Home Blog Design Understanding Data Presentations (Guide + Examples)

Understanding Data Presentations (Guide + Examples)
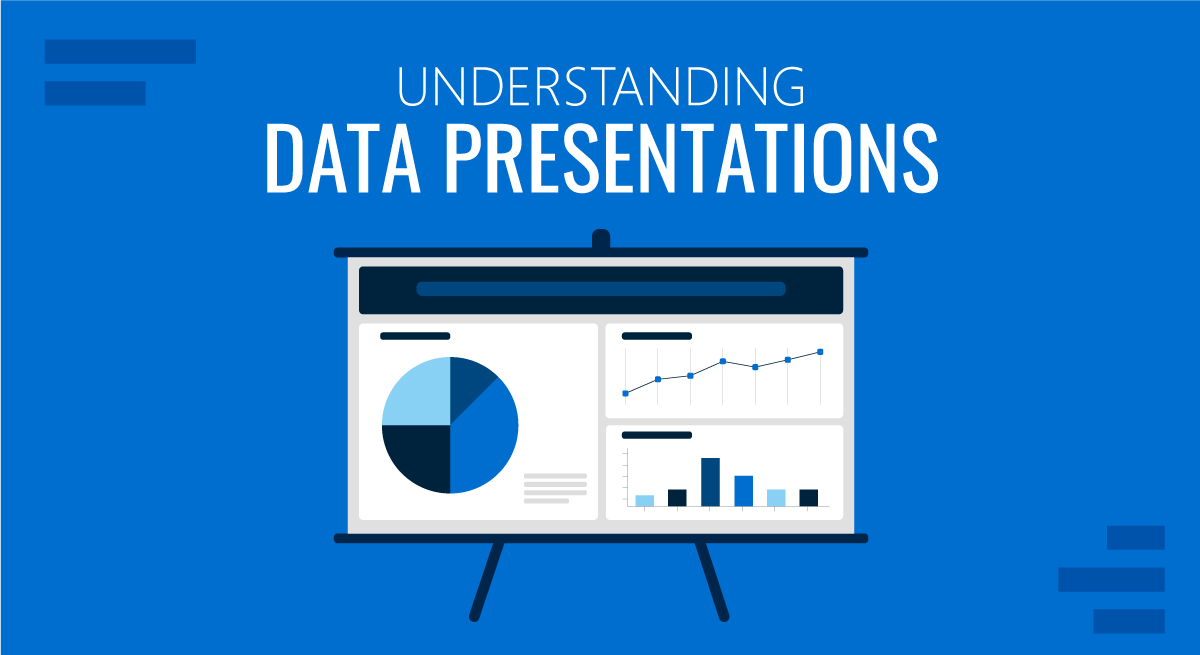
In this age of overwhelming information, the skill to effectively convey data has become extremely valuable. Initiating a discussion on data presentation types involves thoughtful consideration of the nature of your data and the message you aim to convey. Different types of visualizations serve distinct purposes. Whether you’re dealing with how to develop a report or simply trying to communicate complex information, how you present data influences how well your audience understands and engages with it. This extensive guide leads you through the different ways of data presentation.
Table of Contents
What is a Data Presentation?
What should a data presentation include, line graphs, treemap chart, scatter plot, how to choose a data presentation type, recommended data presentation templates, common mistakes done in data presentation.
A data presentation is a slide deck that aims to disclose quantitative information to an audience through the use of visual formats and narrative techniques derived from data analysis, making complex data understandable and actionable. This process requires a series of tools, such as charts, graphs, tables, infographics, dashboards, and so on, supported by concise textual explanations to improve understanding and boost retention rate.
Data presentations require us to cull data in a format that allows the presenter to highlight trends, patterns, and insights so that the audience can act upon the shared information. In a few words, the goal of data presentations is to enable viewers to grasp complicated concepts or trends quickly, facilitating informed decision-making or deeper analysis.
Data presentations go beyond the mere usage of graphical elements. Seasoned presenters encompass visuals with the art of data storytelling , so the speech skillfully connects the points through a narrative that resonates with the audience. Depending on the purpose – inspire, persuade, inform, support decision-making processes, etc. – is the data presentation format that is better suited to help us in this journey.
To nail your upcoming data presentation, ensure to count with the following elements:
- Clear Objectives: Understand the intent of your presentation before selecting the graphical layout and metaphors to make content easier to grasp.
- Engaging introduction: Use a powerful hook from the get-go. For instance, you can ask a big question or present a problem that your data will answer. Take a look at our guide on how to start a presentation for tips & insights.
- Structured Narrative: Your data presentation must tell a coherent story. This means a beginning where you present the context, a middle section in which you present the data, and an ending that uses a call-to-action. Check our guide on presentation structure for further information.
- Visual Elements: These are the charts, graphs, and other elements of visual communication we ought to use to present data. This article will cover one by one the different types of data representation methods we can use, and provide further guidance on choosing between them.
- Insights and Analysis: This is not just showcasing a graph and letting people get an idea about it. A proper data presentation includes the interpretation of that data, the reason why it’s included, and why it matters to your research.
- Conclusion & CTA: Ending your presentation with a call to action is necessary. Whether you intend to wow your audience into acquiring your services, inspire them to change the world, or whatever the purpose of your presentation, there must be a stage in which you convey all that you shared and show the path to staying in touch. Plan ahead whether you want to use a thank-you slide, a video presentation, or which method is apt and tailored to the kind of presentation you deliver.
- Q&A Session: After your speech is concluded, allocate 3-5 minutes for the audience to raise any questions about the information you disclosed. This is an extra chance to establish your authority on the topic. Check our guide on questions and answer sessions in presentations here.
Bar charts are a graphical representation of data using rectangular bars to show quantities or frequencies in an established category. They make it easy for readers to spot patterns or trends. Bar charts can be horizontal or vertical, although the vertical format is commonly known as a column chart. They display categorical, discrete, or continuous variables grouped in class intervals [1] . They include an axis and a set of labeled bars horizontally or vertically. These bars represent the frequencies of variable values or the values themselves. Numbers on the y-axis of a vertical bar chart or the x-axis of a horizontal bar chart are called the scale.
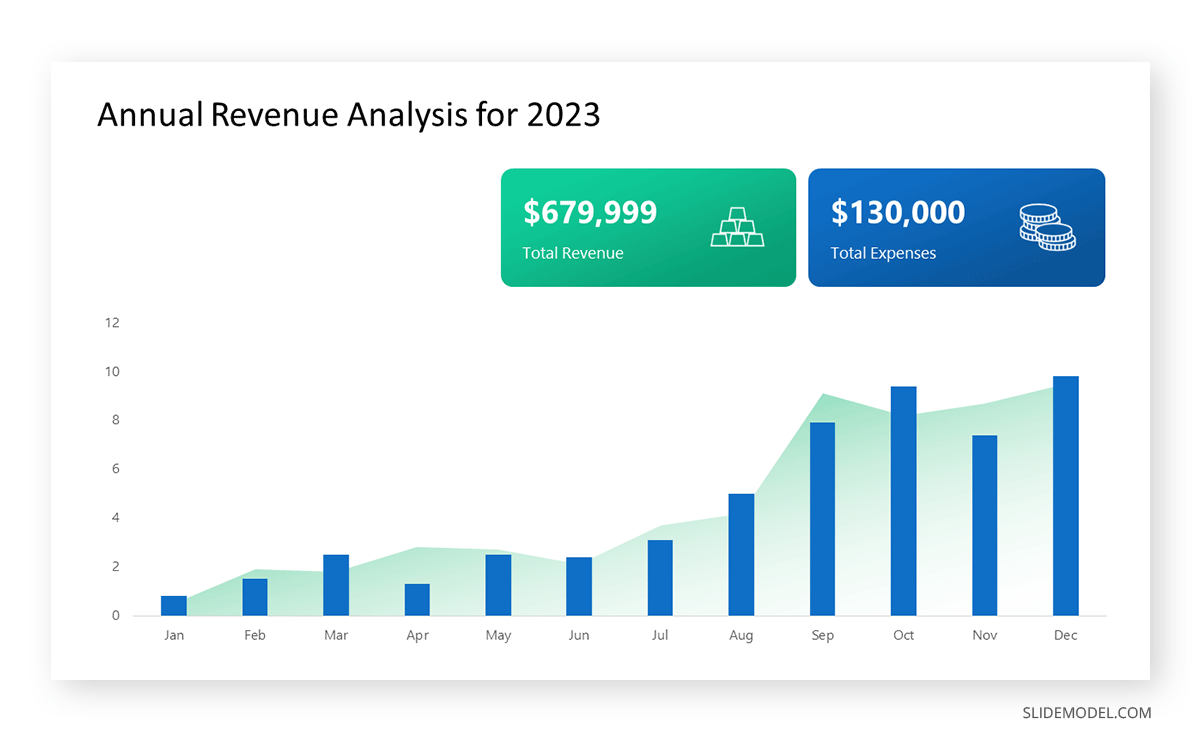
Real-Life Application of Bar Charts
Let’s say a sales manager is presenting sales to their audience. Using a bar chart, he follows these steps.
Step 1: Selecting Data
The first step is to identify the specific data you will present to your audience.
The sales manager has highlighted these products for the presentation.
- Product A: Men’s Shoes
- Product B: Women’s Apparel
- Product C: Electronics
- Product D: Home Decor
Step 2: Choosing Orientation
Opt for a vertical layout for simplicity. Vertical bar charts help compare different categories in case there are not too many categories [1] . They can also help show different trends. A vertical bar chart is used where each bar represents one of the four chosen products. After plotting the data, it is seen that the height of each bar directly represents the sales performance of the respective product.
It is visible that the tallest bar (Electronics – Product C) is showing the highest sales. However, the shorter bars (Women’s Apparel – Product B and Home Decor – Product D) need attention. It indicates areas that require further analysis or strategies for improvement.
Step 3: Colorful Insights
Different colors are used to differentiate each product. It is essential to show a color-coded chart where the audience can distinguish between products.
- Men’s Shoes (Product A): Yellow
- Women’s Apparel (Product B): Orange
- Electronics (Product C): Violet
- Home Decor (Product D): Blue
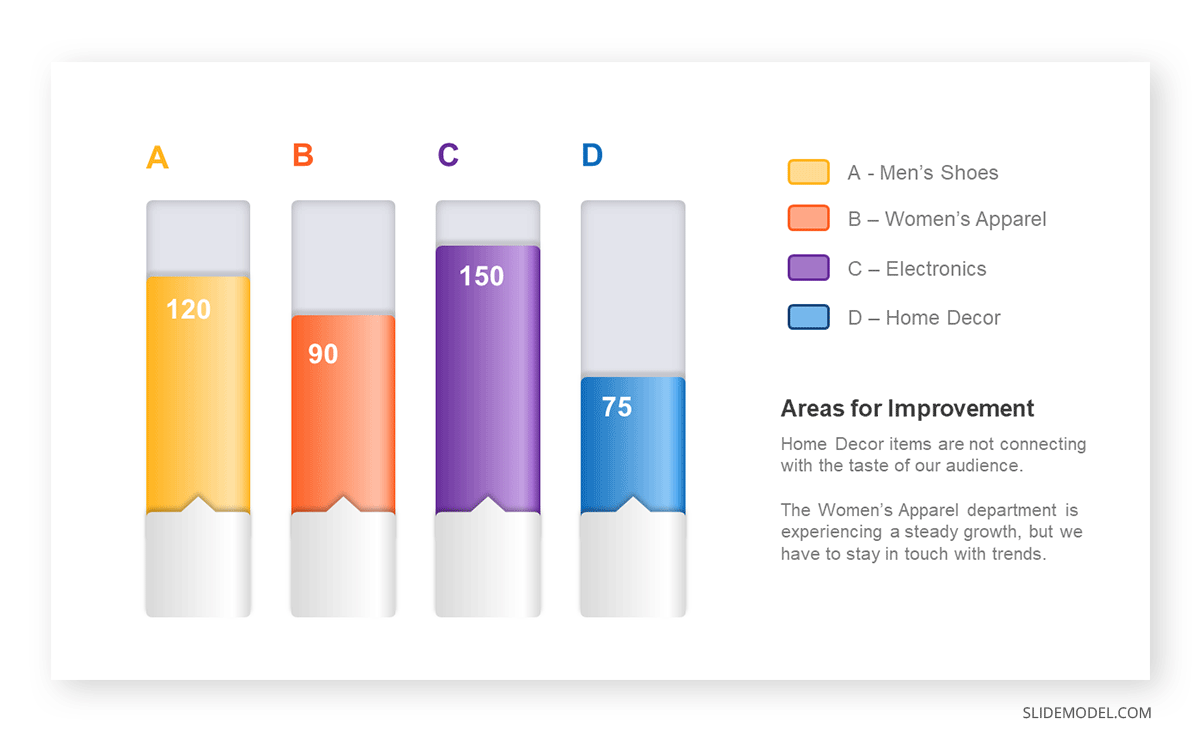
Bar charts are straightforward and easily understandable for presenting data. They are versatile when comparing products or any categorical data [2] . Bar charts adapt seamlessly to retail scenarios. Despite that, bar charts have a few shortcomings. They cannot illustrate data trends over time. Besides, overloading the chart with numerous products can lead to visual clutter, diminishing its effectiveness.
For more information, check our collection of bar chart templates for PowerPoint .
