch • Search Engine People Blog • License Attribution (http://creativecommons.org/licenses/by/2.0/)
- Thinking • Caramdir • License Attribution, Non Commercial (http://creativecommons.org/licenses/by-nc/2.0/)
- Thumb Update: The hot-glue induced burn now has a purple blister. Purple is my favorite color. (September 26, 2012 at 04:16PM) • elisharene • License Attribution, Non Commercial (http://creativecommons.org/licenses/by-nc/2.0/)
- Washing my Hands 2 • AlishaV • License Attribution (http://creativecommons.org/licenses/by/2.0/)
- Windows • Stanley Zimny (Thank You for 18 Million views) • License Attribution, Non Commercial (http://creativecommons.org/licenses/by-nc/2.0/)
- wire • Dyroc • License Attribution (http://creativecommons.org/licenses/by/2.0/)
Pricing for Schools & Districts
Limited Time
- 5 Teachers for One Year
- 1 Hour of Virtual PD
30 Day Money Back Guarantee • New Customers Only • Full Price After Introductory Offer • Access is for 1 Calendar Year
- 30 Day Money Back Guarantee
- New Customers Only
- Full Price After Introductory Offer
Introductory School Offer
30 Day Money Back Guarantee. New Customers Only. Full Price After Introductory Offer. Access is for 1 Calendar Year
Generating a Quote
This is usually pretty quick :)
Quote Sent!
Email Sent to
Have a language expert improve your writing
Run a free plagiarism check in 10 minutes, generate accurate citations for free.
- Knowledge Base
Methodology
- Guide to Experimental Design | Overview, Steps, & Examples
Guide to Experimental Design | Overview, 5 steps & Examples
Published on December 3, 2019 by Rebecca Bevans . Revised on June 21, 2023.
Experiments are used to study causal relationships . You manipulate one or more independent variables and measure their effect on one or more dependent variables.
Experimental design create a set of procedures to systematically test a hypothesis . A good experimental design requires a strong understanding of the system you are studying.
There are five key steps in designing an experiment:
- Consider your variables and how they are related
- Write a specific, testable hypothesis
- Design experimental treatments to manipulate your independent variable
- Assign subjects to groups, either between-subjects or within-subjects
- Plan how you will measure your dependent variable
For valid conclusions, you also need to select a representative sample and control any extraneous variables that might influence your results. If random assignment of participants to control and treatment groups is impossible, unethical, or highly difficult, consider an observational study instead. This minimizes several types of research bias, particularly sampling bias , survivorship bias , and attrition bias as time passes.
Table of contents
Step 1: define your variables, step 2: write your hypothesis, step 3: design your experimental treatments, step 4: assign your subjects to treatment groups, step 5: measure your dependent variable, other interesting articles, frequently asked questions about experiments.
You should begin with a specific research question . We will work with two research question examples, one from health sciences and one from ecology:
To translate your research question into an experimental hypothesis, you need to define the main variables and make predictions about how they are related.
Start by simply listing the independent and dependent variables .
Research question | Independent variable | Dependent variable |
---|---|---|
Phone use and sleep | Minutes of phone use before sleep | Hours of sleep per night |
Temperature and soil respiration | Air temperature just above the soil surface | CO2 respired from soil |
Then you need to think about possible extraneous and confounding variables and consider how you might control them in your experiment.
Extraneous variable | How to control | |
---|---|---|
Phone use and sleep | in sleep patterns among individuals. | measure the average difference between sleep with phone use and sleep without phone use rather than the average amount of sleep per treatment group. |
Temperature and soil respiration | also affects respiration, and moisture can decrease with increasing temperature. | monitor soil moisture and add water to make sure that soil moisture is consistent across all treatment plots. |
Finally, you can put these variables together into a diagram. Use arrows to show the possible relationships between variables and include signs to show the expected direction of the relationships.
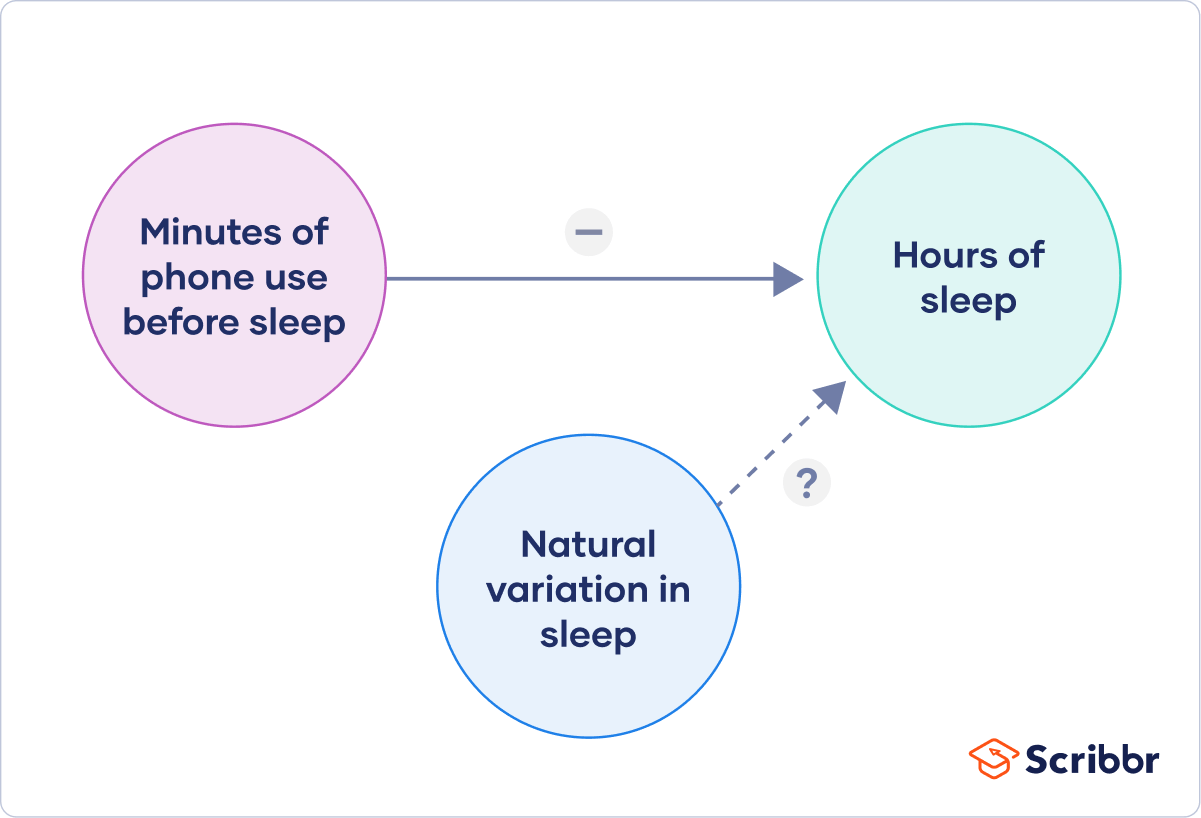
Here we predict that increasing temperature will increase soil respiration and decrease soil moisture, while decreasing soil moisture will lead to decreased soil respiration.
Here's why students love Scribbr's proofreading services
Discover proofreading & editing
Now that you have a strong conceptual understanding of the system you are studying, you should be able to write a specific, testable hypothesis that addresses your research question.
Null hypothesis (H ) | Alternate hypothesis (H ) | |
---|---|---|
Phone use and sleep | Phone use before sleep does not correlate with the amount of sleep a person gets. | Increasing phone use before sleep leads to a decrease in sleep. |
Temperature and soil respiration | Air temperature does not correlate with soil respiration. | Increased air temperature leads to increased soil respiration. |
The next steps will describe how to design a controlled experiment . In a controlled experiment, you must be able to:
- Systematically and precisely manipulate the independent variable(s).
- Precisely measure the dependent variable(s).
- Control any potential confounding variables.
If your study system doesn’t match these criteria, there are other types of research you can use to answer your research question.
How you manipulate the independent variable can affect the experiment’s external validity – that is, the extent to which the results can be generalized and applied to the broader world.
First, you may need to decide how widely to vary your independent variable.
- just slightly above the natural range for your study region.
- over a wider range of temperatures to mimic future warming.
- over an extreme range that is beyond any possible natural variation.
Second, you may need to choose how finely to vary your independent variable. Sometimes this choice is made for you by your experimental system, but often you will need to decide, and this will affect how much you can infer from your results.
- a categorical variable : either as binary (yes/no) or as levels of a factor (no phone use, low phone use, high phone use).
- a continuous variable (minutes of phone use measured every night).
How you apply your experimental treatments to your test subjects is crucial for obtaining valid and reliable results.
First, you need to consider the study size : how many individuals will be included in the experiment? In general, the more subjects you include, the greater your experiment’s statistical power , which determines how much confidence you can have in your results.
Then you need to randomly assign your subjects to treatment groups . Each group receives a different level of the treatment (e.g. no phone use, low phone use, high phone use).
You should also include a control group , which receives no treatment. The control group tells us what would have happened to your test subjects without any experimental intervention.
When assigning your subjects to groups, there are two main choices you need to make:
- A completely randomized design vs a randomized block design .
- A between-subjects design vs a within-subjects design .
Randomization
An experiment can be completely randomized or randomized within blocks (aka strata):
- In a completely randomized design , every subject is assigned to a treatment group at random.
- In a randomized block design (aka stratified random design), subjects are first grouped according to a characteristic they share, and then randomly assigned to treatments within those groups.
Completely randomized design | Randomized block design | |
---|---|---|
Phone use and sleep | Subjects are all randomly assigned a level of phone use using a random number generator. | Subjects are first grouped by age, and then phone use treatments are randomly assigned within these groups. |
Temperature and soil respiration | Warming treatments are assigned to soil plots at random by using a number generator to generate map coordinates within the study area. | Soils are first grouped by average rainfall, and then treatment plots are randomly assigned within these groups. |
Sometimes randomization isn’t practical or ethical , so researchers create partially-random or even non-random designs. An experimental design where treatments aren’t randomly assigned is called a quasi-experimental design .
Between-subjects vs. within-subjects
In a between-subjects design (also known as an independent measures design or classic ANOVA design), individuals receive only one of the possible levels of an experimental treatment.
In medical or social research, you might also use matched pairs within your between-subjects design to make sure that each treatment group contains the same variety of test subjects in the same proportions.
In a within-subjects design (also known as a repeated measures design), every individual receives each of the experimental treatments consecutively, and their responses to each treatment are measured.
Within-subjects or repeated measures can also refer to an experimental design where an effect emerges over time, and individual responses are measured over time in order to measure this effect as it emerges.
Counterbalancing (randomizing or reversing the order of treatments among subjects) is often used in within-subjects designs to ensure that the order of treatment application doesn’t influence the results of the experiment.
Between-subjects (independent measures) design | Within-subjects (repeated measures) design | |
---|---|---|
Phone use and sleep | Subjects are randomly assigned a level of phone use (none, low, or high) and follow that level of phone use throughout the experiment. | Subjects are assigned consecutively to zero, low, and high levels of phone use throughout the experiment, and the order in which they follow these treatments is randomized. |
Temperature and soil respiration | Warming treatments are assigned to soil plots at random and the soils are kept at this temperature throughout the experiment. | Every plot receives each warming treatment (1, 3, 5, 8, and 10C above ambient temperatures) consecutively over the course of the experiment, and the order in which they receive these treatments is randomized. |
Prevent plagiarism. Run a free check.
Finally, you need to decide how you’ll collect data on your dependent variable outcomes. You should aim for reliable and valid measurements that minimize research bias or error.
Some variables, like temperature, can be objectively measured with scientific instruments. Others may need to be operationalized to turn them into measurable observations.
- Ask participants to record what time they go to sleep and get up each day.
- Ask participants to wear a sleep tracker.
How precisely you measure your dependent variable also affects the kinds of statistical analysis you can use on your data.
Experiments are always context-dependent, and a good experimental design will take into account all of the unique considerations of your study system to produce information that is both valid and relevant to your research question.
If you want to know more about statistics , methodology , or research bias , make sure to check out some of our other articles with explanations and examples.
- Student’s t -distribution
- Normal distribution
- Null and Alternative Hypotheses
- Chi square tests
- Confidence interval
- Cluster sampling
- Stratified sampling
- Data cleansing
- Reproducibility vs Replicability
- Peer review
- Likert scale
Research bias
- Implicit bias
- Framing effect
- Cognitive bias
- Placebo effect
- Hawthorne effect
- Hindsight bias
- Affect heuristic
Experimental design means planning a set of procedures to investigate a relationship between variables . To design a controlled experiment, you need:
- A testable hypothesis
- At least one independent variable that can be precisely manipulated
- At least one dependent variable that can be precisely measured
When designing the experiment, you decide:
- How you will manipulate the variable(s)
- How you will control for any potential confounding variables
- How many subjects or samples will be included in the study
- How subjects will be assigned to treatment levels
Experimental design is essential to the internal and external validity of your experiment.
The key difference between observational studies and experimental designs is that a well-done observational study does not influence the responses of participants, while experiments do have some sort of treatment condition applied to at least some participants by random assignment .
A confounding variable , also called a confounder or confounding factor, is a third variable in a study examining a potential cause-and-effect relationship.
A confounding variable is related to both the supposed cause and the supposed effect of the study. It can be difficult to separate the true effect of the independent variable from the effect of the confounding variable.
In your research design , it’s important to identify potential confounding variables and plan how you will reduce their impact.
In a between-subjects design , every participant experiences only one condition, and researchers assess group differences between participants in various conditions.
In a within-subjects design , each participant experiences all conditions, and researchers test the same participants repeatedly for differences between conditions.
The word “between” means that you’re comparing different conditions between groups, while the word “within” means you’re comparing different conditions within the same group.
An experimental group, also known as a treatment group, receives the treatment whose effect researchers wish to study, whereas a control group does not. They should be identical in all other ways.
Cite this Scribbr article
If you want to cite this source, you can copy and paste the citation or click the “Cite this Scribbr article” button to automatically add the citation to our free Citation Generator.
Bevans, R. (2023, June 21). Guide to Experimental Design | Overview, 5 steps & Examples. Scribbr. Retrieved September 18, 2024, from https://www.scribbr.com/methodology/experimental-design/
Is this article helpful?
Rebecca Bevans
Other students also liked, random assignment in experiments | introduction & examples, quasi-experimental design | definition, types & examples, how to write a lab report, "i thought ai proofreading was useless but..".
I've been using Scribbr for years now and I know it's a service that won't disappoint. It does a good job spotting mistakes”
19+ Experimental Design Examples (Methods + Types)