Line graphs help illustrate data trends, progressions, or fluctuations by connecting a series of data points called ‘markers’ with straight line segments. This provides a straightforward representation of how values change [5] . Their versatility makes them invaluable for scenarios requiring a visual understanding of continuous data. In addition, line graphs are also useful for comparing multiple datasets over the same timeline. Using multiple line graphs allows us to compare more than one data set. They simplify complex information so the audience can quickly grasp the ups and downs of values. From tracking stock prices to analyzing experimental results, you can use line graphs to show how data changes over a continuous timeline. They show trends with simplicity and clarity.
Real-life Application of Line Graphs
To understand line graphs thoroughly, we will use a real case. Imagine you’re a financial analyst presenting a tech company’s monthly sales for a licensed product over the past year. Investors want insights into sales behavior by month, how market trends may have influenced sales performance and reception to the new pricing strategy. To present data via a line graph, you will complete these steps.
First, you need to gather the data. In this case, your data will be the sales numbers. For example:
- January: $45,000
- February: $55,000
- March: $45,000
- April: $60,000
- May: $ 70,000
- June: $65,000
- July: $62,000
- August: $68,000
- September: $81,000
- October: $76,000
- November: $87,000
- December: $91,000
After choosing the data, the next step is to select the orientation. Like bar charts, you can use vertical or horizontal line graphs. However, we want to keep this simple, so we will keep the timeline (x-axis) horizontal while the sales numbers (y-axis) vertical.
Step 3: Connecting Trends
After adding the data to your preferred software, you will plot a line graph. In the graph, each month’s sales are represented by data points connected by a line.
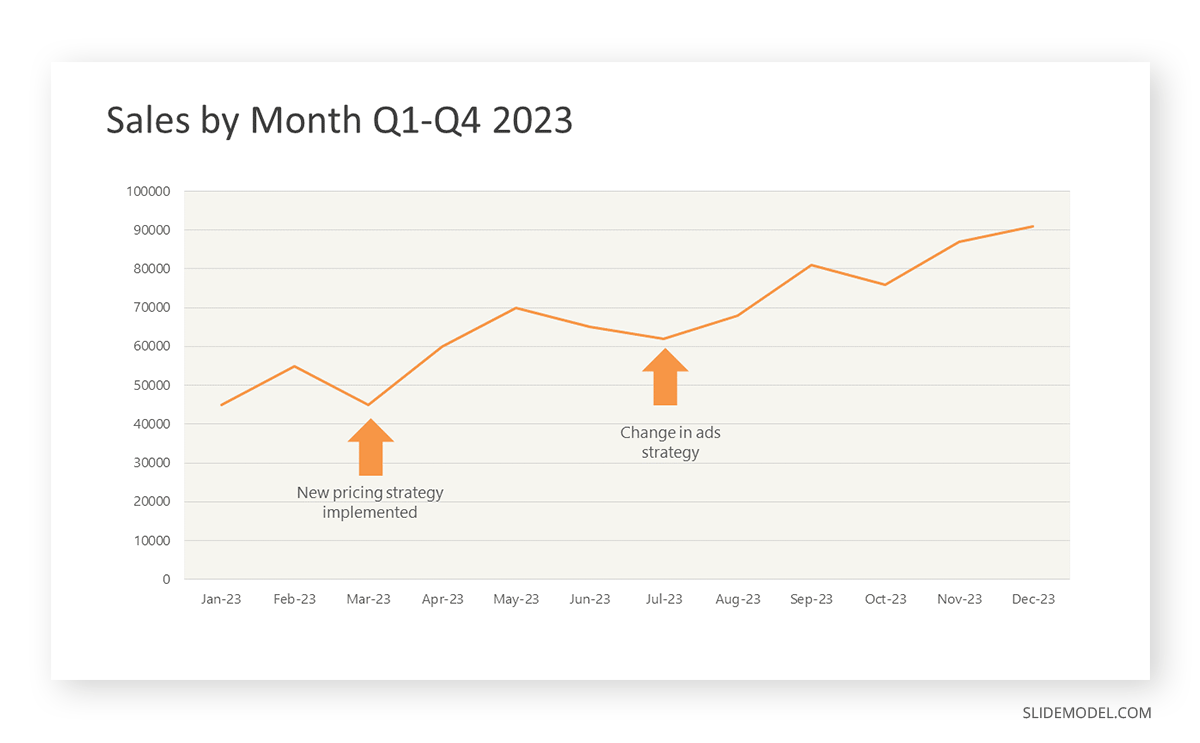
Step 4: Adding Clarity with Color
If there are multiple lines, you can also add colors to highlight each one, making it easier to follow.
Line graphs excel at visually presenting trends over time. These presentation aids identify patterns, like upward or downward trends. However, too many data points can clutter the graph, making it harder to interpret. Line graphs work best with continuous data but are not suitable for categories.
For more information, check our collection of line chart templates for PowerPoint and our article about how to make a presentation graph .
A data dashboard is a visual tool for analyzing information. Different graphs, charts, and tables are consolidated in a layout to showcase the information required to achieve one or more objectives. Dashboards help quickly see Key Performance Indicators (KPIs). You don’t make new visuals in the dashboard; instead, you use it to display visuals you’ve already made in worksheets [3] .
Keeping the number of visuals on a dashboard to three or four is recommended. Adding too many can make it hard to see the main points [4]. Dashboards can be used for business analytics to analyze sales, revenue, and marketing metrics at a time. They are also used in the manufacturing industry, as they allow users to grasp the entire production scenario at the moment while tracking the core KPIs for each line.
Real-Life Application of a Dashboard
Consider a project manager presenting a software development project’s progress to a tech company’s leadership team. He follows the following steps.
Step 1: Defining Key Metrics
To effectively communicate the project’s status, identify key metrics such as completion status, budget, and bug resolution rates. Then, choose measurable metrics aligned with project objectives.
Step 2: Choosing Visualization Widgets
After finalizing the data, presentation aids that align with each metric are selected. For this project, the project manager chooses a progress bar for the completion status and uses bar charts for budget allocation. Likewise, he implements line charts for bug resolution rates.
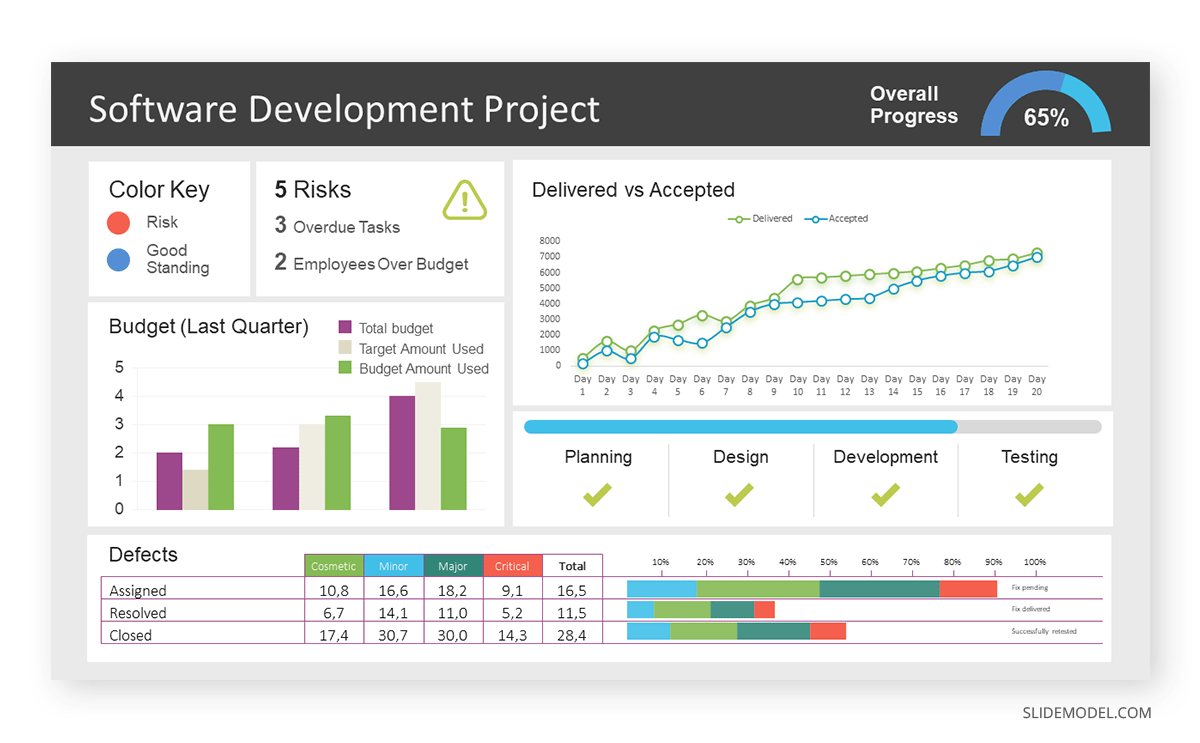
Step 3: Dashboard Layout
Key metrics are prominently placed in the dashboard for easy visibility, and the manager ensures that it appears clean and organized.
Dashboards provide a comprehensive view of key project metrics. Users can interact with data, customize views, and drill down for detailed analysis. However, creating an effective dashboard requires careful planning to avoid clutter. Besides, dashboards rely on the availability and accuracy of underlying data sources.
For more information, check our article on how to design a dashboard presentation , and discover our collection of dashboard PowerPoint templates .
Treemap charts represent hierarchical data structured in a series of nested rectangles [6] . As each branch of the ‘tree’ is given a rectangle, smaller tiles can be seen representing sub-branches, meaning elements on a lower hierarchical level than the parent rectangle. Each one of those rectangular nodes is built by representing an area proportional to the specified data dimension.
Treemaps are useful for visualizing large datasets in compact space. It is easy to identify patterns, such as which categories are dominant. Common applications of the treemap chart are seen in the IT industry, such as resource allocation, disk space management, website analytics, etc. Also, they can be used in multiple industries like healthcare data analysis, market share across different product categories, or even in finance to visualize portfolios.
Real-Life Application of a Treemap Chart
Let’s consider a financial scenario where a financial team wants to represent the budget allocation of a company. There is a hierarchy in the process, so it is helpful to use a treemap chart. In the chart, the top-level rectangle could represent the total budget, and it would be subdivided into smaller rectangles, each denoting a specific department. Further subdivisions within these smaller rectangles might represent individual projects or cost categories.
Step 1: Define Your Data Hierarchy
While presenting data on the budget allocation, start by outlining the hierarchical structure. The sequence will be like the overall budget at the top, followed by departments, projects within each department, and finally, individual cost categories for each project.
- Top-level rectangle: Total Budget
- Second-level rectangles: Departments (Engineering, Marketing, Sales)
- Third-level rectangles: Projects within each department
- Fourth-level rectangles: Cost categories for each project (Personnel, Marketing Expenses, Equipment)
Step 2: Choose a Suitable Tool
It’s time to select a data visualization tool supporting Treemaps. Popular choices include Tableau, Microsoft Power BI, PowerPoint, or even coding with libraries like D3.js. It is vital to ensure that the chosen tool provides customization options for colors, labels, and hierarchical structures.
Here, the team uses PowerPoint for this guide because of its user-friendly interface and robust Treemap capabilities.
Step 3: Make a Treemap Chart with PowerPoint
After opening the PowerPoint presentation, they chose “SmartArt” to form the chart. The SmartArt Graphic window has a “Hierarchy” category on the left. Here, you will see multiple options. You can choose any layout that resembles a Treemap. The “Table Hierarchy” or “Organization Chart” options can be adapted. The team selects the Table Hierarchy as it looks close to a Treemap.
Step 5: Input Your Data
After that, a new window will open with a basic structure. They add the data one by one by clicking on the text boxes. They start with the top-level rectangle, representing the total budget.
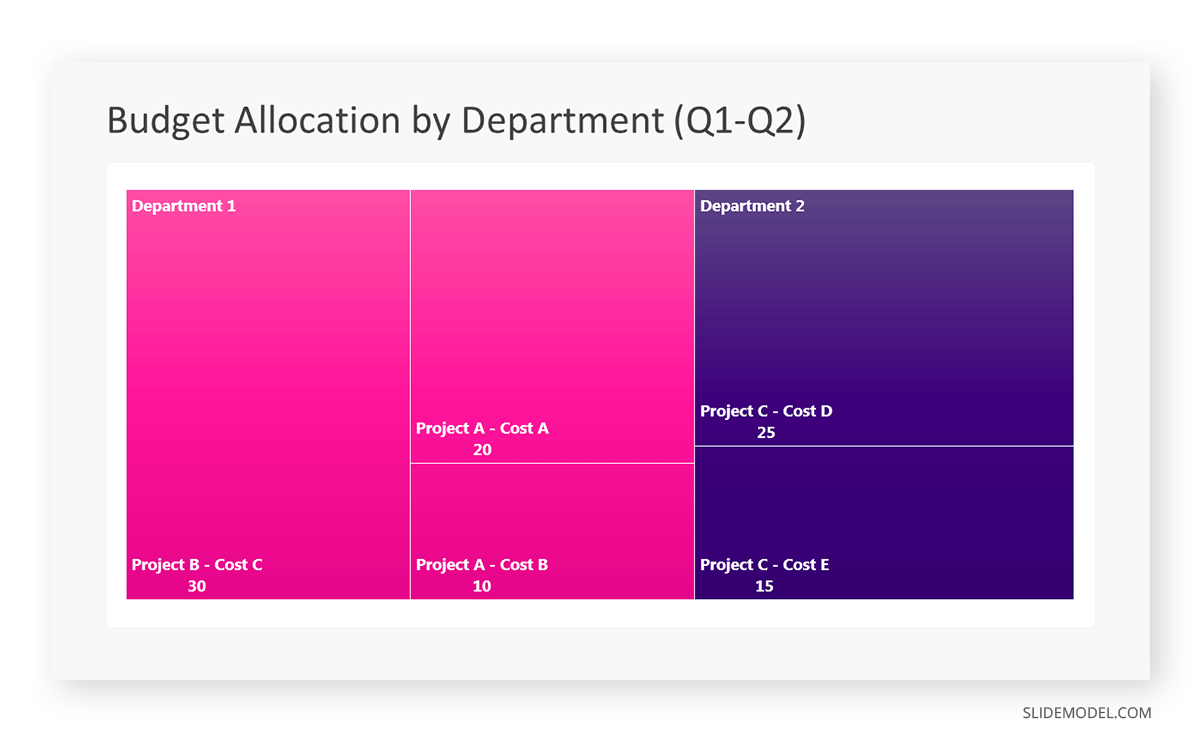
Step 6: Customize the Treemap
By clicking on each shape, they customize its color, size, and label. At the same time, they can adjust the font size, style, and color of labels by using the options in the “Format” tab in PowerPoint. Using different colors for each level enhances the visual difference.
Treemaps excel at illustrating hierarchical structures. These charts make it easy to understand relationships and dependencies. They efficiently use space, compactly displaying a large amount of data, reducing the need for excessive scrolling or navigation. Additionally, using colors enhances the understanding of data by representing different variables or categories.
In some cases, treemaps might become complex, especially with deep hierarchies. It becomes challenging for some users to interpret the chart. At the same time, displaying detailed information within each rectangle might be constrained by space. It potentially limits the amount of data that can be shown clearly. Without proper labeling and color coding, there’s a risk of misinterpretation.
A heatmap is a data visualization tool that uses color coding to represent values across a two-dimensional surface. In these, colors replace numbers to indicate the magnitude of each cell. This color-shaded matrix display is valuable for summarizing and understanding data sets with a glance [7] . The intensity of the color corresponds to the value it represents, making it easy to identify patterns, trends, and variations in the data.
As a tool, heatmaps help businesses analyze website interactions, revealing user behavior patterns and preferences to enhance overall user experience. In addition, companies use heatmaps to assess content engagement, identifying popular sections and areas of improvement for more effective communication. They excel at highlighting patterns and trends in large datasets, making it easy to identify areas of interest.
We can implement heatmaps to express multiple data types, such as numerical values, percentages, or even categorical data. Heatmaps help us easily spot areas with lots of activity, making them helpful in figuring out clusters [8] . When making these maps, it is important to pick colors carefully. The colors need to show the differences between groups or levels of something. And it is good to use colors that people with colorblindness can easily see.
Check our detailed guide on how to create a heatmap here. Also discover our collection of heatmap PowerPoint templates .
Pie charts are circular statistical graphics divided into slices to illustrate numerical proportions. Each slice represents a proportionate part of the whole, making it easy to visualize the contribution of each component to the total.
The size of the pie charts is influenced by the value of data points within each pie. The total of all data points in a pie determines its size. The pie with the highest data points appears as the largest, whereas the others are proportionally smaller. However, you can present all pies of the same size if proportional representation is not required [9] . Sometimes, pie charts are difficult to read, or additional information is required. A variation of this tool can be used instead, known as the donut chart , which has the same structure but a blank center, creating a ring shape. Presenters can add extra information, and the ring shape helps to declutter the graph.
Pie charts are used in business to show percentage distribution, compare relative sizes of categories, or present straightforward data sets where visualizing ratios is essential.
Real-Life Application of Pie Charts
Consider a scenario where you want to represent the distribution of the data. Each slice of the pie chart would represent a different category, and the size of each slice would indicate the percentage of the total portion allocated to that category.
Step 1: Define Your Data Structure
Imagine you are presenting the distribution of a project budget among different expense categories.
- Column A: Expense Categories (Personnel, Equipment, Marketing, Miscellaneous)
- Column B: Budget Amounts ($40,000, $30,000, $20,000, $10,000) Column B represents the values of your categories in Column A.
Step 2: Insert a Pie Chart
Using any of the accessible tools, you can create a pie chart. The most convenient tools for forming a pie chart in a presentation are presentation tools such as PowerPoint or Google Slides. You will notice that the pie chart assigns each expense category a percentage of the total budget by dividing it by the total budget.
For instance:
- Personnel: $40,000 / ($40,000 + $30,000 + $20,000 + $10,000) = 40%
- Equipment: $30,000 / ($40,000 + $30,000 + $20,000 + $10,000) = 30%
- Marketing: $20,000 / ($40,000 + $30,000 + $20,000 + $10,000) = 20%
- Miscellaneous: $10,000 / ($40,000 + $30,000 + $20,000 + $10,000) = 10%
You can make a chart out of this or just pull out the pie chart from the data.
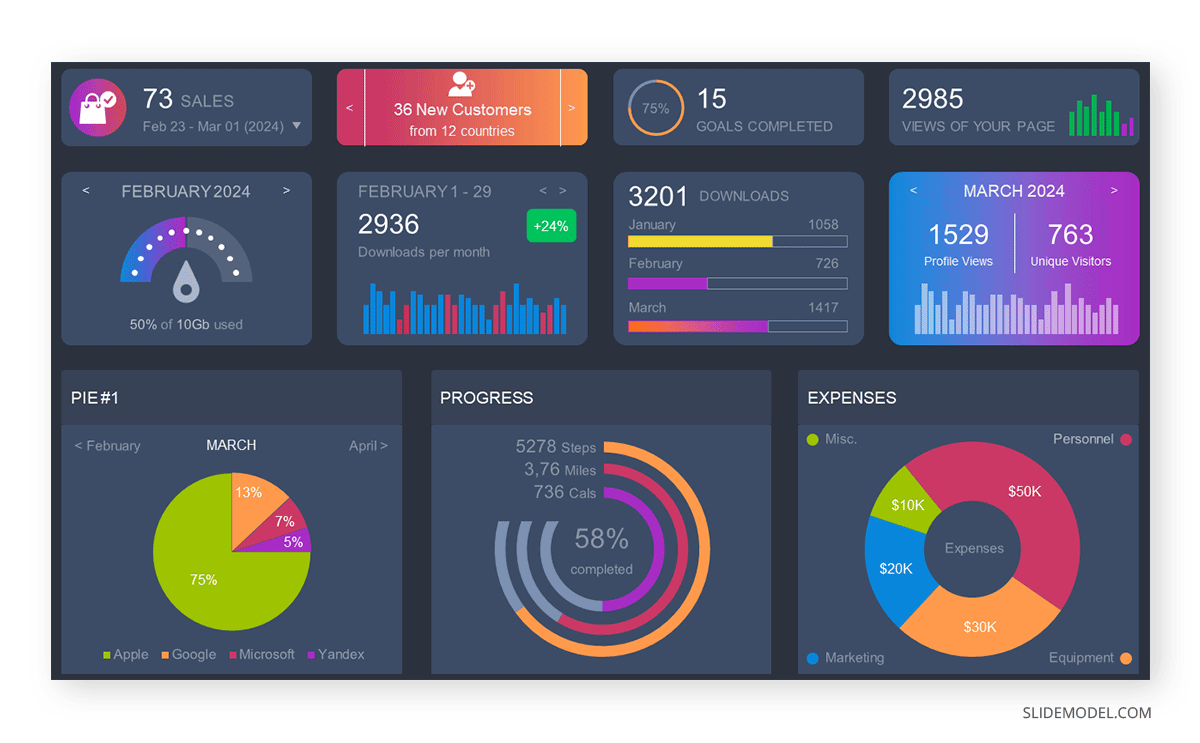
3D pie charts and 3D donut charts are quite popular among the audience. They stand out as visual elements in any presentation slide, so let’s take a look at how our pie chart example would look in 3D pie chart format.
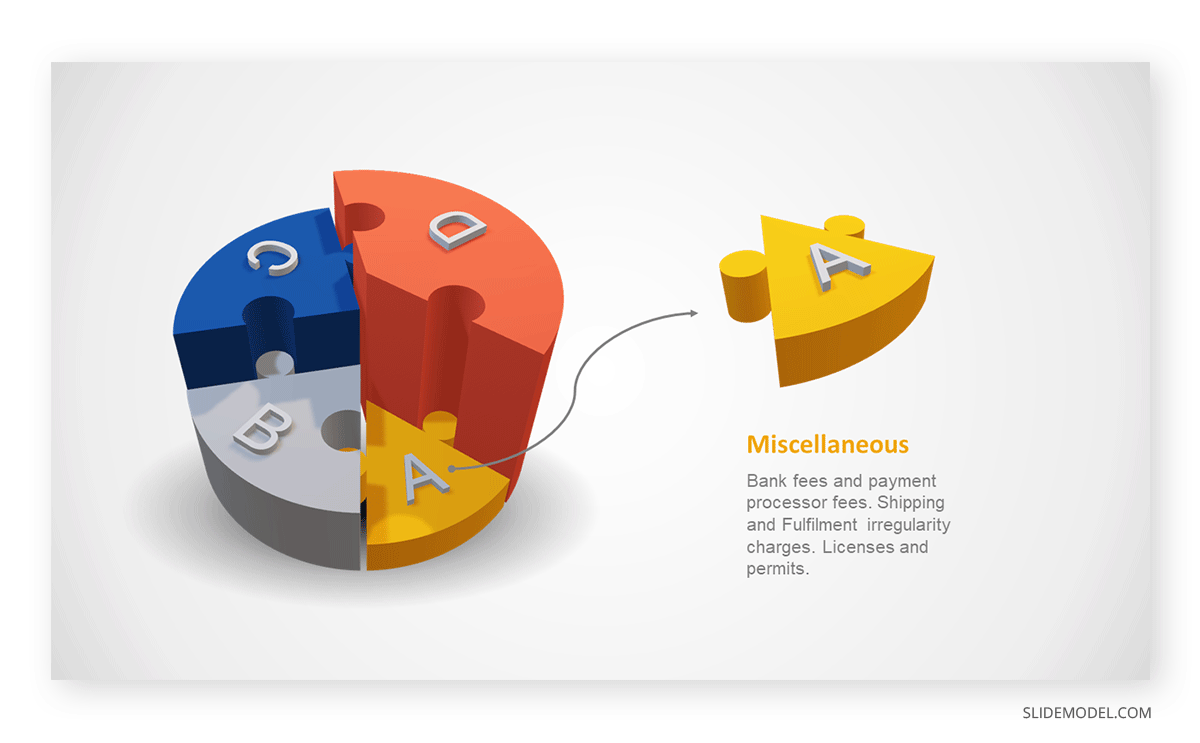
Step 03: Results Interpretation
The pie chart visually illustrates the distribution of the project budget among different expense categories. Personnel constitutes the largest portion at 40%, followed by equipment at 30%, marketing at 20%, and miscellaneous at 10%. This breakdown provides a clear overview of where the project funds are allocated, which helps in informed decision-making and resource management. It is evident that personnel are a significant investment, emphasizing their importance in the overall project budget.
Pie charts provide a straightforward way to represent proportions and percentages. They are easy to understand, even for individuals with limited data analysis experience. These charts work well for small datasets with a limited number of categories.
However, a pie chart can become cluttered and less effective in situations with many categories. Accurate interpretation may be challenging, especially when dealing with slight differences in slice sizes. In addition, these charts are static and do not effectively convey trends over time.
For more information, check our collection of pie chart templates for PowerPoint .
Histograms present the distribution of numerical variables. Unlike a bar chart that records each unique response separately, histograms organize numeric responses into bins and show the frequency of reactions within each bin [10] . The x-axis of a histogram shows the range of values for a numeric variable. At the same time, the y-axis indicates the relative frequencies (percentage of the total counts) for that range of values.
Whenever you want to understand the distribution of your data, check which values are more common, or identify outliers, histograms are your go-to. Think of them as a spotlight on the story your data is telling. A histogram can provide a quick and insightful overview if you’re curious about exam scores, sales figures, or any numerical data distribution.