Ever wondered how scientists discover new medicines, psychologists learn about behavior, or even how marketers figure out what kind of ads you like? Well, they all have something in common: they use a special plan or recipe called an "experimental design."
Imagine you're baking cookies. You can't just throw random amounts of flour, sugar, and chocolate chips into a bowl and hope for the best. You follow a recipe, right? Scientists and researchers do something similar. They follow a "recipe" called an experimental design to make sure their experiments are set up in a way that the answers they find are meaningful and reliable.
Experimental design is the roadmap researchers use to answer questions. It's a set of rules and steps that researchers follow to collect information, or "data," in a way that is fair, accurate, and makes sense.
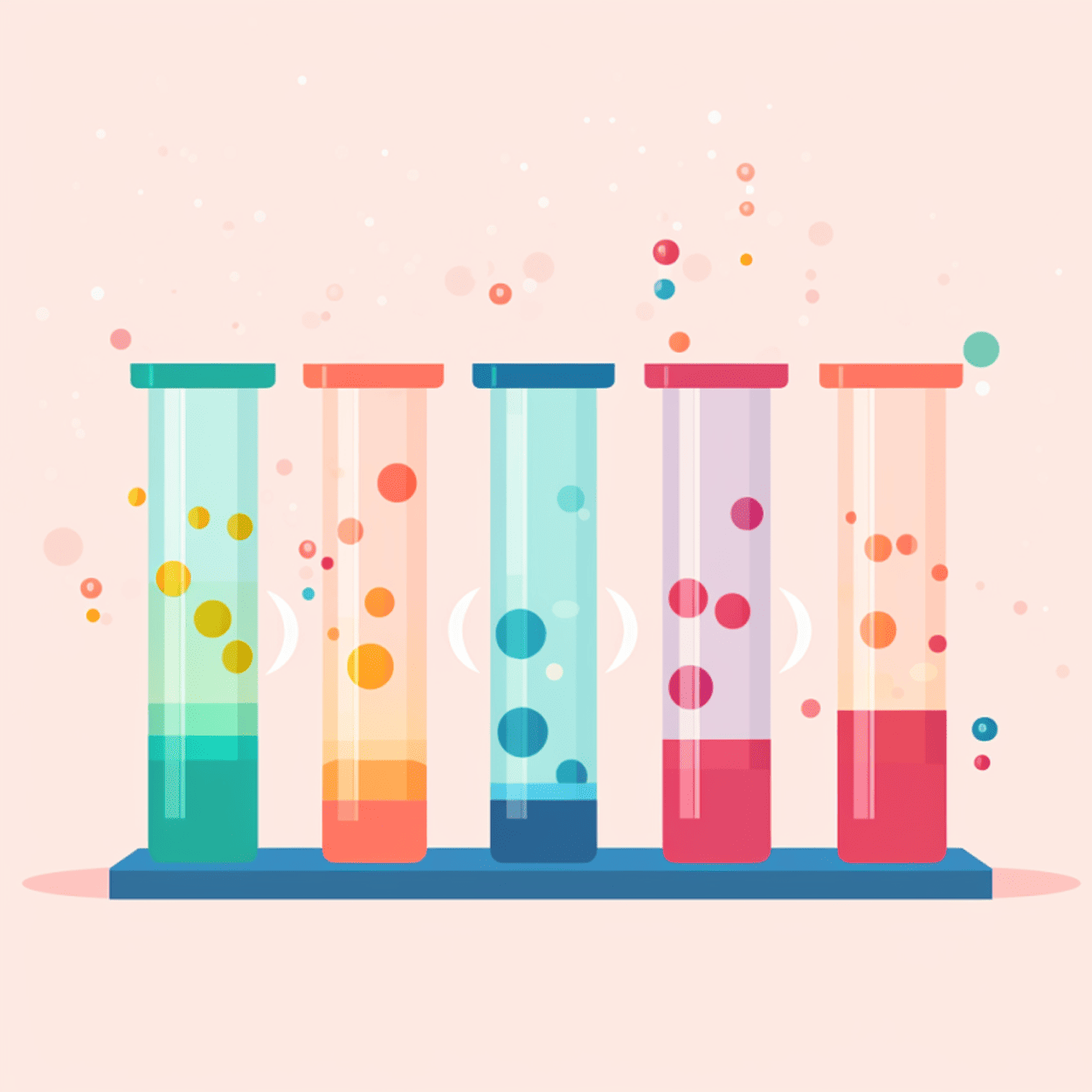
Long ago, people didn't have detailed game plans for experiments. They often just tried things out and saw what happened. But over time, people got smarter about this. They started creating structured plans—what we now call experimental designs—to get clearer, more trustworthy answers to their questions.
In this article, we'll take you on a journey through the world of experimental designs. We'll talk about the different types, or "flavors," of experimental designs, where they're used, and even give you a peek into how they came to be.
What Is Experimental Design?
Alright, before we dive into the different types of experimental designs, let's get crystal clear on what experimental design actually is.
Imagine you're a detective trying to solve a mystery. You need clues, right? Well, in the world of research, experimental design is like the roadmap that helps you find those clues. It's like the game plan in sports or the blueprint when you're building a house. Just like you wouldn't start building without a good blueprint, researchers won't start their studies without a strong experimental design.
So, why do we need experimental design? Think about baking a cake. If you toss ingredients into a bowl without measuring, you'll end up with a mess instead of a tasty dessert.
Similarly, in research, if you don't have a solid plan, you might get confusing or incorrect results. A good experimental design helps you ask the right questions ( think critically ), decide what to measure ( come up with an idea ), and figure out how to measure it (test it). It also helps you consider things that might mess up your results, like outside influences you hadn't thought of.
For example, let's say you want to find out if listening to music helps people focus better. Your experimental design would help you decide things like: Who are you going to test? What kind of music will you use? How will you measure focus? And, importantly, how will you make sure that it's really the music affecting focus and not something else, like the time of day or whether someone had a good breakfast?
In short, experimental design is the master plan that guides researchers through the process of collecting data, so they can answer questions in the most reliable way possible. It's like the GPS for the journey of discovery!
History of Experimental Design
Around 350 BCE, people like Aristotle were trying to figure out how the world works, but they mostly just thought really hard about things. They didn't test their ideas much. So while they were super smart, their methods weren't always the best for finding out the truth.
Fast forward to the Renaissance (14th to 17th centuries), a time of big changes and lots of curiosity. People like Galileo started to experiment by actually doing tests, like rolling balls down inclined planes to study motion. Galileo's work was cool because he combined thinking with doing. He'd have an idea, test it, look at the results, and then think some more. This approach was a lot more reliable than just sitting around and thinking.
Now, let's zoom ahead to the 18th and 19th centuries. This is when people like Francis Galton, an English polymath, started to get really systematic about experimentation. Galton was obsessed with measuring things. Seriously, he even tried to measure how good-looking people were ! His work helped create the foundations for a more organized approach to experiments.
Next stop: the early 20th century. Enter Ronald A. Fisher , a brilliant British statistician. Fisher was a game-changer. He came up with ideas that are like the bread and butter of modern experimental design.
Fisher invented the concept of the " control group "—that's a group of people or things that don't get the treatment you're testing, so you can compare them to those who do. He also stressed the importance of " randomization ," which means assigning people or things to different groups by chance, like drawing names out of a hat. This makes sure the experiment is fair and the results are trustworthy.
Around the same time, American psychologists like John B. Watson and B.F. Skinner were developing " behaviorism ." They focused on studying things that they could directly observe and measure, like actions and reactions.
Skinner even built boxes—called Skinner Boxes —to test how animals like pigeons and rats learn. Their work helped shape how psychologists design experiments today. Watson performed a very controversial experiment called The Little Albert experiment that helped describe behaviour through conditioning—in other words, how people learn to behave the way they do.
In the later part of the 20th century and into our time, computers have totally shaken things up. Researchers now use super powerful software to help design their experiments and crunch the numbers.
With computers, they can simulate complex experiments before they even start, which helps them predict what might happen. This is especially helpful in fields like medicine, where getting things right can be a matter of life and death.
Also, did you know that experimental designs aren't just for scientists in labs? They're used by people in all sorts of jobs, like marketing, education, and even video game design! Yes, someone probably ran an experiment to figure out what makes a game super fun to play.
So there you have it—a quick tour through the history of experimental design, from Aristotle's deep thoughts to Fisher's groundbreaking ideas, and all the way to today's computer-powered research. These designs are the recipes that help people from all walks of life find answers to their big questions.
Key Terms in Experimental Design
Before we dig into the different types of experimental designs, let's get comfy with some key terms. Understanding these terms will make it easier for us to explore the various types of experimental designs that researchers use to answer their big questions.
Independent Variable : This is what you change or control in your experiment to see what effect it has. Think of it as the "cause" in a cause-and-effect relationship. For example, if you're studying whether different types of music help people focus, the kind of music is the independent variable.
Dependent Variable : This is what you're measuring to see the effect of your independent variable. In our music and focus experiment, how well people focus is the dependent variable—it's what "depends" on the kind of music played.
Control Group : This is a group of people who don't get the special treatment or change you're testing. They help you see what happens when the independent variable is not applied. If you're testing whether a new medicine works, the control group would take a fake pill, called a placebo , instead of the real medicine.
Experimental Group : This is the group that gets the special treatment or change you're interested in. Going back to our medicine example, this group would get the actual medicine to see if it has any effect.
Randomization : This is like shaking things up in a fair way. You randomly put people into the control or experimental group so that each group is a good mix of different kinds of people. This helps make the results more reliable.
Sample : This is the group of people you're studying. They're a "sample" of a larger group that you're interested in. For instance, if you want to know how teenagers feel about a new video game, you might study a sample of 100 teenagers.
Bias : This is anything that might tilt your experiment one way or another without you realizing it. Like if you're testing a new kind of dog food and you only test it on poodles, that could create a bias because maybe poodles just really like that food and other breeds don't.
Data : This is the information you collect during the experiment. It's like the treasure you find on your journey of discovery!
Replication : This means doing the experiment more than once to make sure your findings hold up. It's like double-checking your answers on a test.
Hypothesis : This is your educated guess about what will happen in the experiment. It's like predicting the end of a movie based on the first half.
Steps of Experimental Design
Alright, let's say you're all fired up and ready to run your own experiment. Cool! But where do you start? Well, designing an experiment is a bit like planning a road trip. There are some key steps you've got to take to make sure you reach your destination. Let's break it down:
- Ask a Question : Before you hit the road, you've got to know where you're going. Same with experiments. You start with a question you want to answer, like "Does eating breakfast really make you do better in school?"
- Do Some Homework : Before you pack your bags, you look up the best places to visit, right? In science, this means reading up on what other people have already discovered about your topic.
- Form a Hypothesis : This is your educated guess about what you think will happen. It's like saying, "I bet this route will get us there faster."
- Plan the Details : Now you decide what kind of car you're driving (your experimental design), who's coming with you (your sample), and what snacks to bring (your variables).
- Randomization : Remember, this is like shuffling a deck of cards. You want to mix up who goes into your control and experimental groups to make sure it's a fair test.
- Run the Experiment : Finally, the rubber hits the road! You carry out your plan, making sure to collect your data carefully.
- Analyze the Data : Once the trip's over, you look at your photos and decide which ones are keepers. In science, this means looking at your data to see what it tells you.
- Draw Conclusions : Based on your data, did you find an answer to your question? This is like saying, "Yep, that route was faster," or "Nope, we hit a ton of traffic."
- Share Your Findings : After a great trip, you want to tell everyone about it, right? Scientists do the same by publishing their results so others can learn from them.
- Do It Again? : Sometimes one road trip just isn't enough. In the same way, scientists often repeat their experiments to make sure their findings are solid.
So there you have it! Those are the basic steps you need to follow when you're designing an experiment. Each step helps make sure that you're setting up a fair and reliable way to find answers to your big questions.
Let's get into examples of experimental designs.
1) True Experimental Design
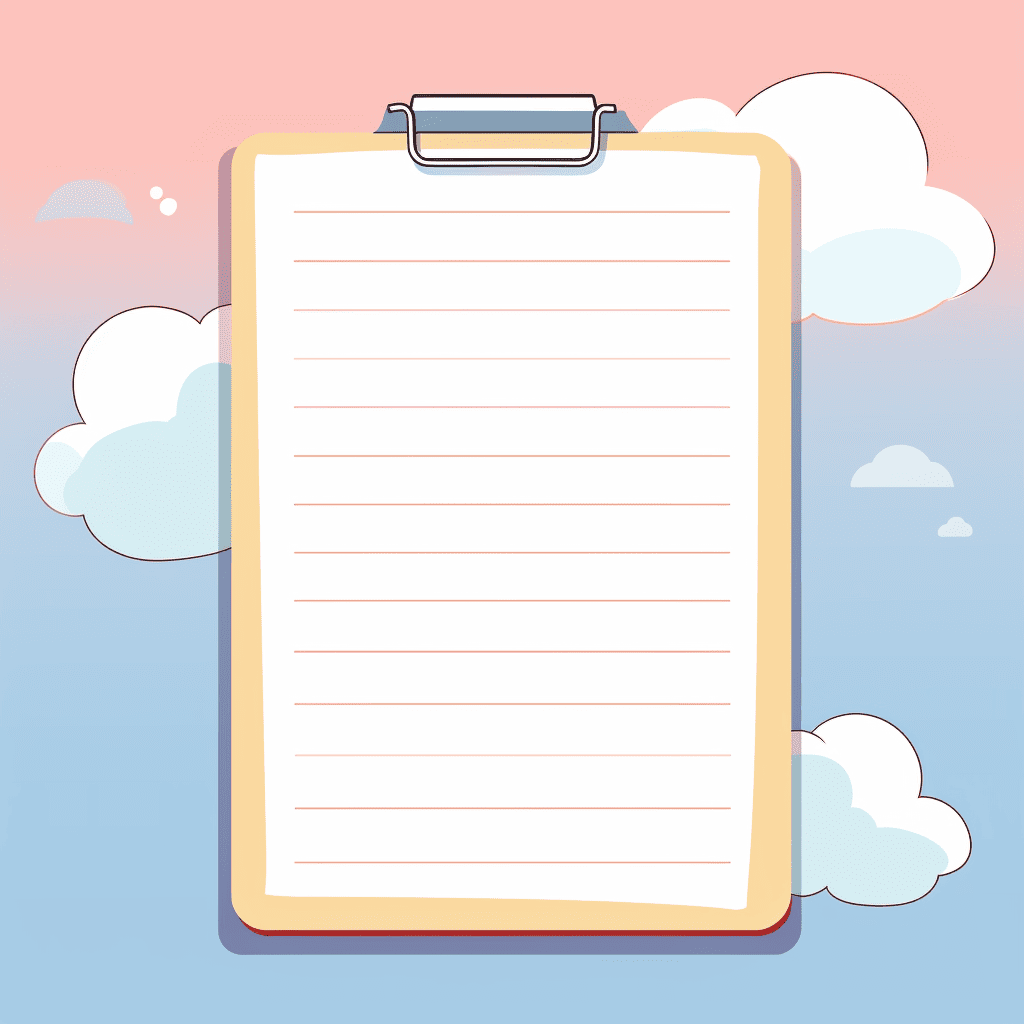
In the world of experiments, the True Experimental Design is like the superstar quarterback everyone talks about. Born out of the early 20th-century work of statisticians like Ronald A. Fisher, this design is all about control, precision, and reliability.
Researchers carefully pick an independent variable to manipulate (remember, that's the thing they're changing on purpose) and measure the dependent variable (the effect they're studying). Then comes the magic trick—randomization. By randomly putting participants into either the control or experimental group, scientists make sure their experiment is as fair as possible.
No sneaky biases here!
True Experimental Design Pros
The pros of True Experimental Design are like the perks of a VIP ticket at a concert: you get the best and most trustworthy results. Because everything is controlled and randomized, you can feel pretty confident that the results aren't just a fluke.
True Experimental Design Cons
However, there's a catch. Sometimes, it's really tough to set up these experiments in a real-world situation. Imagine trying to control every single detail of your day, from the food you eat to the air you breathe. Not so easy, right?
True Experimental Design Uses
The fields that get the most out of True Experimental Designs are those that need super reliable results, like medical research.
When scientists were developing COVID-19 vaccines, they used this design to run clinical trials. They had control groups that received a placebo (a harmless substance with no effect) and experimental groups that got the actual vaccine. Then they measured how many people in each group got sick. By comparing the two, they could say, "Yep, this vaccine works!"
So next time you read about a groundbreaking discovery in medicine or technology, chances are a True Experimental Design was the VIP behind the scenes, making sure everything was on point. It's been the go-to for rigorous scientific inquiry for nearly a century, and it's not stepping off the stage anytime soon.
2) Quasi-Experimental Design
So, let's talk about the Quasi-Experimental Design. Think of this one as the cool cousin of True Experimental Design. It wants to be just like its famous relative, but it's a bit more laid-back and flexible. You'll find quasi-experimental designs when it's tricky to set up a full-blown True Experimental Design with all the bells and whistles.
Quasi-experiments still play with an independent variable, just like their stricter cousins. The big difference? They don't use randomization. It's like wanting to divide a bag of jelly beans equally between your friends, but you can't quite do it perfectly.
In real life, it's often not possible or ethical to randomly assign people to different groups, especially when dealing with sensitive topics like education or social issues. And that's where quasi-experiments come in.
Quasi-Experimental Design Pros
Even though they lack full randomization, quasi-experimental designs are like the Swiss Army knives of research: versatile and practical. They're especially popular in fields like education, sociology, and public policy.
For instance, when researchers wanted to figure out if the Head Start program , aimed at giving young kids a "head start" in school, was effective, they used a quasi-experimental design. They couldn't randomly assign kids to go or not go to preschool, but they could compare kids who did with kids who didn't.
Quasi-Experimental Design Cons
Of course, quasi-experiments come with their own bag of pros and cons. On the plus side, they're easier to set up and often cheaper than true experiments. But the flip side is that they're not as rock-solid in their conclusions. Because the groups aren't randomly assigned, there's always that little voice saying, "Hey, are we missing something here?"
Quasi-Experimental Design Uses
Quasi-Experimental Design gained traction in the mid-20th century. Researchers were grappling with real-world problems that didn't fit neatly into a laboratory setting. Plus, as society became more aware of ethical considerations, the need for flexible designs increased. So, the quasi-experimental approach was like a breath of fresh air for scientists wanting to study complex issues without a laundry list of restrictions.
In short, if True Experimental Design is the superstar quarterback, Quasi-Experimental Design is the versatile player who can adapt and still make significant contributions to the game.
3) Pre-Experimental Design
Now, let's talk about the Pre-Experimental Design. Imagine it as the beginner's skateboard you get before you try out for all the cool tricks. It has wheels, it rolls, but it's not built for the professional skatepark.
Similarly, pre-experimental designs give researchers a starting point. They let you dip your toes in the water of scientific research without diving in head-first.
So, what's the deal with pre-experimental designs?
Pre-Experimental Designs are the basic, no-frills versions of experiments. Researchers still mess around with an independent variable and measure a dependent variable, but they skip over the whole randomization thing and often don't even have a control group.
It's like baking a cake but forgetting the frosting and sprinkles; you'll get some results, but they might not be as complete or reliable as you'd like.
Pre-Experimental Design Pros
Why use such a simple setup? Because sometimes, you just need to get the ball rolling. Pre-experimental designs are great for quick-and-dirty research when you're short on time or resources. They give you a rough idea of what's happening, which you can use to plan more detailed studies later.
A good example of this is early studies on the effects of screen time on kids. Researchers couldn't control every aspect of a child's life, but they could easily ask parents to track how much time their kids spent in front of screens and then look for trends in behavior or school performance.
Pre-Experimental Design Cons
But here's the catch: pre-experimental designs are like that first draft of an essay. It helps you get your ideas down, but you wouldn't want to turn it in for a grade. Because these designs lack the rigorous structure of true or quasi-experimental setups, they can't give you rock-solid conclusions. They're more like clues or signposts pointing you in a certain direction.
Pre-Experimental Design Uses
This type of design became popular in the early stages of various scientific fields. Researchers used them to scratch the surface of a topic, generate some initial data, and then decide if it's worth exploring further. In other words, pre-experimental designs were the stepping stones that led to more complex, thorough investigations.
So, while Pre-Experimental Design may not be the star player on the team, it's like the practice squad that helps everyone get better. It's the starting point that can lead to bigger and better things.
4) Factorial Design
Now, buckle up, because we're moving into the world of Factorial Design, the multi-tasker of the experimental universe.
Imagine juggling not just one, but multiple balls in the air—that's what researchers do in a factorial design.
In Factorial Design, researchers are not satisfied with just studying one independent variable. Nope, they want to study two or more at the same time to see how they interact.
It's like cooking with several spices to see how they blend together to create unique flavors.
Factorial Design became the talk of the town with the rise of computers. Why? Because this design produces a lot of data, and computers are the number crunchers that help make sense of it all. So, thanks to our silicon friends, researchers can study complicated questions like, "How do diet AND exercise together affect weight loss?" instead of looking at just one of those factors.
Factorial Design Pros
This design's main selling point is its ability to explore interactions between variables. For instance, maybe a new study drug works really well for young people but not so great for older adults. A factorial design could reveal that age is a crucial factor, something you might miss if you only studied the drug's effectiveness in general. It's like being a detective who looks for clues not just in one room but throughout the entire house.
Factorial Design Cons
However, factorial designs have their own bag of challenges. First off, they can be pretty complicated to set up and run. Imagine coordinating a four-way intersection with lots of cars coming from all directions—you've got to make sure everything runs smoothly, or you'll end up with a traffic jam. Similarly, researchers need to carefully plan how they'll measure and analyze all the different variables.
Factorial Design Uses
Factorial designs are widely used in psychology to untangle the web of factors that influence human behavior. They're also popular in fields like marketing, where companies want to understand how different aspects like price, packaging, and advertising influence a product's success.
And speaking of success, the factorial design has been a hit since statisticians like Ronald A. Fisher (yep, him again!) expanded on it in the early-to-mid 20th century. It offered a more nuanced way of understanding the world, proving that sometimes, to get the full picture, you've got to juggle more than one ball at a time.
So, if True Experimental Design is the quarterback and Quasi-Experimental Design is the versatile player, Factorial Design is the strategist who sees the entire game board and makes moves accordingly.
5) Longitudinal Design
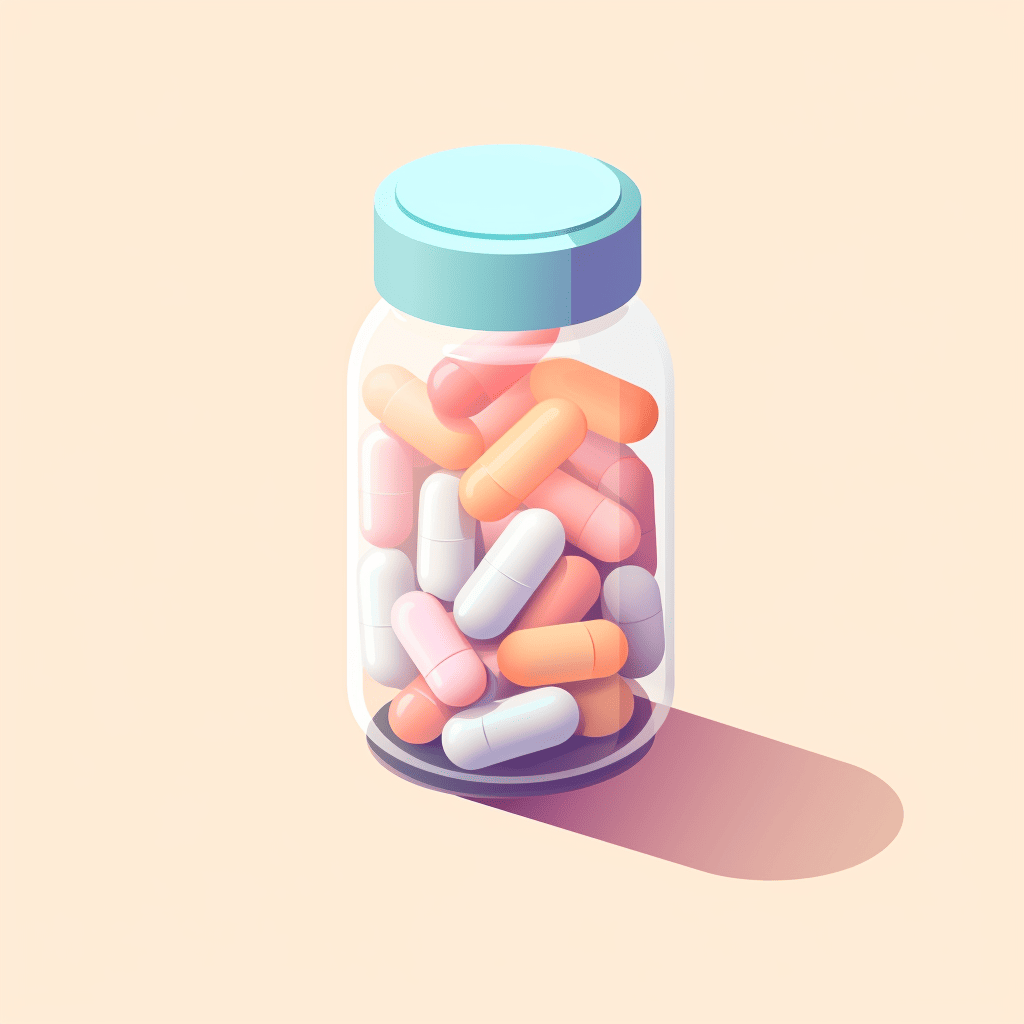
Alright, let's take a step into the world of Longitudinal Design. Picture it as the grand storyteller, the kind who doesn't just tell you about a single event but spins an epic tale that stretches over years or even decades. This design isn't about quick snapshots; it's about capturing the whole movie of someone's life or a long-running process.
You know how you might take a photo every year on your birthday to see how you've changed? Longitudinal Design is kind of like that, but for scientific research.
With Longitudinal Design, instead of measuring something just once, researchers come back again and again, sometimes over many years, to see how things are going. This helps them understand not just what's happening, but why it's happening and how it changes over time.
This design really started to shine in the latter half of the 20th century, when researchers began to realize that some questions can't be answered in a hurry. Think about studies that look at how kids grow up, or research on how a certain medicine affects you over a long period. These aren't things you can rush.
The famous Framingham Heart Study , started in 1948, is a prime example. It's been studying heart health in a small town in Massachusetts for decades, and the findings have shaped what we know about heart disease.
Longitudinal Design Pros
So, what's to love about Longitudinal Design? First off, it's the go-to for studying change over time, whether that's how people age or how a forest recovers from a fire.
Longitudinal Design Cons
But it's not all sunshine and rainbows. Longitudinal studies take a lot of patience and resources. Plus, keeping track of participants over many years can be like herding cats—difficult and full of surprises.
Longitudinal Design Uses
Despite these challenges, longitudinal studies have been key in fields like psychology, sociology, and medicine. They provide the kind of deep, long-term insights that other designs just can't match.
So, if the True Experimental Design is the superstar quarterback, and the Quasi-Experimental Design is the flexible athlete, then the Factorial Design is the strategist, and the Longitudinal Design is the wise elder who has seen it all and has stories to tell.
6) Cross-Sectional Design
Now, let's flip the script and talk about Cross-Sectional Design, the polar opposite of the Longitudinal Design. If Longitudinal is the grand storyteller, think of Cross-Sectional as the snapshot photographer. It captures a single moment in time, like a selfie that you take to remember a fun day. Researchers using this design collect all their data at one point, providing a kind of "snapshot" of whatever they're studying.
In a Cross-Sectional Design, researchers look at multiple groups all at the same time to see how they're different or similar.
This design rose to popularity in the mid-20th century, mainly because it's so quick and efficient. Imagine wanting to know how people of different ages feel about a new video game. Instead of waiting for years to see how opinions change, you could just ask people of all ages what they think right now. That's Cross-Sectional Design for you—fast and straightforward.
You'll find this type of research everywhere from marketing studies to healthcare. For instance, you might have heard about surveys asking people what they think about a new product or political issue. Those are usually cross-sectional studies, aimed at getting a quick read on public opinion.
Cross-Sectional Design Pros
So, what's the big deal with Cross-Sectional Design? Well, it's the go-to when you need answers fast and don't have the time or resources for a more complicated setup.
Cross-Sectional Design Cons
Remember, speed comes with trade-offs. While you get your results quickly, those results are stuck in time. They can't tell you how things change or why they're changing, just what's happening right now.
Cross-Sectional Design Uses
Also, because they're so quick and simple, cross-sectional studies often serve as the first step in research. They give scientists an idea of what's going on so they can decide if it's worth digging deeper. In that way, they're a bit like a movie trailer, giving you a taste of the action to see if you're interested in seeing the whole film.
So, in our lineup of experimental designs, if True Experimental Design is the superstar quarterback and Longitudinal Design is the wise elder, then Cross-Sectional Design is like the speedy running back—fast, agile, but not designed for long, drawn-out plays.
7) Correlational Design
Next on our roster is the Correlational Design, the keen observer of the experimental world. Imagine this design as the person at a party who loves people-watching. They don't interfere or get involved; they just observe and take mental notes about what's going on.
In a correlational study, researchers don't change or control anything; they simply observe and measure how two variables relate to each other.
The correlational design has roots in the early days of psychology and sociology. Pioneers like Sir Francis Galton used it to study how qualities like intelligence or height could be related within families.
This design is all about asking, "Hey, when this thing happens, does that other thing usually happen too?" For example, researchers might study whether students who have more study time get better grades or whether people who exercise more have lower stress levels.
One of the most famous correlational studies you might have heard of is the link between smoking and lung cancer. Back in the mid-20th century, researchers started noticing that people who smoked a lot also seemed to get lung cancer more often. They couldn't say smoking caused cancer—that would require a true experiment—but the strong correlation was a red flag that led to more research and eventually, health warnings.
Correlational Design Pros
This design is great at proving that two (or more) things can be related. Correlational designs can help prove that more detailed research is needed on a topic. They can help us see patterns or possible causes for things that we otherwise might not have realized.
Correlational Design Cons
But here's where you need to be careful: correlational designs can be tricky. Just because two things are related doesn't mean one causes the other. That's like saying, "Every time I wear my lucky socks, my team wins." Well, it's a fun thought, but those socks aren't really controlling the game.
Correlational Design Uses
Despite this limitation, correlational designs are popular in psychology, economics, and epidemiology, to name a few fields. They're often the first step in exploring a possible relationship between variables. Once a strong correlation is found, researchers may decide to conduct more rigorous experimental studies to examine cause and effect.
So, if the True Experimental Design is the superstar quarterback and the Longitudinal Design is the wise elder, the Factorial Design is the strategist, and the Cross-Sectional Design is the speedster, then the Correlational Design is the clever scout, identifying interesting patterns but leaving the heavy lifting of proving cause and effect to the other types of designs.
8) Meta-Analysis
Last but not least, let's talk about Meta-Analysis, the librarian of experimental designs.
If other designs are all about creating new research, Meta-Analysis is about gathering up everyone else's research, sorting it, and figuring out what it all means when you put it together.
Imagine a jigsaw puzzle where each piece is a different study. Meta-Analysis is the process of fitting all those pieces together to see the big picture.
The concept of Meta-Analysis started to take shape in the late 20th century, when computers became powerful enough to handle massive amounts of data. It was like someone handed researchers a super-powered magnifying glass, letting them examine multiple studies at the same time to find common trends or results.
You might have heard of the Cochrane Reviews in healthcare . These are big collections of meta-analyses that help doctors and policymakers figure out what treatments work best based on all the research that's been done.
For example, if ten different studies show that a certain medicine helps lower blood pressure, a meta-analysis would pull all that information together to give a more accurate answer.
Meta-Analysis Pros
The beauty of Meta-Analysis is that it can provide really strong evidence. Instead of relying on one study, you're looking at the whole landscape of research on a topic.
Meta-Analysis Cons
However, it does have some downsides. For one, Meta-Analysis is only as good as the studies it includes. If those studies are flawed, the meta-analysis will be too. It's like baking a cake: if you use bad ingredients, it doesn't matter how good your recipe is—the cake won't turn out well.
Meta-Analysis Uses
Despite these challenges, meta-analyses are highly respected and widely used in many fields like medicine, psychology, and education. They help us make sense of a world that's bursting with information by showing us the big picture drawn from many smaller snapshots.
So, in our all-star lineup, if True Experimental Design is the quarterback and Longitudinal Design is the wise elder, the Factorial Design is the strategist, the Cross-Sectional Design is the speedster, and the Correlational Design is the scout, then the Meta-Analysis is like the coach, using insights from everyone else's plays to come up with the best game plan.
9) Non-Experimental Design
Now, let's talk about a player who's a bit of an outsider on this team of experimental designs—the Non-Experimental Design. Think of this design as the commentator or the journalist who covers the game but doesn't actually play.
In a Non-Experimental Design, researchers are like reporters gathering facts, but they don't interfere or change anything. They're simply there to describe and analyze.
Non-Experimental Design Pros
So, what's the deal with Non-Experimental Design? Its strength is in description and exploration. It's really good for studying things as they are in the real world, without changing any conditions.
Non-Experimental Design Cons
Because a non-experimental design doesn't manipulate variables, it can't prove cause and effect. It's like a weather reporter: they can tell you it's raining, but they can't tell you why it's raining.
The downside? Since researchers aren't controlling variables, it's hard to rule out other explanations for what they observe. It's like hearing one side of a story—you get an idea of what happened, but it might not be the complete picture.
Non-Experimental Design Uses
Non-Experimental Design has always been a part of research, especially in fields like anthropology, sociology, and some areas of psychology.
For instance, if you've ever heard of studies that describe how people behave in different cultures or what teens like to do in their free time, that's often Non-Experimental Design at work. These studies aim to capture the essence of a situation, like painting a portrait instead of taking a snapshot.
One well-known example you might have heard about is the Kinsey Reports from the 1940s and 1950s, which described sexual behavior in men and women. Researchers interviewed thousands of people but didn't manipulate any variables like you would in a true experiment. They simply collected data to create a comprehensive picture of the subject matter.
So, in our metaphorical team of research designs, if True Experimental Design is the quarterback and Longitudinal Design is the wise elder, Factorial Design is the strategist, Cross-Sectional Design is the speedster, Correlational Design is the scout, and Meta-Analysis is the coach, then Non-Experimental Design is the sports journalist—always present, capturing the game, but not part of the action itself.
10) Repeated Measures Design
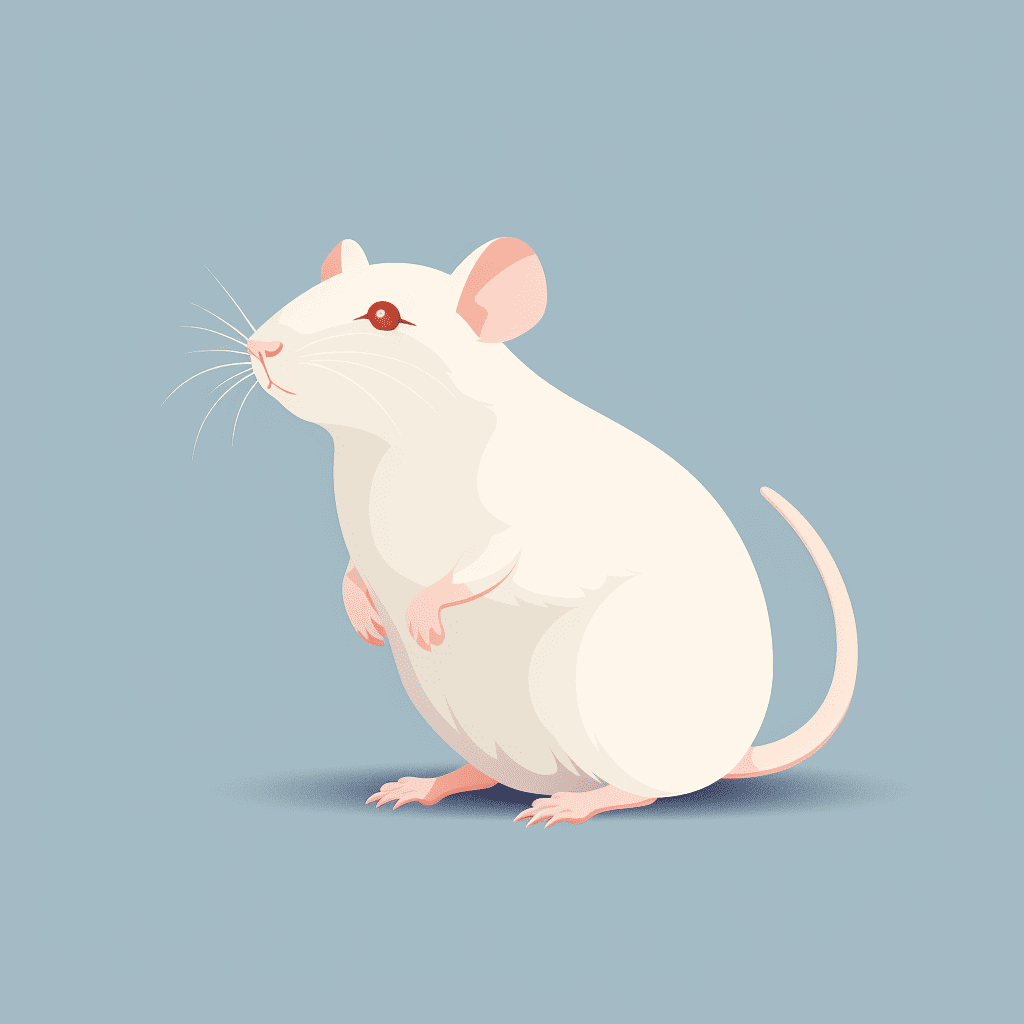
Time to meet the Repeated Measures Design, the time traveler of our research team. If this design were a player in a sports game, it would be the one who keeps revisiting past plays to figure out how to improve the next one.
Repeated Measures Design is all about studying the same people or subjects multiple times to see how they change or react under different conditions.
The idea behind Repeated Measures Design isn't new; it's been around since the early days of psychology and medicine. You could say it's a cousin to the Longitudinal Design, but instead of looking at how things naturally change over time, it focuses on how the same group reacts to different things.
Imagine a study looking at how a new energy drink affects people's running speed. Instead of comparing one group that drank the energy drink to another group that didn't, a Repeated Measures Design would have the same group of people run multiple times—once with the energy drink, and once without. This way, you're really zeroing in on the effect of that energy drink, making the results more reliable.
Repeated Measures Design Pros
The strong point of Repeated Measures Design is that it's super focused. Because it uses the same subjects, you don't have to worry about differences between groups messing up your results.
Repeated Measures Design Cons
But the downside? Well, people can get tired or bored if they're tested too many times, which might affect how they respond.
Repeated Measures Design Uses
A famous example of this design is the "Little Albert" experiment, conducted by John B. Watson and Rosalie Rayner in 1920. In this study, a young boy was exposed to a white rat and other stimuli several times to see how his emotional responses changed. Though the ethical standards of this experiment are often criticized today, it was groundbreaking in understanding conditioned emotional responses.
In our metaphorical lineup of research designs, if True Experimental Design is the quarterback and Longitudinal Design is the wise elder, Factorial Design is the strategist, Cross-Sectional Design is the speedster, Correlational Design is the scout, Meta-Analysis is the coach, and Non-Experimental Design is the journalist, then Repeated Measures Design is the time traveler—always looping back to fine-tune the game plan.
11) Crossover Design
Next up is Crossover Design, the switch-hitter of the research world. If you're familiar with baseball, you'll know a switch-hitter is someone who can bat both right-handed and left-handed.
In a similar way, Crossover Design allows subjects to experience multiple conditions, flipping them around so that everyone gets a turn in each role.
This design is like the utility player on our team—versatile, flexible, and really good at adapting.
The Crossover Design has its roots in medical research and has been popular since the mid-20th century. It's often used in clinical trials to test the effectiveness of different treatments.
Crossover Design Pros
The neat thing about this design is that it allows each participant to serve as their own control group. Imagine you're testing two new kinds of headache medicine. Instead of giving one type to one group and another type to a different group, you'd give both kinds to the same people but at different times.
Crossover Design Cons
What's the big deal with Crossover Design? Its major strength is in reducing the "noise" that comes from individual differences. Since each person experiences all conditions, it's easier to see real effects. However, there's a catch. This design assumes that there's no lasting effect from the first condition when you switch to the second one. That might not always be true. If the first treatment has a long-lasting effect, it could mess up the results when you switch to the second treatment.
Crossover Design Uses
A well-known example of Crossover Design is in studies that look at the effects of different types of diets—like low-carb vs. low-fat diets. Researchers might have participants follow a low-carb diet for a few weeks, then switch them to a low-fat diet. By doing this, they can more accurately measure how each diet affects the same group of people.
In our team of experimental designs, if True Experimental Design is the quarterback and Longitudinal Design is the wise elder, Factorial Design is the strategist, Cross-Sectional Design is the speedster, Correlational Design is the scout, Meta-Analysis is the coach, Non-Experimental Design is the journalist, and Repeated Measures Design is the time traveler, then Crossover Design is the versatile utility player—always ready to adapt and play multiple roles to get the most accurate results.
12) Cluster Randomized Design
Meet the Cluster Randomized Design, the team captain of group-focused research. In our imaginary lineup of experimental designs, if other designs focus on individual players, then Cluster Randomized Design is looking at how the entire team functions.
This approach is especially common in educational and community-based research, and it's been gaining traction since the late 20th century.
Here's how Cluster Randomized Design works: Instead of assigning individual people to different conditions, researchers assign entire groups, or "clusters." These could be schools, neighborhoods, or even entire towns. This helps you see how the new method works in a real-world setting.
Imagine you want to see if a new anti-bullying program really works. Instead of selecting individual students, you'd introduce the program to a whole school or maybe even several schools, and then compare the results to schools without the program.
Cluster Randomized Design Pros
Why use Cluster Randomized Design? Well, sometimes it's just not practical to assign conditions at the individual level. For example, you can't really have half a school following a new reading program while the other half sticks with the old one; that would be way too confusing! Cluster Randomization helps get around this problem by treating each "cluster" as its own mini-experiment.
Cluster Randomized Design Cons
There's a downside, too. Because entire groups are assigned to each condition, there's a risk that the groups might be different in some important way that the researchers didn't account for. That's like having one sports team that's full of veterans playing against a team of rookies; the match wouldn't be fair.
Cluster Randomized Design Uses
A famous example is the research conducted to test the effectiveness of different public health interventions, like vaccination programs. Researchers might roll out a vaccination program in one community but not in another, then compare the rates of disease in both.
In our metaphorical research team, if True Experimental Design is the quarterback, Longitudinal Design is the wise elder, Factorial Design is the strategist, Cross-Sectional Design is the speedster, Correlational Design is the scout, Meta-Analysis is the coach, Non-Experimental Design is the journalist, Repeated Measures Design is the time traveler, and Crossover Design is the utility player, then Cluster Randomized Design is the team captain—always looking out for the group as a whole.
13) Mixed-Methods Design
Say hello to Mixed-Methods Design, the all-rounder or the "Renaissance player" of our research team.
Mixed-Methods Design uses a blend of both qualitative and quantitative methods to get a more complete picture, just like a Renaissance person who's good at lots of different things. It's like being good at both offense and defense in a sport; you've got all your bases covered!
Mixed-Methods Design is a fairly new kid on the block, becoming more popular in the late 20th and early 21st centuries as researchers began to see the value in using multiple approaches to tackle complex questions. It's the Swiss Army knife in our research toolkit, combining the best parts of other designs to be more versatile.
Here's how it could work: Imagine you're studying the effects of a new educational app on students' math skills. You might use quantitative methods like tests and grades to measure how much the students improve—that's the 'numbers part.'
But you also want to know how the students feel about math now, or why they think they got better or worse. For that, you could conduct interviews or have students fill out journals—that's the 'story part.'
Mixed-Methods Design Pros
So, what's the scoop on Mixed-Methods Design? The strength is its versatility and depth; you're not just getting numbers or stories, you're getting both, which gives a fuller picture.
Mixed-Methods Design Cons
But, it's also more challenging. Imagine trying to play two sports at the same time! You have to be skilled in different research methods and know how to combine them effectively.
Mixed-Methods Design Uses
A high-profile example of Mixed-Methods Design is research on climate change. Scientists use numbers and data to show temperature changes (quantitative), but they also interview people to understand how these changes are affecting communities (qualitative).
In our team of experimental designs, if True Experimental Design is the quarterback, Longitudinal Design is the wise elder, Factorial Design is the strategist, Cross-Sectional Design is the speedster, Correlational Design is the scout, Meta-Analysis is the coach, Non-Experimental Design is the journalist, Repeated Measures Design is the time traveler, Crossover Design is the utility player, and Cluster Randomized Design is the team captain, then Mixed-Methods Design is the Renaissance player—skilled in multiple areas and able to bring them all together for a winning strategy.
14) Multivariate Design
Now, let's turn our attention to Multivariate Design, the multitasker of the research world.
If our lineup of research designs were like players on a basketball court, Multivariate Design would be the player dribbling, passing, and shooting all at once. This design doesn't just look at one or two things; it looks at several variables simultaneously to see how they interact and affect each other.
Multivariate Design is like baking a cake with many ingredients. Instead of just looking at how flour affects the cake, you also consider sugar, eggs, and milk all at once. This way, you understand how everything works together to make the cake taste good or bad.
Multivariate Design has been a go-to method in psychology, economics, and social sciences since the latter half of the 20th century. With the advent of computers and advanced statistical software, analyzing multiple variables at once became a lot easier, and Multivariate Design soared in popularity.
Multivariate Design Pros
So, what's the benefit of using Multivariate Design? Its power lies in its complexity. By studying multiple variables at the same time, you can get a really rich, detailed understanding of what's going on.
Multivariate Design Cons
But that complexity can also be a drawback. With so many variables, it can be tough to tell which ones are really making a difference and which ones are just along for the ride.
Multivariate Design Uses
Imagine you're a coach trying to figure out the best strategy to win games. You wouldn't just look at how many points your star player scores; you'd also consider assists, rebounds, turnovers, and maybe even how loud the crowd is. A Multivariate Design would help you understand how all these factors work together to determine whether you win or lose.
A well-known example of Multivariate Design is in market research. Companies often use this approach to figure out how different factors—like price, packaging, and advertising—affect sales. By studying multiple variables at once, they can find the best combination to boost profits.
In our metaphorical research team, if True Experimental Design is the quarterback, Longitudinal Design is the wise elder, Factorial Design is the strategist, Cross-Sectional Design is the speedster, Correlational Design is the scout, Meta-Analysis is the coach, Non-Experimental Design is the journalist, Repeated Measures Design is the time traveler, Crossover Design is the utility player, Cluster Randomized Design is the team captain, and Mixed-Methods Design is the Renaissance player, then Multivariate Design is the multitasker—juggling many variables at once to get a fuller picture of what's happening.
15) Pretest-Posttest Design
Let's introduce Pretest-Posttest Design, the "Before and After" superstar of our research team. You've probably seen those before-and-after pictures in ads for weight loss programs or home renovations, right?
Well, this design is like that, but for science! Pretest-Posttest Design checks out what things are like before the experiment starts and then compares that to what things are like after the experiment ends.
This design is one of the classics, a staple in research for decades across various fields like psychology, education, and healthcare. It's so simple and straightforward that it has stayed popular for a long time.
In Pretest-Posttest Design, you measure your subject's behavior or condition before you introduce any changes—that's your "before" or "pretest." Then you do your experiment, and after it's done, you measure the same thing again—that's your "after" or "posttest."
Pretest-Posttest Design Pros
What makes Pretest-Posttest Design special? It's pretty easy to understand and doesn't require fancy statistics.
Pretest-Posttest Design Cons
But there are some pitfalls. For example, what if the kids in our math example get better at multiplication just because they're older or because they've taken the test before? That would make it hard to tell if the program is really effective or not.
Pretest-Posttest Design Uses
Let's say you're a teacher and you want to know if a new math program helps kids get better at multiplication. First, you'd give all the kids a multiplication test—that's your pretest. Then you'd teach them using the new math program. At the end, you'd give them the same test again—that's your posttest. If the kids do better on the second test, you might conclude that the program works.
One famous use of Pretest-Posttest Design is in evaluating the effectiveness of driver's education courses. Researchers will measure people's driving skills before and after the course to see if they've improved.
16) Solomon Four-Group Design
Next up is the Solomon Four-Group Design, the "chess master" of our research team. This design is all about strategy and careful planning. Named after Richard L. Solomon who introduced it in the 1940s, this method tries to correct some of the weaknesses in simpler designs, like the Pretest-Posttest Design.
Here's how it rolls: The Solomon Four-Group Design uses four different groups to test a hypothesis. Two groups get a pretest, then one of them receives the treatment or intervention, and both get a posttest. The other two groups skip the pretest, and only one of them receives the treatment before they both get a posttest.
Sound complicated? It's like playing 4D chess; you're thinking several moves ahead!
Solomon Four-Group Design Pros
What's the pro and con of the Solomon Four-Group Design? On the plus side, it provides really robust results because it accounts for so many variables.
Solomon Four-Group Design Cons
The downside? It's a lot of work and requires a lot of participants, making it more time-consuming and costly.
Solomon Four-Group Design Uses
Let's say you want to figure out if a new way of teaching history helps students remember facts better. Two classes take a history quiz (pretest), then one class uses the new teaching method while the other sticks with the old way. Both classes take another quiz afterward (posttest).
Meanwhile, two more classes skip the initial quiz, and then one uses the new method before both take the final quiz. Comparing all four groups will give you a much clearer picture of whether the new teaching method works and whether the pretest itself affects the outcome.
The Solomon Four-Group Design is less commonly used than simpler designs but is highly respected for its ability to control for more variables. It's a favorite in educational and psychological research where you really want to dig deep and figure out what's actually causing changes.
17) Adaptive Designs
Now, let's talk about Adaptive Designs, the chameleons of the experimental world.
Imagine you're a detective, and halfway through solving a case, you find a clue that changes everything. You wouldn't just stick to your old plan; you'd adapt and change your approach, right? That's exactly what Adaptive Designs allow researchers to do.
In an Adaptive Design, researchers can make changes to the study as it's happening, based on early results. In a traditional study, once you set your plan, you stick to it from start to finish.
Adaptive Design Pros
This method is particularly useful in fast-paced or high-stakes situations, like developing a new vaccine in the middle of a pandemic. The ability to adapt can save both time and resources, and more importantly, it can save lives by getting effective treatments out faster.
Adaptive Design Cons
But Adaptive Designs aren't without their drawbacks. They can be very complex to plan and carry out, and there's always a risk that the changes made during the study could introduce bias or errors.
Adaptive Design Uses
Adaptive Designs are most often seen in clinical trials, particularly in the medical and pharmaceutical fields.
For instance, if a new drug is showing really promising results, the study might be adjusted to give more participants the new treatment instead of a placebo. Or if one dose level is showing bad side effects, it might be dropped from the study.
The best part is, these changes are pre-planned. Researchers lay out in advance what changes might be made and under what conditions, which helps keep everything scientific and above board.
In terms of applications, besides their heavy usage in medical and pharmaceutical research, Adaptive Designs are also becoming increasingly popular in software testing and market research. In these fields, being able to quickly adjust to early results can give companies a significant advantage.
Adaptive Designs are like the agile startups of the research world—quick to pivot, keen to learn from ongoing results, and focused on rapid, efficient progress. However, they require a great deal of expertise and careful planning to ensure that the adaptability doesn't compromise the integrity of the research.
18) Bayesian Designs
Next, let's dive into Bayesian Designs, the data detectives of the research universe. Named after Thomas Bayes, an 18th-century statistician and minister, this design doesn't just look at what's happening now; it also takes into account what's happened before.
Imagine if you were a detective who not only looked at the evidence in front of you but also used your past cases to make better guesses about your current one. That's the essence of Bayesian Designs.
Bayesian Designs are like detective work in science. As you gather more clues (or data), you update your best guess on what's really happening. This way, your experiment gets smarter as it goes along.
In the world of research, Bayesian Designs are most notably used in areas where you have some prior knowledge that can inform your current study. For example, if earlier research shows that a certain type of medicine usually works well for a specific illness, a Bayesian Design would include that information when studying a new group of patients with the same illness.
Bayesian Design Pros
One of the major advantages of Bayesian Designs is their efficiency. Because they use existing data to inform the current experiment, often fewer resources are needed to reach a reliable conclusion.
Bayesian Design Cons
However, they can be quite complicated to set up and require a deep understanding of both statistics and the subject matter at hand.
Bayesian Design Uses
Bayesian Designs are highly valued in medical research, finance, environmental science, and even in Internet search algorithms. Their ability to continually update and refine hypotheses based on new evidence makes them particularly useful in fields where data is constantly evolving and where quick, informed decisions are crucial.
Here's a real-world example: In the development of personalized medicine, where treatments are tailored to individual patients, Bayesian Designs are invaluable. If a treatment has been effective for patients with similar genetics or symptoms in the past, a Bayesian approach can use that data to predict how well it might work for a new patient.
This type of design is also increasingly popular in machine learning and artificial intelligence. In these fields, Bayesian Designs help algorithms "learn" from past data to make better predictions or decisions in new situations. It's like teaching a computer to be a detective that gets better and better at solving puzzles the more puzzles it sees.
19) Covariate Adaptive Randomization
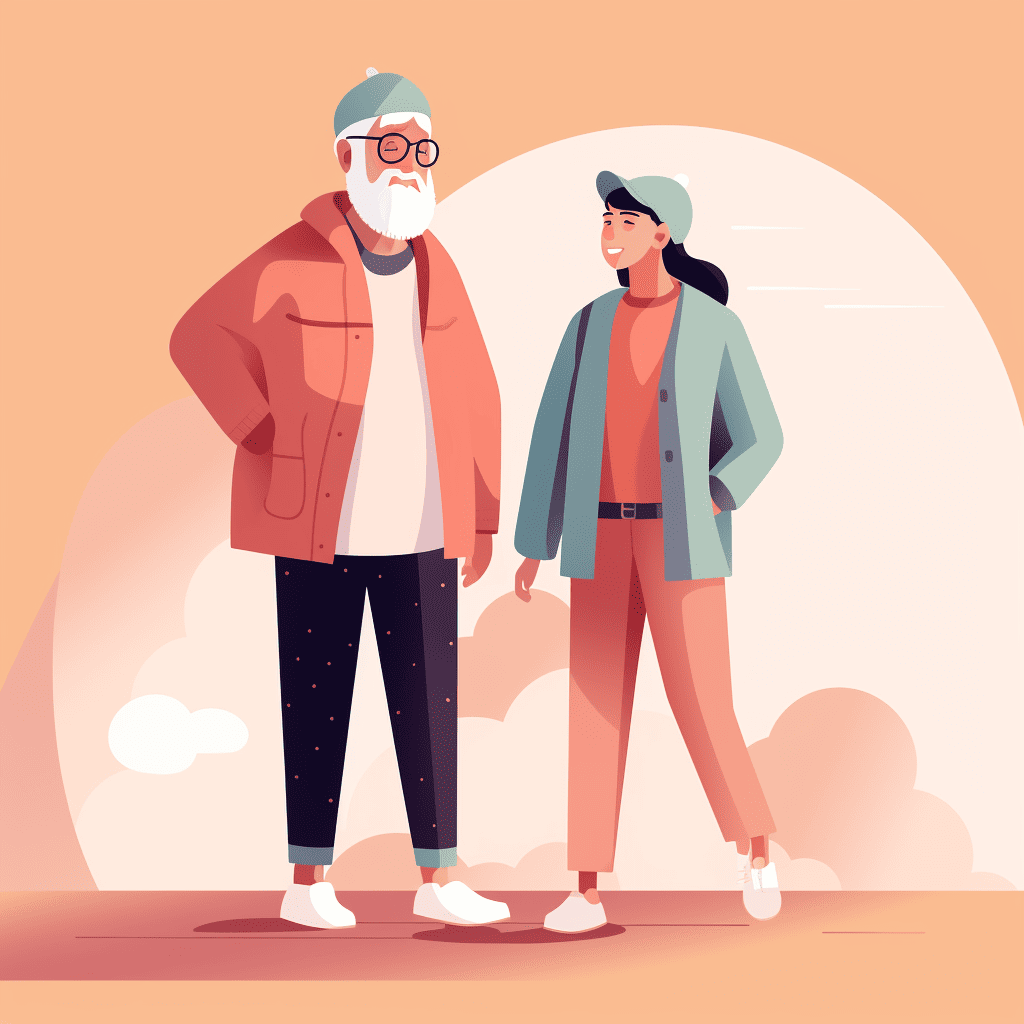
Now let's turn our attention to Covariate Adaptive Randomization, which you can think of as the "matchmaker" of experimental designs.
Picture a soccer coach trying to create the most balanced teams for a friendly match. They wouldn't just randomly assign players; they'd take into account each player's skills, experience, and other traits.
Covariate Adaptive Randomization is all about creating the most evenly matched groups possible for an experiment.
In traditional randomization, participants are allocated to different groups purely by chance. This is a pretty fair way to do things, but it can sometimes lead to unbalanced groups.
Imagine if all the professional-level players ended up on one soccer team and all the beginners on another; that wouldn't be a very informative match! Covariate Adaptive Randomization fixes this by using important traits or characteristics (called "covariates") to guide the randomization process.
Covariate Adaptive Randomization Pros
The benefits of this design are pretty clear: it aims for balance and fairness, making the final results more trustworthy.
Covariate Adaptive Randomization Cons
But it's not perfect. It can be complex to implement and requires a deep understanding of which characteristics are most important to balance.
Covariate Adaptive Randomization Uses
This design is particularly useful in medical trials. Let's say researchers are testing a new medication for high blood pressure. Participants might have different ages, weights, or pre-existing conditions that could affect the results.
Covariate Adaptive Randomization would make sure that each treatment group has a similar mix of these characteristics, making the results more reliable and easier to interpret.
In practical terms, this design is often seen in clinical trials for new drugs or therapies, but its principles are also applicable in fields like psychology, education, and social sciences.
For instance, in educational research, it might be used to ensure that classrooms being compared have similar distributions of students in terms of academic ability, socioeconomic status, and other factors.
Covariate Adaptive Randomization is like the wise elder of the group, ensuring that everyone has an equal opportunity to show their true capabilities, thereby making the collective results as reliable as possible.
20) Stepped Wedge Design
Let's now focus on the Stepped Wedge Design, a thoughtful and cautious member of the experimental design family.
Imagine you're trying out a new gardening technique, but you're not sure how well it will work. You decide to apply it to one section of your garden first, watch how it performs, and then gradually extend the technique to other sections. This way, you get to see its effects over time and across different conditions. That's basically how Stepped Wedge Design works.
In a Stepped Wedge Design, all participants or clusters start off in the control group, and then, at different times, they 'step' over to the intervention or treatment group. This creates a wedge-like pattern over time where more and more participants receive the treatment as the study progresses. It's like rolling out a new policy in phases, monitoring its impact at each stage before extending it to more people.
Stepped Wedge Design Pros
The Stepped Wedge Design offers several advantages. Firstly, it allows for the study of interventions that are expected to do more good than harm, which makes it ethically appealing.
Secondly, it's useful when resources are limited and it's not feasible to roll out a new treatment to everyone at once. Lastly, because everyone eventually receives the treatment, it can be easier to get buy-in from participants or organizations involved in the study.
Stepped Wedge Design Cons
However, this design can be complex to analyze because it has to account for both the time factor and the changing conditions in each 'step' of the wedge. And like any study where participants know they're receiving an intervention, there's the potential for the results to be influenced by the placebo effect or other biases.
Stepped Wedge Design Uses
This design is particularly useful in health and social care research. For instance, if a hospital wants to implement a new hygiene protocol, it might start in one department, assess its impact, and then roll it out to other departments over time. This allows the hospital to adjust and refine the new protocol based on real-world data before it's fully implemented.
In terms of applications, Stepped Wedge Designs are commonly used in public health initiatives, organizational changes in healthcare settings, and social policy trials. They are particularly useful in situations where an intervention is being rolled out gradually and it's important to understand its impacts at each stage.
21) Sequential Design
Next up is Sequential Design, the dynamic and flexible member of our experimental design family.
Imagine you're playing a video game where you can choose different paths. If you take one path and find a treasure chest, you might decide to continue in that direction. If you hit a dead end, you might backtrack and try a different route. Sequential Design operates in a similar fashion, allowing researchers to make decisions at different stages based on what they've learned so far.
In a Sequential Design, the experiment is broken down into smaller parts, or "sequences." After each sequence, researchers pause to look at the data they've collected. Based on those findings, they then decide whether to stop the experiment because they've got enough information, or to continue and perhaps even modify the next sequence.
Sequential Design Pros
This allows for a more efficient use of resources, as you're only continuing with the experiment if the data suggests it's worth doing so.
One of the great things about Sequential Design is its efficiency. Because you're making data-driven decisions along the way, you can often reach conclusions more quickly and with fewer resources.
Sequential Design Cons
However, it requires careful planning and expertise to ensure that these "stop or go" decisions are made correctly and without bias.
Sequential Design Uses
In terms of its applications, besides healthcare and medicine, Sequential Design is also popular in quality control in manufacturing, environmental monitoring, and financial modeling. In these areas, being able to make quick decisions based on incoming data can be a big advantage.
This design is often used in clinical trials involving new medications or treatments. For example, if early results show that a new drug has significant side effects, the trial can be stopped before more people are exposed to it.
On the flip side, if the drug is showing promising results, the trial might be expanded to include more participants or to extend the testing period.
Think of Sequential Design as the nimble athlete of experimental designs, capable of quick pivots and adjustments to reach the finish line in the most effective way possible. But just like an athlete needs a good coach, this design requires expert oversight to make sure it stays on the right track.
22) Field Experiments
Last but certainly not least, let's explore Field Experiments—the adventurers of the experimental design world.
Picture a scientist leaving the controlled environment of a lab to test a theory in the real world, like a biologist studying animals in their natural habitat or a social scientist observing people in a real community. These are Field Experiments, and they're all about getting out there and gathering data in real-world settings.
Field Experiments embrace the messiness of the real world, unlike laboratory experiments, where everything is controlled down to the smallest detail. This makes them both exciting and challenging.
Field Experiment Pros
On one hand, the results often give us a better understanding of how things work outside the lab.
While Field Experiments offer real-world relevance, they come with challenges like controlling for outside factors and the ethical considerations of intervening in people's lives without their knowledge.
Field Experiment Cons
On the other hand, the lack of control can make it harder to tell exactly what's causing what. Yet, despite these challenges, they remain a valuable tool for researchers who want to understand how theories play out in the real world.
Field Experiment Uses
Let's say a school wants to improve student performance. In a Field Experiment, they might change the school's daily schedule for one semester and keep track of how students perform compared to another school where the schedule remained the same.
Because the study is happening in a real school with real students, the results could be very useful for understanding how the change might work in other schools. But since it's the real world, lots of other factors—like changes in teachers or even the weather—could affect the results.
Field Experiments are widely used in economics, psychology, education, and public policy. For example, you might have heard of the famous "Broken Windows" experiment in the 1980s that looked at how small signs of disorder, like broken windows or graffiti, could encourage more serious crime in neighborhoods. This experiment had a big impact on how cities think about crime prevention.
From the foundational concepts of control groups and independent variables to the sophisticated layouts like Covariate Adaptive Randomization and Sequential Design, it's clear that the realm of experimental design is as varied as it is fascinating.
We've seen that each design has its own special talents, ideal for specific situations. Some designs, like the Classic Controlled Experiment, are like reliable old friends you can always count on.
Others, like Sequential Design, are flexible and adaptable, making quick changes based on what they learn. And let's not forget the adventurous Field Experiments, which take us out of the lab and into the real world to discover things we might not see otherwise.
Choosing the right experimental design is like picking the right tool for the job. The method you choose can make a big difference in how reliable your results are and how much people will trust what you've discovered. And as we've learned, there's a design to suit just about every question, every problem, and every curiosity.
So the next time you read about a new discovery in medicine, psychology, or any other field, you'll have a better understanding of the thought and planning that went into figuring things out. Experimental design is more than just a set of rules; it's a structured way to explore the unknown and answer questions that can change the world.
Related posts:
- Experimental Psychologist Career (Salary + Duties + Interviews)
- 40+ Famous Psychologists (Images + Biographies)
- 11+ Psychology Experiment Ideas (Goals + Methods)
- The Little Albert Experiment
- 41+ White Collar Job Examples (Salary + Path)
Reference this article:
About The Author
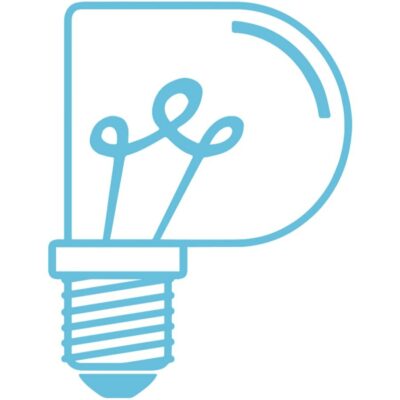
Free Personality Test
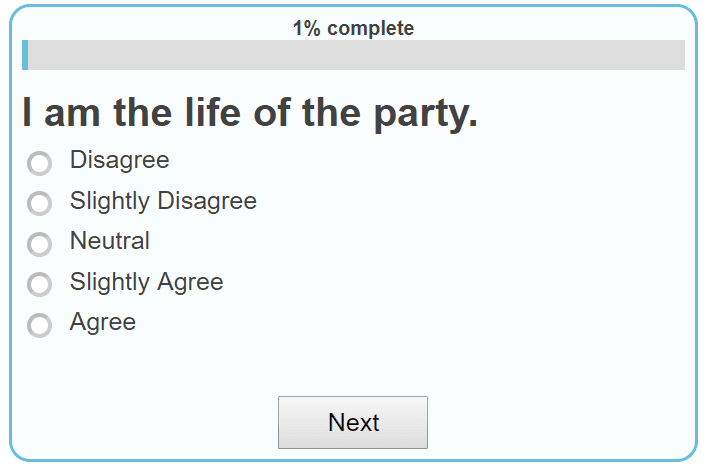
Free Memory Test
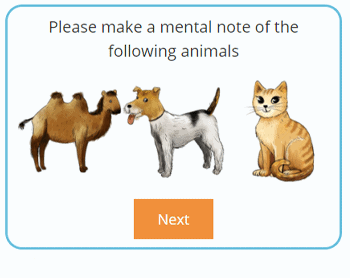
Free IQ Test
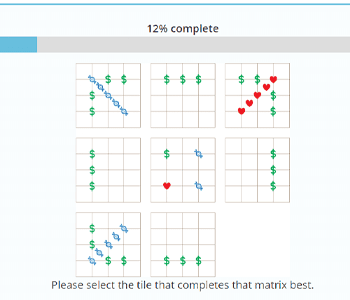
PracticalPie.com is a participant in the Amazon Associates Program. As an Amazon Associate we earn from qualifying purchases.
Follow Us On:
Youtube Facebook Instagram X/Twitter
Psychology Resources
Developmental
Personality
Relationships
Psychologists
Serial Killers
Psychology Tests
Personality Quiz
Memory Test
Depression test
Type A/B Personality Test
© PracticalPsychology. All rights reserved
Privacy Policy | Terms of Use
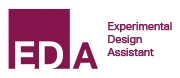
- Animal characteristics
- Independent variables
- Group and sample size
- Experimental unit
- Inclusion and exclusion
- Intervention
- Measurement
- Overview and demonstration of the EDA
- Getting the most out of the EDA
- What is the experiment diagram?
- Troubleshooting
The Experimental Design Assistant
A free resource from the NC3Rs used by over 5,000 researchers worldwide to help you design robust experiments more likely to yield reliable and reproducible results.
The EDA helps you build a diagram representing your experimental plan, which can be critiqued by the system to provide bespoke feedback . The EDA also:
- Recommends statistical analysis methods
- Provides support for randomisation and blinding
- Performs sample size calculations
For an overview of how the EDA works, watch our 1 minute video.
The EDA website also provides information about the different concepts of experimental design , and how to apply these in your experiments.
Chemix is an online editor for drawing lab diagrams and school experiment apparatus. Easy sketching for both students and teachers.
loading….
experimental design .online
Factors and Levels
Factorial design settings, response surface design settings, factor analysis, regresson model, optimization, quick guide.
Enter your experimental design variable names (factors) and values (levels). Examples of factors are ‘color’, ‘size’, ‘shape’, etc. Examples of levels are ‘red’ and ‘blue’ for ‘color’; 10, 20, 30 for ‘size’; ‘square’ and ‘round’ for 'shape'.
Select the number of measured responses and DoE types. Enter your responses into the DoE table. Use the ‘Settings’ button to set up the particular design.
Study the effect size for each factor and response breakdown.
Select the regression model and create it by pressing the ‘Create the Model’ button. Study the statistics.
Use the buttons to find factor levels for minimum and maximum possible responses. Use sliders to fine-tune the response values.
General Partner
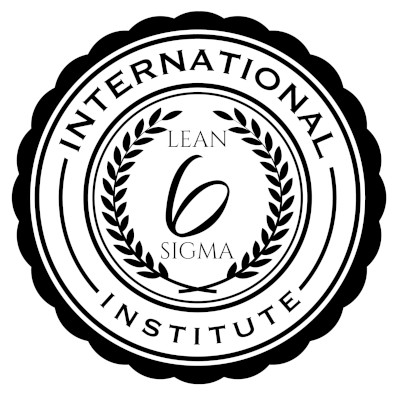
Partnership
- Privacy Policy

Home » Experimental Design – Types, Methods, Guide
Experimental Design – Types, Methods, Guide
Table of Contents

Experimental Design
Experimental design is a process of planning and conducting scientific experiments to investigate a hypothesis or research question. It involves carefully designing an experiment that can test the hypothesis, and controlling for other variables that may influence the results.
Experimental design typically includes identifying the variables that will be manipulated or measured, defining the sample or population to be studied, selecting an appropriate method of sampling, choosing a method for data collection and analysis, and determining the appropriate statistical tests to use.
Types of Experimental Design
Here are the different types of experimental design:
Completely Randomized Design
In this design, participants are randomly assigned to one of two or more groups, and each group is exposed to a different treatment or condition.
Randomized Block Design
This design involves dividing participants into blocks based on a specific characteristic, such as age or gender, and then randomly assigning participants within each block to one of two or more treatment groups.
Factorial Design
In a factorial design, participants are randomly assigned to one of several groups, each of which receives a different combination of two or more independent variables.

Repeated Measures Design
In this design, each participant is exposed to all of the different treatments or conditions, either in a random order or in a predetermined order.
Crossover Design
This design involves randomly assigning participants to one of two or more treatment groups, with each group receiving one treatment during the first phase of the study and then switching to a different treatment during the second phase.
Split-plot Design
In this design, the researcher manipulates one or more variables at different levels and uses a randomized block design to control for other variables.
Nested Design
This design involves grouping participants within larger units, such as schools or households, and then randomly assigning these units to different treatment groups.
Laboratory Experiment
Laboratory experiments are conducted under controlled conditions, which allows for greater precision and accuracy. However, because laboratory conditions are not always representative of real-world conditions, the results of these experiments may not be generalizable to the population at large.
Field Experiment
Field experiments are conducted in naturalistic settings and allow for more realistic observations. However, because field experiments are not as controlled as laboratory experiments, they may be subject to more sources of error.
Experimental Design Methods
Experimental design methods refer to the techniques and procedures used to design and conduct experiments in scientific research. Here are some common experimental design methods:
Randomization
This involves randomly assigning participants to different groups or treatments to ensure that any observed differences between groups are due to the treatment and not to other factors.
Control Group
The use of a control group is an important experimental design method that involves having a group of participants that do not receive the treatment or intervention being studied. The control group is used as a baseline to compare the effects of the treatment group.
Blinding involves keeping participants, researchers, or both unaware of which treatment group participants are in, in order to reduce the risk of bias in the results.
Counterbalancing
This involves systematically varying the order in which participants receive treatments or interventions in order to control for order effects.
Replication
Replication involves conducting the same experiment with different samples or under different conditions to increase the reliability and validity of the results.
This experimental design method involves manipulating multiple independent variables simultaneously to investigate their combined effects on the dependent variable.
This involves dividing participants into subgroups or blocks based on specific characteristics, such as age or gender, in order to reduce the risk of confounding variables.
Data Collection Method
Experimental design data collection methods are techniques and procedures used to collect data in experimental research. Here are some common experimental design data collection methods:
Direct Observation
This method involves observing and recording the behavior or phenomenon of interest in real time. It may involve the use of structured or unstructured observation, and may be conducted in a laboratory or naturalistic setting.
Self-report Measures
Self-report measures involve asking participants to report their thoughts, feelings, or behaviors using questionnaires, surveys, or interviews. These measures may be administered in person or online.
Behavioral Measures
Behavioral measures involve measuring participants’ behavior directly, such as through reaction time tasks or performance tests. These measures may be administered using specialized equipment or software.
Physiological Measures
Physiological measures involve measuring participants’ physiological responses, such as heart rate, blood pressure, or brain activity, using specialized equipment. These measures may be invasive or non-invasive, and may be administered in a laboratory or clinical setting.
Archival Data
Archival data involves using existing records or data, such as medical records, administrative records, or historical documents, as a source of information. These data may be collected from public or private sources.
Computerized Measures
Computerized measures involve using software or computer programs to collect data on participants’ behavior or responses. These measures may include reaction time tasks, cognitive tests, or other types of computer-based assessments.
Video Recording
Video recording involves recording participants’ behavior or interactions using cameras or other recording equipment. This method can be used to capture detailed information about participants’ behavior or to analyze social interactions.
Data Analysis Method
Experimental design data analysis methods refer to the statistical techniques and procedures used to analyze data collected in experimental research. Here are some common experimental design data analysis methods:
Descriptive Statistics
Descriptive statistics are used to summarize and describe the data collected in the study. This includes measures such as mean, median, mode, range, and standard deviation.
Inferential Statistics
Inferential statistics are used to make inferences or generalizations about a larger population based on the data collected in the study. This includes hypothesis testing and estimation.
Analysis of Variance (ANOVA)
ANOVA is a statistical technique used to compare means across two or more groups in order to determine whether there are significant differences between the groups. There are several types of ANOVA, including one-way ANOVA, two-way ANOVA, and repeated measures ANOVA.
Regression Analysis
Regression analysis is used to model the relationship between two or more variables in order to determine the strength and direction of the relationship. There are several types of regression analysis, including linear regression, logistic regression, and multiple regression.
Factor Analysis
Factor analysis is used to identify underlying factors or dimensions in a set of variables. This can be used to reduce the complexity of the data and identify patterns in the data.
Structural Equation Modeling (SEM)
SEM is a statistical technique used to model complex relationships between variables. It can be used to test complex theories and models of causality.
Cluster Analysis
Cluster analysis is used to group similar cases or observations together based on similarities or differences in their characteristics.
Time Series Analysis
Time series analysis is used to analyze data collected over time in order to identify trends, patterns, or changes in the data.
Multilevel Modeling
Multilevel modeling is used to analyze data that is nested within multiple levels, such as students nested within schools or employees nested within companies.
Applications of Experimental Design
Experimental design is a versatile research methodology that can be applied in many fields. Here are some applications of experimental design:
- Medical Research: Experimental design is commonly used to test new treatments or medications for various medical conditions. This includes clinical trials to evaluate the safety and effectiveness of new drugs or medical devices.
- Agriculture : Experimental design is used to test new crop varieties, fertilizers, and other agricultural practices. This includes randomized field trials to evaluate the effects of different treatments on crop yield, quality, and pest resistance.
- Environmental science: Experimental design is used to study the effects of environmental factors, such as pollution or climate change, on ecosystems and wildlife. This includes controlled experiments to study the effects of pollutants on plant growth or animal behavior.
- Psychology : Experimental design is used to study human behavior and cognitive processes. This includes experiments to test the effects of different interventions, such as therapy or medication, on mental health outcomes.
- Engineering : Experimental design is used to test new materials, designs, and manufacturing processes in engineering applications. This includes laboratory experiments to test the strength and durability of new materials, or field experiments to test the performance of new technologies.
- Education : Experimental design is used to evaluate the effectiveness of teaching methods, educational interventions, and programs. This includes randomized controlled trials to compare different teaching methods or evaluate the impact of educational programs on student outcomes.
- Marketing : Experimental design is used to test the effectiveness of marketing campaigns, pricing strategies, and product designs. This includes experiments to test the impact of different marketing messages or pricing schemes on consumer behavior.
Examples of Experimental Design
Here are some examples of experimental design in different fields:
- Example in Medical research : A study that investigates the effectiveness of a new drug treatment for a particular condition. Patients are randomly assigned to either a treatment group or a control group, with the treatment group receiving the new drug and the control group receiving a placebo. The outcomes, such as improvement in symptoms or side effects, are measured and compared between the two groups.
- Example in Education research: A study that examines the impact of a new teaching method on student learning outcomes. Students are randomly assigned to either a group that receives the new teaching method or a group that receives the traditional teaching method. Student achievement is measured before and after the intervention, and the results are compared between the two groups.
- Example in Environmental science: A study that tests the effectiveness of a new method for reducing pollution in a river. Two sections of the river are selected, with one section treated with the new method and the other section left untreated. The water quality is measured before and after the intervention, and the results are compared between the two sections.
- Example in Marketing research: A study that investigates the impact of a new advertising campaign on consumer behavior. Participants are randomly assigned to either a group that is exposed to the new campaign or a group that is not. Their behavior, such as purchasing or product awareness, is measured and compared between the two groups.
- Example in Social psychology: A study that examines the effect of a new social intervention on reducing prejudice towards a marginalized group. Participants are randomly assigned to either a group that receives the intervention or a control group that does not. Their attitudes and behavior towards the marginalized group are measured before and after the intervention, and the results are compared between the two groups.
When to use Experimental Research Design
Experimental research design should be used when a researcher wants to establish a cause-and-effect relationship between variables. It is particularly useful when studying the impact of an intervention or treatment on a particular outcome.
Here are some situations where experimental research design may be appropriate:
- When studying the effects of a new drug or medical treatment: Experimental research design is commonly used in medical research to test the effectiveness and safety of new drugs or medical treatments. By randomly assigning patients to treatment and control groups, researchers can determine whether the treatment is effective in improving health outcomes.
- When evaluating the effectiveness of an educational intervention: An experimental research design can be used to evaluate the impact of a new teaching method or educational program on student learning outcomes. By randomly assigning students to treatment and control groups, researchers can determine whether the intervention is effective in improving academic performance.
- When testing the effectiveness of a marketing campaign: An experimental research design can be used to test the effectiveness of different marketing messages or strategies. By randomly assigning participants to treatment and control groups, researchers can determine whether the marketing campaign is effective in changing consumer behavior.
- When studying the effects of an environmental intervention: Experimental research design can be used to study the impact of environmental interventions, such as pollution reduction programs or conservation efforts. By randomly assigning locations or areas to treatment and control groups, researchers can determine whether the intervention is effective in improving environmental outcomes.
- When testing the effects of a new technology: An experimental research design can be used to test the effectiveness and safety of new technologies or engineering designs. By randomly assigning participants or locations to treatment and control groups, researchers can determine whether the new technology is effective in achieving its intended purpose.
How to Conduct Experimental Research
Here are the steps to conduct Experimental Research:
- Identify a Research Question : Start by identifying a research question that you want to answer through the experiment. The question should be clear, specific, and testable.
- Develop a Hypothesis: Based on your research question, develop a hypothesis that predicts the relationship between the independent and dependent variables. The hypothesis should be clear and testable.
- Design the Experiment : Determine the type of experimental design you will use, such as a between-subjects design or a within-subjects design. Also, decide on the experimental conditions, such as the number of independent variables, the levels of the independent variable, and the dependent variable to be measured.
- Select Participants: Select the participants who will take part in the experiment. They should be representative of the population you are interested in studying.
- Randomly Assign Participants to Groups: If you are using a between-subjects design, randomly assign participants to groups to control for individual differences.
- Conduct the Experiment : Conduct the experiment by manipulating the independent variable(s) and measuring the dependent variable(s) across the different conditions.
- Analyze the Data: Analyze the data using appropriate statistical methods to determine if there is a significant effect of the independent variable(s) on the dependent variable(s).
- Draw Conclusions: Based on the data analysis, draw conclusions about the relationship between the independent and dependent variables. If the results support the hypothesis, then it is accepted. If the results do not support the hypothesis, then it is rejected.
- Communicate the Results: Finally, communicate the results of the experiment through a research report or presentation. Include the purpose of the study, the methods used, the results obtained, and the conclusions drawn.
Purpose of Experimental Design
The purpose of experimental design is to control and manipulate one or more independent variables to determine their effect on a dependent variable. Experimental design allows researchers to systematically investigate causal relationships between variables, and to establish cause-and-effect relationships between the independent and dependent variables. Through experimental design, researchers can test hypotheses and make inferences about the population from which the sample was drawn.
Experimental design provides a structured approach to designing and conducting experiments, ensuring that the results are reliable and valid. By carefully controlling for extraneous variables that may affect the outcome of the study, experimental design allows researchers to isolate the effect of the independent variable(s) on the dependent variable(s), and to minimize the influence of other factors that may confound the results.
Experimental design also allows researchers to generalize their findings to the larger population from which the sample was drawn. By randomly selecting participants and using statistical techniques to analyze the data, researchers can make inferences about the larger population with a high degree of confidence.
Overall, the purpose of experimental design is to provide a rigorous, systematic, and scientific method for testing hypotheses and establishing cause-and-effect relationships between variables. Experimental design is a powerful tool for advancing scientific knowledge and informing evidence-based practice in various fields, including psychology, biology, medicine, engineering, and social sciences.
Advantages of Experimental Design
Experimental design offers several advantages in research. Here are some of the main advantages:
- Control over extraneous variables: Experimental design allows researchers to control for extraneous variables that may affect the outcome of the study. By manipulating the independent variable and holding all other variables constant, researchers can isolate the effect of the independent variable on the dependent variable.
- Establishing causality: Experimental design allows researchers to establish causality by manipulating the independent variable and observing its effect on the dependent variable. This allows researchers to determine whether changes in the independent variable cause changes in the dependent variable.
- Replication : Experimental design allows researchers to replicate their experiments to ensure that the findings are consistent and reliable. Replication is important for establishing the validity and generalizability of the findings.
- Random assignment: Experimental design often involves randomly assigning participants to conditions. This helps to ensure that individual differences between participants are evenly distributed across conditions, which increases the internal validity of the study.
- Precision : Experimental design allows researchers to measure variables with precision, which can increase the accuracy and reliability of the data.
- Generalizability : If the study is well-designed, experimental design can increase the generalizability of the findings. By controlling for extraneous variables and using random assignment, researchers can increase the likelihood that the findings will apply to other populations and contexts.
Limitations of Experimental Design
Experimental design has some limitations that researchers should be aware of. Here are some of the main limitations:
- Artificiality : Experimental design often involves creating artificial situations that may not reflect real-world situations. This can limit the external validity of the findings, or the extent to which the findings can be generalized to real-world settings.
- Ethical concerns: Some experimental designs may raise ethical concerns, particularly if they involve manipulating variables that could cause harm to participants or if they involve deception.
- Participant bias : Participants in experimental studies may modify their behavior in response to the experiment, which can lead to participant bias.
- Limited generalizability: The conditions of the experiment may not reflect the complexities of real-world situations. As a result, the findings may not be applicable to all populations and contexts.
- Cost and time : Experimental design can be expensive and time-consuming, particularly if the experiment requires specialized equipment or if the sample size is large.
- Researcher bias : Researchers may unintentionally bias the results of the experiment if they have expectations or preferences for certain outcomes.
- Lack of feasibility : Experimental design may not be feasible in some cases, particularly if the research question involves variables that cannot be manipulated or controlled.
About the author
Muhammad Hassan
Researcher, Academic Writer, Web developer
You may also like