Real-Life Application of a Histogram
In the histogram data analysis presentation example, imagine an instructor analyzing a class’s grades to identify the most common score range. A histogram could effectively display the distribution. It will show whether most students scored in the average range or if there are significant outliers.
Step 1: Gather Data
He begins by gathering the data. The scores of each student in class are gathered to analyze exam scores.
Names | Score |
---|---|
Alice | 78 |
Bob | 85 |
Clara | 92 |
David | 65 |
Emma | 72 |
Frank | 88 |
Grace | 76 |
Henry | 95 |
Isabel | 81 |
Jack | 70 |
Kate | 60 |
Liam | 89 |
Mia | 75 |
Noah | 84 |
Olivia | 92 |
After arranging the scores in ascending order, bin ranges are set.
Step 2: Define Bins
Bins are like categories that group similar values. Think of them as buckets that organize your data. The presenter decides how wide each bin should be based on the range of the values. For instance, the instructor sets the bin ranges based on score intervals: 60-69, 70-79, 80-89, and 90-100.
Step 3: Count Frequency
Now, he counts how many data points fall into each bin. This step is crucial because it tells you how often specific ranges of values occur. The result is the frequency distribution, showing the occurrences of each group.
Here, the instructor counts the number of students in each category.
- 60-69: 1 student (Kate)
- 70-79: 4 students (David, Emma, Grace, Jack)
- 80-89: 7 students (Alice, Bob, Frank, Isabel, Liam, Mia, Noah)
- 90-100: 3 students (Clara, Henry, Olivia)
Step 4: Create the Histogram
It’s time to turn the data into a visual representation. Draw a bar for each bin on a graph. The width of the bar should correspond to the range of the bin, and the height should correspond to the frequency. To make your histogram understandable, label the X and Y axes.
In this case, the X-axis should represent the bins (e.g., test score ranges), and the Y-axis represents the frequency.
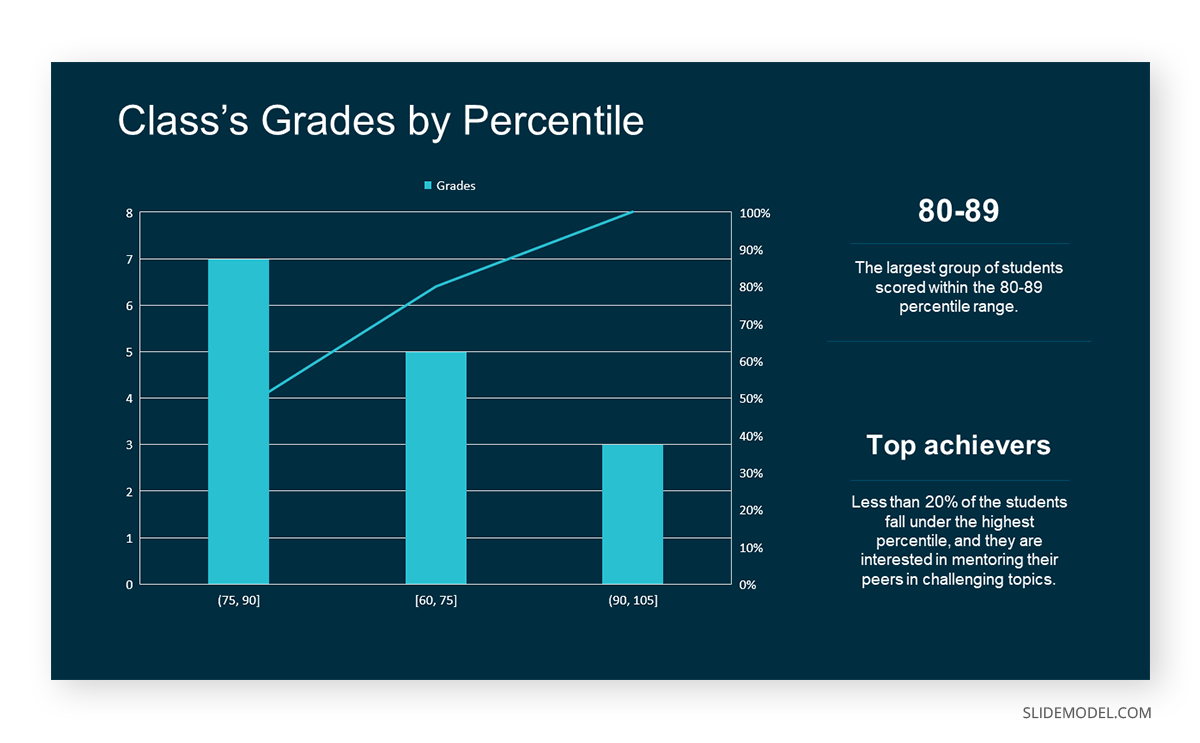
The histogram of the class grades reveals insightful patterns in the distribution. Most students, with seven students, fall within the 80-89 score range. The histogram provides a clear visualization of the class’s performance. It showcases a concentration of grades in the upper-middle range with few outliers at both ends. This analysis helps in understanding the overall academic standing of the class. It also identifies the areas for potential improvement or recognition.
Thus, histograms provide a clear visual representation of data distribution. They are easy to interpret, even for those without a statistical background. They apply to various types of data, including continuous and discrete variables. One weak point is that histograms do not capture detailed patterns in students’ data, with seven compared to other visualization methods.
A scatter plot is a graphical representation of the relationship between two variables. It consists of individual data points on a two-dimensional plane. This plane plots one variable on the x-axis and the other on the y-axis. Each point represents a unique observation. It visualizes patterns, trends, or correlations between the two variables.
Scatter plots are also effective in revealing the strength and direction of relationships. They identify outliers and assess the overall distribution of data points. The points’ dispersion and clustering reflect the relationship’s nature, whether it is positive, negative, or lacks a discernible pattern. In business, scatter plots assess relationships between variables such as marketing cost and sales revenue. They help present data correlations and decision-making.
Real-Life Application of Scatter Plot
A group of scientists is conducting a study on the relationship between daily hours of screen time and sleep quality. After reviewing the data, they managed to create this table to help them build a scatter plot graph:
Participant ID | Daily Hours of Screen Time | Sleep Quality Rating |
---|---|---|
1 | 9 | 3 |
2 | 2 | 8 |
3 | 1 | 9 |
4 | 0 | 10 |
5 | 1 | 9 |
6 | 3 | 7 |
7 | 4 | 7 |
8 | 5 | 6 |
9 | 5 | 6 |
10 | 7 | 3 |
11 | 10 | 1 |
12 | 6 | 5 |
13 | 7 | 3 |
14 | 8 | 2 |
15 | 9 | 2 |
16 | 4 | 7 |
17 | 5 | 6 |
18 | 4 | 7 |
19 | 9 | 2 |
20 | 6 | 4 |
21 | 3 | 7 |
22 | 10 | 1 |
23 | 2 | 8 |
24 | 5 | 6 |
25 | 3 | 7 |
26 | 1 | 9 |
27 | 8 | 2 |
28 | 4 | 6 |
29 | 7 | 3 |
30 | 2 | 8 |
31 | 7 | 4 |
32 | 9 | 2 |
33 | 10 | 1 |
34 | 10 | 1 |
35 | 10 | 1 |
In the provided example, the x-axis represents Daily Hours of Screen Time, and the y-axis represents the Sleep Quality Rating.
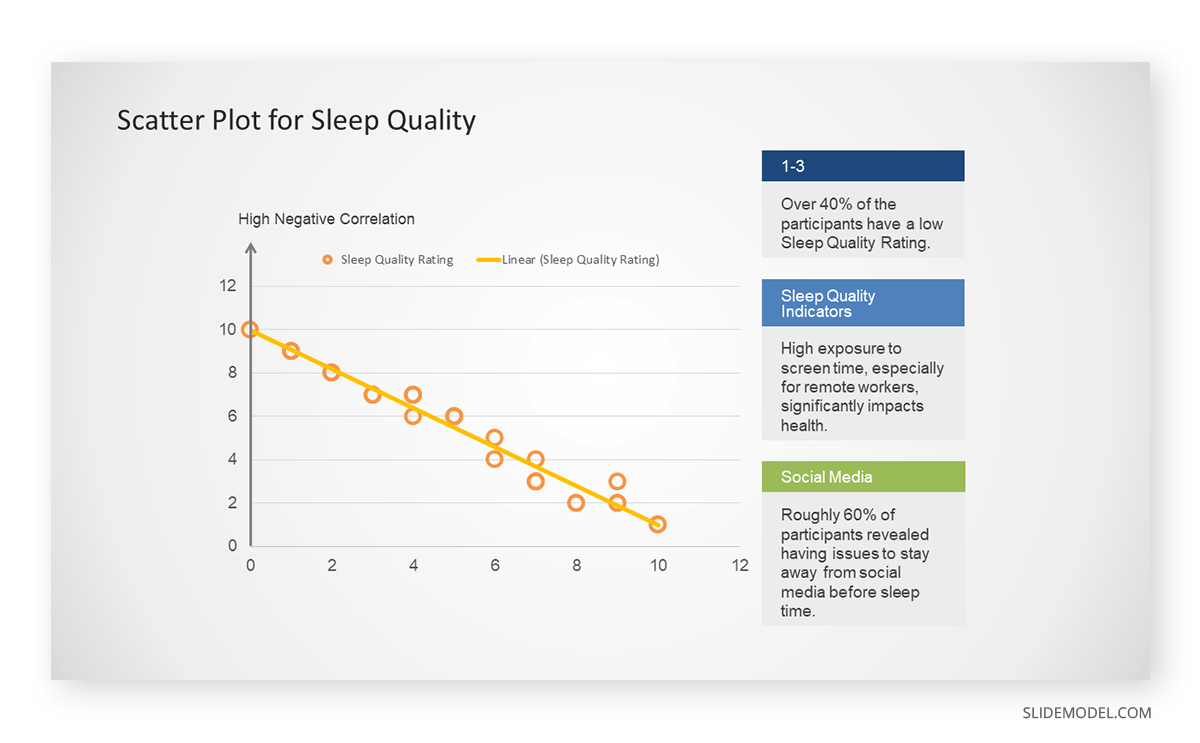
The scientists observe a negative correlation between the amount of screen time and the quality of sleep. This is consistent with their hypothesis that blue light, especially before bedtime, has a significant impact on sleep quality and metabolic processes.
There are a few things to remember when using a scatter plot. Even when a scatter diagram indicates a relationship, it doesn’t mean one variable affects the other. A third factor can influence both variables. The more the plot resembles a straight line, the stronger the relationship is perceived [11] . If it suggests no ties, the observed pattern might be due to random fluctuations in data. When the scatter diagram depicts no correlation, whether the data might be stratified is worth considering.
Choosing the appropriate data presentation type is crucial when making a presentation . Understanding the nature of your data and the message you intend to convey will guide this selection process. For instance, when showcasing quantitative relationships, scatter plots become instrumental in revealing correlations between variables. If the focus is on emphasizing parts of a whole, pie charts offer a concise display of proportions. Histograms, on the other hand, prove valuable for illustrating distributions and frequency patterns.
Bar charts provide a clear visual comparison of different categories. Likewise, line charts excel in showcasing trends over time, while tables are ideal for detailed data examination. Starting a presentation on data presentation types involves evaluating the specific information you want to communicate and selecting the format that aligns with your message. This ensures clarity and resonance with your audience from the beginning of your presentation.
1. Fact Sheet Dashboard for Data Presentation
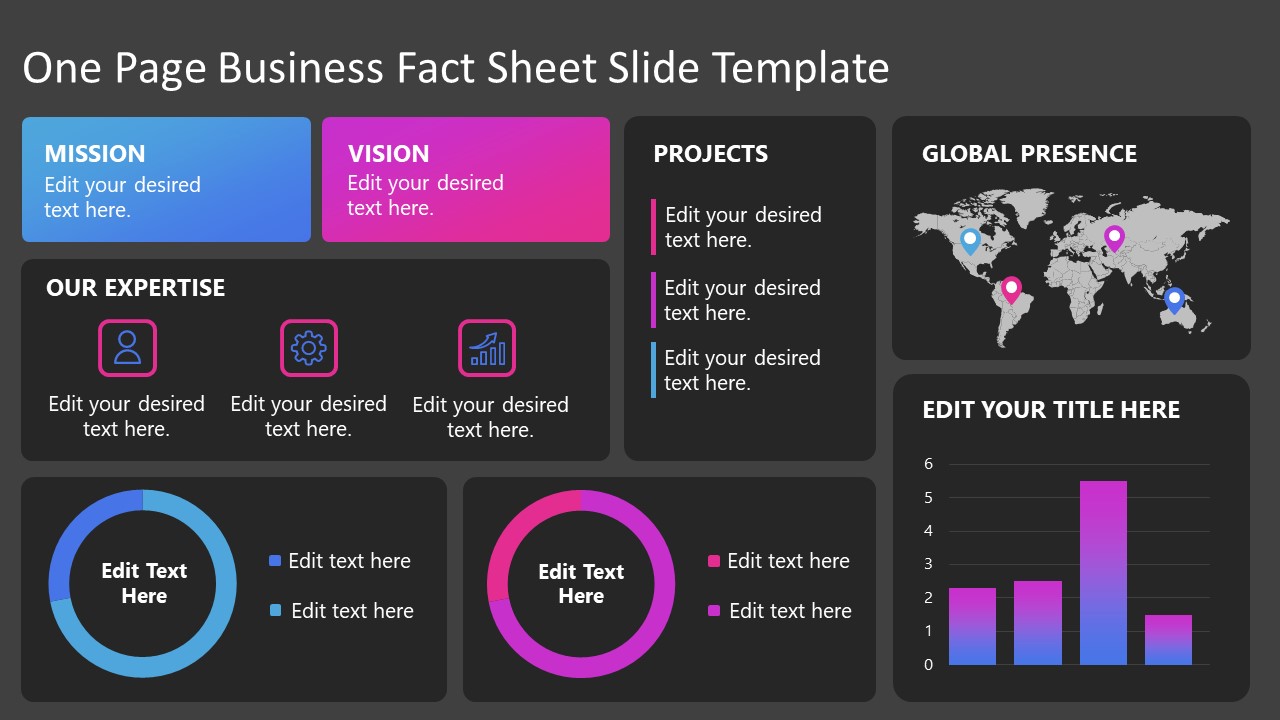
Convey all the data you need to present in this one-pager format, an ideal solution tailored for users looking for presentation aids. Global maps, donut chats, column graphs, and text neatly arranged in a clean layout presented in light and dark themes.
Use This Template
2. 3D Column Chart Infographic PPT Template
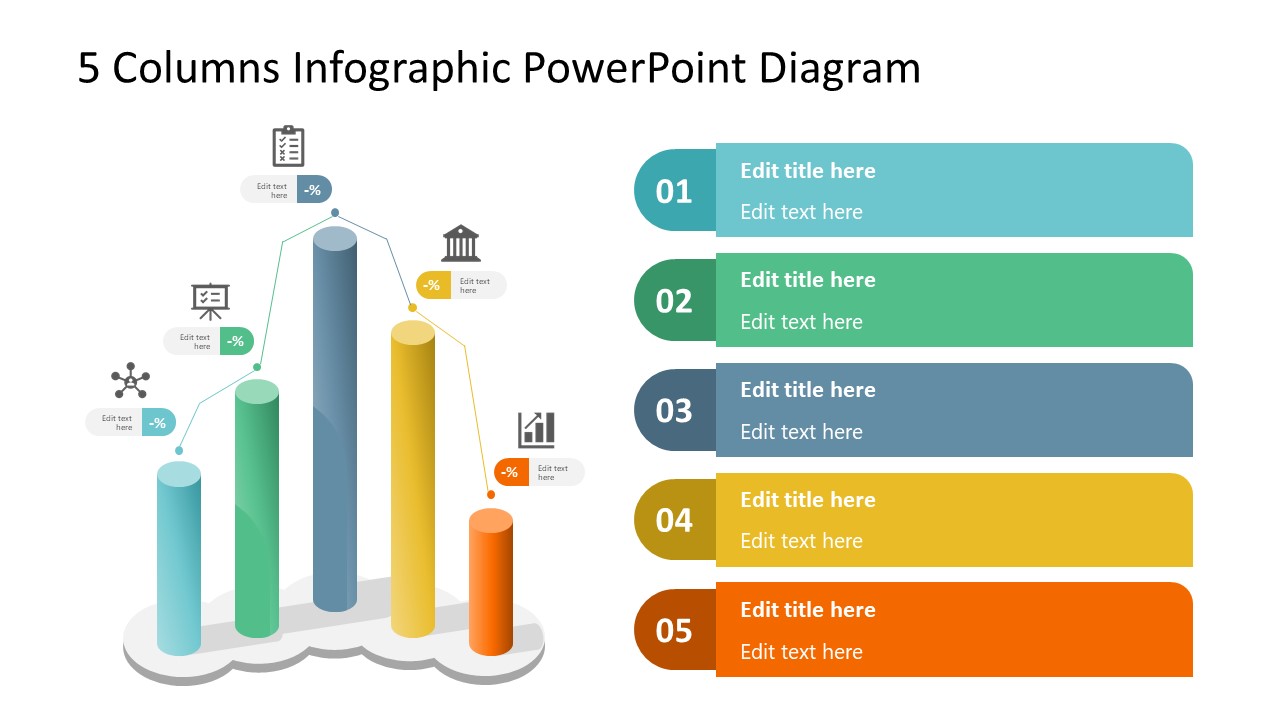
Represent column charts in a highly visual 3D format with this PPT template. A creative way to present data, this template is entirely editable, and we can craft either a one-page infographic or a series of slides explaining what we intend to disclose point by point.
3. Data Circles Infographic PowerPoint Template
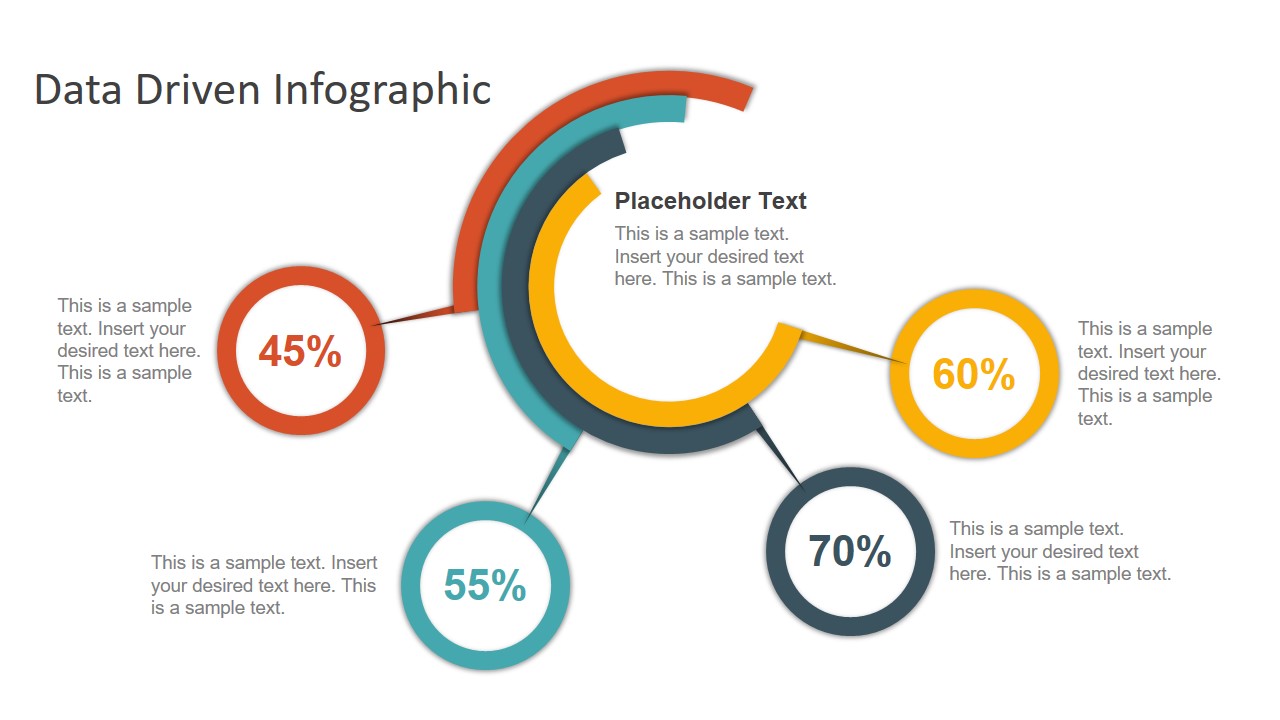
An alternative to the pie chart and donut chart diagrams, this template features a series of curved shapes with bubble callouts as ways of presenting data. Expand the information for each arch in the text placeholder areas.
4. Colorful Metrics Dashboard for Data Presentation
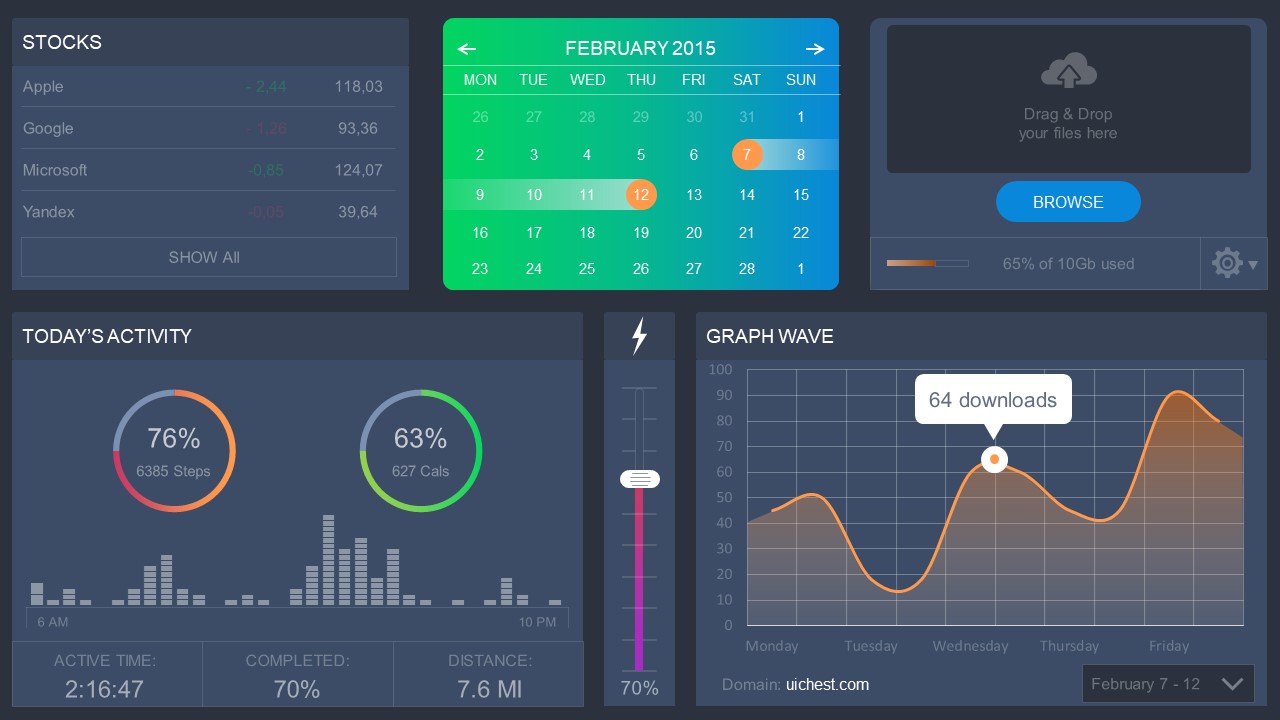
This versatile dashboard template helps us in the presentation of the data by offering several graphs and methods to convert numbers into graphics. Implement it for e-commerce projects, financial projections, project development, and more.
5. Animated Data Presentation Tools for PowerPoint & Google Slides
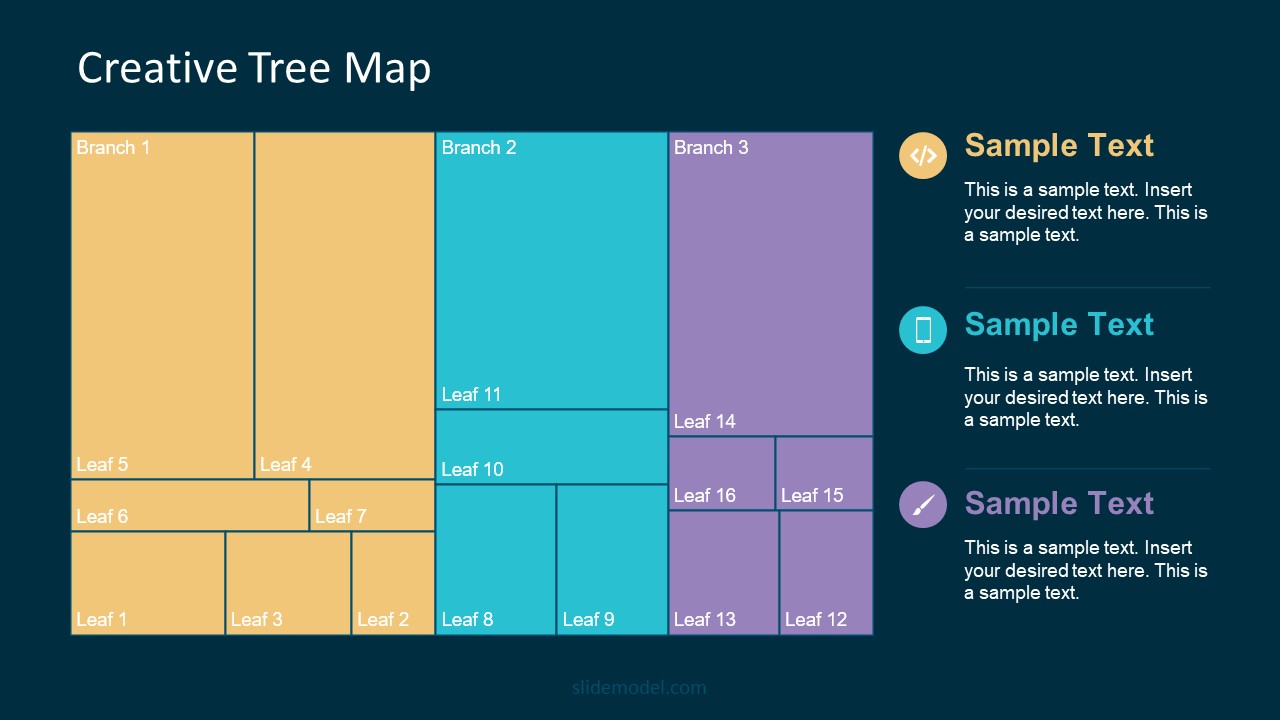
A slide deck filled with most of the tools mentioned in this article, from bar charts, column charts, treemap graphs, pie charts, histogram, etc. Animated effects make each slide look dynamic when sharing data with stakeholders.
6. Statistics Waffle Charts PPT Template for Data Presentations
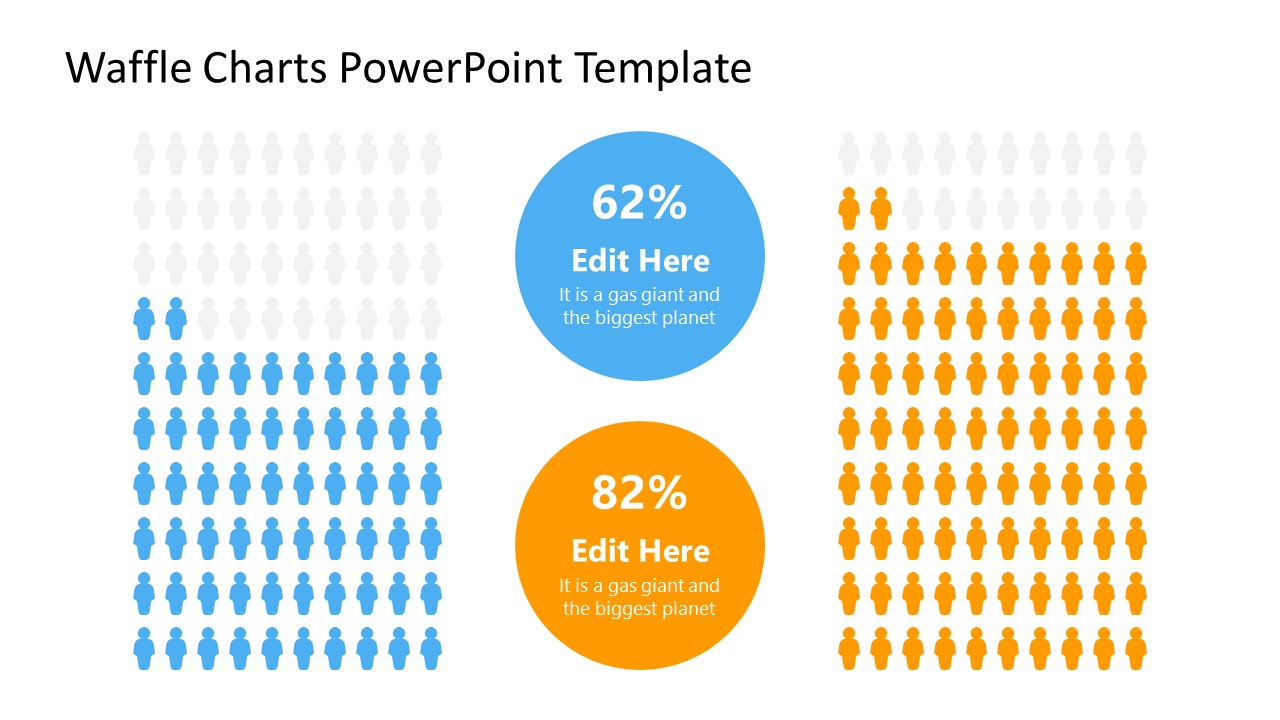
This PPT template helps us how to present data beyond the typical pie chart representation. It is widely used for demographics, so it’s a great fit for marketing teams, data science professionals, HR personnel, and more.
7. Data Presentation Dashboard Template for Google Slides
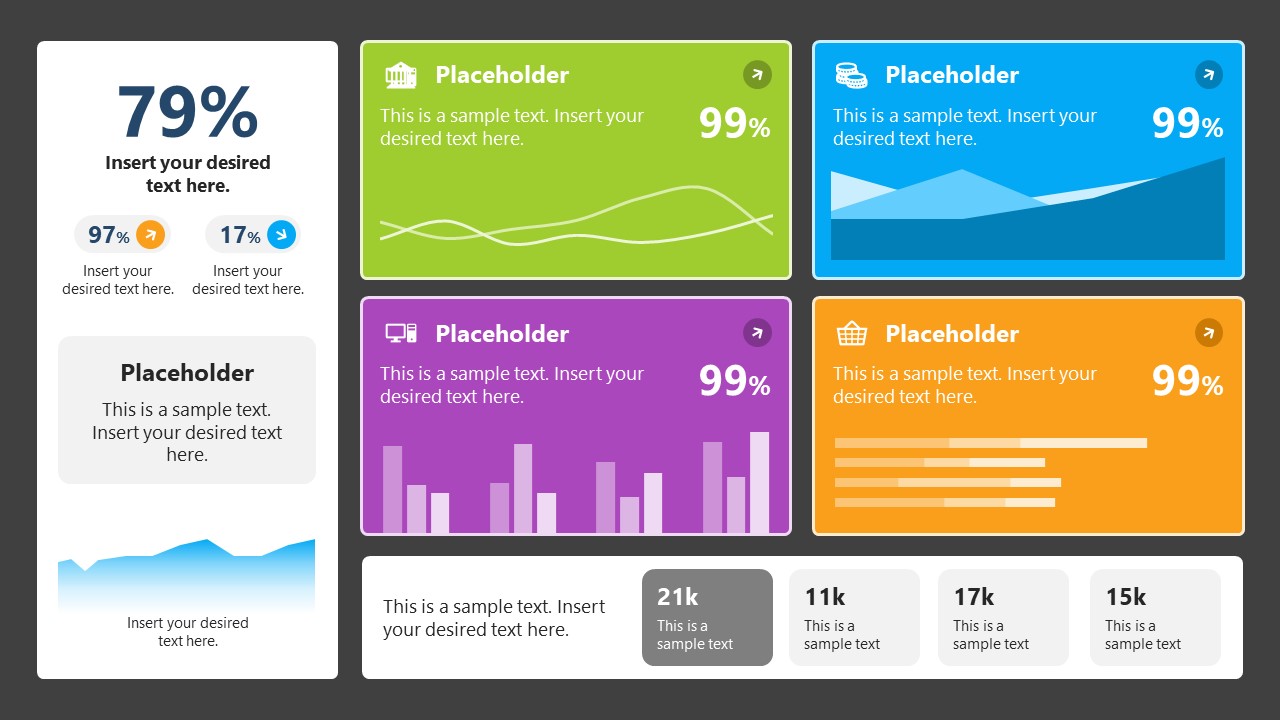
A compendium of tools in dashboard format featuring line graphs, bar charts, column charts, and neatly arranged placeholder text areas.
8. Weather Dashboard for Data Presentation
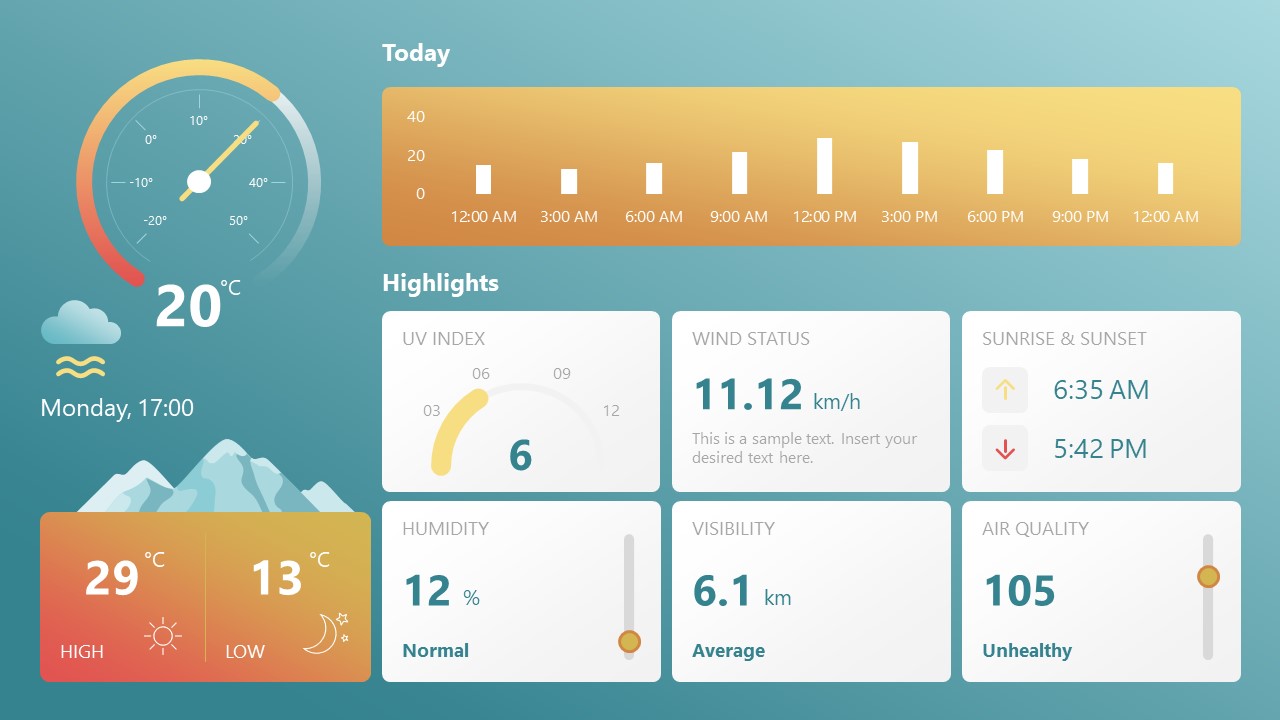
Share weather data for agricultural presentation topics, environmental studies, or any kind of presentation that requires a highly visual layout for weather forecasting on a single day. Two color themes are available.
9. Social Media Marketing Dashboard Data Presentation Template
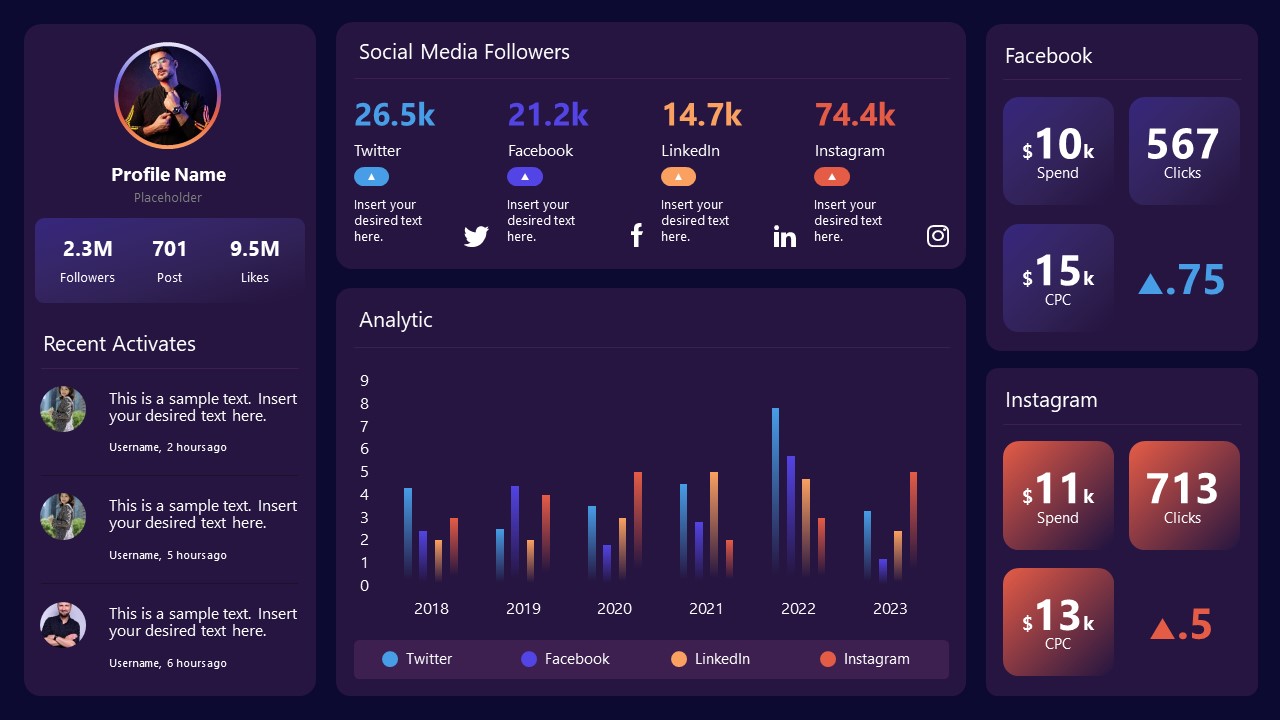
Intended for marketing professionals, this dashboard template for data presentation is a tool for presenting data analytics from social media channels. Two slide layouts featuring line graphs and column charts.
10. Project Management Summary Dashboard Template
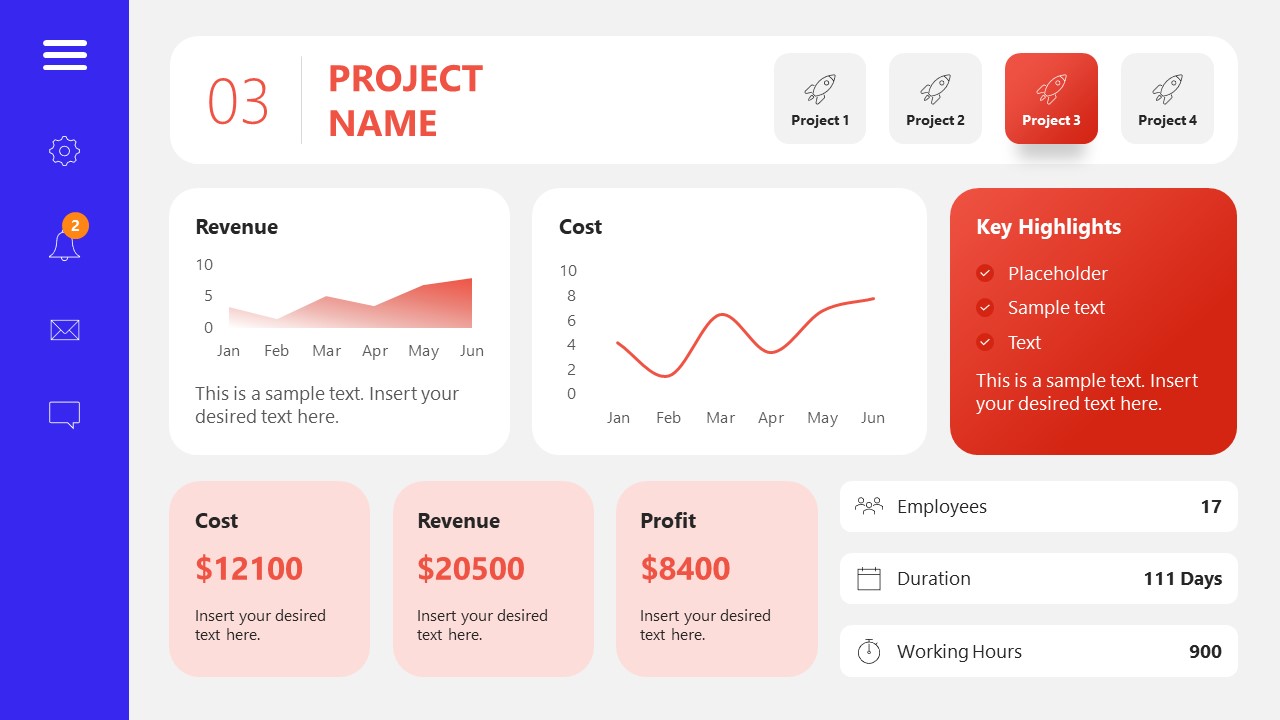
A tool crafted for project managers to deliver highly visual reports on a project’s completion, the profits it delivered for the company, and expenses/time required to execute it. 4 different color layouts are available.
11. Profit & Loss Dashboard for PowerPoint and Google Slides
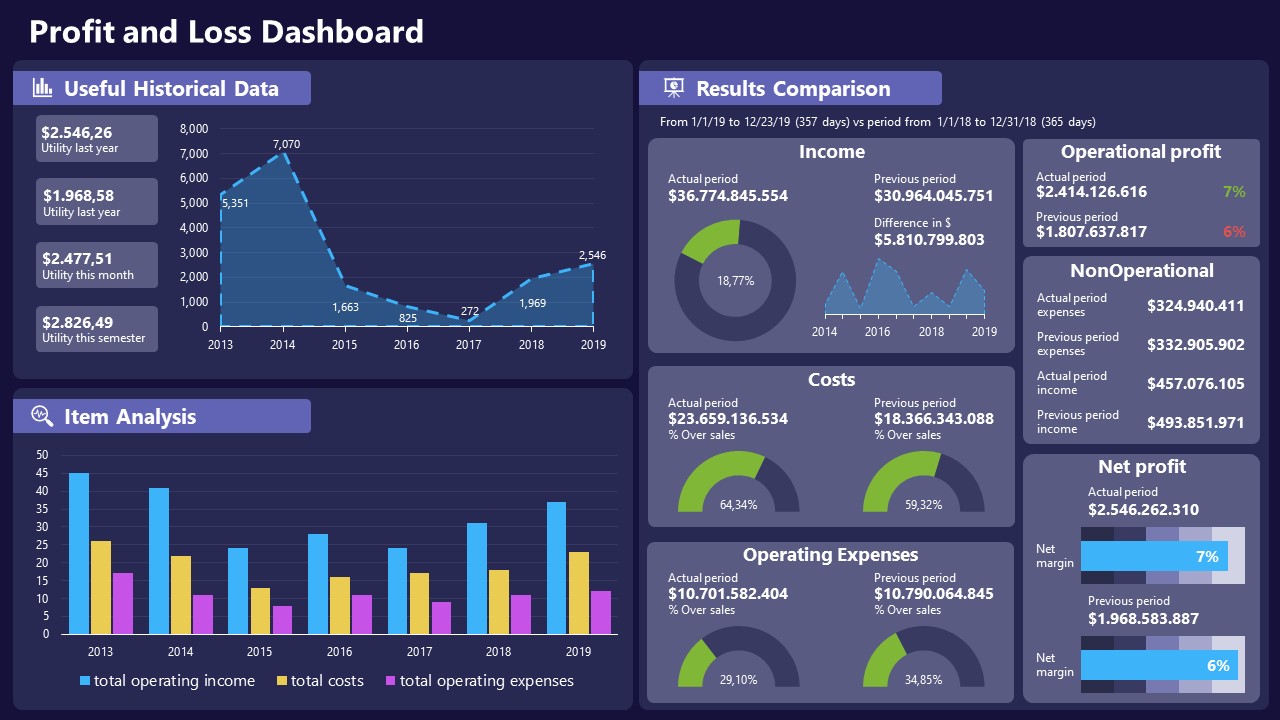
A must-have for finance professionals. This typical profit & loss dashboard includes progress bars, donut charts, column charts, line graphs, and everything that’s required to deliver a comprehensive report about a company’s financial situation.
Overwhelming visuals
One of the mistakes related to using data-presenting methods is including too much data or using overly complex visualizations. They can confuse the audience and dilute the key message.
Inappropriate chart types
Choosing the wrong type of chart for the data at hand can lead to misinterpretation. For example, using a pie chart for data that doesn’t represent parts of a whole is not right.
Lack of context
Failing to provide context or sufficient labeling can make it challenging for the audience to understand the significance of the presented data.
Inconsistency in design
Using inconsistent design elements and color schemes across different visualizations can create confusion and visual disarray.
Failure to provide details
Simply presenting raw data without offering clear insights or takeaways can leave the audience without a meaningful conclusion.
Lack of focus
Not having a clear focus on the key message or main takeaway can result in a presentation that lacks a central theme.
Visual accessibility issues
Overlooking the visual accessibility of charts and graphs can exclude certain audience members who may have difficulty interpreting visual information.
In order to avoid these mistakes in data presentation, presenters can benefit from using presentation templates . These templates provide a structured framework. They ensure consistency, clarity, and an aesthetically pleasing design, enhancing data communication’s overall impact.
Understanding and choosing data presentation types are pivotal in effective communication. Each method serves a unique purpose, so selecting the appropriate one depends on the nature of the data and the message to be conveyed. The diverse array of presentation types offers versatility in visually representing information, from bar charts showing values to pie charts illustrating proportions.
Using the proper method enhances clarity, engages the audience, and ensures that data sets are not just presented but comprehensively understood. By appreciating the strengths and limitations of different presentation types, communicators can tailor their approach to convey information accurately, developing a deeper connection between data and audience understanding.
If you need a quick method to create a data presentation, check out our AI presentation maker . A tool in which you add the topic, curate the outline, select a design, and let AI do the work for you.
[1] Government of Canada, S.C. (2021) 5 Data Visualization 5.2 Bar Chart , 5.2 Bar chart . https://www150.statcan.gc.ca/n1/edu/power-pouvoir/ch9/bargraph-diagrammeabarres/5214818-eng.htm
[2] Kosslyn, S.M., 1989. Understanding charts and graphs. Applied cognitive psychology, 3(3), pp.185-225. https://apps.dtic.mil/sti/pdfs/ADA183409.pdf
[3] Creating a Dashboard . https://it.tufts.edu/book/export/html/1870
[4] https://www.goldenwestcollege.edu/research/data-and-more/data-dashboards/index.html
[5] https://www.mit.edu/course/21/21.guide/grf-line.htm
[6] Jadeja, M. and Shah, K., 2015, January. Tree-Map: A Visualization Tool for Large Data. In GSB@ SIGIR (pp. 9-13). https://ceur-ws.org/Vol-1393/gsb15proceedings.pdf#page=15
[7] Heat Maps and Quilt Plots. https://www.publichealth.columbia.edu/research/population-health-methods/heat-maps-and-quilt-plots
[8] EIU QGIS WORKSHOP. https://www.eiu.edu/qgisworkshop/heatmaps.php
[9] About Pie Charts. https://www.mit.edu/~mbarker/formula1/f1help/11-ch-c8.htm
[10] Histograms. https://sites.utexas.edu/sos/guided/descriptive/numericaldd/descriptiven2/histogram/ [11] https://asq.org/quality-resources/scatter-diagram
Like this article? Please share
Data Analysis, Data Science, Data Visualization Filed under Design
Related Articles
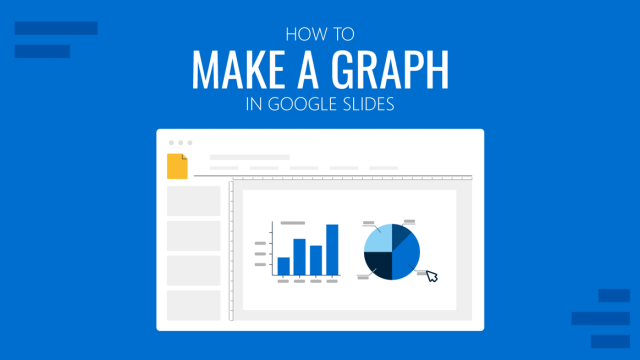
Filed under Google Slides Tutorials • June 3rd, 2024
How To Make a Graph on Google Slides
Creating quality graphics is an essential aspect of designing data presentations. Learn how to make a graph in Google Slides with this guide.
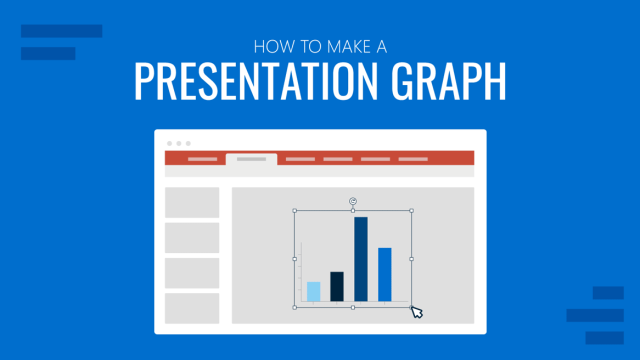
Filed under Design • March 27th, 2024
How to Make a Presentation Graph
Detailed step-by-step instructions to master the art of how to make a presentation graph in PowerPoint and Google Slides. Check it out!
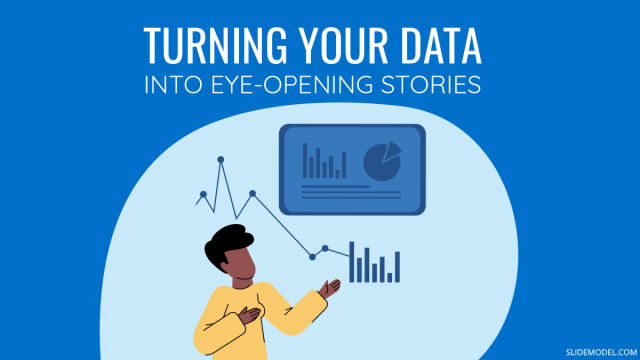
Filed under Presentation Ideas • February 12th, 2024
Turning Your Data into Eye-opening Stories
What is Data Storytelling is a question that people are constantly asking now. If you seek to understand how to create a data storytelling ppt that will complete the information for your audience, you should read this blog post.
Leave a Reply
Call Us Today! +91 99907 48956 | [email protected]