Applied Research – Types, Methods and Examples

Descriptive Research Design – Types, Methods and...

Correlational Research – Methods, Types and...

Basic Research – Types, Methods and Examples

Research Methods – Types, Examples and Guide

Phenomenology – Methods, Examples and Guide
professional Online Experiments made easy
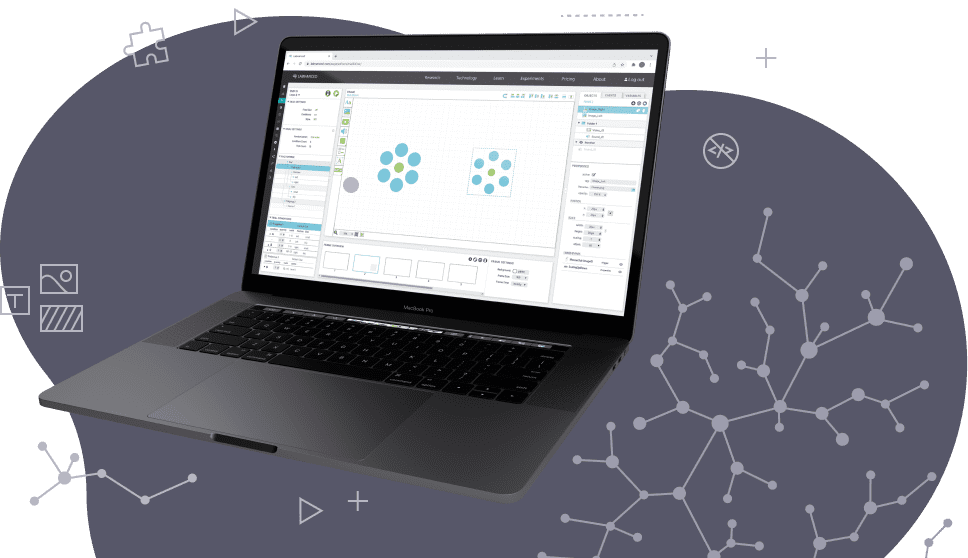
Supporting world-class research for:
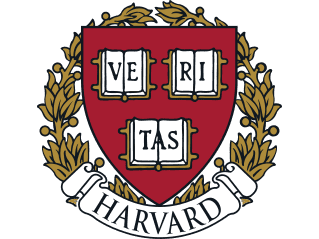
Harvard University
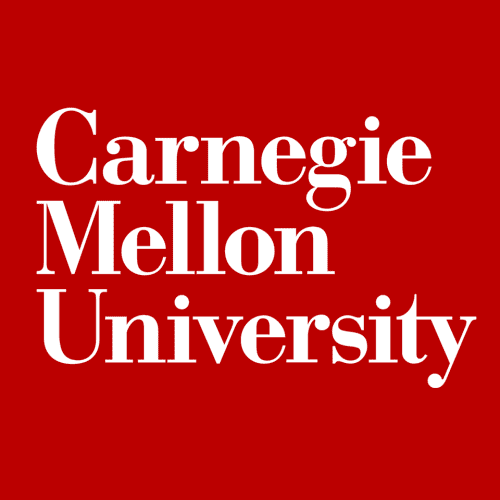
Carnegie Mellon University
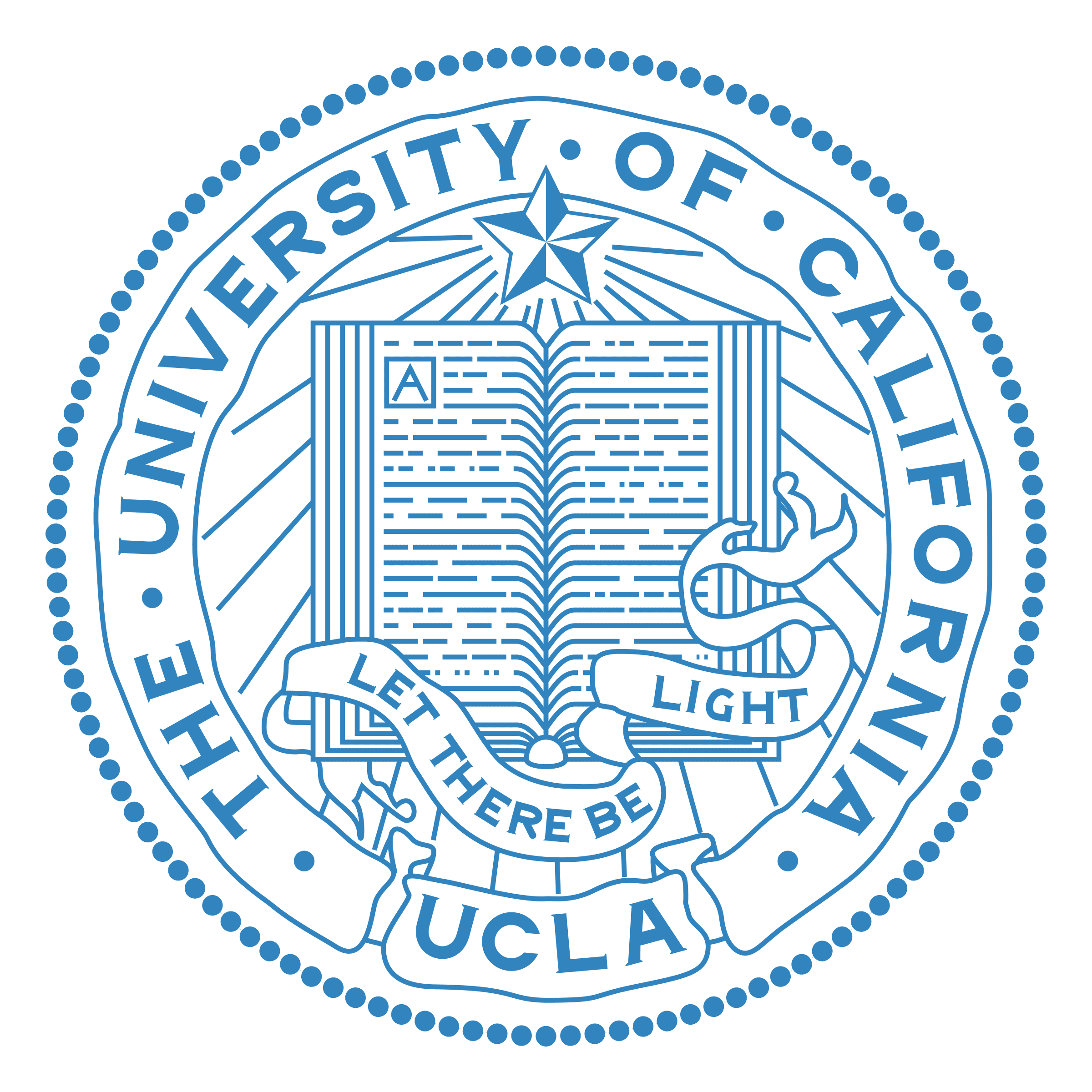
Max Planck Institute
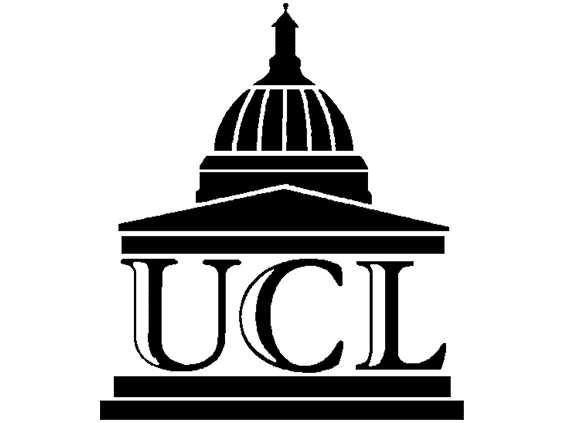
University College London
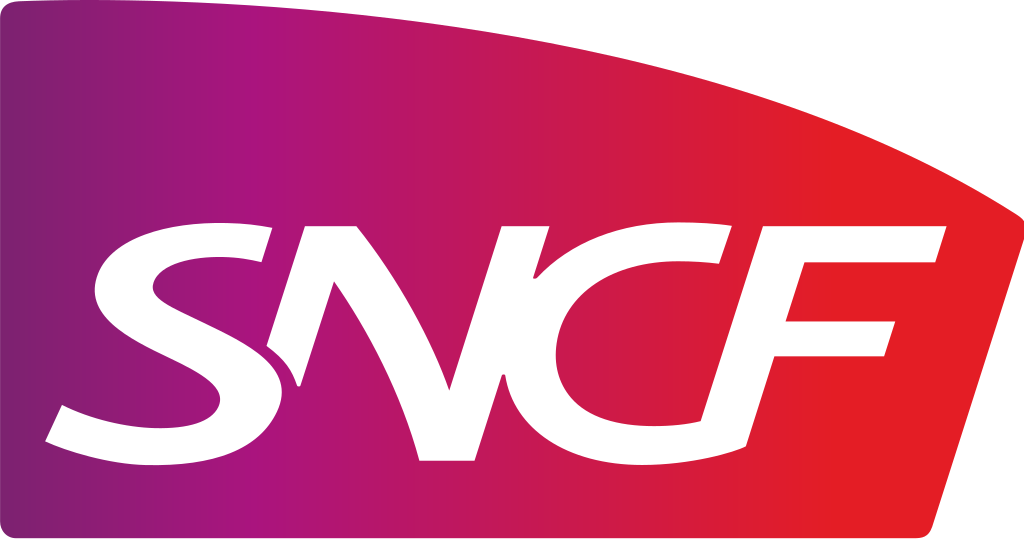
Have questions about your project or want a webinar?
Meet a Labvanced expert for free
- Request a Call
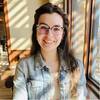
What is Labvanced?
Record Complex Behavior with Simple Design.
Intuitive Visual Editor
Building a state-of-the-art online experiment does not need to be difficult! With our editor you can visually create and run any experiment without needing to write a single line of code. The Labvanced experiment editor is built based on design principles from Experimental Psychology, which means you'll understand the system in a short time! All of your experiments structure is visible at a glance and you can play the current task to test how this looks for the participant.
Powerful Event System
The event system enables you to create powerful interactions and dynamics within your experiment. To define an event, you can listen for user input, variable changes, audio and video events, and more. Then, simply define the actions to determine what should happen: change stimuli, read or write variables, jump to a different part of the study, and many further options. Combining triggers and actions allows for infinite complexity with a simple to use interface.
Precise Reaction Times
Labvanced implements the current best-practices for recording reaction times in the most accurate way, But we don't stop there! In fact, we go beyond by using the intelligent caching of binary files, dynamic pre-rendering of all DOM content, frame onset correction based on requestAnimationFrame(), hardware performance tests, and passive recording of the JavaScript event-loop precision. Importantly, a 3rd party recently confirmed our superior methodology in this peer-reviewed publication.
Advanced Calibration Techniques
Our calibration measures go above and beyond what other online platforms offer. With Labvanced, you can determine much more regarding the experimental parameters and the participant's online environment. Our calibration techniques include measurements of physical screen size (inch/mm), distance to screen (inch/mm), screen refresh rate (hz), CPU and CPU performance (calc/sec), whether the participant is wearing headphones (yes/no), and much more. All these parameters can be either passively recorded or used as participant inclusion criteria.
Superior Eye-Tracking Technology
It is no overstatement to say that our eye-tracking technology (100% built by us) is by far the most accurate and capable webcam eye-tracking solution available on the market today. If you've only tried Webgazer or a Webgazer-based system so far, you will be surprised by the difference! Our solution finally offers researchers the powerful opportunity to go beyond standard behavioral measures for online experiments. Find out more here and make sure to subscribe to our newsletter, as our eye-tracking white paper will be published soon!
Flexible Audio, Video & Screen Recordings
If you aim to record precise audio or video files, using the webcam or the microphone of participants in a GDPR compliant way, then you can easily achieve that with our system. You can even record the whole screen of the participant if that matches your experimental requirements. For audio recordings we offer both uncompressed (.wav) and size efficient (.mp3) encoding formats, and all binary files can be end-to-end (PGP) encrypted for maximal data security and data privacy.
User-Friendly Longitudinal Studies
Longitudinal studies offer deep insights into the progress and performance of subjects over time. Labvanced has several built-in mechanisms to re-identify subjects in an anonymous way, i.e. using a self generated token, or using external identifiers such as the PROLIFIC_PID, provided by our partners at Prolific. Importantly, our system then sends out several reminder emails at customizable time slots to improve return rates in longitudinal designs.
Innovative Multi User Studies
Multi-user studies, often also called multiplayer studies, are studies in which 2 or more people perform a task together. Prisoner's Dilemma or the Public Goods Game are two standard examples here, but with our system you can go way beyond that by transferring any kind of data between subjects and building truly interactive tests or even games. Our system takes care of synchronizing state/variables between people automatically, so you can just focus on building the content.
Offline, Lab Application & API's
After you finish building the experiment, running the study online on our platform is only one out of several possibilities. For studies that need to run offline, we offer a lab/offline version. Also, using a local setup, you have the option of connecting Labvanced with external devices, such as EEGs, ECGs, GSRs, or eye-tracking hardware. A third option is to collect data online, but directly stream the data from the participant to your own server using our recording REST-API.
Built-in Translations
Labvanced serves customers from all over the world. Hence, there are multiple functions to translate and customize to language-specific requirements. We have automated translations for all of our 200+ system messages into 8 main languages. You can modify all of these translations to customize any message or even add a new language. Furthermore, you can also add translations for your own tasks so that people can choose their preferred language and get customized translations for all content provided.
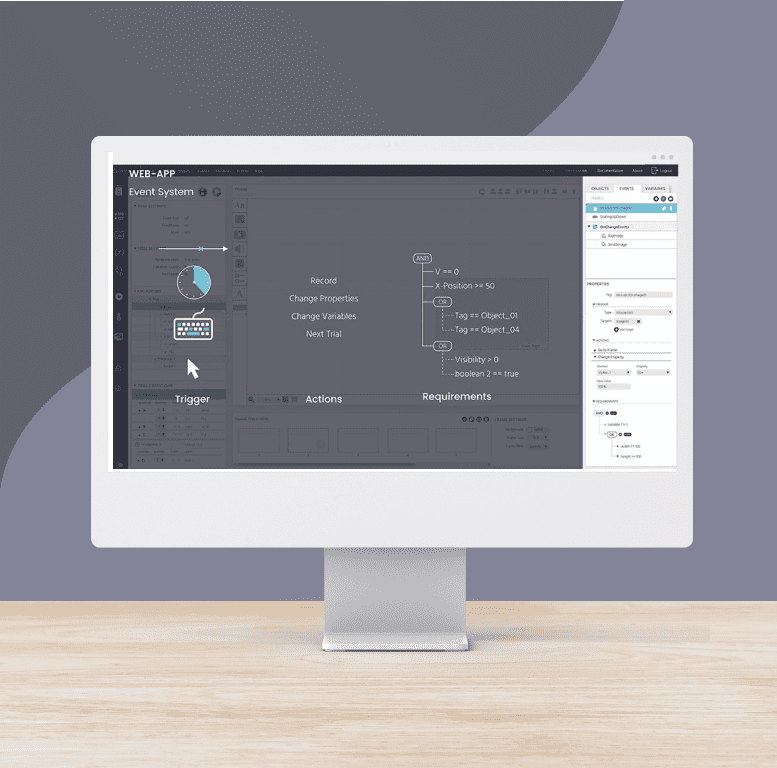
Labvanced in 3 Minutes
Testimonials
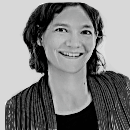
Dr. Katrin Starcke
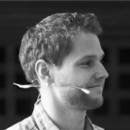
Dr. Malte Woestmann
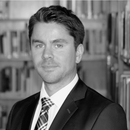
Prof. Alexander Edeling
Students love using Labvanced because of its user-friendliness, the great customer support we offer, and the time it saves them from learning a coding language. Joining the Labvanced community helps students get one step closer to completing their theses.
Single Researchers
From presenting stimuli to tracking reaction times and eye movements, Labvanced makes online studies faster and more insightful for professional researchers. This helps them to finish projects sooner and accelerate their careers.
Research Groups
Labvanced can greatly improve research and teaching of whole departments. Each researcher can run their own study or collaborate with other members during study creation. Also, Labvanced is a great tool to teach students experimental design in a hands-on fashion.
Behavioral Psychology
Cognitive & neuropsychology, developmental psychology, personality & social psychology, our customer support.
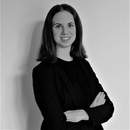
Dr. Mireia Marimon
Answers to your most important questions
Can I use Labvanced for free? Are there limitations?
Yes, you can use Labvanced completely for free but of course there are some limitations. Experiment creation will be unlimited and without any restrictions as long as you do not use more than 300MB of storage space. You can test-record as much as you want, but online recordings are limited to 10 subjects (data sets) per month. Further recordings will then be locked, but they can be unlocked for a fee. Joint experiment creation/collaboration and external data storage are not available in the free version, and study imports are limited to Labvanced studies/templates only. In short, you might be able to use it completely for free if you have more time (a few months) for the data collection or only need a very few subjects. Otherwise, the free version is a great way to familiarize yourself, before making a decision to buy a single or group license. There are also special discounts for Bachelor and Master students! Find out more about our pricing.
How can I be sure that the platform is capable of creating my experiment?
We are 99.99% sure that the answer to this question is YES it is possible because we have almost never seen a paradigm that cannot be realized with our system. Importantly, our Sample Studies Page provides you with over 30 complete paradigm studies, feature demos, and other resources that you can directly use/import to find out if your idea is workable with our system. Even more studies and resources are available in our Public Experiment Library, filled with hundreds of previous studies from other researchers. This will give you a good idea of the flexibility of Labvanced and the range of studies that are possible to create. If you have checked that and are still not sure if your idea can be accomplished with Labvanced, just reach out to us via the Live-Chat. We are always happy to help!
Can Labvanced staff help me create my study? What level of support can I expect?
First and foremost, we try to help every user be successful in building their study, irrespective of the license they have. That said, the main portion of study creation must (in most cases) be completely carried out by you. If you want us to build it for you, it will incur additional costs of 50 Euro/hour of work we put into it. Furthermore, it is important to mention that the general level of support we can provide also depends on your commitment to us, i.e. the type of license you have. We will try to help all users by answering the Live-Chat requests and emails, but at times when we are busy, we have to prioritize based on user licenses. For instance, for group license owners, we give free bi-yearly consultation sessions, and in general we try to fix problems for them by actively supervising studies and logging into their experiments if required. This cannot be done for all users at all times.
What makes Labvanced different from other online platforms?
The main strength of our platform is the simultaneous combination of user-friendliness and offering powerful technological features. Labvanced is built around concepts and terminology of experimental psychology, so it will be especially easy for psychologists to pick up on how things work. Furthermore, we are a team of technology enthusiasts, committed to pushing the boundaries of what kind of online experiments are possible. The best example of this is our self-built webcam eye-tracking technology, with an improvement of 300-400% in accuracy compared to existing technologies like Webgazer.
Where do I find publications/previous work using Labvanced? How can I cite Labvanced?
We try to stay up to date regarding people citing our platform, and we are nearing a total of 100 citations from peer-reviewed journals! However not every work is published and some people also forgot to cite us in the past. A recent overview of citations mentioning Labvanced can be found here (please let us know if we missed your study/publication on the list). There, you will also find a publication that can be used to cite us / Labvanced as a platform. Even more importantly, we are currently preparing two new manuscripts (expected publication dates are Q4 2022 and Q1 2023). These two important studies will review all of our platform's features and our cutting-edge eye-tracking technology, respectively. Stay tuned for more information coming soon!
Get a free license and use 10 recordings / data-sets per month.
Previous Grants and Sponsorships
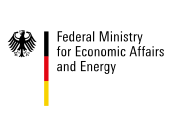
Want to get useful updates about new features and our roadmap?
Copyright © Scicovery GmbH 2024. All Rights Reserved
Critical Thinking in Science
Author: | Daniell DiFrancesca |
Level: | Middle School |
Content Area: | General Science |
Part 1: Introduction to Experimental Design
- Part 2: The Story of Pi
- Part 3: Experimenting with pH
- Part 4: Water Quality
- Part 5: Change Over Time
- Part 6: Cells
- Part 7: Microbiology and Infectious Disease
- About the Author
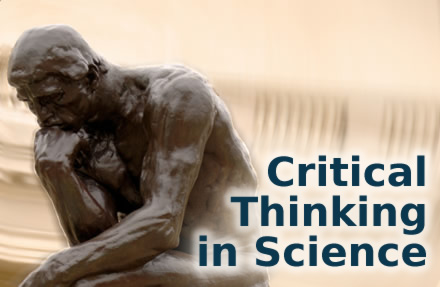
Introduction:
Students will learn and implement experimental design vocabulary while practicing their critical thinking skills in an inquiry based experiment. This lesson is written using the 5E Learning Model.
Learning Outcomes:
- Students will define and apply the experimental design vocabulary.
- Students will use the experimental design graphic organizer to plan an investigation.
- Students will design and complete their own scientific experiment.
Curriculum Alignment:
1.01 Identify and create questions and hypotheses that can be answered through scientific investigations.
1.02 Develop appropriate experimental procedures for:
- Given questions.
- Student generated questions.
1.04 Analyze variables in scientific investigations:
- Identify dependent and independent.
- Use of a control.
- Manipulate.
- Describe relationships between.
- Define operationally.
1.05 Analyze evidence to:
- Explain observations.
- Make inferences and predictions.
- Develop the relationship between evidence and explanation.
1.06 Use mathematics to gather, organize, and present quantitative data resulting from scientific investigations:
- Measurement.
- Analysis of data.
- Prediction models.
1.08 Use oral and written language to:
- Communicate findings.
- Defend conclusions of scientific investigations.
- Describe strengths and weaknesses of claims, arguments, and/or data
Classroom Time Required:
Approximately 6 class periods (~50 minutes each) are needed, however, some things can be assigned as homework to decrease the time spent in class.
Materials Needed:
- Overhead transparency of Experimental Design Graphic Organizer
- Student copies of: Experimental Design Graphic Organizer, Vocabulary Graphic Organizer, Explore worksheet, Explain worksheet
- Supplies for experiment: Dixie drinking cups, Pepsi and Coke (~1.5 to 2 ounces per student) Possibly ice to keep soda cold
- Copies of worksheet, Overhead, Small drinking cups (2 per student), Pepsi and Coke
- Dictionaries or definition sheets for the vocabulary words
Technology Resources:
- Overhead Projector
Pre-Activities/ Activities:
- What are the “rules” for designing an experiment?
- Teacher and class will discuss the following questions:
- Is there a specific way to design an experiment? (Try to get them to mention the scientific method and discuss any “holes” in this.
- Are their rules scientists follow when designing an experiment?
- Are all experiments designed the same?
- What kinds of experiments have you done on your own? (Good things to discuss are cooking, testing sports techniques, trying to fix things, etc….. Try to relate experimentation to their everyday life.)
- Review an experiment and answer questions.
- Students will read a description of an experiment and answer questions about the design of the experiment without using the vocabulary. (See Worksheet 1)
- Vocabulary introduction and application
- Students will define the experimental design vocabulary using the graphic organizer (See Worksheet 2).
- Independent variable, Dependent variable, Control, Constant, Hypothesis, Qualitative observation, Quantitative observation, Inference (Definitions available)
- Students will review the worksheet from the explore section and match the vocabulary to the pieces of the experiment. Review answers with the class.
- Students will read a second experiment description and identify the pieces of the experiment using their vocabulary definitions (See Worksheet 3).
- Introduce Experimental Design Graphic Organizer (EDGO) and complete class designed experiment.
- The teacher should review the EXAMPLE PEPSI VS COKE EDGO for any ideas or questions
- Use overhead projector to review the blank EDGO and complete as a class (See Worksheet 4)
- Tell the class that you are going to do the Pepsi Coke Challenge. The question they need to answer is: Can girls taste the difference between Pepsi and Coke better than the boys?
- As a class, plan the Pepsi verses Coke experiment. This is a good time to discuss double blind studies and why it is important to make this a double blind study. Students can look at the results within their own class as well as the whole team.
- This is a good chance to also test multiple variables. You do not need to let students know this, but if the data chart also records things like age, frequency of drinking soda (daily, weekly, monthly, rarely), ability to roll tongue, or anything else they think might be interested in, the results can be analyzed for each variable.
- I removed labels from the bottles and labeled them A and B. I used a different labeling system for under the cups so the students did not see a pattern (numbered cups were Pepsi, lettered cups were Coke).
- I recorded the data and organized the tasting while students completed other work in their seats. Two students at a time tasted the soda and I recorded data. You could also have a volunteer who is not participating help with this.
- Check for students who do not want to drink soda as well as any dietary needs such as diet soda.
- Do not verify guesses until all of the classes have completed the experiment.
- How can you accurately remember the pieces of an experiment?
- Write a poem about four of the vocabulary words.
- Write a song about four of the vocabulary words.
- Create a memorization tool for four of the vocabulary words.
- Make a poster about four of the vocabulary words.
Teachers should evaluate these choices to ensure they show an understanding of the vocabulary.
Assessment:
See Evaluate piece of Activities Section.
Modifications:
- A different experiment can be designed in the Elaborate section.
- EDGO can be edited for any motor skill deficiencies by making it larger, or making it available to be typed on.
- All basic modifications can be used for these activities.
Alternative Assessments:
- Make necessary adjustments for different experiments.
Critical Vocabulary
- Independent Variable- the part of the experiment that is controlled or changed by the experimenter
- Dependent Variable- the part of the experiment that is observed or measured to gather data; changes because of the independent variable
- Control- standard of comparison in the experiment; level of the independent variable that is left in the natural state, unchanged
- Constant- part of the experiment that is kept the same to avoid affecting the independent variable
- Hypothesis- educated guess or prediction about the experimental results
- Qualitative observation- word observations such as color or texture
- Quantitative observation- number observations or measurements
- Inference- attempt to explain the observations
This is the first lesson in the Critical Thinking in Science Unit. The other lessons continue using the vocabulary and Experimental Design Graphic Organizer while teaching the 8th grade content. Students are designing their own experiments to improve their ability to approach problems and questions scientifically. By developing their ability to reason through problems they are becoming critical thinkers.
Supplemental Files:

Address: Campus Box 7006. Raleigh, NC 27695 Telephone: 919.515.5118 Fax: 919.515.5831 E-Mail: [email protected]

- Skip to secondary menu
- Skip to main content
- Skip to primary sidebar
Statistics By Jim
Making statistics intuitive
Experimental Design: Definition and Types
By Jim Frost 3 Comments
What is Experimental Design?
An experimental design is a detailed plan for collecting and using data to identify causal relationships. Through careful planning, the design of experiments allows your data collection efforts to have a reasonable chance of detecting effects and testing hypotheses that answer your research questions.
An experiment is a data collection procedure that occurs in controlled conditions to identify and understand causal relationships between variables. Researchers can use many potential designs. The ultimate choice depends on their research question, resources, goals, and constraints. In some fields of study, researchers refer to experimental design as the design of experiments (DOE). Both terms are synonymous.