It is the simplest form of data Presentation often used in schools or universities to provide a clearer picture to students, who are better able to capture the concepts effectively through a pictorial Presentation of simple data.
2. Column chart

It is a simplified version of the pictorial Presentation which involves the management of a larger amount of data being shared during the presentations and providing suitable clarity to the insights of the data.
3. Pie Charts

Pie charts provide a very descriptive & a 2D depiction of the data pertaining to comparisons or resemblance of data in two separate fields.
4. Bar charts

A bar chart that shows the accumulation of data with cuboid bars with different dimensions & lengths which are directly proportionate to the values they represent. The bars can be placed either vertically or horizontally depending on the data being represented.
5. Histograms
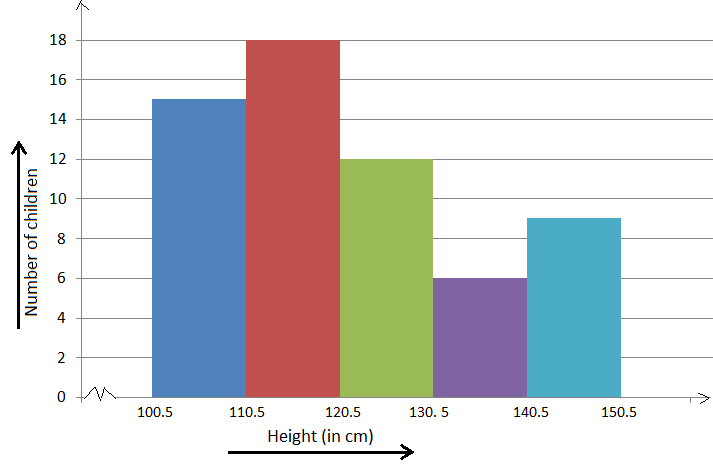
It is a perfect Presentation of the spread of numerical data. The main differentiation that separates data graphs and histograms are the gaps in the data graphs.
6. Box plots

Box plot or Box-plot is a way of representing groups of numerical data through quartiles. Data Presentation is easier with this style of graph dealing with the extraction of data to the minutes of difference.

Map Data graphs help you with data Presentation over an area to display the areas of concern. Map graphs are useful to make an exact depiction of data over a vast case scenario.
All these visual presentations share a common goal of creating meaningful insights and a platform to understand and manage the data in relation to the growth and expansion of one’s in-depth understanding of data & details to plan or execute future decisions or actions.
Importance of Data Presentation
Data Presentation could be both can be a deal maker or deal breaker based on the delivery of the content in the context of visual depiction.
Data Presentation tools are powerful communication tools that can simplify the data by making it easily understandable & readable at the same time while attracting & keeping the interest of its readers and effectively showcase large amounts of complex data in a simplified manner.
If the user can create an insightful presentation of the data in hand with the same sets of facts and figures, then the results promise to be impressive.
There have been situations where the user has had a great amount of data and vision for expansion but the presentation drowned his/her vision.
To impress the higher management and top brass of a firm, effective presentation of data is needed.
Data Presentation helps the clients or the audience to not spend time grasping the concept and the future alternatives of the business and to convince them to invest in the company & turn it profitable both for the investors & the company.
Although data presentation has a lot to offer, the following are some of the major reason behind the essence of an effective presentation:-
- Many consumers or higher authorities are interested in the interpretation of data, not the raw data itself. Therefore, after the analysis of the data, users should represent the data with a visual aspect for better understanding and knowledge.
- The user should not overwhelm the audience with a number of slides of the presentation and inject an ample amount of texts as pictures that will speak for themselves.
- Data presentation often happens in a nutshell with each department showcasing their achievements towards company growth through a graph or a histogram.
- Providing a brief description would help the user to attain attention in a small amount of time while informing the audience about the context of the presentation
- The inclusion of pictures, charts, graphs and tables in the presentation help for better understanding the potential outcomes.
- An effective presentation would allow the organization to determine the difference with the fellow organization and acknowledge its flaws. Comparison of data would assist them in decision making.
Recommended Courses

Data Visualization
Using powerbi &tableau.

Tableau for Data Analysis

MySQL Certification Program

The PowerBI Masterclass
Need help call our support team 7:00 am to 10:00 pm (ist) at (+91 999-074-8956 | 9650-308-956), keep in touch, email: [email protected].
WhatsApp us
Data presentation: A comprehensive guide
Learn how to create data presentation effectively and communicate your insights in a way that is clear, concise, and engaging.
Raja Bothra
Building presentations
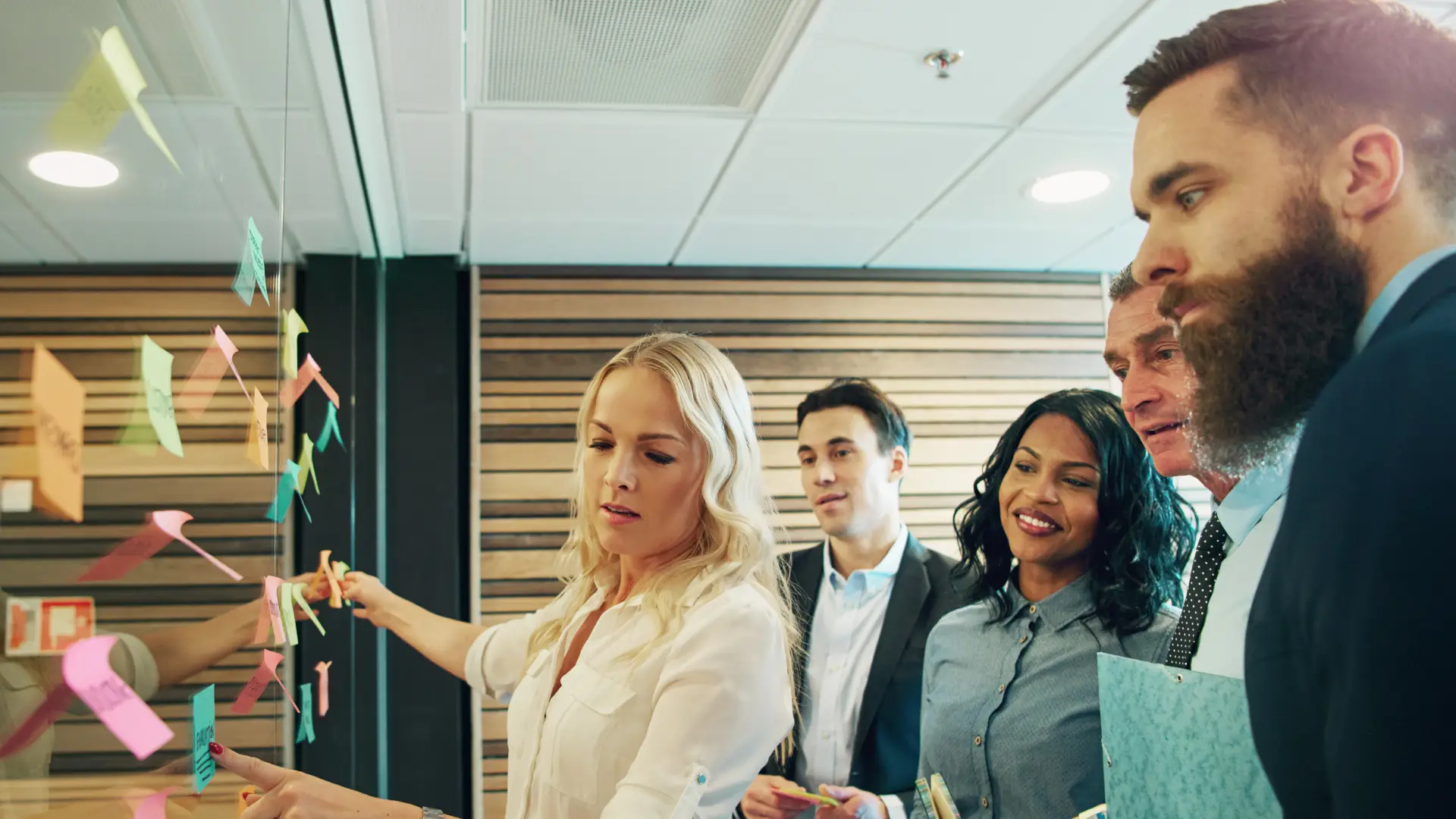
Hey there, fellow data enthusiast!
Welcome to our comprehensive guide on data presentation.
Whether you're an experienced presenter or just starting, this guide will help you present your data like a pro. We'll dive deep into what data presentation is, why it's crucial, and how to master it. So, let's embark on this data-driven journey together.
What is data presentation?
Data presentation is the art of transforming raw data into a visual format that's easy to understand and interpret. It's like turning numbers and statistics into a captivating story that your audience can quickly grasp. When done right, data presentation can be a game-changer, enabling you to convey complex information effectively.
Why are data presentations important?
Imagine drowning in a sea of numbers and figures. That's how your audience might feel without proper data presentation. Here's why it's essential:
- Clarity : Data presentations make complex information clear and concise.
- Engagement : Visuals, such as charts and graphs, grab your audience's attention.
- Comprehension : Visual data is easier to understand than long, numerical reports.
- Decision-making : Well-presented data aids informed decision-making.
- Impact : It leaves a lasting impression on your audience.
Types of data presentation:
Now, let's delve into the diverse array of data presentation methods, each with its own unique strengths and applications. We have three primary types of data presentation, and within these categories, numerous specific visualization techniques can be employed to effectively convey your data.
1. Textual presentation
Textual presentation harnesses the power of words and sentences to elucidate and contextualize your data. This method is commonly used to provide a narrative framework for the data, offering explanations, insights, and the broader implications of your findings. It serves as a foundation for a deeper understanding of the data's significance.
2. Tabular presentation
Tabular presentation employs tables to arrange and structure your data systematically. These tables are invaluable for comparing various data groups or illustrating how data evolves over time. They present information in a neat and organized format, facilitating straightforward comparisons and reference points.
3. Graphical presentation
Graphical presentation harnesses the visual impact of charts and graphs to breathe life into your data. Charts and graphs are powerful tools for spotlighting trends, patterns, and relationships hidden within the data. Let's explore some common graphical presentation methods:
- Bar charts: They are ideal for comparing different categories of data. In this method, each category is represented by a distinct bar, and the height of the bar corresponds to the value it represents. Bar charts provide a clear and intuitive way to discern differences between categories.
- Pie charts: It excel at illustrating the relative proportions of different data categories. Each category is depicted as a slice of the pie, with the size of each slice corresponding to the percentage of the total value it represents. Pie charts are particularly effective for showcasing the distribution of data.
- Line graphs: They are the go-to choice when showcasing how data evolves over time. Each point on the line represents a specific value at a particular time period. This method enables viewers to track trends and fluctuations effortlessly, making it perfect for visualizing data with temporal dimensions.
- Scatter plots: They are the tool of choice when exploring the relationship between two variables. In this method, each point on the plot represents a pair of values for the two variables in question. Scatter plots help identify correlations, outliers, and patterns within data pairs.
The selection of the most suitable data presentation method hinges on the specific dataset and the presentation's objectives. For instance, when comparing sales figures of different products, a bar chart shines in its simplicity and clarity. On the other hand, if your aim is to display how a product's sales have changed over time, a line graph provides the ideal visual narrative.
Additionally, it's crucial to factor in your audience's level of familiarity with data presentations. For a technical audience, more intricate visualization methods may be appropriate. However, when presenting to a general audience, opting for straightforward and easily understandable visuals is often the wisest choice.
In the world of data presentation, choosing the right method is akin to selecting the perfect brush for a masterpiece. Each tool has its place, and understanding when and how to use them is key to crafting compelling and insightful presentations. So, consider your data carefully, align your purpose, and paint a vivid picture that resonates with your audience.
What to include in data presentation?
When creating your data presentation, remember these key components:
- Data points : Clearly state the data points you're presenting.
- Comparison : Highlight comparisons and trends in your data.
- Graphical methods : Choose the right chart or graph for your data.
- Infographics : Use visuals like infographics to make information more digestible.
- Numerical values : Include numerical values to support your visuals.
- Qualitative information : Explain the significance of the data.
- Source citation : Always cite your data sources.
How to structure an effective data presentation?
Creating a well-structured data presentation is not just important; it's the backbone of a successful presentation. Here's a step-by-step guide to help you craft a compelling and organized presentation that captivates your audience:
1. Know your audience
Understanding your audience is paramount. Consider their needs, interests, and existing knowledge about your topic. Tailor your presentation to their level of understanding, ensuring that it resonates with them on a personal level. Relevance is the key.
2. Have a clear message
Every effective data presentation should convey a clear and concise message. Determine what you want your audience to learn or take away from your presentation, and make sure your message is the guiding light throughout your presentation. Ensure that all your data points align with and support this central message.
3. Tell a compelling story
Human beings are naturally wired to remember stories. Incorporate storytelling techniques into your presentation to make your data more relatable and memorable. Your data can be the backbone of a captivating narrative, whether it's about a trend, a problem, or a solution. Take your audience on a journey through your data.
4. Leverage visuals
Visuals are a powerful tool in data presentation. They make complex information accessible and engaging. Utilize charts, graphs, and images to illustrate your points and enhance the visual appeal of your presentation. Visuals should not just be an accessory; they should be an integral part of your storytelling.
5. Be clear and concise
Avoid jargon or technical language that your audience may not comprehend. Use plain language and explain your data points clearly. Remember, clarity is king. Each piece of information should be easy for your audience to digest.
6. Practice your delivery
Practice makes perfect. Rehearse your presentation multiple times before the actual delivery. This will help you deliver it smoothly and confidently, reducing the chances of stumbling over your words or losing track of your message.
A basic structure for an effective data presentation
Armed with a comprehensive comprehension of how to construct a compelling data presentation, you can now utilize this fundamental template for guidance:
In the introduction, initiate your presentation by introducing both yourself and the topic at hand. Clearly articulate your main message or the fundamental concept you intend to communicate.
Moving on to the body of your presentation, organize your data in a coherent and easily understandable sequence. Employ visuals generously to elucidate your points and weave a narrative that enhances the overall story. Ensure that the arrangement of your data aligns with and reinforces your central message.
As you approach the conclusion, succinctly recapitulate your key points and emphasize your core message once more. Conclude by leaving your audience with a distinct and memorable takeaway, ensuring that your presentation has a lasting impact.
Additional tips for enhancing your data presentation
To take your data presentation to the next level, consider these additional tips:
- Consistent design : Maintain a uniform design throughout your presentation. This not only enhances visual appeal but also aids in seamless comprehension.
- High-quality visuals : Ensure that your visuals are of high quality, easy to read, and directly relevant to your topic.
- Concise text : Avoid overwhelming your slides with excessive text. Focus on the most critical points, using visuals to support and elaborate.
- Anticipate questions : Think ahead about the questions your audience might pose. Be prepared with well-thought-out answers to foster productive discussions.
By following these guidelines, you can structure an effective data presentation that not only informs but also engages and inspires your audience. Remember, a well-structured presentation is the bridge that connects your data to your audience's understanding and appreciation.
Do’s and don'ts on a data presentation
- Use visuals : Incorporate charts and graphs to enhance understanding.
- Keep it simple : Avoid clutter and complexity.
- Highlight key points : Emphasize crucial data.
- Engage the audience : Encourage questions and discussions.
- Practice : Rehearse your presentation.
Don'ts:
- Overload with data : Less is often more; don't overwhelm your audience.
- Fit Unrelated data : Stay on topic; don't include irrelevant information.
- Neglect the audience : Ensure your presentation suits your audience's level of expertise.
- Read word-for-word : Avoid reading directly from slides.
- Lose focus : Stick to your presentation's purpose.
Summarizing key takeaways
- Definition : Data presentation is the art of visualizing complex data for better understanding.
- Importance : Data presentations enhance clarity, engage the audience, aid decision-making, and leave a lasting impact.
- Types : Textual, Tabular, and Graphical presentations offer various ways to present data.
- Choosing methods : Select the right method based on data, audience, and purpose.
- Components : Include data points, comparisons, visuals, infographics, numerical values, and source citations.
- Structure : Know your audience, have a clear message, tell a compelling story, use visuals, be concise, and practice.
- Do's and don'ts : Do use visuals, keep it simple, highlight key points, engage the audience, and practice. Don't overload with data, include unrelated information, neglect the audience's expertise, read word-for-word, or lose focus.
FAQ's on a data presentation
1. what is data presentation, and why is it important in 2024.
Data presentation is the process of visually representing data sets to convey information effectively to an audience. In an era where the amount of data generated is vast, visually presenting data using methods such as diagrams, graphs, and charts has become crucial. By simplifying complex data sets, presentation of the data may helps your audience quickly grasp much information without drowning in a sea of chart's, analytics, facts and figures.
2. What are some common methods of data presentation?
There are various methods of data presentation, including graphs and charts, histograms, and cumulative frequency polygons. Each method has its strengths and is often used depending on the type of data you're using and the message you want to convey. For instance, if you want to show data over time, try using a line graph. If you're presenting geographical data, consider to use a heat map.
3. How can I ensure that my data presentation is clear and readable?
To ensure that your data presentation is clear and readable, pay attention to the design and labeling of your charts. Don't forget to label the axes appropriately, as they are critical for understanding the values they represent. Don't fit all the information in one slide or in a single paragraph. Presentation software like Prezent and PowerPoint can help you simplify your vertical axis, charts and tables, making them much easier to understand.
4. What are some common mistakes presenters make when presenting data?
One common mistake is trying to fit too much data into a single chart, which can distort the information and confuse the audience. Another mistake is not considering the needs of the audience. Remember that your audience won't have the same level of familiarity with the data as you do, so it's essential to present the data effectively and respond to questions during a Q&A session.
5. How can I use data visualization to present important data effectively on platforms like LinkedIn?
When presenting data on platforms like LinkedIn, consider using eye-catching visuals like bar graphs or charts. Use concise captions and e.g., examples to highlight the single most important information in your data report. Visuals, such as graphs and tables, can help you stand out in the sea of textual content, making your data presentation more engaging and shareable among your LinkedIn connections.
Create your data presentation with prezent
Prezent can be a valuable tool for creating data presentations. Here's how Prezent can help you in this regard:
- Time savings : Prezent saves up to 70% of presentation creation time, allowing you to focus on data analysis and insights.
- On-brand consistency : Ensure 100% brand alignment with Prezent's brand-approved designs for professional-looking data presentations.
- Effortless collaboration : Real-time sharing and collaboration features make it easy for teams to work together on data presentations.
- Data storytelling : Choose from 50+ storylines to effectively communicate data insights and engage your audience.
- Personalization : Create tailored data presentations that resonate with your audience's preferences, enhancing the impact of your data.
In summary, Prezent streamlines the process of creating data presentations by offering time-saving features, ensuring brand consistency, promoting collaboration, and providing tools for effective data storytelling. Whether you need to present data to clients, stakeholders, or within your organization, Prezent can significantly enhance your presentation-making process.
So, go ahead, present your data with confidence, and watch your audience be wowed by your expertise.
Thank you for joining us on this data-driven journey. Stay tuned for more insights, and remember, data presentation is your ticket to making numbers come alive! Sign up for our free trial or book a demo !
More zenpedia articles

The role of effective team communication for enhanced organizational effectiveness