Ultimately, the design of experiments helps ensure that your procedures and data will evaluate your research question effectively. Without an experimental design, you might waste your efforts in a process that, for many potential reasons, can’t answer your research question. In short, it helps you trust your results.
Learn more about Independent and Dependent Variables .
Design of Experiments: Goals & Settings
Experiments occur in many settings, ranging from psychology, social sciences, medicine, physics, engineering, and industrial and service sectors. Typically, experimental goals are to discover a previously unknown effect , confirm a known effect, or test a hypothesis.
Effects represent causal relationships between variables. For example, in a medical experiment, does the new medicine cause an improvement in health outcomes? If so, the medicine has a causal effect on the outcome.
An experimental design’s focus depends on the subject area and can include the following goals:
- Understanding the relationships between variables.
- Identifying the variables that have the largest impact on the outcomes.
- Finding the input variable settings that produce an optimal result.
For example, psychologists have conducted experiments to understand how conformity affects decision-making. Sociologists have performed experiments to determine whether ethnicity affects the public reaction to staged bike thefts. These experiments map out the causal relationships between variables, and their primary goal is to understand the role of various factors.
Conversely, in a manufacturing environment, the researchers might use an experimental design to find the factors that most effectively improve their product’s strength, identify the optimal manufacturing settings, and do all that while accounting for various constraints. In short, a manufacturer’s goal is often to use experiments to improve their products cost-effectively.
In a medical experiment, the goal might be to quantify the medicine’s effect and find the optimum dosage.
Developing an Experimental Design
Developing an experimental design involves planning that maximizes the potential to collect data that is both trustworthy and able to detect causal relationships. Specifically, these studies aim to see effects when they exist in the population the researchers are studying, preferentially favor causal effects, isolate each factor’s true effect from potential confounders, and produce conclusions that you can generalize to the real world.
To accomplish these goals, experimental designs carefully manage data validity and reliability , and internal and external experimental validity. When your experiment is valid and reliable, you can expect your procedures and data to produce trustworthy results.
An excellent experimental design involves the following:
- Lots of preplanning.
- Developing experimental treatments.
- Determining how to assign subjects to treatment groups.
The remainder of this article focuses on how experimental designs incorporate these essential items to accomplish their research goals.
Learn more about Data Reliability vs. Validity and Internal and External Experimental Validity .
Preplanning, Defining, and Operationalizing for Design of Experiments

This phase of the design of experiments helps you identify critical variables, know how to measure them while ensuring reliability and validity, and understand the relationships between them. The review can also help you find ways to reduce sources of variability, which increases your ability to detect treatment effects. Notably, the literature review allows you to learn how similar studies designed their experiments and the challenges they faced.
Operationalizing a study involves taking your research question, using the background information you gathered, and formulating an actionable plan.
This process should produce a specific and testable hypothesis using data that you can reasonably collect given the resources available to the experiment.
- Null hypothesis : The jumping exercise intervention does not affect bone density.
- Alternative hypothesis : The jumping exercise intervention affects bone density.
To learn more about this early phase, read Five Steps for Conducting Scientific Studies with Statistical Analyses .
Formulating Treatments in Experimental Designs
In an experimental design, treatments are variables that the researchers control. They are the primary independent variables of interest. Researchers administer the treatment to the subjects or items in the experiment and want to know whether it causes changes in the outcome.
As the name implies, a treatment can be medical in nature, such as a new medicine or vaccine. But it’s a general term that applies to other things such as training programs, manufacturing settings, teaching methods, and types of fertilizers. I helped run an experiment where the treatment was a jumping exercise intervention that we hoped would increase bone density. All these treatment examples are things that potentially influence a measurable outcome.
Even when you know your treatment generally, you must carefully consider the amount. How large of a dose? If you’re comparing three different temperatures in a manufacturing process, how far apart are they? For my bone mineral density study, we had to determine how frequently the exercise sessions would occur and how long each lasted.
How you define the treatments in the design of experiments can affect your findings and the generalizability of your results.
Assigning Subjects to Experimental Groups
A crucial decision for all experimental designs is determining how researchers assign subjects to the experimental conditions—the treatment and control groups. The control group is often, but not always, the lack of a treatment. It serves as a basis for comparison by showing outcomes for subjects who don’t receive a treatment. Learn more about Control Groups .
How your experimental design assigns subjects to the groups affects how confident you can be that the findings represent true causal effects rather than mere correlation caused by confounders. Indeed, the assignment method influences how you control for confounding variables. This is the difference between correlation and causation .
Imagine a study finds that vitamin consumption correlates with better health outcomes. As a researcher, you want to be able to say that vitamin consumption causes the improvements. However, with the wrong experimental design, you might only be able to say there is an association. A confounder, and not the vitamins, might actually cause the health benefits.
Let’s explore some of the ways to assign subjects in design of experiments.
Completely Randomized Designs
A completely randomized experimental design randomly assigns all subjects to the treatment and control groups. You simply take each participant and use a random process to determine their group assignment. You can flip coins, roll a die, or use a computer. Randomized experiments must be prospective studies because they need to be able to control group assignment.
Random assignment in the design of experiments helps ensure that the groups are roughly equivalent at the beginning of the study. This equivalence at the start increases your confidence that any differences you see at the end were caused by the treatments. The randomization tends to equalize confounders between the experimental groups and, thereby, cancels out their effects, leaving only the treatment effects.
For example, in a vitamin study, the researchers can randomly assign participants to either the control or vitamin group. Because the groups are approximately equal when the experiment starts, if the health outcomes are different at the end of the study, the researchers can be confident that the vitamins caused those improvements.
Statisticians consider randomized experimental designs to be the best for identifying causal relationships.
If you can’t randomly assign subjects but want to draw causal conclusions about an intervention, consider using a quasi-experimental design .
Learn more about Randomized Controlled Trials and Random Assignment in Experiments .
Randomized Block Designs
Nuisance factors are variables that can affect the outcome, but they are not the researcher’s primary interest. Unfortunately, they can hide or distort the treatment results. When experimenters know about specific nuisance factors, they can use a randomized block design to minimize their impact.
This experimental design takes subjects with a shared “nuisance” characteristic and groups them into blocks. The participants in each block are then randomly assigned to the experimental groups. This process allows the experiment to control for known nuisance factors.
Blocking in the design of experiments reduces the impact of nuisance factors on experimental error. The analysis assesses the effects of the treatment within each block, which removes the variability between blocks. The result is that blocked experimental designs can reduce the impact of nuisance variables, increasing the ability to detect treatment effects accurately.
Suppose you’re testing various teaching methods. Because grade level likely affects educational outcomes, you might use grade level as a blocking factor. To use a randomized block design for this scenario, divide the participants by grade level and then randomly assign the members of each grade level to the experimental groups.
A standard guideline for an experimental design is to “Block what you can, randomize what you cannot.” Use blocking for a few primary nuisance factors. Then use random assignment to distribute the unblocked nuisance factors equally between the experimental conditions.
You can also use covariates to control nuisance factors. Learn about Covariates: Definition and Uses .
Observational Studies
In some experimental designs, randomly assigning subjects to the experimental conditions is impossible or unethical. The researchers simply can’t assign participants to the experimental groups. However, they can observe them in their natural groupings, measure the essential variables, and look for correlations. These observational studies are also known as quasi-experimental designs. Retrospective studies must be observational in nature because they look back at past events.
Imagine you’re studying the effects of depression on an activity. Clearly, you can’t randomly assign participants to the depression and control groups. But you can observe participants with and without depression and see how their task performance differs.
Observational studies let you perform research when you can’t control the treatment. However, quasi-experimental designs increase the problem of confounding variables. For this design of experiments, correlation does not necessarily imply causation. While special procedures can help control confounders in an observational study, you’re ultimately less confident that the results represent causal findings.
Learn more about Observational Studies .
For a good comparison, learn about the differences and tradeoffs between Observational Studies and Randomized Experiments .
Between-Subjects vs. Within-Subjects Experimental Designs
When you think of the design of experiments, you probably picture a treatment and control group. Researchers assign participants to only one of these groups, so each group contains entirely different subjects than the other groups. Analysts compare the groups at the end of the experiment. Statisticians refer to this method as a between-subjects, or independent measures, experimental design.
In a between-subjects design , you can have more than one treatment group, but each subject is exposed to only one condition, the control group or one of the treatment groups.
A potential downside to this approach is that differences between groups at the beginning can affect the results at the end. As you’ve read earlier, random assignment can reduce those differences, but it is imperfect. There will always be some variability between the groups.
In a within-subjects experimental design , also known as repeated measures, subjects experience all treatment conditions and are measured for each. Each subject acts as their own control, which reduces variability and increases the statistical power to detect effects.
In this experimental design, you minimize pre-existing differences between the experimental conditions because they all contain the same subjects. However, the order of treatments can affect the results. Beware of practice and fatigue effects. Learn more about Repeated Measures Designs .
Assigned to one experimental condition | Participates in all experimental conditions |
Requires more subjects | Fewer subjects |
Differences between subjects in the groups can affect the results | Uses same subjects in all conditions. |
No order of treatment effects. | Order of treatments can affect results. |
Design of Experiments Examples
For example, a bone density study has three experimental groups—a control group, a stretching exercise group, and a jumping exercise group.
In a between-subjects experimental design, scientists randomly assign each participant to one of the three groups.
In a within-subjects design, all subjects experience the three conditions sequentially while the researchers measure bone density repeatedly. The procedure can switch the order of treatments for the participants to help reduce order effects.
Matched Pairs Experimental Design
A matched pairs experimental design is a between-subjects study that uses pairs of similar subjects. Researchers use this approach to reduce pre-existing differences between experimental groups. It’s yet another design of experiments method for reducing sources of variability.
Researchers identify variables likely to affect the outcome, such as demographics. When they pick a subject with a set of characteristics, they try to locate another participant with similar attributes to create a matched pair. Scientists randomly assign one member of a pair to the treatment group and the other to the control group.
On the plus side, this process creates two similar groups, and it doesn’t create treatment order effects. While matched pairs do not produce the perfectly matched groups of a within-subjects design (which uses the same subjects in all conditions), it aims to reduce variability between groups relative to a between-subjects study.
On the downside, finding matched pairs is very time-consuming. Additionally, if one member of a matched pair drops out, the other subject must leave the study too.
Learn more about Matched Pairs Design: Uses & Examples .
Another consideration is whether you’ll use a cross-sectional design (one point in time) or use a longitudinal study to track changes over time .
A case study is a research method that often serves as a precursor to a more rigorous experimental design by identifying research questions, variables, and hypotheses to test. Learn more about What is a Case Study? Definition & Examples .
In conclusion, the design of experiments is extremely sensitive to subject area concerns and the time and resources available to the researchers. Developing a suitable experimental design requires balancing a multitude of considerations. A successful design is necessary to obtain trustworthy answers to your research question and to have a reasonable chance of detecting treatment effects when they exist.
Share this:

Reader Interactions
March 23, 2024 at 2:35 pm
Dear Jim You wrote a superb document, I will use it in my Buistatistics course, along with your three books. Thank you very much! Miguel
March 23, 2024 at 5:43 pm
Thanks so much, Miguel! Glad this post was helpful and I trust the books will be as well.
April 10, 2023 at 4:36 am
What are the purpose and uses of experimental research design?
Comments and Questions Cancel reply
Experimental Design: Types, Examples & Methods
Saul McLeod, PhD
Editor-in-Chief for Simply Psychology
BSc (Hons) Psychology, MRes, PhD, University of Manchester
Saul McLeod, PhD., is a qualified psychology teacher with over 18 years of experience in further and higher education. He has been published in peer-reviewed journals, including the Journal of Clinical Psychology.
Learn about our Editorial Process
Olivia Guy-Evans, MSc
Associate Editor for Simply Psychology
BSc (Hons) Psychology, MSc Psychology of Education
Olivia Guy-Evans is a writer and associate editor for Simply Psychology. She has previously worked in healthcare and educational sectors.
On This Page:
Experimental design refers to how participants are allocated to different groups in an experiment. Types of design include repeated measures, independent groups, and matched pairs designs.
Probably the most common way to design an experiment in psychology is to divide the participants into two groups, the experimental group and the control group, and then introduce a change to the experimental group, not the control group.
The researcher must decide how he/she will allocate their sample to the different experimental groups. For example, if there are 10 participants, will all 10 participants participate in both groups (e.g., repeated measures), or will the participants be split in half and take part in only one group each?
Three types of experimental designs are commonly used:
1. Independent Measures
Independent measures design, also known as between-groups , is an experimental design where different participants are used in each condition of the independent variable. This means that each condition of the experiment includes a different group of participants.
This should be done by random allocation, ensuring that each participant has an equal chance of being assigned to one group.
Independent measures involve using two separate groups of participants, one in each condition. For example:
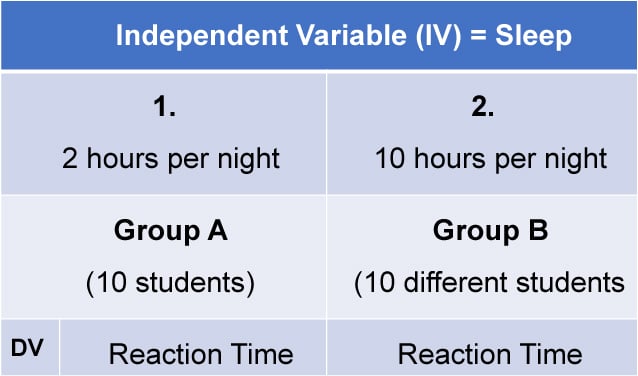
- Con : More people are needed than with the repeated measures design (i.e., more time-consuming).
- Pro : Avoids order effects (such as practice or fatigue) as people participate in one condition only. If a person is involved in several conditions, they may become bored, tired, and fed up by the time they come to the second condition or become wise to the requirements of the experiment!
- Con : Differences between participants in the groups may affect results, for example, variations in age, gender, or social background. These differences are known as participant variables (i.e., a type of extraneous variable ).
- Control : After the participants have been recruited, they should be randomly assigned to their groups. This should ensure the groups are similar, on average (reducing participant variables).
2. Repeated Measures Design
Repeated Measures design is an experimental design where the same participants participate in each independent variable condition. This means that each experiment condition includes the same group of participants.
Repeated Measures design is also known as within-groups or within-subjects design .
- Pro : As the same participants are used in each condition, participant variables (i.e., individual differences) are reduced.
- Con : There may be order effects. Order effects refer to the order of the conditions affecting the participants’ behavior. Performance in the second condition may be better because the participants know what to do (i.e., practice effect). Or their performance might be worse in the second condition because they are tired (i.e., fatigue effect). This limitation can be controlled using counterbalancing.
- Pro : Fewer people are needed as they participate in all conditions (i.e., saves time).
- Control : To combat order effects, the researcher counter-balances the order of the conditions for the participants. Alternating the order in which participants perform in different conditions of an experiment.
Counterbalancing
Suppose we used a repeated measures design in which all of the participants first learned words in “loud noise” and then learned them in “no noise.”
We expect the participants to learn better in “no noise” because of order effects, such as practice. However, a researcher can control for order effects using counterbalancing.
The sample would be split into two groups: experimental (A) and control (B). For example, group 1 does ‘A’ then ‘B,’ and group 2 does ‘B’ then ‘A.’ This is to eliminate order effects.
Although order effects occur for each participant, they balance each other out in the results because they occur equally in both groups.
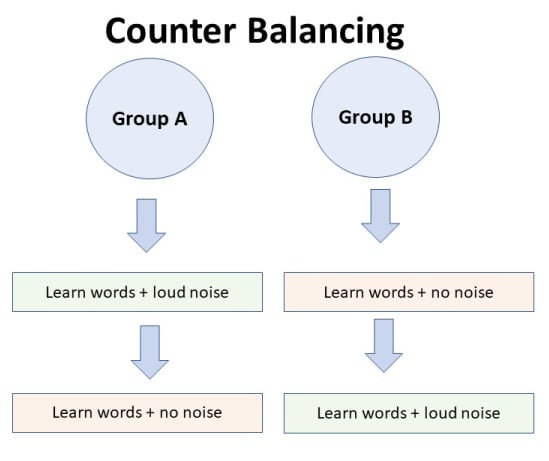
3. Matched Pairs Design
A matched pairs design is an experimental design where pairs of participants are matched in terms of key variables, such as age or socioeconomic status. One member of each pair is then placed into the experimental group and the other member into the control group .
One member of each matched pair must be randomly assigned to the experimental group and the other to the control group.
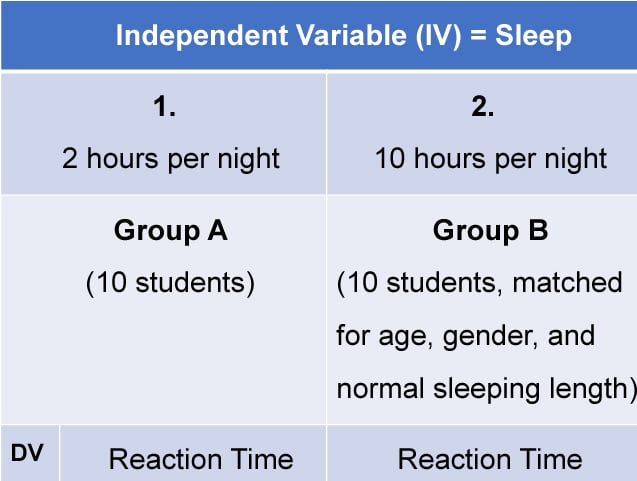
- Con : If one participant drops out, you lose 2 PPs’ data.
- Pro : Reduces participant variables because the researcher has tried to pair up the participants so that each condition has people with similar abilities and characteristics.
- Con : Very time-consuming trying to find closely matched pairs.
- Pro : It avoids order effects, so counterbalancing is not necessary.
- Con : Impossible to match people exactly unless they are identical twins!
- Control : Members of each pair should be randomly assigned to conditions. However, this does not solve all these problems.
Experimental design refers to how participants are allocated to an experiment’s different conditions (or IV levels). There are three types:
1. Independent measures / between-groups : Different participants are used in each condition of the independent variable.
2. Repeated measures /within groups : The same participants take part in each condition of the independent variable.
3. Matched pairs : Each condition uses different participants, but they are matched in terms of important characteristics, e.g., gender, age, intelligence, etc.
Learning Check
Read about each of the experiments below. For each experiment, identify (1) which experimental design was used; and (2) why the researcher might have used that design.
1 . To compare the effectiveness of two different types of therapy for depression, depressed patients were assigned to receive either cognitive therapy or behavior therapy for a 12-week period.
The researchers attempted to ensure that the patients in the two groups had similar severity of depressed symptoms by administering a standardized test of depression to each participant, then pairing them according to the severity of their symptoms.
2 . To assess the difference in reading comprehension between 7 and 9-year-olds, a researcher recruited each group from a local primary school. They were given the same passage of text to read and then asked a series of questions to assess their understanding.
3 . To assess the effectiveness of two different ways of teaching reading, a group of 5-year-olds was recruited from a primary school. Their level of reading ability was assessed, and then they were taught using scheme one for 20 weeks.
At the end of this period, their reading was reassessed, and a reading improvement score was calculated. They were then taught using scheme two for a further 20 weeks, and another reading improvement score for this period was calculated. The reading improvement scores for each child were then compared.
4 . To assess the effect of the organization on recall, a researcher randomly assigned student volunteers to two conditions.
Condition one attempted to recall a list of words that were organized into meaningful categories; condition two attempted to recall the same words, randomly grouped on the page.
Experiment Terminology
Ecological validity.
The degree to which an investigation represents real-life experiences.
Experimenter effects
These are the ways that the experimenter can accidentally influence the participant through their appearance or behavior.
Demand characteristics
The clues in an experiment lead the participants to think they know what the researcher is looking for (e.g., the experimenter’s body language).
Independent variable (IV)
The variable the experimenter manipulates (i.e., changes) is assumed to have a direct effect on the dependent variable.
Dependent variable (DV)
Variable the experimenter measures. This is the outcome (i.e., the result) of a study.
Extraneous variables (EV)
All variables which are not independent variables but could affect the results (DV) of the experiment. Extraneous variables should be controlled where possible.
Confounding variables
Variable(s) that have affected the results (DV), apart from the IV. A confounding variable could be an extraneous variable that has not been controlled.
Random Allocation
Randomly allocating participants to independent variable conditions means that all participants should have an equal chance of taking part in each condition.
The principle of random allocation is to avoid bias in how the experiment is carried out and limit the effects of participant variables.
Order effects
Changes in participants’ performance due to their repeating the same or similar test more than once. Examples of order effects include:
(i) practice effect: an improvement in performance on a task due to repetition, for example, because of familiarity with the task;
(ii) fatigue effect: a decrease in performance of a task due to repetition, for example, because of boredom or tiredness.

TOC Help Line
Everything you need to survive substitute teaching.
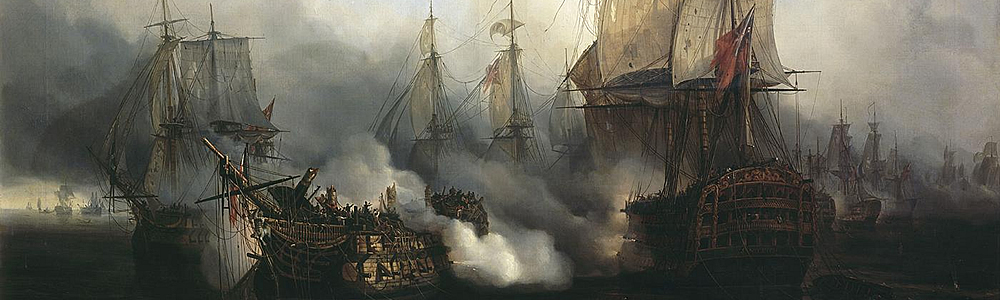
Experimental Design Activities
These series of activities are designed for students to collaborate together to come up with something unique and interesting. For many of the experimental questions, students will work in pairs or groups to come up with their own experimental design. If the school has resources and there is enough time, students can test their designs. If students are not able to make and test their designs, they can draw and explain what their ideas are to the class or hand it in if you wish to look at their ideas. Students are encouraged to use the scientific method when coming up with their experimental designs.
See how Sheldon and Leonard use the scientific method:
Download the full document with descriptions here: Experimental Design Activities
Experiment 1: Egg Drop
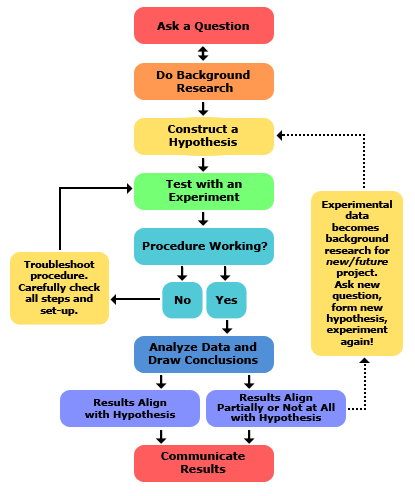
The Scientific Method: obtained from www.sciencebuddies.org
Experimental question: What attire will you design in order to prevent an egg from breaking if dropped from a 2-3 story building?
Questions to consider:
- What materials will you use?
- How will you ensure the egg does not break if dropped off a roof top?
- How will you test and re-test your design?
Ask students to write their thought process in terms of the scientific method (research, hypothesis, test hypothesis, re-evaluate, conclude).
Students should lay out exactly how they plan to test their design. Students should also draw a picture of their design and mention why they think it will be so effective.
Material limitations:
- 6 cotton balls
- 3 ft of tape
- 2 sheets of paper towel
- 4 popsicle sticks
- How will you use the materials?
- What design will allow you to use the materials most effectively and wisely?
- How will you re-test if the first design is not successful?
Note: many of these materials are very inexpensive and the school may have them. If you wish, you might be able to come prepared with a bin of materials and have students test out some designs.
Take a look at how the Mythbusters take on the egg drop challenge!
Experiment 2: Mold Test
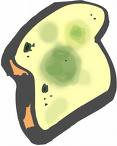
Experimental question: What conditions are best for keeping food fresh?
Design an experiment to show which conditions are best for keeping bread fresh. (ie. does bread stay fresher in cold temperatures, darkness, moist environments, etc.)
- What will your control be?
- What are your independent and dependent variable?
- How many different conditions will you test? What will these variables be?
- From experience, what do you notice about the freshness of your food? Where is it stored?
Download the full version below!
Experiment 3: Cargo Ship
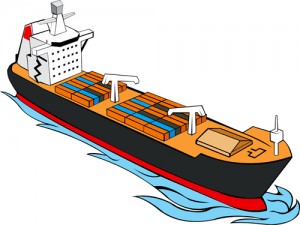
Experimental question: What design of boat will hold the most amount of pennies?
Design a style of boat using limited materials that will hold the moat amount of pennies when placed in water.
- One sheet of aluminum foil (approx 12 inches long and 6 inches wide)
- 1ft of tape
- 6 popsicle sticks
- 2 small birthday candles (optional)
Note: you may alter the materials as necessary, adding more or removing, or changing the current materials listed
Instructions:
- Students get into groups of 4 or 5
- Students use materials to design a boat that will hold as many pennies as possible without sinking
- Students can use as little or as much of the provided material as needed
- Give students 30 minutes to design their boat
- Have groups test their designs against other groups – fill up a sink with water, place two boats in the sink or water bath, place one penny at a time in the boat until one of them finally sinks
Download: Experimental Design Activities
Leave a Reply Cancel reply
Your email address will not be published. Required fields are marked *
Spam prevention powered by Akismet
This short, targeted simulation is adapted from the full-length “Experimental Design” simulation.
How do research scientists know where to begin? In this simulation, you will learn how to design a scientific experiment. As a pharmaceutical detective, you have the chance to perform experiments with human volunteers, animals, and living human cells. Make sure that you choose the correct experimental model to design a scientifically sound experiment for testing the effect of the suspicious drug.
The scientific method
Humans have always strived to explain natural phenomena. In the first mission of the experimental design simulation, you will learn how to use the scientific method to investigate phenomena, acquire new knowledge, or correct and integrate existing knowledge. Every tool you need to use is available in this virtual lab.
Design an experiment and formulate a hypothesis
In your next mission you have the freedom to design your own experiment from scratch while examining biopsies under a microscope. Define your scientific question and choose the right model to test your hypothesis. And don’t be afraid to make mistakes - in this virtual simulation you can repeat the experiment as many times as you want.
Experimental controls
You will notice that there are many experimental variables in your experiment. Learn how to adjust them and understand why it’s so important to use experimental controls to verify your results. These controls will let you conclude whether a suspicious compound is the reason behind the reported epidemic. You’ll get to investigate the effect of different compounds and various concentrations of the same compound.
Will you be able to set up an experiment that can prove your suspicions?
The Scientific Method | Virtual Lab
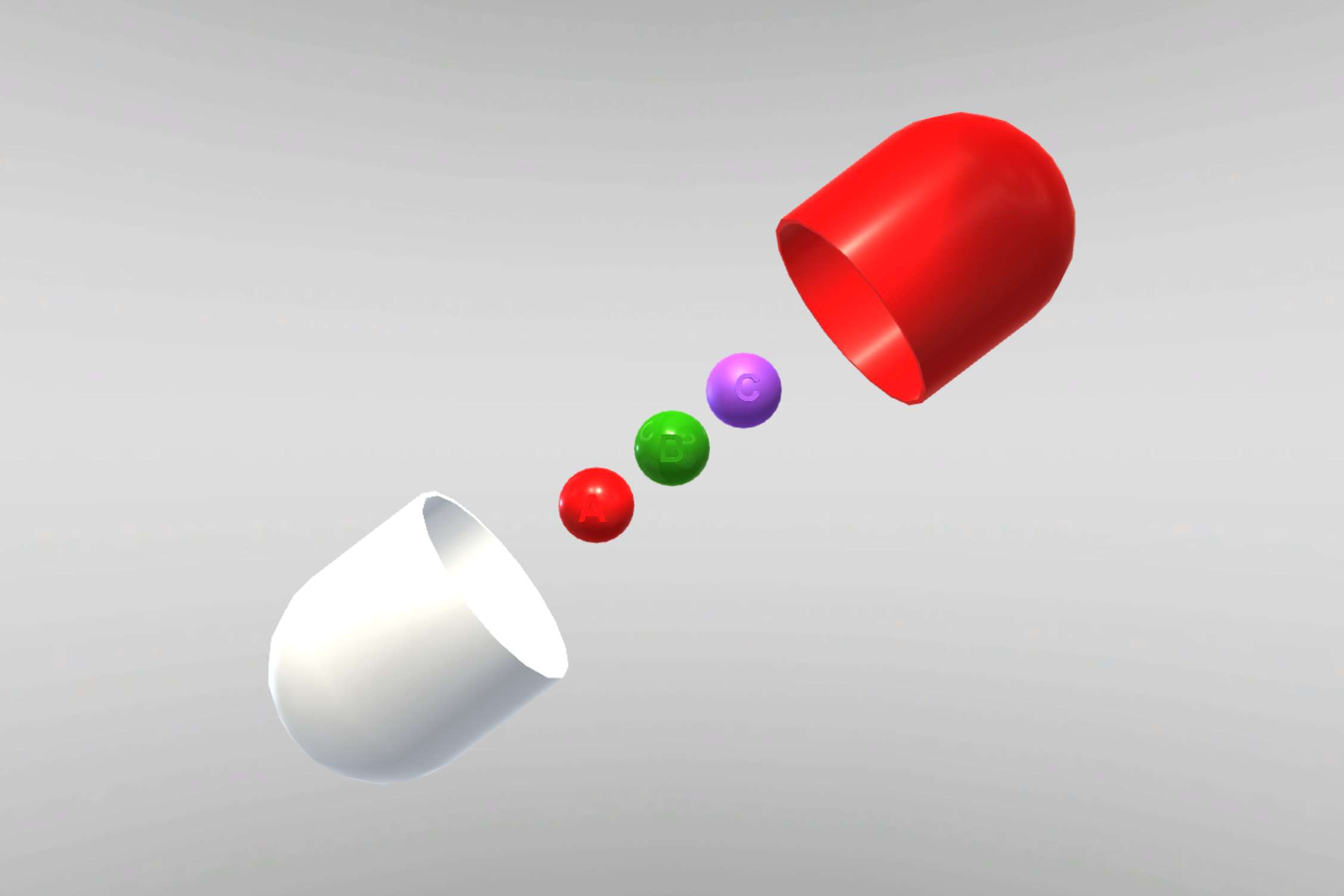
Lorem ipsum dolor sit amet, consectetur adipiscing elit, sed do eiusmod tempor incididunt ut labore et dolore magna aliqua. Ut enim ad minim veniam, quis nostrud exercitation ullamco laboris nisi ut aliquip ex ea commodo consequat. Duis aute irure dolor in reprehenderit in voluptate velit esse cillum dolore eu fugiat nulla pariatur.
Block quote
Ordered list
Unordered list
Superscript
About This Simulation
Work as a pharmaceutical detective to identify the link between a new drug and a recent epidemic. Use the scientific method to come up with a hypothesis and subsequently design an experiment that will test the validity of your hypothesis.
Learning Objectives
- Describe the scientific method
- Know what to consider when choosing an experimental model
- Design an experiment and test a hypothesis
- Select the correct experimental controls to verify your hypothesis
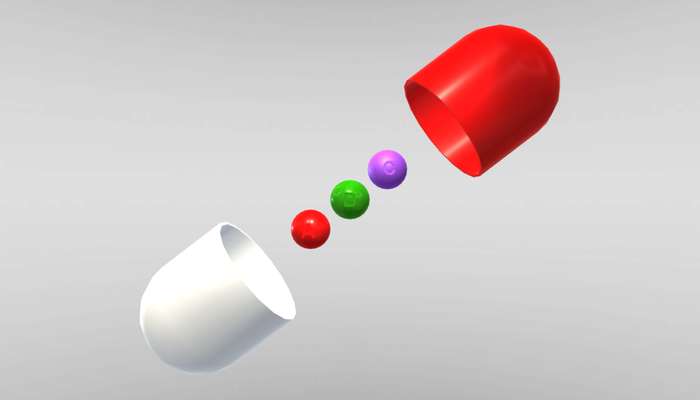
Lab Techniques
- Apoptosis assay
Related Standards
- Generic experimental design support
Learn More About This Simulation
Experience labster for yourself, boost learning with fun.
75% of students show high engagement and improved grades with Labster
Discover Simulations That Match Your Syllabus
Easily bolster your learning objectives with relevant, interactive content
Place Students in the Shoes of Real Scientists
Practice a lab procedure or visualize theory through narrative-driven scenarios
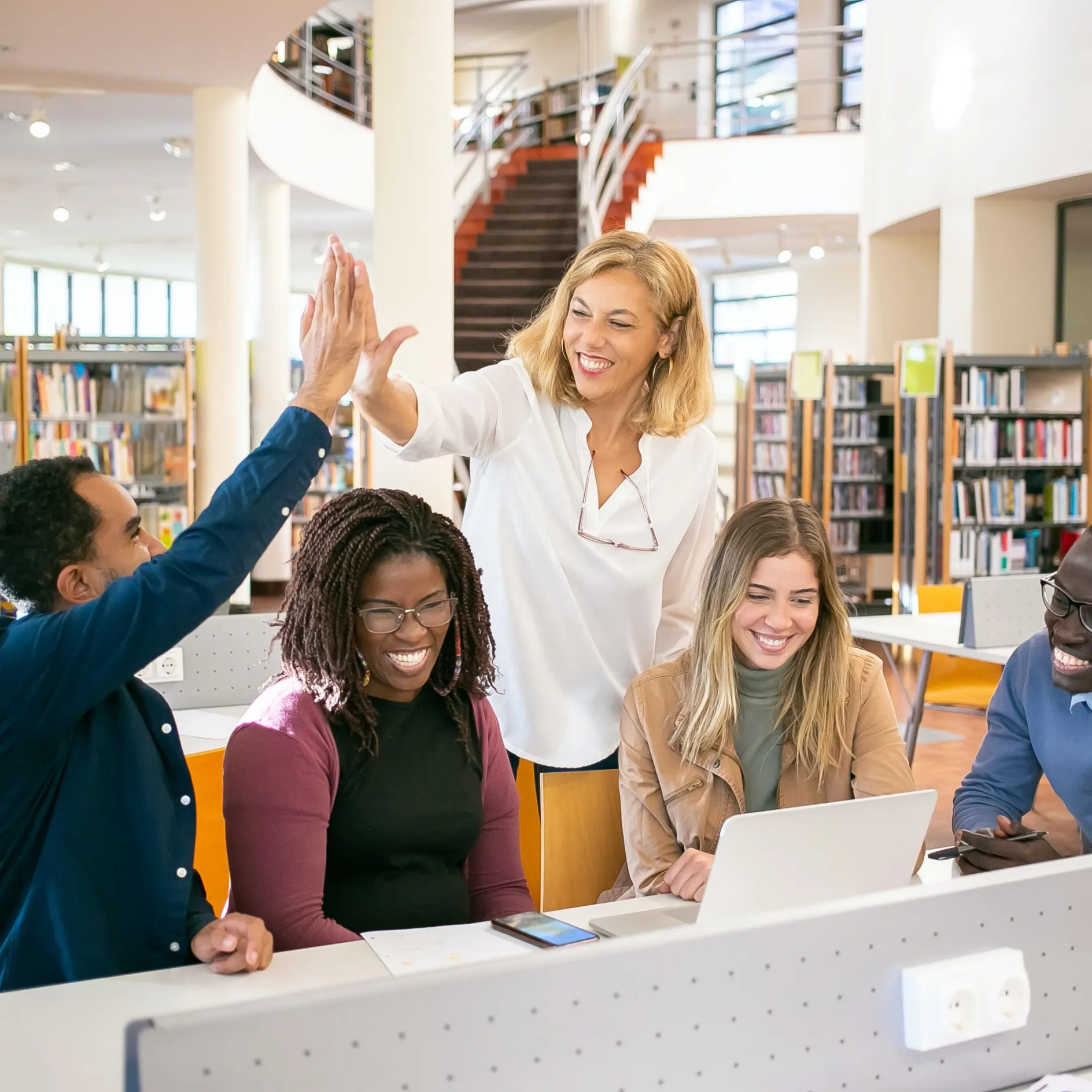
Related Course
High school chemistry, introduction to biology, food science and nutrition, +20 other courses, related simulations.
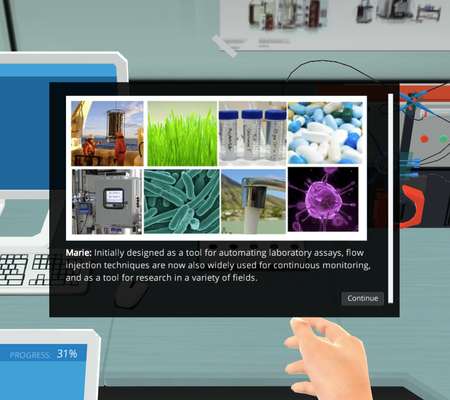
Flow Injection Analysis
Let’s awaken your curiosity for the chemistry of caffeine. Learn how Flow Injection Analysis (FIA) helps scientists measure the concentration of caffeine in your drinks.
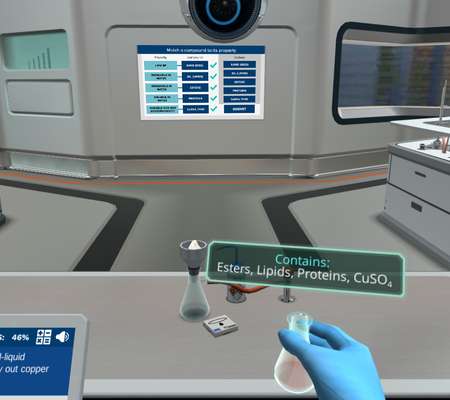
Purification and Separation of a Mixture: Test your analytical chemistry skills
In this simulation, you will learn how to use a range of techniques to separate compounds from a mixture. Using their individual properties, develop and execute an experimental procedure to separate out each compound.
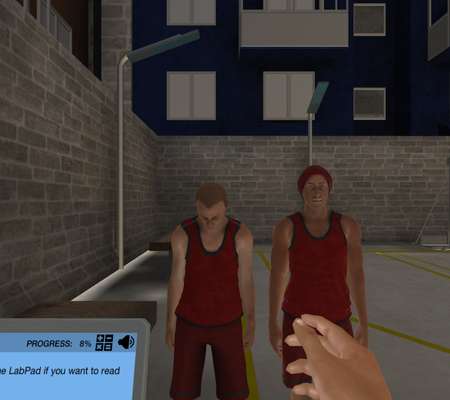
Cellular Respiration: Glycolysis
Help the basketball players understand how the food they eat gets converted to energy by investigating glycolysis, the first stage of cellular respiration.
For Science Programs Providing a Learning Advantage

“They did the simulation at home, then completed the in-person lab within 30 minutes, no questions asked, and passed the quiz with flying colors.”
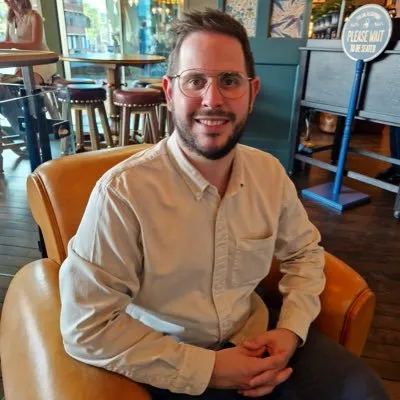
"I saw some of the students who clearly didn’t necessarily like sitting there reading a book discover they could turn on Labster and keep up with the rest of the class because it spoke to them.
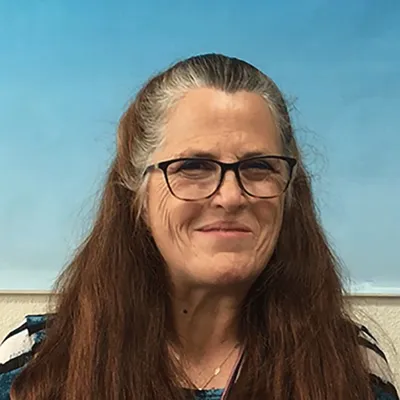
"Having something that's engaging for the students gives teachers that opportunity to breathe and get excited again. Because they're seeing the kids light up, they're seeing the kids engage with content."
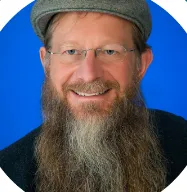
"The question always is, ‘Can we demonstrate that the students are meeting course outcomes?’ Check! We can do that.”

"We surveyed over 400 students. More than 90% thought Labster was easy to navigate, and that it was fun, but more importantly, most of them felt confident that they could execute the labs in person. And that confidence is a big deal."

“The Labster simulations get students to do things, and they're not just sitting there consuming a webinar where their mind can drift. They become an active participant in that learning experience.”
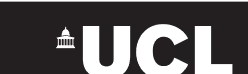
Find answers to frequently asked questions.
Labster can be integrated within a school's LMS (Learning Management System), and students can access it like any other assignment in their LMS. If your Institution does not choose an LMS integration, students will log in to Labster's Course Manager once they have an account created. Your institution will decide the access method during the sales process.
Labster is only available for purchase by faculty and administration at academic institutions. To procure Labster, simply reach out to us on our website. Schedule a demo, book a meeting to discuss pricing, start a free trial, or simply fill out our contact form.
Labster simulations are created by real scientists and designed with unparalleled interactivity. Unlike point and click competitors, Labster simulations immerse students and encourage mastery through active learning.
Labster supports a wide range of courses at the high school and university level across fields in biology, chemistry and physics. Some simulations mimic lab procedures with high fidelity to train foundational skills, while others are meant to bring theory to life through interactive scenarios.
Thank you for visiting nature.com. You are using a browser version with limited support for CSS. To obtain the best experience, we recommend you use a more up to date browser (or turn off compatibility mode in Internet Explorer). In the meantime, to ensure continued support, we are displaying the site without styles and JavaScript.
- View all journals
- Explore content
- About the journal
- Publish with us
- Sign up for alerts
- Open access
- Published: 18 September 2024
UPLC-PDA factorial design assisted method for simultaneous determination of oseltamivir, dexamethasone, and remdesivir in human plasma
- Hanan I. EL-Shorbagy 1 ,
- Mona A. Mohamed 2 ,
- Alaa El-Gindy 1 ,
- Ghada M. Hadad 1 &
- Fathalla Belal 3
Scientific Reports volume 14 , Article number: 21758 ( 2024 ) Cite this article
1 Altmetric
Metrics details
- Bioanalytical chemistry
- Environmental sciences
A green and simple UPLC method was developed and optimized, adopting a factorial design for simultaneous determination of oseltamivir phosphate and remdesivir with dexamethasone as a co-administered drug in human plasma and using daclatasvir dihydrochloride as an internal standard within 5 min. The separation was established on UPLC column BEH C 18 1.7 μm (2.1 \(\times \) 100.0 mm) connected to UPLC pre-column BEH 1.7 μm (2.1 \(\times \) 5.0 mm) at 50 °C with an injection volume of 10 μL. The photodiode array detector (PDA) was set at three wavelengths of 220, 315, and 245 nm for oseltamivir phosphate, the internal standard, and both dexamethasone and remdesivir, respectively. The mobile phase consisted of methanol and ammonium acetate solution (40 mM) adjusted to pH 4 in a ratio of 61.5:38.5 (v/v) with a flow rate of 0.25 mL min −1 . The calibration curves were linear over 500.0–5000.0 ng mL −1 for oseltamivir phosphate, over 10.0–500.0 ng mL −1 and 500.0–5000.0 ng mL −1 for dexamethasone, and over 20.0–500 ng mL −1 and 500.0–5000.0 ng mL −1 for remdesivir. The Gibbs free energy and Van't Hoff plots were used to investigate the effect of column oven temperatures on retention times. Fluoride-EDTA anticoagulant showed inhibition activity on the esterase enzyme in plasma. The proposed method was validated according to the M10 ICH, FDA, and EMA’s bioanalytical guidelines. According to Eco-score, GAPI, and AGREE criteria, the proposed method was considered acceptable green.
Similar content being viewed by others
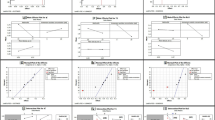
Development of UPLC method for simultaneous assay of some COVID-19 drugs utilizing novel instrumental standard addition and factorial design
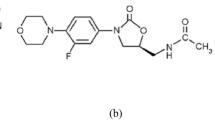
An eco-friendly and cost-effective HPTLC method for quantification of COVID-19 antiviral drug and co-administered medications in spiked human plasma
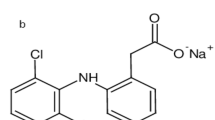
Green UPLC method for estimation of ciprofloxacin, diclofenac sodium, and ibuprofen with application to pharmacokinetic study of human samples
Introduction.
COVID-19 created a serious threat to economies and healthcare systems. SARS-CoV-2 viruses, which are encapsulated viruses with a single-stranded RNA genome, are the main cause of COVID-19 1 , 2 . In the management protocol for COVID-19, oseltamivir phosphate (OSTP) (Fig. S1 A) is indicated for mild cases, while remdesivir (REM) (Fig. S1 C) is indicated for moderate-to-severe cases 3 , 4 , 5 , 6 , 7 , 8 , 9 , 10 . It was indicated that dexamethasone (DEX) (Fig. S1 B), as an anti-infamatory glucocorticoid, is coadministered with OSTP or REM in COVID-19 viral infections. The U.S. Food and Drug Administration (FDA) authorized OSTP in August 2016 11 . It stops viral budding from the host cell by inhibiting the neuraminidase enzyme on the surface of the virus. It is used to both treat and prevent influenza virus infections. The FDA authorized REM for the treatment of COVID-19 in October 2020 7 , 8 , 9 . It is an analog of a nucleoside that inhibits the viral polymerase enzyme, preventing the replication of the virus.
According to literatures, OSTP was estimated by capillary zone electrophoresis (CE), chromatographic, mass spectrometric (MS), enzymatic, colorimetric, spectrophotometric, and spectrofluorimetric methods since its FDA authorization until 2011 12 , 13 . Since 2011, OSTP has been determined by RP-HPLC 14 , 15 , 16 , UPLC 17 , LC–MS /MS 18 , 19 , spectrofluorimetric 20 , mass spectrometry (MS) 21 , potentiometric 22 , and spectrophotometric 23 methods. DEX was estimated by spectrophotometric, UPLC-UV, HPLC–UV, and LC–MS/MS techniques since its FDA authorization until 2022 24 . REM was determined by UPLC 25 , LC–MS/MS 26 , RP-HPLC 27 , electrochemical 28 , and spectrofluorimetric 29 methods. No analytical method has yet been reported to determine OSTP, DEX, and REM simultaneously in human plasma. So, it was important to develop a method that is fast, accurate, and green to simultaneously separate OSTP, DEX, and REM from human plasma samples to ease the management of these drugs during the treatment of the huge numbers of COVID-19 patients.
The UPLC method was the first candidate method due to its several advantages, including its ability to increase chromatographic efficiency and sensitivity, improve analyte resolution, decrease run time, and lower solvent consumption 30 .
Several significant concerns were carefully investigated during this work. Before application to human plasma, this suggested method was initially optimized by means of the design of experiments (DOE) technique at a column oven temperature of 25 °C. Full factorial designs with one center-point experiment were used to determine the curvature possibilities (lack of fit) of the selected dependent responses, followed by multiple regression analysis. The Gibbs free energy (G°) of the solute interactions with the stationary phase was then used to investigate the effect of column oven temperatures of 25, 30, 45, and 50 °C on the retention times of the studied drugs in reversed-phase chromatography. The proposed method was validated according to the M10 ICH 31 , FDA 32 , and EMA’s 33 bioanalytical method validation guidelines 31 . The bench-top (short-term) stability of each drug at room temperature for 2 h in different types of human plasma samples withdrawn into fluoride, K 3 EDTA, and fluoride-EDTA tubes was investigated. In addition, their matrix effect, extraction efficiency, process efficiency, and internal standard normalized values were estimated according to the M10 ICH's bioanalytical method validation guidelines 31 .
The proposed method has several advantages over other reported LC methods 14 , 17 , 25 , 27 . It is appropriate for UPLC-MS method development since the mobile phases utilized (methanol and ammonium acetate) are compatible with the ionization within mass detectors. In UPLC-MS, methanol is a safe solvent, while ammonium acetate is a volatile salt that is often used to buffer mobile phases. According to the lack of fit test of the factorial design experiments, the effects of methanol percentage, flow rate, or ammonium acetate concentration against k' (OSTP) or R S2 (DAC) are linear (no curvature possibilities), indicating good precision and easy robustness prediction of the proposed method. Fluoride-EDTA human plasma showed inhibition activity on the esterase enzyme in plasma, hence the highest stability results for the studied drugs. The proposed method is simple in sample preparation and extraction. Furthermore, the suggested method's greenness was evaluated using AGREE (Analytical GREEnness) analysis 34 , Eco-Scale 35 , and the Green Analytical Procedure Index (GAPI) criteria 36 .
Experimental
Apparatus and software.
The developed method was carried out on an ACQUITY UPLC H-Class PLUS System (USA) equipped with a Waters quaternary solvent manager (M20QSP 471A), a Waters column heater (K20CHA 249G) with an UPLC column BEH C 18 (1.7-μm, 2.1 \(\times \) 100 mm) connected to an UPLC pre-column BEH (1.7-μm Van Guard, 2.1 \(\times \) 5 mm), a Waters temperature-controlled sample manager-flow-through needle (SM-FTN-H), and a PDA detector (L20UPL 182A). The data was recorded and analyzed using the WatersEmpower3 chromatography software. For the preparation of the mobile phase, a water deionizer (Stakpure-OmniaLab, Germany), a balance (Sartorius, Germany), a pH meter (Jenway 3510, UK), an ultrasonicator (Elma, Germany), a vortex mixer (WiseMix, Portugal), a vacuum pump (Rocker 400, Taiwan), and nylon membrane filters (47 mm in diameter, 0.22 μm pore size, Chromtech, UK) were used. Pro manual Pipet4u micropipettes (Biotechnologie GmbH, Germany) were used for aliquot transfer during the preparation of the samples. An ultra-low-temperature freezer (Binder GmbH, Germany), a tabletop centrifuge (Harmonic series, Taiwan), and a thermostatic water bath (Gemmy Industrial Crop, Taiwan) were used for the preservation and preparation of plasma samples. All extracted samples were filtered using syringe filters (13 mm in diameter, 0.22 μm pore size, Chromtech PTFE, UK) before automatic injection into the UPLC chromatograph. Minitab Statistical Software (Release 16 37 , State College, Pennsylvania, USA) was used for the factorial design statistical analyses.
Materials and solvents
The standard powders of OSTP (purity: 99.976% w/w), DEX (purity: 99.307% w/w), REM (purity: 99.841% w/w), and the internal standard daclatasvir dihydrochloride (DAC) (purity: 101.171% w/w) were provided by NODCAR, Cairo, Egypt. Methanol (HPLC grade, Fisher Scientific, Germany, purity: ≥ 99.9%), ammonium acetate (AA) (Oxford laboratory reagent, India, purity: 96%), and diethyl ether (laboratory reagent grade, UK) were bought from Cornell Lab-Fine Chemicals & Lab Equipments, Cairo, Egypt. K 3 EDTA (Golden Vac, Turkey) and glucose/fluoride (Golden Vac, Turkey) blood tube kits were purchased from local laboratory stores. Human blood samples were kindly donated individually by four adult females: volunteer A (aged 21 years old, donated 10 mL of blood), volunteer B (aged 24 years old, donated 5 mL of blood), volunteer C (aged 30 years old, donated 5 mL of blood), and volunteer D (aged 37 years old, donated 135 mL of blood). From the collected blood, nearly 80 mL of plasma was obtained, from which nearly 155 samples were prepared. We confirm that all experiments were performed in accordance with relevant guidelines and regulations. The study was conducted according to the rules of the Ethics Committee at the Faculty of Pharmacy, Suez Canal University (number: 202009PHDH1). Informed consent was obtained from all subjects and/or their legal guardian(s).
Standard solutions
Four stock solutions of OSTP, DEX, REM, and DAC were each prepared in a volumetric flask (10 mL) and completed to the mark with methanol (HPLC grade, ≥ 99.9%) as a solvent. Each stock solution contained 500 μg mL −1 for each drug. Two different working ternary solutions of OSTP, DEX, and REM were prepared from their methanolic stock solutions. Each working ternary solution was prepared in 10-mL volumetric flasks and diluted with methanol (HPLC grade, ≥ 99.9%). The first methanolic working ternary solution contained 50.0 μg mL −1 of each drug, while the second methanolic working ternary solution contained 5.0 μg mL −1 of each drug. From the methanolic DAC stock solution, an internal standard (IS) (50.0 μg mL −1 DAC) was prepared in a volumetric flask (10 mL) and completed to the mark with methanol (HPLC grade, ≥ 99.9%) as a solvent. All prepared methanolic solutions were preserved in the freezer (from – 15 °C to − 28 °C) and protected from light.
General recommended procedures
We confirm that all experiments were performed in accordance with relevant guidelines and regulations. The study was conducted according to the rules of the Ethics Committee at the Faculty of Pharmacy, Suez Canal University (number: 202009PHDH1). Informed consent was obtained from all subjects and/or their legal guardian(s).
Preparation of human fluoride-EDTA plasma
Human blood samples were withdrawn into K 3 -EDTA tubes (2 mL per tube) and immediately transferred into sodium fluoride tubes to prepare human fluoride-EDTA plasma. The tubes were then left for 5 min, then centrifuged at 3000 rpm for 10 min. The layers of separated plasma were then transferred into Eppendorf tubes and preserved in an ultralow freezer (− 80 °C).
Construction of calibration curves in human plasma
Ternary standard concentrations of OSTP, DEX, and REM ranging from 10.0 ng mL −1 to 5000.0 ng mL −1 for each drug were first prepared to estimate the linearity ranges of each drug. Different aliquots (ranging from 1 to 50 μL by micropipette) of the methanolic working ternary solution (5 μg mL −1 of each drug) were transferred into a series of stoppered test tubes to prepare ternary standard mixtures ranging from 10.0 ng mL −1 to 500.0 ng mL −1 for each drug in human plasma. Also, ternary standard concentrations ranging from 500.0 ng mL −1 to 5000.0 ng mL −1 for each drug were prepared by transferring different aliquots (ranging from 5 to 50 μL by micropipette) of the working ternary solution (50.0 μg mL −1 of each drug) into a series of stoppered test tubes. After the evaporation of methanol solvent at room temperature, 500 μL of plasma samples were added to the residues in each stoppered test tube and vortexed for 30 s. To each tube, 5 μL aliquots of the internal standard (50.0 μg mL −1 ) were added to the spiked plasma, then vortexed for 30 s. Finally, 2 mL aliquots of diethyl ether (DEE) were added to each tube, tightly closed, and vortexed for 1 min. The tubes were centrifuged at 3500 rpm for 5 min, and then 1.5 mL aliquots of the supernatant from each stoppered test tube were separately transferred into tailed test tubes and evaporated in a water bath at 40 °C. Finally, the produced residue was reconstituted in 100 μL of 60% (v/v) methanol, vortexed for 30 s, and filtrated using a membrane filter (pore size of 0.22 μm). Volume (10 μL) was analyzed (n = 6) under the suggested chromatographic conditions. The regression equations were generated by plotting the drug peak area to IS peak area ratios against their respective final concentrations.
Assessment of bench-top (short-term) stability
Aliquots of the methanolic working ternary solutions (50.0 μg mL −1 and 5.0 μg mL −1 of each drug) were transferred into a series of stoppered test tubes to prepare ternary standard solutions containing 1000.0 ng mL −1 and 300.0 ng mL −1 of each drug in plasma, respectively. After evaporation of methanol solvent at room temperature, 500 μL of fresh human plasma samples were added to the residue in each stoppered test tube and vortexed for 30 s. Each concentration, consisting of nine stoppered test tubes, was divided into three groups depending on the time of analysis: 0, 1, and 2 h. At the recommended time interval for each group, 5 μL aliquots of the IS (50.0 μg mL −1 ) were added to each test tube, and then the test tubes were vortexed for 30 s. Then they were extracted by the same procedure under Section “construction of calibration curves in human plasma”. Volumes of 10 μL were injected under optimal chromatographic conditions after filtration using 0.22 μm membrane filters. The peak areas were obtained, and the stability was estimated.
Estimation of matrix effect, extraction and process efficiency and their IS normalized values
For this test, three groups of samples (n = 3 for each group) were analyzed using four blank plasma samples (plasma A, B, C, and D) collected from different sources. The first group, known as Pre-Extraction Samples (PrES), contained extracted spiked human plasma; the second group, known as Post-Extraction Samples (PoES), contained extracted blank human plasma that was then spiked with the tested drugs and IS at the predicted final concentrations; and the third group, known as Solvent Samples (SS), contained the tested drugs and IS diluted in 60% (v/v) methanol at the predicted final concentrations. Two quality control levels were tested (1000.0 ng mL −1 and 300.0 ng mL −1 for each drug) in human plasma samples.
In the first group (PrES), aliquots of the working ternary solution (50.0 μg mL −1 of each drug) were transferred into a series of stoppered test tubes to prepare ternary standard concentrations of OSTP, DEX, and REM of 1000.0 ng mL -1 for each drug in human plasma. After evaporation of methanol solvent at room temperature, 500 μL of human plasma was added to the residues in each stoppered test tube, which was then vortexed for 30 s. Then, 5 μL of the IS (50.0 μg mL −1 ) was added to each one, and all stoppered test tubes were vortexed for 30 s. Then they were extracted by the same procedure under Section “Construction of Calibration Curves in Human Plasma." Finally, the produced residue was reconstituted in 100 μL of 60% (v/v) methanol for each stoppered test tube and vortexed for 30 s. In the second group (PoES), 500 μL of human plasma was added to each stoppered test tube and then extracted by the same procedure described under Section “Construction of Calibration Curves in Human Plasma." Finally, aliquots of 10 μL from the working ternary solution (50.0 μg mL −1 of each drug) and 5 μL from the IS (50.0 μg mL −1 ) were transferred into each of the evaporated-tailed test tubes and then completed to 100 μL by 60% (v/v) methanol. The final reconstitutions were then vortexed for 30 s. The third group (SS) was for the tested drugs diluted in 60% (v/v) methanol. The concentrations of the drugs in this quaternary standard mixture were 5.0 μg mL −1 for OSTP, DEX, and REM and 2.5 μg mL −1 for IS (DAC). These previous steps were repeated for the preparation of pre-extracted, post-extracted, and solvent samples of the 300 ng mL −1 ternary human samples.
After filtering each prepared sample using a membrane filter (pore size of 0.22 μm), a volume of 10 μL was analyzed under the suggested chromatographic conditions. The peak areas were obtained, and the matrix effect, extraction efficiency, process efficiency, and their IS normalized values were estimated for each group.
Experimental design
The purpose of experimental design, also known as design of experimentation (DOE), is to collect as much data as possible from as few experimental trials as possible so that statistical models can be developed and significant conclusions are derived 38 . The full factorial design with/without one centerpoint, response optimizer, and optimization plot were studied in this work 38 , 39 .
Results and discussion
Method development, selection of column and guard column.
According to a previous study 40 , the UPLC Ethylene (-CH 2 -CH 2 -) Bridged Hybrid (BEH) C 18 columns were the first choice for UPLC separations 41 . A UPLC column BEH C 18 7 μm (2.1 \(\times \) 100.0 mm) connected to the ultra-low volume guard column (2.1 \(\text{x}\) 5-mm-length) efficiently maintains UPLC column performance 42 .
Selection of the mobile phase
According to a previous study 40 , methanol (HPLC grade, ≥ 99.9%) was chosen as an eco-friendly organic mobile phase (pH 7–8.3 43 ), while ammonium acetate solution was chosen as the aqueous mobile phase. Ammonium acetate is commercially available at a low price. It is also a safe, chemically stable, and effective buffering medium with very high solubility in methanol. Ammonium acetate solution greatly enhances drug separations because of its excellent residual silanol masking effect on the chromatographic media, and it is harmonious with all LC detectors 44 .
Selection of the suitable wavelength
The PDA detector was theoretically required to be tuned above 215 nm because the UV cutoffs of both ammonium acetate and methanol are at 205 nm 45 , 46 . After the PDA scan of the tested drugs, 220 nm was set for OSTP, 315 nm was set for DAC (IS) (λ max of DAC = 315 nm), and 245 nm was set for DEX (λ max of DEX = 239 nm) and REM (λ max of REM = 245 nm) (Fig. S2 and Table S2 ) 47 . As a result, PDA was set at three wavelengths (λ) during the in vivo analysis, which gave the highest responses for the tested drugs and hence the highest sensitivities. On the other hand, 239 nm was chosen during method development and factorial design optimization for all drug detections.
The drug order of separation
The separation sequence of the compounds is dependent on their log P and pka values 48 . Log P (Table S3 ) suggests that these medications should ideally elute in the following order: OSTP, DEX, and REM. However, the retention time and tailing of the chromatographic peak of a drug are significantly influenced by the pH of the mobile phase, the drug ionization, and the interaction of the ionized drug molecules with the free silanols within the stationary phase. So, based on their pKa values (Table S3 ), DEX and REM would not be greatly affected by the fluctuation of pH from 8 to 2, as they would be unionized. At the same time, OSTP retention time would be greatly affected by the pH of the mobile phase, as it would be less ionized at high pHs than at low values. So, at pH 7, the predicted order would be DEX, OSTP, and then REM. At pH 4, however, with effectively masked silanol groups, the expected order is OSTP, DEX, and finally REM. This is due to the fact that ionizable compounds have significantly shorter retention times than un-ionizable ones in RP- HPLC 48 .
Chromatographic trials during method development
It was suggested to choose favipiravir (FAV) 49 , DAC 50 , or ledipasivir (LED) 51 as the internal standard (IS). Several conditions were tried during the development of the UPLC method (Table S2 , Trials 1–35). DAC as an internal standard was chosen (Table S2 notes). It was observed that the retention times of OSTP and DAC were significantly influenced by pH of the ammonium acetate aqueous mobile phase. So, it was concluded that ammonium acetate (10–50 mM) as an aqueous mobile phase solution adjusted at pH 4 with phosphoric acid showed the most suitable peak order (OSTP, DEX, DAC, and then REM) with good system suitability parameters. Finally, Trials 35–38 (Table S2 ) were established to determine the independent factors of the factorial design and their levels.
Method optimization by factorial design
Full factorial design with centerpoint experiment.
Three independent factors affected the chromatographic performance: methanol percentage (MOH%) in the mobile phase at levels of 60 and 65%, flow rate (FR) at levels of 0.2 and 0.3 mL min −1 , and ammonium acetate (AA) at levels of 10 and 50 mM (Table S2 , Trials 35–38, and Table S4 ). The dependent responses obtained from the chromatograms at a UV wavelength of 239 nm were computerized. According to Table S4 , k ' (OSTP) and R S2 (DAC) as dependent responses were greatly affected by the selected independent factors (predictor variables). To determine the curvature possibility (lack of fit) of the selected dependent responses, 2 3 full factorial designs with one center point experiment (total experiments = 9) (Table S4 and Fig. S3 ) were first done, and then multiple regression analysis was estimated.
The results of k ' (OSTP) and R S2(DAC) obtained from the nine factorial designs were within the (0.81–1.30) and (1.50–4.56) ranges, respectively. Theoretically, the acceptable limit for k ' is more than 0.5 52 , while the acceptable limit for R S is more than 1.5 53 . However, the acceptable minimum limit in plasma analysis for k ' is 1 and R S is 2 at 25 °C.
Multiple regression analysis
From the data obtained from the full factorial with center-point designs, a three-predictor model using multiple regression analyses for k ' (OSTP) and R S2 (DAC) was evaluated.
The obtained regression equations were:
Interpreting the P-value for intercept
The intercept term in the regression (Table S5 ) indicates the average expected value for the dependent factor when all the independent factors are equal to zero.
From Eqs. ( 1 ) and ( 2 ) and from Table S5 , the regression coefficients for the intercepts are equal to 5.16710 and 25.64900 for k ' (OSTP) and R S2 (DAC) , respectively. This means that at chromatographic conditions of zero methanol percentage, 0.00 mL min -1 flow rate, and 0 mM ammonium acetate, the average expected k ' (OSTP) and R S2 (DAC) values are 5.167 and 25.65, respectively. In conducting regression analyses, a p-value for each regression coefficient is estimated. From Table S5 , the p-values are 0.000 (less than α-level (0.05)), which means that the intercept term is statistically different from zero.
Interpreting the P-value for methanol percentage predictor variable
From Eqs. ( 1 ) and ( 2 ) and from Table S5 , methanol% is a predictor variable that ranges from 60 to 65%. The regression coefficients for methanol percentage are − 0.06495 and − 0.33349 for k ' (OSTP) and R S2 (DAC) , respectively. This means that, on average, each additional percentage of methanol is associated with a decrease of 0.06495 and 0.33349 values on k ' (OSTP) and R S2 (DAC) , respectively, assuming the predictor variables flow rate and ammonium acetate concentration are held constant.
From Table S5 , the corresponding p-values are 0.000, which are statistically significant at an alpha level of 0.05. This means that the average change in k ' (OSTP) and R S2 (DAC) for each additional percentage of methanol is statistically significantly different than zero (i.e., methanol percentage has a statistically significant relationship with the response variables k ' (OSTP) and R S2 (DAC) ).
Interpreting the P-value for flow rate predictor variable
From Eqs. ( 1 ) and ( 2 ) and from Table S5 , the flow rate is a predictor variable that ranges from 0.2 to 0.3 mL min −1 . The regression coefficients for the flow rate are − 0.62680 and − 2.92800 for k ' (OSTP) and R S2 (DAC) , respectively. This means that, on average, each additional mL min −1 of the flow rate is associated with a decrease of 0.62680 and 2.92800 values on k ' (OSTP) and R S2 (DAC) , respectively, assuming the predictor variables methanol percentage and ammonium acetate concentration are held constant.
From Table S5 , the corresponding p-values are more than 0.05, which is not statistically significant at an alpha level of 0.05. This means that the average changes in k ' (OSTP) and R S2 (DAC) for each additional mL min −1 of the flow rate are not statistically significantly different from zero (i.e., the flow rate does not have a statistically significant relationship with k ' (OSTP) and R S2 (DAC) ). This indicates that the effect of the flow rate on k ' (OSTP) and R S2 (DAC) could have been due to random chance.
Interpreting the P-value for ammonium acetate concentration predictor variable
From Eqs. ( 1 ) and ( 2 ) and from Table S5 , ammonium acetate concentration (mM) is a predictor variable that ranges from 10 to 50 mM. The regression coefficients for ammonium acetate concentration (mM) are 0.00269 and − 0.03133 for k ' (OSTP) and R S2 (DAC) , respectively. This means that, on average, each additional mM of ammonium acetate is associated with an increase of 0.00269 and a decrease of 0.03133 values on k ' (OSTP) and R S2 (DAC) , respectively, assuming the predictor variables methanol percentage and flow rate are held constant.
From Table S5 , the corresponding p-values are less than 0.05, which is statistically significant at an alpha level of 0.05. This means that the average change in k ' (OSTP) and R S2 (DAC) for each additional mM of ammonium acetate is statistically significantly different than zero (i.e., ammonium acetate concentration (mM) has a statistically significant relationship with the response variables k ' (OSTP) and R S2 (DAC) ).
From the previous interpretations of p-values, the optimized regression analyses for k ' (OSTP) and R S2 (DAC) after removing the insignificant term (FR) from regression Eqs. ( 1 ) and ( 2 ) were evaluated to obtain Eqs. ( 3 ) and ( 4 ):
Interpreting the data subsetting lack of fit test, the R 2 , predicted R 2 , and adjusted R 2
Minitab calculates two types of lack of fit tests. The first test is the pure error lack of fit test. This test is used if the data contains replicates and the model needs to be reduced. Replicates represent "pure error" because only random variation can cause differences between the observed response values. If we are reducing our model and the resulting p-value for lack of fit is less than 0.05, then we should retain the term we removed from the model. The second test is the data subsetting lack of fit test. This test is used if the data does not contain replicates and we want to determine if we are accurately modeling the curvature. This method identifies curvature in the data and interactions among predictors that may affect the model fit. Whenever the data subsetting p-value is less than 0.05, Minitab displays the message "Possible curvature in variable X." If evidence exists that this curvature is not adequately modeled, a higher-order term to model the curvature may be tried after examining the raw data in a scatter plot.
According to Table S5 , the data subsetting lack of fit test was done. There was no evidence of curvature in the regression lines of the methanol percentage, flow rate, or ammonium acetate concentration against k ' (OSTP) or R S2 (DAC) , indicating that the optimum condition can be obtained from the 2 3 full factorial design model without conducting a centerpoint experiment.
From Table S5 , the R 2 values indicate that the independent responses explain 96.4% and 96.8% of the variance in k ' (OSTP) and R S2 (DAC) , respectively. The adjusted R 2 values were 94.3% and 95.0% for k ' (OSTP) and R S2 (DAC) , respectively, which accounts for the number of independent responses in the factorial design model. Both values indicate that the model fits the data well. The predicted R 2 values were 90.50% and 91.28% for k ' (OSTP) and R S2 (DAC) , respectively. Because the predicted R 2 values are close to the R 2 and adjusted R 2 values, the model does not appear to be overfit and has adequate predictive ability.
Interpreting the residual plots
The residual value is the difference between an observed value and its corresponding fitted value. The probability plot is used to evaluate the fit of a distribution to the data. The residual normal probability plots of k ' (OSTP) and R S2 (DAC) show an approximately linear pattern consistent with a normal distribution (Fig. 1 ). The histogram is a graph used to assess the shape and spread of continuous sample data. The histogram can be created prior to or in conjunction with an analysis to help confirm assumptions and guide further analysis. To draw a histogram, minitab divides sample values into many intervals called bins. By default, bars represent the number of observations falling within each bin. According to Fig. 1 , the residual histogram of k ' (OSTP) demonstrated the presence of three negative residual observations (less than − 0.25), four positive residual observations (more than 0.25), and three residual values between − 0.25 and 0.25. On the other hand, the residual histogram of R S2 (DAC) demonstrated the presence of four negative residual observations (less than − 0.25), three positive residual observations (more than 0.25), and three residual values between − 0.25 and 0.25.
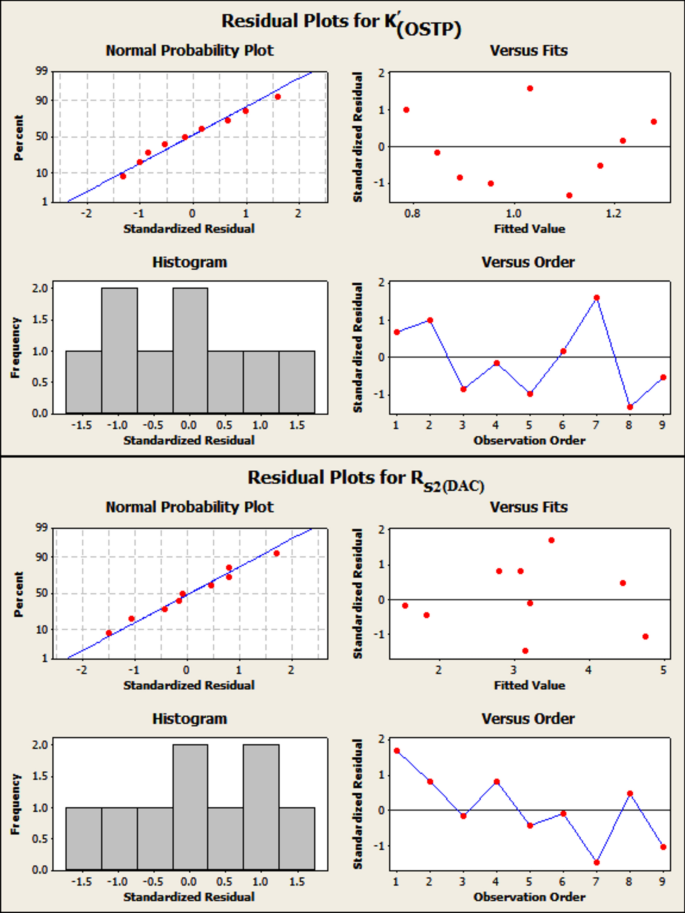
Residual plots for k ' (OSTP) and R S2 (DAC) .
In addition, the residual plot histograms of k ' (OSTP) and R S2 (DAC) indicate that there are no outliers (unusual large or small observations) that may exist in the data, as shown by the continuous bars on the standardized residuals axis of the plot. The plots of residuals versus the fitted values (predicted values) of k ' (OSTP) and R S2 (DAC) show a random pattern of residuals on both sides of 0 (reference line) (Fig. 1 ). The plot of residuals versus the run order is a plot of all residuals in the order that the data was collected in Table S4 and can be used to find non-random errors. The residuals versus order plots of k ' (OSTP) and R S2 (DAC) display a random pattern, so the residuals are uncorrelated with each other (Fig. 1 ).
Optimum conditions determination by 2 3 FFD without center-point experiment
Using a 2 3 full factorial design (total experiments = 8), the response optimizer program was fed with the results of k ' (OSTP) and R S2 (DAC) dependent responses with their desired lower, target, and upper values (Table S1 ). After program data analysis, an optimization plots (Table S1 ) was obtained and the optimum k ' (OSTP) and R S2 (DAC) conditions with their desirability values and the highest composite desirability (D) value were evaluated 39 . According to Table S1 , the final optimal UPLC conditions were 61.5% (v/v) methanol with 38.5% (v/v) AA (40 mM) as a mobile phase with a flow rate of 0.25 mL min -1 at a column oven temperature of 25 °C while adjusting the sample injection volume at 10 μL (Table S2 , Trial 39).
Effect of column oven temperature
UPLC column BEH C 18 1.7 μm (2.1 \(\times \) 100 mm) can withstand elevated temperatures to 80 °C at low pH. Elevating the temperature of the column oven has many benefits. As the column oven’s temperature increases, the mobile phase’s viscosity decreases, and the column’s backpressure decreases. It was observed that raising the temperature from 25 to 50 °C reduced the column’s backpressure from 13,000 psi to 9000 psi. This enables higher flow rates to be used if needed. In addition, the lower pressure reduces the gradual damage to the UPLC instrumentation and thereby increases column life. Increased temperature improves column efficiency while decreasing operating pressure 54 . Also, as the temperature of the column oven is increased, the retention times of the peaks decrease (Fig. 2 , Table 1 , and Table S2 , Trials 39–41).
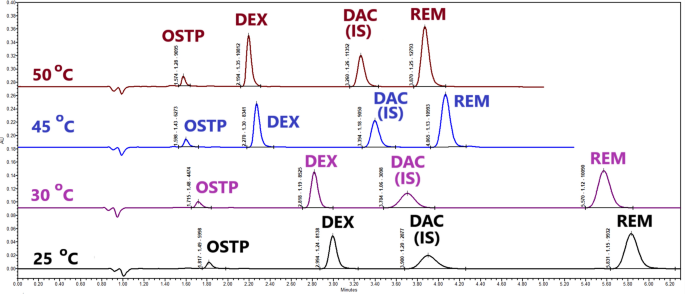
UPLC Chromatograms of oseltamivir phosphate, dexamethasone, daclatasvir dihydrochrolide (IS), and remdesivir (500 ng mL −1 each) were simultaneously separated at different column champer’s temperatures (25, 30, 45, and 50 °C) (each peak labeled by (Rt-T-N)).
Chromatography is a sequence of equilibrium reactions in which the analytes are either dissolved in the mobile phase or adsorbed to the column’s stationary phase. The higher the temperature, the faster the analytes exchange between the mobile and stationary phases. The overlay of the chromatograms at 25, 30, 45, and 50 °C (Fig. 2 and Table 1 ) reveals that peak heights are increasing while peak widths are decreasing. As a result, the number of theoretical plates (N) of the peaks and the sensitivity of the suggested method increase.
The effect of the column oven’s temperature on retention times in reversed-phase chromatography is largely determined by the Gibbs free energy (ΔG°) of the solute interaction with the stationary phase. The ΔG° energy is estimated from the slope of plots of log K ' versus 1/T, called van't Hoff plots (Eq. ( 5 )).
where K ' is the capacity factor, R is the gas constant (8.3145 J mol −1 K −1 ), T is the absolute temperature in kelvin, and Φ is the phase ratio of the column 55 , 56 , 57 . The phase ratio is defined as the ratio between the volume of the stationary phase (V s ) and the volume of the mobile phase (void volume, V o ) in a column 58 .
Van't Hoff plots were constructed for each drug (Fig. 3 ). In general, for components with identical stationary phase interactions, the Van't Hoff plots are linear with slightly different slopes as a result of minor differences in ΔG° values. If Van't Hoff plots deviate from linearity, the retention of the solute molecules in the stationary phase results from very different types of interactions, and the temperature in this case had a great influence on the selectivity 57 .
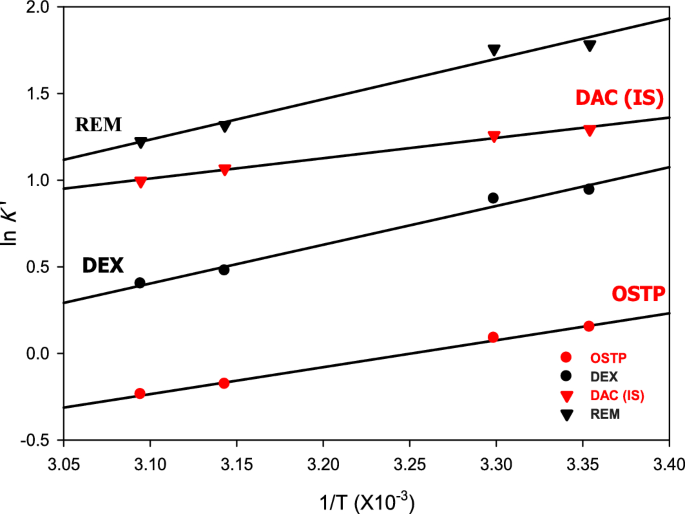
Van’t Hoff plots of oseltamivir phosphate, dexamethasone, daclatasvir dihydrochrolide (IS), and remdesivir.
From Table 2 and Fig. 3 , it was observed that OSTP and DAC (IS) had identical stationary phase interactions as their Van't Hoff plots had slightly different slopes and minor differences in ΔG° values, as well as for DEX and REM. Furthermore, Van't Hoff plots for OSTP and DAC (R 2 greater than 0.99) were linear, while those for DEX and REM deviated slightly from linearity (R 2 lower than 0.99), which indicated differences in the retention mechanisms. The column oven temperature of 50 °C was the most suitable temperature according to Table 1 , with good system suitability parameters (Fig. 4 and Table S2 , Trial 42).
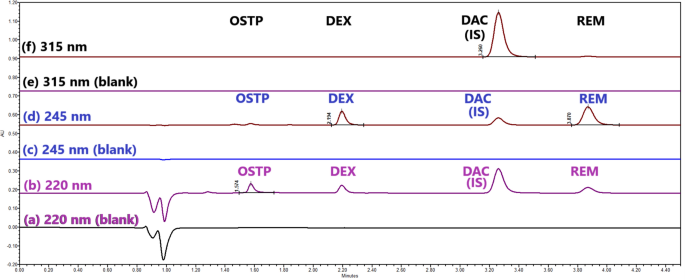
Chromatograms of 30% (v/v) aqueous methanol (blank), oseltamivir phosphate, dexamethasone, daclatasvir dihydrochrolide (IS), and remdesivir (500 ng mL −1 each) at different PDA channels (220, 245, and 315 nm) and column temperature of 50 °C.
The drug extraction technique from human plasma
OSTP, DEX, DAC (IS), and REM have high plasma protein binding of 42% 59 , 77% 60 , 99% 50 , and 88–93.6% 7 , respectively. They also have high log Ps of 1.30 59 , 1.93 60 , 3.47 50 , and 2.10–3.20 7 , respectively. As a result, the most suitable and available method of extraction was liquid–liquid extraction (LLE). Unlike plasma protein precipitation techniques, LLE does not precipitate plasma proteins with the attached drugs. As a result, the drug recovery from plasma by LLE is greater than that achieved by protein precipitation techniques. Also, the high log Ps of the studied drugs permit good extraction of these nonpolar drugs into the organic phase. LLE allows the purification and pre-concentration of the evaporated residues in a small amount of the appropriate solvent, which increases the sensitivity of the method to detect small amounts of the studied drugs in plasma. So, this technique is suitable for drugs with a low plasma C max .
LLE is a separation technique that is frequently employed in industrial operations as well as on the laboratory scale due to its simplicity, low cost, and applicability for thermally labile and high-boiling chemicals 61 . However, the main disadvantages of LLE are the consumption of high volumes of organic solvents needed for the extraction of the drug, a time-consuming process when compared to other methods, the requirement of an evaporation step prior to analysis to remove excess organic solvent, and the possibility of emulsion formation when two immiscible phases are used in the extraction procedure. To overcome these disadvantages, one organic solvent was used to ease the extraction process. A low volume of 2 mL of the organic solvent per sample was used to minimize the pollution effect. Diethyl ether (DEE) as an extraction solvent was used. It is most commonly used in LLE. It has high volatility properties at low temperatures (boiling point: 34.6 °C). So, it is suitable for the extraction of thermolabile drugs. DEE is an inert compound with a high solvation capacity for nonpolar compounds because ethers do not have a hydrogen bonding network that would have to be broken up to dissolve the solute 62 .
Drugs extraction from human blood withdrawn in different types of blood withdrawing tubes
Samples of human blood were withdrawn in different tubes (K 3 EDTA, fluoride, and fluoride-EDTA) to study their effect on the stability of the studied drugs. The same procedure under Section “assessment of bench-top (short-term) stability” was adopted for this study. Under the final chromatographic conditions of Table 3 , the stability of the tested drugs in these kits was studied at time intervals of 0, 1, and 2 h (Eq. ( 6 ) and Table S6 ).
Where AUC is the area under the curve response obtained from the chromatogram.
It was observed that fluoride-EDTA plasma deactivated the esterase enzyme of human blood plasma more than EDTA or fluoride alone and hence increased the stability of the analyzed drugs, especially OSTP 63 , 64 , 65 (Table S6 ).
Method characteristics
A methanolic quaternary mixture of 500 ng mL -1 of each drug was prepared to determine the independent factors. The 2 3 full factorial designs were applied at a column oven temperature of 25 °C. The effect of the temperature of the column oven on drugs’ separation was studied. Finally, the optimum conditions of the proposed method were developed for simultaneous determination of OSTP, DEX, and REM in human plasma using DAC as the IS (Table 3 ).
Method validation
The proposed method was validated according to the bioanalytical method validation M10 ICH 31 , FDA 32 , and EMA’s 33 guidelines. The validation parameters of linearity, limits of detection and quantitation, accuracy and precision, selectivity and specificity, system suitability parameters, stability of stock solutions, freeze–thaw cycles, short-term, long-term, and processed treated samples stability tests, matrix effect, extraction efficiency (recovery), and process efficiency and their IS normalized values were evaluated:
Six concentrations were analyzed to evaluate the method's linearity for each drug (Table 4 ). The suggested method's calibration curves (n = 6) (Fig. S4 ) were plotted within the stated linearity limits for each drug, as shown in Table S7 . The high coefficients of determination (R 2 > 0.9930) suggested that the calibration curves were linear. Table 4 summarizes the quantitative statistical criteria for the studied drugs.
These linearity ranges cover their concentrations in human plasma. Oral doses of 500- and 1000-mg OSTP have a C max of 564 (± 222) and 809 (± 216) ng mL −1 , respectively 66 . The plasma C max of oral 1.5-mg DEX, intramuscular 3-mg DEX injection, and bolus intravenous 4-mg DEX injection are 13.9 ± 6.8 67 , 34.6 ± 6.0 67 , and nearly 100 ng mL −1 68 , respectively. In high single doses of DEX in cancer patients, the plasma C max reaches 1000‒5000 ng mL −1 69 , 70 . The intravenous infusion of 3-mg REM for two hours yields a plasma mean C max of 57.5 ng mL −1 71 , while the intravenous infusion of 225-mg REM for two hours yields a plasma mean C max of 4420 ng mL −1 71 .
Accuracy and precision
The suggested method's accuracy and precision for the within runs (n = 5) and for the between runs within three days (n = 3 per day) were evaluated using low (LQC), middle (MQC), and high (HQC) quality controls at each linearity range besides their lower limits of quantitation (LLOQ) (Table S8 ). The standard deviation (SD) and percentage relative standard deviation (% CV) of the obtained findings were calculated. The CV was found to be small (less than 8.31% for OSTP, 11.41% for DEX, and 11.01% for REM), indicating that the proposed method had appropriate accuracy and precision (Table S8 ).
Selectivity and specificity
Blank, OSTP, DEX, DAC (IS), and REM in human plasma were all investigated at different wavelengths. The blank chromatograms illustrated no peaks within the retention times of the studied drugs. Moreover, no peaks were noticed within any of the drug chromatograms except for their own. So, they were prepared in a quaternary solution (Fig. 5 ). The retention times of OSTP, DEX, the internal standard (DAC), and REM were 1.563 ± 0.006, 2.178 ± 0.017, 3.416 ± 0.016, and 3.826 ± 0.021 min (eight replicates), respectively.
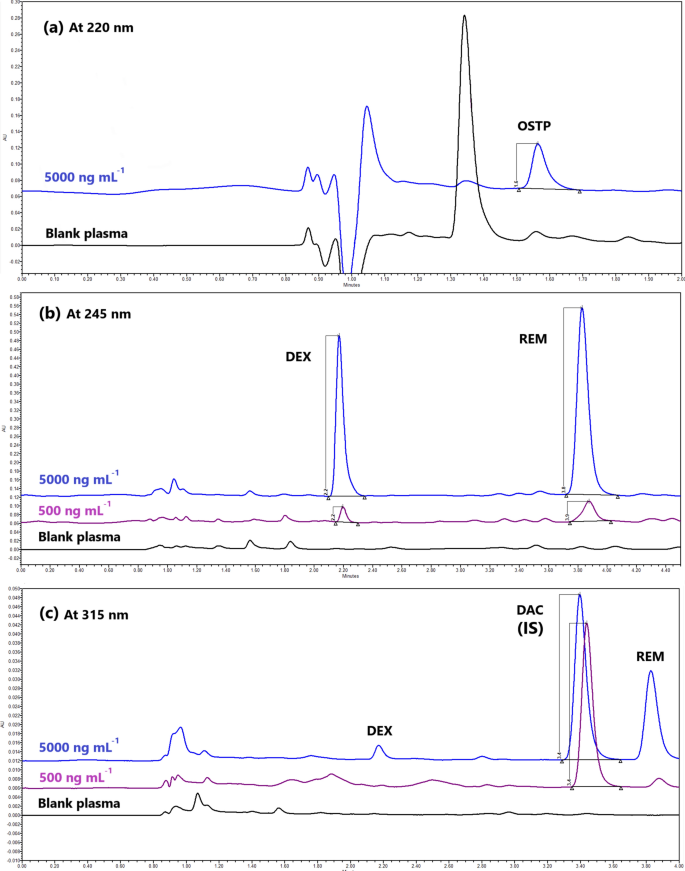
Chromatograms of plasma extracted by the proposed method for specificity.
System suitability parameters
The system suitability parameters were determined by using “Equations (S1-S4)” 39 . According to Table 5 , the RSD% of system suitability parameters obtained from eight chromatograms on four separate days suggested that the system was performing effectively.
Stability of the analyte and IS in stock and working solutions
According to a previous study 40 , the stock and working solutions were stable in the freezer for nearly three weeks for OSTP and DEX analysis but for only about one week in the case of DAC and REM analysis.
The freeze–thaw cycles, short-term, long-term, and processed treated samples stability tests
The freeze–thaw cycles, the short-term stability, the long-term stability for one week, and the stability in processed treated samples for 300 and 1000 ng mL -1 of each drug were estimated (Table 6 ). The tested quality control samples showed high percent recoveries within the range of 86.72% to 106.23% of the nominal concentration, which lies within the acceptable range. The relative standard deviation percentage (RSD% or CV%) of precision was not more than 12.15% (Table 6 ).
Matrix effect, extraction efficiency, and process efficiency
They were estimated at low and high levels of QC. The 300 (LQC) and 1000 (HQC) ng mL -1 of each drug were analyzed using blank human plasma from four different sources (plasma A, B, C, and D).For each analyzed drug, the matrix effect (%ME), extraction efficiency or extraction coefficient (%Recovery efficiency, %RE), and process efficiency (%PE) and their IS normalized values were calculated.
The matrix effect (%ME) is defined as an alteration of the analyte response due to an interfering component (s) in the sample matrix (human plasma) (Eq. ( 7 )). The IS normalized matrix effect (%) is defined as the difference percentage in the "response of the analyte/response of IS" ratio between the same analyte concentrations in the sample matrix (human plasma) spiked with the analyte after extraction and in pure solvents (Eq. ( 8 )) 72 . The accuracies of %ME and the IS normalized matrix effect (%) should be within ± 15% of the nominal concentration, and the %RSD should not be greater than 15% 31 . As indicated in Table S9 , the %RSD of %ME and IS normalized ME (%) values were not more than 2.37%, 5.02%, and 4.67% for OSTP, DEX, and REM, respectively, at both concentration levels. Thus, the plasma matrix did not appear to interfere significantly with the method.
The extraction efficiency (%recovery efficiency, %RE) is the percentage of solute that moves into the extracting phase (Eq. ( 9 )). The high extraction efficiency percentage indicates high extraction process effectiveness, high method sensitivity, and high drug stability in the matrix. The IS normalized extraction efficiency (%) is defined as the difference percentage in the "response of the analyte/response of IS" ratio between the same analyte concentrations in the sample matrix (human plasma) spiked with the analyte before extraction and in the sample matrix (human plasma) spiked with the analyte after extraction (Eq. ( 10 )). As indicated in Table S9 , the %RE and IS normalized RE (%) values were more than 93%, 88%, and 94% for OSTP, DEX, and REM, respectively, at both concentration levels. Thus, the proposed method appeared to have high extraction efficiency for the tested drugs.
The process efficiency (%PE) is expressed as the ratio of the response of an analyte spiked in the sample matrix (human plasma) before extraction to the response of the same analyte in pure solvents multiplied by 100 (Eq. ( 11 )). It would be equivalent to the recovery as per M10 ICH Guidelines 31 . The RE% value represented the true recovery value that was not affected by the sample matrix (human plasma), while the PE% value represented the overall process efficiency that was unfortunately affected by the sample matrix (human plasma) 73 . The IS normalized process efficiency (%) is defined as the difference percentage in the "response of the analyte/response of IS" ratio between the same analyte concentrations in the sample matrix (human plasma) spiked with the analyte before extraction and in pure solvents (Eq. ( 12 )).As indicated in Table S9 , the %RSD of %PE and IS normalized PE (%) values were not more than 5.04%, 5.08%, and 5.02% for OSTP, DEX, and REM, respectively, at both concentration levels. Thus, the proposed method appeared to have good overall process efficiency.
Greenness assessment
The greenness of the proposed method was assessed using the analytical Eco-Scale 35 , the Green Analytical Procedure Index (GAPI) 36 , and the AGREE method 34 . The analytical Eco-Scale 35 is a semi-quantitative tool for greenness assessment of the analytical methods. The simultaneous analysis of OSTP, DEX, and REM in human plasma by the proposed method was an acceptable green analysis with low laboratory needs (Eco-score 68). Moreover, the suggested method provided acceptable green analysis when applied to the assessment of OSTP with DEX mixture, DEX with REM mixture, or each drug separately in human plasma (Eco-score greater than 50) (Table S10 ). Moreover, the suggested method provided acceptable green analysis when applied to the assessment of OSTP with DEX mixture, DEX with REM mixture, or each drug separately in human plasma (Eco-score greater than 50) (Table S10 ).
According to the Green Analytical Procedure Index (GAPI) 36 , the suggested method was an indirect procedure with a LLE extraction process (Fig. S5 ). It also used small volumes of safe chemicals with little waste due to the low flow rate (0.25 mL min −1 ). Furthermore, the suggested method was for qualitative and quantitative analyses.
The AGREE method (Analytical GREEnness Metric Approach and Software) 34 is a comprehensive method that incorporates 12 significance principles for greenness assessment of the analytical methods. The obtained colorful pictogram shows the structure of weak and strong points of the analytical method. According to Fig. S6 , the total score was illustrated in the center of the pictogram, with a number near one (0.62) and a light green color suggesting that the suggested method was acceptable green.
The proposed method was optimized and validated according to the M10 ICH's bioanalytical method validation guideline. The developed method is highly sensitive, accurate, and precise, with a wide range of linearity in human plasma. It is suitable for assaying OSTP, DEX, and REM in human plasma, as the linearity ranges cover their plasma C max . The PDA detector is used for OSTP, DEX, and REM analysis using three wavelengths. The full factorial designs with one centerpoint experiment were used to determine the curvature possibilities (lack of fit) of the selected dependent responses, followed by multiple regression analysis. There was no evidence of curvatures in the regression lines of the methanol percentage, flow rate, or ammonium acetate concentration against k ' (OSTP) or R S2 (DAC) , indicating that the optimum condition can be obtained from the 2 3 full factorial design without conducting a centerpoint experiment. The method was optimized at a column oven temperature of 25 °C. The effect of column oven temperatures at 25, 30, 45, and 50 °C on the drugs’ retention times was investigated by the Van't Hoff plots and Gibbs’ free energy (G°) of the tested drugs. OSTP and DAC (IS) have identical stationary phase interactions as their Van't Hoff plots had slightly different slopes and minor differences in ΔG° values, as well as for DEX and REM. The column oven temperature of 50 °C is the most suitable temperature with good system suitability parameters. The benchtop (short-term) stability of each drug at room temperature for 2 h in different types of human plasma was investigated. Fluoride-EDTA human plasma shows the highest stability results for the studied drugs. The matrix effect, extraction efficiency, process efficiency, and internal standard normalized values of the proposed method are high. The optimization by factorial method is the most eco-friendly technique. Because it consumes the least amount of instrumental energy and money and releases the least amount of hazardous waste into the environment. Furthermore, the analytical Eco-Scale, GAPI, and AGREE proved the greenness of the proposed method. It uses small volumes of safe chemicals with little waste due to the low flow rate (0.25 mL min −1 ).
Data availability
The datasets generated and/or analyzed during the current study are available from the corresponding author on reasonable request.
Gulyaeva, A. A. & Gorbalenya, A. E. A nidovirus perspective on SARS-CoV-2. Biochem. Biophys. Res. Commun. 538 , 24–34 (2021).
Article PubMed Google Scholar
Malone, B., Urakova, N., Snijder, E. J. & Campbell, E. A. Structures and functions of coronavirus replication–transcription complexes and their relevance for SARS-CoV-2 drug design. Nat. Rev. Mol. Cell Biol. 23 , 21–39. https://doi.org/10.1038/s41580-021-00432-z (2022).
Shabani, M., Sadegh Ehdaei, B., Fathi, F. & Dowran, R. A mini-review on sofosbuvir and daclatasvir treatment in coronavirus disease 2019. New Microbes New Infect. 42 , 100895. https://doi.org/10.1016/j.nmni.2021.100895 (2021).
Article PubMed PubMed Central Google Scholar
Vafaei, S., Razmi, M., Mansoori, M., Asadi-Lari, M. & Madjd, Z. Spotlight of remdesivir in comparison with ribavirin, favipiravir, oseltamivir and umifenovir in coronavirus disease 2019 (COVID-19) pandemic. Lancet Infect. Dis. https://doi.org/10.2139/ssrn.3569866 (2020).
Article Google Scholar
Tan, Q. et al. Is oseltamivir suitable for fighting against COVID-19: In silico assessment, in vitro and retrospective study. Bioorg. Chem. 104 , 104257. https://doi.org/10.1016/j.bioorg.2020.104257 (2020).
Qiu, T. et al. Chinese guidelines related to novel coronavirus pneumonia. J. Mark. Access Health Policy 8 , 1818446. https://doi.org/10.1080/20016689.2020.1818446 (2020).
DrugBank. Remdesivir , https://go.drugbank.com/drugs/DB14761 (2019).
Hanafin, P. O. et al. A mechanism-based pharmacokinetic model of remdesivir leveraging interspecies scaling to simulate COVID-19 treatment in humans. CPT Pharmacomet. Syst. Pharmacol. 10 , 89–99. https://doi.org/10.1002/psp4.12584 (2021).
EMA. Veklury , https://www.ema.europa.eu/en/documents/assessment-report/veklury-epar-public-assessment-report_en.pdf (2020).
Masoud, H. et al. Management protocol for COVID-19 patients MoHP protocol for COVID19 November 2020 , https://www.researchgate.net/publication/345813633_Management_Protocol_for_COVID-19_Patients_MoHP_Protocol_for_COVID19_November_2020 (2020).
FDA, U. S. The FDA approves first generic version of widely used influenza drug, Tamiflu , https://www.fda.gov/drugs/postmarket-drug-safety-information-patients-and-providers/fda-approves-first-generic-version-widely-used-influenza-drug-tamiflu#:~:text=On%20August%203%2C%202016%2C%20the,and%20prevention%20of%20the%20flu (2016).
Navas, M. & Jimenez, A. Analytical methods to determine anti-influenza drugs. Crit. Rev. Anal. Chem. 41 , 81–97 (2011).
Bosch, M. E., Ojeda, C. B., Sánchez, A. J. R. & Rojas, F. S. Analytical methodologies for the determination of oseltamivir. Res. J. Pharm. Biol. Chem. Sci. 1 , 368 (2010).
Google Scholar
Junaidy, M. Q., Haque, M. A. & Bakshi, V. RP-HPLC method development and validation for the estimation of oseltamivir phosphate in bulk form and pharmaceutical formulations. Int. J. Innov. Pharma. Sci. Res. 2 , 2786–2791 (2014).
Ramya, V. Z. & Gowda, Y. N. Simultaneous estimation of amantadine hydrochloride and oseltamivir phosphate using precolumn derivatization technique. Int. J. Pharm. Sci. Res. 10 , 5443–5449. https://doi.org/10.13040/IJPSR.0975-8232.10(12).5443-49 (2019).
Al-Bagary, R. I., El-Zaher, A. A., Morsy, F. A. & Fouad, M. M. Kinetic study of the alkaline degradation of oseltamivir phosphate and valacyclovir hydrochloride using validated stability indicating HPLC. Anal. Chem. Insights 9 , 41–48. https://doi.org/10.4137/aci.S13878 (2014).
Reddy, G., Pulipaka, S., Krishna, R., Narayanarao, K. & Rapeti, D. Characterization of oseltamivir phosphate api and simultaneous quantification and validation of its impurities by UPLC. Asian J. Pharm. Clin. Res. 14 , 161–169. https://doi.org/10.22159/ajpcr.2021.v14i4.40595 (2021).
Reddy, S. et al. Development and validation of two LCMS/MS methods for simultaneous estimation of oseltamivir and its metabolite in human plasma and application in bioequivalence study. Asian J. Pharm. Anal. 6 , 91–101 (2016).
Berendsen, B. J., Wegh, R. S., Essers, M. L., Stolker, A. A. & Weigel, S. Quantitative trace analysis of a broad range of antiviral drugs in poultry muscle using column-switch liquid chromatography coupled to tandem mass spectrometry. Anal. Bioanal. Chem. 402 , 1611–1623 (2012).
Omar, M. A., Derayea, S. M. & Mostafa, I. M. Selectivity improvement for spectrofluorimetric determination of oseltamivir phosphate in human plasma and in the presence of its degradation product. Fluorescence 27 , 1323–1330. https://doi.org/10.1007/s10895-017-2066-6 (2017).
Flick, T. G., Leib, R. D. & Williams, E. R. Direct standard-free quantitation of Tamiflu and other pharmaceutical tablets using clustering agents with electrospray ionization mass spectrometry. Anal. Chem. 82 , 1179–1182. https://doi.org/10.1021/ac902277d (2010).
Jebali, I. & Belgaied, J.-E. A novel coated platinum electrode for oseltamivir determination in pharmaceuticals. Mater. Sci. Eng. C 37 , 90–98. https://doi.org/10.1016/j.msec.2013.12.040 (2014).
Gungor, S., Bulduk, I., Sultan Aydın, B. & Ilikci Sagkan, R. A comparative study of HPLC and UV spectrophotometric methods for oseltamivir quantification in pharmaceutical formulations. Acta Chromatogr. 34 , 258–266. https://doi.org/10.1556/1326.2021.00925 (2022).
Esposito, M. C., Santos, A. L. A., Bonfilio, R. & de Araújo, M. B. A critical review of analytical methods in pharmaceutical matrices for determination of corticosteroids. Crit. Rev. Anal. Chem. 50 , 111–124 (2020).
Emam, A. A., Abdelaleem, E. A., Abdelmomen, E. H., Abdelmoety, R. H. & Abdelfatah, R. M. Rapid and ecofriendly UPLC quantification of remdesivir, favipiravir and dexamethasone for accurate therapeutic drug monitoring in Covid-19 Patient’s plasma. Microchem. J. 179 , 107580. https://doi.org/10.1016/j.microc.2022.107580 (2022).
Nguyen, R. et al. Development and validation of a simple, selective, and sensitive LC-MS/MS assay for the quantification of remdesivir in human plasma. J. Chromatogr. B 1171 , 122641. https://doi.org/10.1016/j.jchromb.2021.122641 (2021).
Hamdy, M. M., Abdel Moneim, M. M. & Kamal, M. F. Accelerated stability study of the ester prodrug remdesivir: Recently FDA-approved Covid-19 antiviral using reversed-phase-HPLC with fluorimetric and diode array detection. Biomed. Chromatogr. 35 , e5212 (2021).
Deniz, E., Özaltin, N. & Yilmaz, S. A review on recent electroanalytical methods for the analysis of antiviral COVID-19 drugs. Turk. J. Chem. 3 , 1–8 (2021).
Elmansi, H., Ibrahim, A. E., Mikhail, I. E. & Belal, F. Green and sensitive spectrofluorimetric determination of remdesivir, an FDA approved SARS-CoV-2 candidate antiviral; application in pharmaceutical dosage forms and spiked human plasma. Anal. Methods 13 , 2596–2602. https://doi.org/10.1039/D1AY00469G (2021).
Gumustas, M., Kurbanoglu, S., Uslu, B. & Ozkan, S. A. UPLC versus HPLC on drug analysis: Advantageous, applications and their validation parameters. Chromatographia 76 , 1365–1427 (2013).
Bioanalytical method validation and study sample analysis M10. ICH Harmonised Guideline: Geneva, Switzerland (2022).
Meesters, R. & Voswinkel, S. Bioanalytical method development and validation: From the USFDA 2001 to the USFDA 2018 guidance for industry. J. Appl. Bioanal. 4 , 67–73 (2018).
Smith, G. European medicines agency guideline on bioanalytical method validation: What more is there to say?. Bioanalysis 4 , 865–868 (2012).
Pena-Pereira, F., Wojnowski, W. & Tobiszewski, M. AGREE—Analytical greenness metric approach and software. Anal. Chem. 92 , 10076–10082. https://doi.org/10.1021/acs.analchem.0c01887 (2020).
Gałuszka, A., Migaszewski, Z. M., Konieczka, P. & Namieśnik, J. Analytical Eco-Scale for assessing the greenness of analytical procedures. Trends Anal. Chem. 37 , 61–72 (2012).
Płotka-Wasylka, J. A new tool for the evaluation of the analytical procedure: Green Analytical Procedure Index. Talanta 181 , 204–209. https://doi.org/10.1016/j.talanta.2018.01.013 (2018).
Yi, X., Shi, W., Yu, S. & Li, X. MINITAB ® release 16 statistical software for windows . https://www.minitab.com/en-us/products/minitab/ (2011).
Dejaegher, B. & Heyden, Y. V. Experimental designs and their recent advances in set-up, data interpretation, and analytical applications. J. Pharm. Biomed. Anal. 56 , 141–158. https://doi.org/10.1016/j.jpba.2011.04.023 (2011).
El-Shorbagy, H. I., Elsebaei, F., Hammad, S. F. & El-Brashy, A. M. Optimization and modeling of a green dual detected RP-HPLC method by UV and fluorescence detectors using two level full factorial design for simultaneous determination of sofosbuvir and ledipasvir: Application to average content and uniformity of dosage unit testing. Microchem. J. 147 , 374–392. https://doi.org/10.1016/j.microc.2019.03.039 (2019).
El-Shorbagy, H. I., Mohamed, M. A., El-Gindy, A., Hadad, G. M. & Belal, F. Development of UPLC method for simultaneous assay of some COVID-19 drugs utilizing novel instrumental standard addition and factorial design. Sci. Rep. 13 , 5466. https://doi.org/10.1038/s41598-023-32405-x (2023).
Article ADS PubMed PubMed Central Google Scholar
Waters. ACQUITY UPLC ® BEH C 18 and C 8 columns by Waters , https://www.selectscience.net/products/acquity-uplc-beh-c18-and-c8-columns/?prodID=79501#tab-2 (2020).
Waters. Column particle technologies , https://www.waters.com/waters/en_US/BEH-(Ethylene-Bridged-Hybrid)-Technology/nav.htm?cid=134618172&locale=en_US (2022).
Tindall, G. W. & Dolan, J. W. The interpretation of pH in partially aqueous mobile phases. LC GC Europe 15 , 776–779 (2002).
Lim, C. K. & Peters, T. J. Ammonium acetate: A general purpose buffer for clinical applications of high-performance liquid chromatography. J. Chromatogr. A 316 , 397–406. https://doi.org/10.1016/S0021-9673(00)96168-5 (1984).
Boyes, B. & Dong, M. Modern trends and best practices in mobile-phase selection in reversed-phase chromatography. LC GC Europe 31 , 572–583 (2018).
Wilson, N. S., Morrison, R. & Dolan, J. W. Buffers and baselines. LC GC Europe 19 , 590–595 (2001).
Piórkowska, E., Kaza, M., Fitatiuk, J., Szlaska, I. & Rudzki, P. Rapid and simplified HPLC-UV method with on-line wavelengths switching for determination of capecitabine in human plasma. Die Pharmazie 69 , 500–505. https://doi.org/10.1691/ph.2014.3223 (2014).
Soriano-Meseguer, S., Fuguet, E., Port, A. & Rosés, M. Influence of the acid-base ionization of drugs in their retention in reversed-phase liquid chromatography. Anal. Chim. Acta 1078 , 200–211. https://doi.org/10.1016/j.aca.2019.05.063 (2019).
DrugBank. Favipiravir , https://go.drugbank.com/drugs/DB12466 (2021).
DrugBank. Daclatasvir dihydrochloride , https://go.drugbank.com/salts/DBSALT001166 (2015).
DrugBank. Ledipasvir , https://www.drugbank.ca/drugs/DB09027 (2022).
Wadie, M. A., Mostafa, S. M., El Adl, S. M. & Elgawish, M. S. Development and validation of a new, simple-hplc method for simultaneous determination of sofosbuvir, daclatasvir and ribavirin in tablet dosage form. J. Pharm. Biol. Sci. 12 , 60–68 (2017).
Ng, L. L. Reviewer guidance: Validation of chromatographic methods , https://www.fda.gov/regulatory-information/search-fda-guidance-documents/reviewer-guidance-validation-chromatographic-methods (1994).
Li, J. B. Effect of temperature on column pressure, peak retention time and peak shape , https://www.waters.com/webassets/cms/library/docs/watersamd30.pdf (2023).
Kowalczyk, J. S. & Herbut, G. Influence of temperature on separation processes in adsorption liquid chromatographic systems. J. Chromatogr. A 196 , 11–20. https://doi.org/10.1016/S0021-9673(00)80355-6 (1980).
Hatsis, P. & Lucy, C. Effect of temperature on retention and selectivity in ion chromatography of anions. J. Chromatogr. A 920 , 3–11. https://doi.org/10.1016/S0021-9673(00)01226-7 (2001).
Rosing, H., Doyle, E. & Beijnen, J. H. The impact of column temperature in the high performance liquid chromatographic analysis of topotecan in rat and dog plasma. J. Pharm. Biomed. Anal. 15 , 279–286. https://doi.org/10.1016/0731-7085(96)01838-9 (1996).
Caiali, E., David, V., Aboul-Enein, H. Y. & Moldoveanu, S. C. Evaluation of the phase ratio for three C18 high performance liquid chromatographic columns. J. Chromatogr. A 1435 , 85–91. https://doi.org/10.1016/j.chroma.2016.01.043 (2016).
DrugBank. Oseltamivir , https://go.drugbank.com/drugs/DB00198 (2024).
DrugBank. Dexamethasone , https://go.drugbank.com/drugs/DB01234 (2005).
Tshepelevitsh, S. et al. Systematic optimization of liquid–liquid extraction for isolation of unidentified components. ACS Omega 2 , 7772–7776. https://doi.org/10.1021/acsomega.7b01445 (2017).
Ouellette, R. J. & Rawn, J. D. In Principles of Organic Chemistry (eds Ouellette, R. J. & David Rawn, J.) 239–258 (Elsevier, 2015).
Chapter Google Scholar
Davies, B. E. Pharmacokinetics of oseltamivir: An oral antiviral for the treatment and prophylaxis of influenza in diverse populations. J. Antimicrob. Chemother. 65 , ii5–ii10. https://doi.org/10.1093/jac/dkq015 (2010).
Li, Z., Zhang, J., Zhang, Y. & Zuo, Z. Role of esterase mediated hydrolysis of simvastatin in human and rat blood and its impact on pharmacokinetic profiles of simvastatin and its active metabolite in rat. J. Pharm. Biomed. Anal. 168 , 13–22. https://doi.org/10.1016/j.jpba.2019.02.004 (2019).
Kromdijk, W., Rosing, H., van den Broek, M. P., Beijnen, J. H. & Huitema, A. D. Quantitative determination of oseltamivir and oseltamivir carboxylate in human fluoride EDTA plasma including the ex vivo stability using high-performance liquid chromatography coupled with electrospray ionization tandem mass spectrometry. J. Chromatogr. B Analyt. Technol. Biomed. Life Sci. 891–892 , 57–63. https://doi.org/10.1016/j.jchromb.2012.02.026 (2012).
Massarella, J. W. et al. The pharmacokinetics and tolerability of the oral neuraminidase inhibitor oseltamivir (Ro 64–0796/GS4104) in healthy adult and elderly volunteers. J. Clin. Pharmacol. 40 , 836–843. https://doi.org/10.1177/00912700022009567 (2000).
Loew, D., Schuster, O. & Graul, E. H. Dose-dependent pharmacokinetics of dexamethasone. Eur. J. Clin. Pharmacol. 30 , 225–230. https://doi.org/10.1007/bf00614309 (1986).
Spoorenberg, S. M. C. et al. Pharmacokinetics of oral vs. intravenous dexamethasone in patients hospitalized with community-acquired pneumonia. Br. J. Clin. Pharmacol. 78 , 78–83. https://doi.org/10.1111/bcp.12295 (2014).
Brady, M. E., Sartiano, G. P., Rosenblum, S. L., Zaglama, N. E. & Bauguess, C. T. The pharmacokinetics of single high doses of dexamethasone in cancer patients. Eur. J. Clin. Pharmacol. 32 , 593–596. https://doi.org/10.1007/bf02455994 (1987).
Nakade, S. et al. Population pharmacokinetics of aprepitant and dexamethasone in the prevention of chemotherapy-induced nausea and vomiting. Cancer Chemother. Pharmacol. 63 , 75–83. https://doi.org/10.1007/s00280-008-0713-y (2008).
Humeniuk, R. et al. Safety, tolerability, and pharmacokinetics of remdesivir, an antiviral for treatment of COVID-19, in healthy subjects. Clin. Transl. Sci. 13 , 896–906 (2020).
Elawady, T., Khedr, A., El-Enany, N. & Belal, F. LC-MS/MS determination of erdafitinib in human plasma after SPE: Investigation of the method greenness. Microchem. J. 154 , 104555. https://doi.org/10.1016/j.microc.2019.104555 (2020).
Nouman, E. G., Al-Ghobashy, M. A. & Lotfy, H. M. Development and validation of LC-MS/MS assay for the determination of Butoconazole in human plasma: Evaluation of systemic absorption following topical application in healthy volunteers. Bull. Fac. Pharm. Cairo Univ. 55 , 303–310. https://doi.org/10.1016/j.bfopcu.2017.04.003 (2017).
Download references
Open access funding provided by The Science, Technology & Innovation Funding Authority (STDF) in cooperation with The Egyptian Knowledge Bank (EKB).
Author information
Authors and affiliations.
Pharmaceutical Analytical Chemistry Department, Faculty of Pharmacy, Suez Canal University, Ismailia, 41522, Egypt
Hanan I. EL-Shorbagy, Alaa El-Gindy & Ghada M. Hadad
Pharmaceutical Chemistry Department, Egyptian Drug Authority (EDA), Cairo, Egypt
Mona A. Mohamed
Department of Pharmaceutical Analytical Chemistry, Faculty of Pharmacy, Mansoura University, Mansoura, 35516, Egypt
Fathalla Belal
You can also search for this author in PubMed Google Scholar
Contributions
H.I.: conceptualization and methodology; software and acquisition of data; validation; formal analysis and interpretation of data; and drafting the manuscript. F.B. and G.H.: conceptualization and methodology. All authors reviewed the manuscript critically for important intellectual content and approved its publication.
Corresponding author
Correspondence to Hanan I. EL-Shorbagy .
Ethics declarations
Competing interests.
The authors declare no competing interests.
Ethical approval
We confirm that all experiments were performed in accordance with relevant guidelines and regulations. The study was conducted according to the rules of the Ethics Committee at the Faculty of Pharmacy, Suez Canal University (number: 202009PHDH1). Informed consent was obtained from all subjects and/or their legal guardian(s). The duration of the study was within 1 month and parallel. The retention of the sample in the ultra-low deep freezer was for 7 days. The preparation of spiked solutions for validation was concurrent with the study. The preparation of spiked samples for analysis of the study sample was done after validation and preserved for 1 week.
Additional information
Publisher's note.
Springer Nature remains neutral with regard to jurisdictional claims in published maps and institutional affiliations.
Supplementary Information
Supplementary information., rights and permissions.
Open Access This article is licensed under a Creative Commons Attribution 4.0 International License, which permits use, sharing, adaptation, distribution and reproduction in any medium or format, as long as you give appropriate credit to the original author(s) and the source, provide a link to the Creative Commons licence, and indicate if changes were made. The images or other third party material in this article are included in the article's Creative Commons licence, unless indicated otherwise in a credit line to the material. If material is not included in the article's Creative Commons licence and your intended use is not permitted by statutory regulation or exceeds the permitted use, you will need to obtain permission directly from the copyright holder. To view a copy of this licence, visit http://creativecommons.org/licenses/by/4.0/ .
Reprints and permissions
About this article
Cite this article.
EL-Shorbagy, H.I., Mohamed, M.A., El-Gindy, A. et al. UPLC-PDA factorial design assisted method for simultaneous determination of oseltamivir, dexamethasone, and remdesivir in human plasma. Sci Rep 14 , 21758 (2024). https://doi.org/10.1038/s41598-024-71413-3
Download citation
Received : 22 October 2023
Accepted : 27 August 2024
Published : 18 September 2024
DOI : https://doi.org/10.1038/s41598-024-71413-3
Share this article
Anyone you share the following link with will be able to read this content:
Sorry, a shareable link is not currently available for this article.
Provided by the Springer Nature SharedIt content-sharing initiative
- Fluoride-EDTA plasma
- Gibbs free energy
- Green analysis
By submitting a comment you agree to abide by our Terms and Community Guidelines . If you find something abusive or that does not comply with our terms or guidelines please flag it as inappropriate.
Quick links
- Explore articles by subject
- Guide to authors
- Editorial policies
Sign up for the Nature Briefing newsletter — what matters in science, free to your inbox daily.


COMMENTS
Training Videos & Courses Experimental Design. Scientists from a variety of backgrounds give concrete steps and advice to help you build a framework for how to design experiments in biological research. Learn strategies for successful experimental design, tips to avoid bias, and insights to improve reproducibility with in-depth case studies.
About this course. 'Experiments: From Idea to Design' equips you with the right tools to help develop, plan and refine robust, impactful experiments. You will cover all the core concepts of experimental design and discover strategies to complete the full process of developing a research motivation, formulating hypotheses, assembling an ...
You can choose to make your experiments 'private' or publicly share them with others, enabling others to build on your design and facilitating replication of results. Our paper Giamattei, M., Yahosseini, K. S., Gächter, S. and Molleman, L. (2020): LIONESS Lab: a Free Web-Based Platform for Conducting Interactive Experiments Online.
Prepare 2 identical trays with the same soil mixture. Place 5 plants in each tray; label one set "sunlight" and one set "shade". Position sunlight tray by a south-facing window, and shade tray in a dark closet. Water both trays with 50 mL water every 2 days. After 3 weeks, remove plants and measure heights in cm.
Table of contents. Step 1: Define your variables. Step 2: Write your hypothesis. Step 3: Design your experimental treatments. Step 4: Assign your subjects to treatment groups. Step 5: Measure your dependent variable. Other interesting articles. Frequently asked questions about experiments.
1) True Experimental Design. In the world of experiments, the True Experimental Design is like the superstar quarterback everyone talks about. Born out of the early 20th-century work of statisticians like Ronald A. Fisher, this design is all about control, precision, and reliability.
There are 5 modules in this course. This is a basic course in designing experiments and analyzing the resulting data. The course objective is to learn how to plan, design and conduct experiments efficiently and effectively, and analyze the resulting data to obtain objective conclusions. Both design and statistical analysis issues are discussed.
In the first mission of the experimental design simulation, you will learn how to use the scientific method to investigate phenomena, acquire new knowledge, or correct and integrate existing knowledge. Every tool you need to use is available in this virtual lab. Design an experiment and test your hypothesis.
The Experimental Design Assistant. A free resource from the NC3Rs used by over 5,000 researchers worldwide to help you design robust experiments more likely to yield reliable and reproducible results. The EDA helps you build a diagram representing your experimental plan, which can be critiqued by the system to provide bespoke feedback.
At first, experimental design can be a daunting topic for students to learn. Thankfully, there are creative ways to introduce them in the classroom. Here are five examples of such techniques. 1. Use Interactive Demonstrations of Experimental Design. Experiments are meant to be interactive. Experimental design should be as well.
Chemix is a free online editor for drawing lab diagrams. Simple and intuitive, it is designed for students and pupils to help them draw diagrams of common laboratory equipment and lab setup of science experiments. Chemix is an online editor for drawing lab diagrams and school experiment apparatus. Easy sketching for both students and teachers.
The Experimental Design Online application is managed by Scandinavian Institute of Business Analytics SCANBA. The application is free to use and does does not store or record any data. ... Education is central to ILSSI activities. The institute's training and certification programs are divided by global region. ILSSI have Lean Six Sigma Master ...
Experimental design is a process of planning and conducting scientific experiments to investigate a hypothesis or research question. It involves carefully designing an experiment that can test the hypothesis, and controlling for other variables that may influence the results. Experimental design typically includes identifying the variables that ...
Building a state-of-the-art online experiment does not need to be difficult! With our editor you can visually create and run any experiment without needing to write a single line of code. The Labvanced experiment editor is built based on design principles from Experimental Psychology, which means you'll understand the system in a short time!
1.01 Identify and create questions and hypotheses that can be answered through scientific investigations. 1.02 Develop appropriate experimental procedures for: Given questions. Student generated questions. 1.04 Analyze variables in scientific investigations: Identify dependent and independent. Use of a control.
For example, consider an experimental situation in which field studies repeated at multiple locations are an annual activity, such as uniform cultivar evaluations (e.g., ... In fact, this represents an incomplete description of the experimental design because it is missing the basic design for Factor A, the whole-plot factor. For example, ...
An experimental design is a detailed plan for collecting and using data to identify causal relationships. Through careful planning, the design of experiments allows your data collection efforts to have a reasonable chance of detecting effects and testing hypotheses that answer your research questions. An experiment is a data collection ...
Three types of experimental designs are commonly used: 1. Independent Measures. Independent measures design, also known as between-groups, is an experimental design where different participants are used in each condition of the independent variable. This means that each condition of the experiment includes a different group of participants.
wer before doing the lab:1. What is th. problem? Describe the problem in your own words. Be sure that your description includes known factors (information about the problem given to you in the lab in a problem statement, for example) and unknowns (what yo. need to find out in order to solve the problem). Then restate the problem in the form of ...
Test your knowledge on the scientific method and experimental design!
These series of activities are designed for students to collaborate together to come up with something unique and interesting. For many of the experimental questions, students will work in pairs or groups to come up with their own experimental design. If the school has resources and there is enough time, students can test their designs.
4.9. (7) $3.00. PDF. Students will complete a fun domino-style puzzle. The puzzle includes 20 experimental design review questions. I have included two different sizes of domino templates. The one-page template is ideal for independent work or as a partner activity. The larger, 3-page domino template is best for groups of 3-4 students.
The scientific method. Humans have always strived to explain natural phenomena. In the first mission of the experimental design simulation, you will learn how to use the scientific method to investigate phenomena, acquire new knowledge, or correct and integrate existing knowledge. Every tool you need to use is available in this virtual lab.
COVID-19 created a serious threat to economies and healthcare systems. SARS-CoV-2 viruses, which are encapsulated viruses with a single-stranded RNA genome, are the main cause of COVID-19 1,2.In ...