Employers guide to enhance problem-solving skills for business success

How to create a compelling brand positioning presentation
Get the latest from Prezent community
Join thousands of subscribers who receive our best practices on communication, storytelling, presentation design, and more. New tips weekly. (No spam, we promise!)
A Guide to Effective Data Presentation
Key objectives of data presentation, charts and graphs for great visuals, storytelling with data, visuals, and text, audiences and data presentation, the main idea in data presentation, storyboarding and data presentation, additional resources, data presentation.
Tools for effective data presentation
Financial analysts are required to present their findings in a neat, clear, and straightforward manner. They spend most of their time working with spreadsheets in MS Excel, building financial models , and crunching numbers. These models and calculations can be pretty extensive and complex and may only be understood by the analyst who created them. Effective data presentation skills are critical for being a world-class financial analyst .
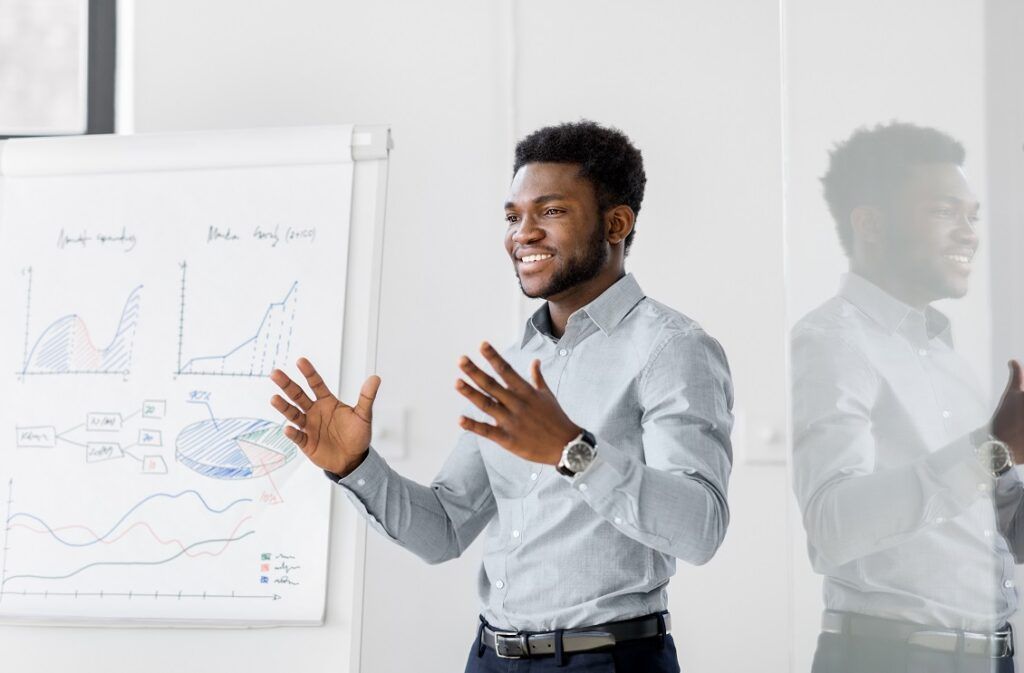
It is the analyst’s job to effectively communicate the output to the target audience, such as the management team or a company’s external investors. This requires focusing on the main points, facts, insights, and recommendations that will prompt the necessary action from the audience.
One challenge is making intricate and elaborate work easy to comprehend through great visuals and dashboards. For example, tables, graphs, and charts are tools that an analyst can use to their advantage to give deeper meaning to a company’s financial information. These tools organize relevant numbers that are rather dull and give life and story to them.
Here are some key objectives to think about when presenting financial analysis:
- Visual communication
- Audience and context
- Charts, graphs, and images
- Focus on important points
- Design principles
- Storytelling
- Persuasiveness
For a breakdown of these objectives, check out Excel Dashboards & Data Visualization course to help you become a world-class financial analyst.
Charts and graphs make any financial analysis readable, easy to follow, and provide great data presentation. They are often included in the financial model’s output, which is essential for the key decision-makers in a company.
The decision-makers comprise executives and managers who usually won’t have enough time to synthesize and interpret data on their own to make sound business decisions. Therefore, it is the job of the analyst to enhance the decision-making process and help guide the executives and managers to create value for the company.
When an analyst uses charts, it is necessary to be aware of what good charts and bad charts look like and how to avoid the latter when telling a story with data.
Examples of Good Charts
As for great visuals, you can quickly see what’s going on with the data presentation, saving you time for deciphering their actual meaning. More importantly, great visuals facilitate business decision-making because their goal is to provide persuasive, clear, and unambiguous numeric communication.
For reference, take a look at the example below that shows a dashboard, which includes a gauge chart for growth rates, a bar chart for the number of orders, an area chart for company revenues, and a line chart for EBITDA margins.
To learn the step-by-step process of creating these essential tools in MS Excel, watch our video course titled “ Excel Dashboard & Data Visualization .” Aside from what is given in the example below, our course will also teach how you can use other tables and charts to make your financial analysis stand out professionally.
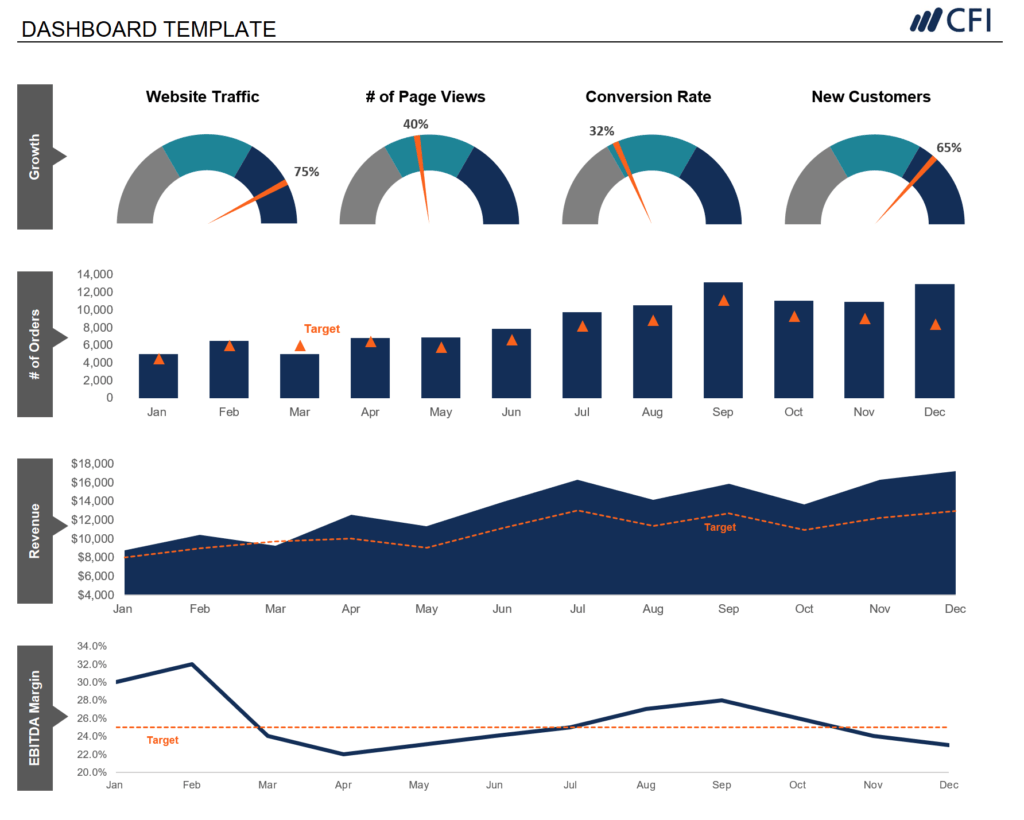
Learn how to build the graph above in our Dashboards Course !
Example of Poorly Crafted Charts
A bad chart, as seen below, will give the reader a difficult time to find the main takeaway of a report or presentation, because it contains too many colors, labels, and legends, and thus, will often look too busy. It also doesn’t help much if a chart, such as a pie chart, is displayed in 3D, as it skews the size and perceived value of the underlying data. A bad chart will be hard to follow and understand.
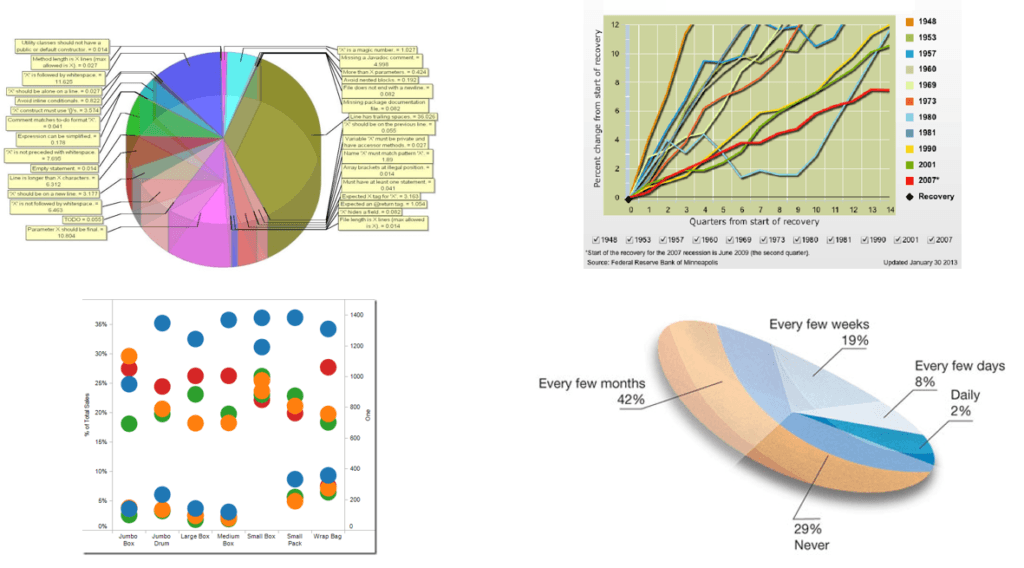
Aside from understanding the meaning of the numbers, a financial analyst must learn to combine numbers and language to craft an effective story. Relying only on data for a presentation may leave your audience finding it difficult to read, interpret, and analyze your data. You must do the work for them, and a good story will be easier to follow. It will help you arrive at the main points faster, rather than just solely presenting your report or live presentation with numbers.
The data can be in the form of revenues, expenses, profits, and cash flow. Simply adding notes, comments, and opinions to each line item will add an extra layer of insight, angle, and a new perspective to the report.
Furthermore, by combining data, visuals, and text, your audience will get a clear understanding of the current situation, past events, and possible conclusions and recommendations that can be made for the future.
The simple diagram below shows the different categories of your audience.
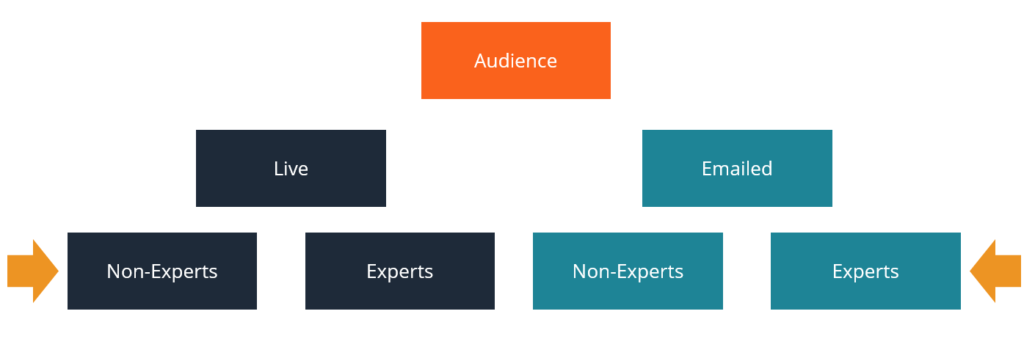
This chart is taken from our course on how to present data .
Internal Audience
An internal audience can either be the executives of the company or any employee who works in that company. For executives, the purpose of communicating a data-filled presentation is to give an update about a certain business activity such as a project or an initiative.
Another important purpose is to facilitate decision-making on managing the company’s operations, growing its core business, acquiring new markets and customers, investing in R&D, and other considerations. Knowing the relevant data and information beforehand will guide the decision-makers in making the right choices that will best position the company toward more success.
External Audience
An external audience can either be the company’s existing clients, where there are projects in progress, or new clients that the company wants to build a relationship with and win new business from. The other external audience is the general public, such as the company’s external shareholders and prospective investors of the company.
When it comes to winning new business, the analyst’s presentation will be more promotional and sales-oriented, whereas a project update will contain more specific information for the client, usually with lots of industry jargon.
Audiences for Live and Emailed Presentation
A live presentation contains more visuals and storytelling to connect more with the audience. It must be more precise and should get to the point faster and avoid long-winded speech or text because of limited time.
In contrast, an emailed presentation is expected to be read, so it will include more text. Just like a document or a book, it will include more detailed information, because its context will not be explained with a voice-over as in a live presentation.
When it comes to details, acronyms, and jargon in the presentation, these things depend on whether your audience are experts or not.
Every great presentation requires a clear “main idea”. It is the core purpose of the presentation and should be addressed clearly. Its significance should be highlighted and should cause the targeted audience to take some action on the matter.
An example of a serious and profound idea is given below.
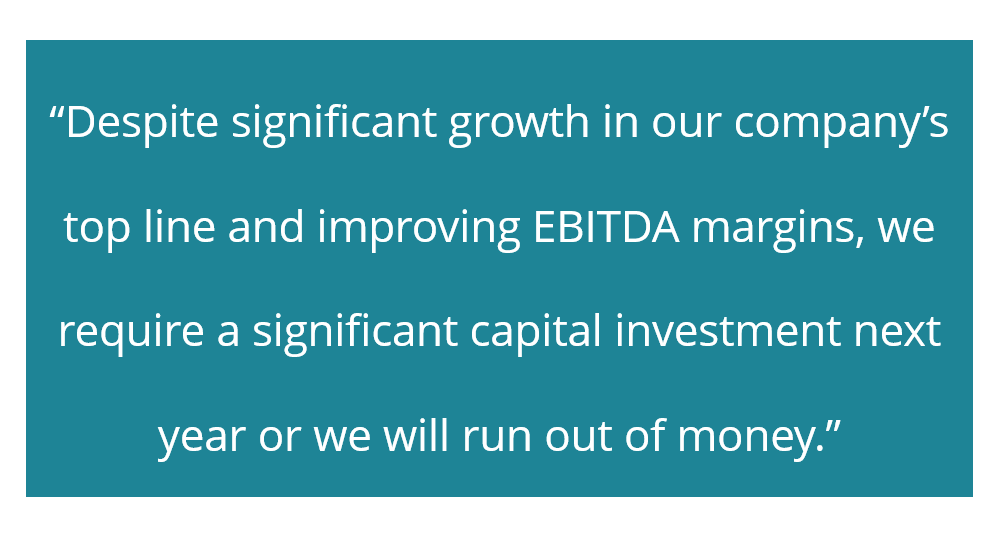
To communicate this big idea, we have to come up with appropriate and effective visual displays to show both the good and bad things surrounding the idea. It should put emphasis and attention on the most important part, which is the critical cash balance and capital investment situation for next year. This is an important component of data presentation.
The storyboarding below is how an analyst would build the presentation based on the big idea. Once the issue or the main idea has been introduced, it will be followed by a demonstration of the positive aspects of the company’s performance, as well as the negative aspects, which are more important and will likely require more attention.
Various ideas will then be suggested to solve the negative issues. However, before choosing the best option, a comparison of the different outcomes of the suggested ideas will be performed. Finally, a recommendation will be made that centers around the optimal choice to address the imminent problem highlighted in the big idea.
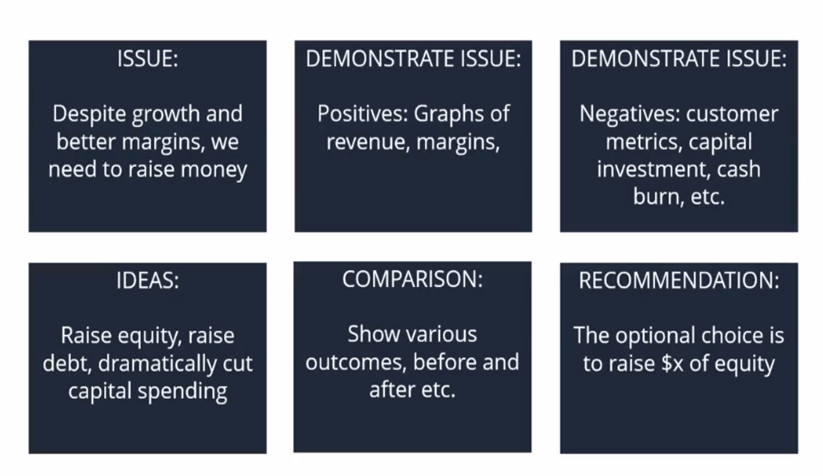
This storyboard is taken from our course on how to present data .
To get to the final point (recommendation), a great deal of analysis has been performed, which includes the charts and graphs discussed earlier, to make the whole presentation easy to follow, convincing, and compelling for your audience.
CFI offers the Business Intelligence & Data Analyst (BIDA)® certification program for those looking to take their careers to the next level. To keep learning and developing your knowledge base, please explore the additional relevant resources below:
- Investment Banking Pitch Books
- Excel Dashboards
- Financial Modeling Guide
- Startup Pitch Book
- See all business intelligence resources
- Share this article
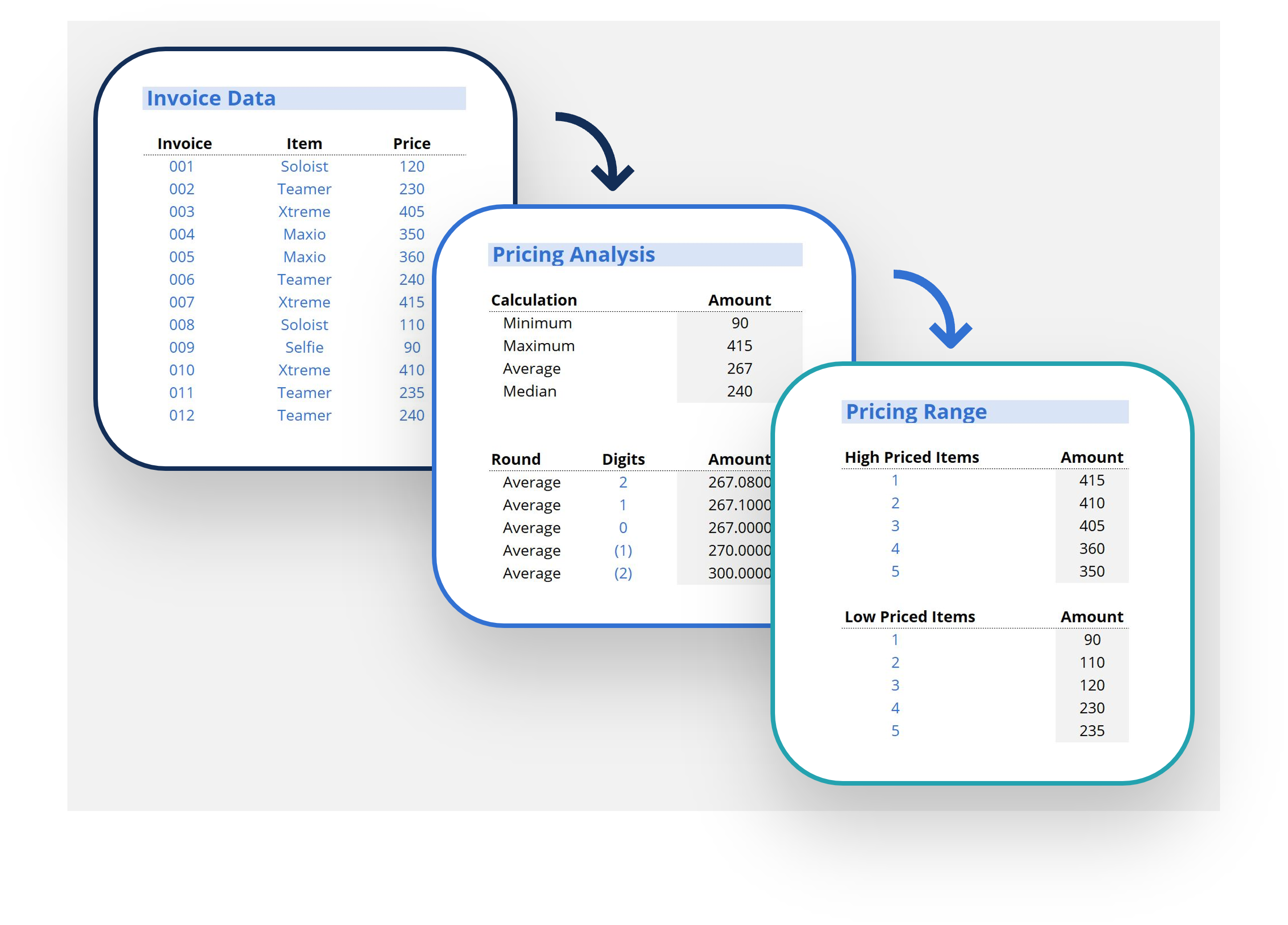
Create a free account to unlock this Template
Access and download collection of free Templates to help power your productivity and performance.
Already have an account? Log in
Supercharge your skills with Premium Templates
Take your learning and productivity to the next level with our Premium Templates.
Upgrading to a paid membership gives you access to our extensive collection of plug-and-play Templates designed to power your performance—as well as CFI's full course catalog and accredited Certification Programs.
Already have a Self-Study or Full-Immersion membership? Log in
Access Exclusive Templates
Gain unlimited access to more than 250 productivity Templates, CFI's full course catalog and accredited Certification Programs, hundreds of resources, expert reviews and support, the chance to work with real-world finance and research tools, and more.
Already have a Full-Immersion membership? Log in
Present Your Data Like a Pro
by Joel Schwartzberg

Summary .
While a good presentation has data, data alone doesn’t guarantee a good presentation. It’s all about how that data is presented. The quickest way to confuse your audience is by sharing too many details at once. The only data points you should share are those that significantly support your point — and ideally, one point per chart. To avoid the debacle of sheepishly translating hard-to-see numbers and labels, rehearse your presentation with colleagues sitting as far away as the actual audience would. While you’ve been working with the same chart for weeks or months, your audience will be exposed to it for mere seconds. Give them the best chance of comprehending your data by using simple, clear, and complete language to identify X and Y axes, pie pieces, bars, and other diagrammatic elements. Try to avoid abbreviations that aren’t obvious, and don’t assume labeled components on one slide will be remembered on subsequent slides. Every valuable chart or pie graph has an “Aha!” zone — a number or range of data that reveals something crucial to your point. Make sure you visually highlight the “Aha!” zone, reinforcing the moment by explaining it to your audience.
With so many ways to spin and distort information these days, a presentation needs to do more than simply share great ideas — it needs to support those ideas with credible data. That’s true whether you’re an executive pitching new business clients, a vendor selling her services, or a CEO making a case for change.
Partner Center
We use essential cookies to make Venngage work. By clicking “Accept All Cookies”, you agree to the storing of cookies on your device to enhance site navigation, analyze site usage, and assist in our marketing efforts.
Manage Cookies
Cookies and similar technologies collect certain information about how you’re using our website. Some of them are essential, and without them you wouldn’t be able to use Venngage. But others are optional, and you get to choose whether we use them or not.
Strictly Necessary Cookies
These cookies are always on, as they’re essential for making Venngage work, and making it safe. Without these cookies, services you’ve asked for can’t be provided.
Show cookie providers
- Google Login
Functionality Cookies
These cookies help us provide enhanced functionality and personalisation, and remember your settings. They may be set by us or by third party providers.
Performance Cookies
These cookies help us analyze how many people are using Venngage, where they come from and how they're using it. If you opt out of these cookies, we can’t get feedback to make Venngage better for you and all our users.
- Google Analytics
Targeting Cookies
These cookies are set by our advertising partners to track your activity and show you relevant Venngage ads on other sites as you browse the internet.
- Google Tag Manager
- Infographics
- Daily Infographics
- Popular Templates
- Accessibility
- Graphic Design
- Graphs and Charts
- Data Visualization
- Human Resources
- Beginner Guides
Blog Data Visualization 10 Data Presentation Examples For Strategic Communication
10 Data Presentation Examples For Strategic Communication
Written by: Krystle Wong Sep 28, 2023
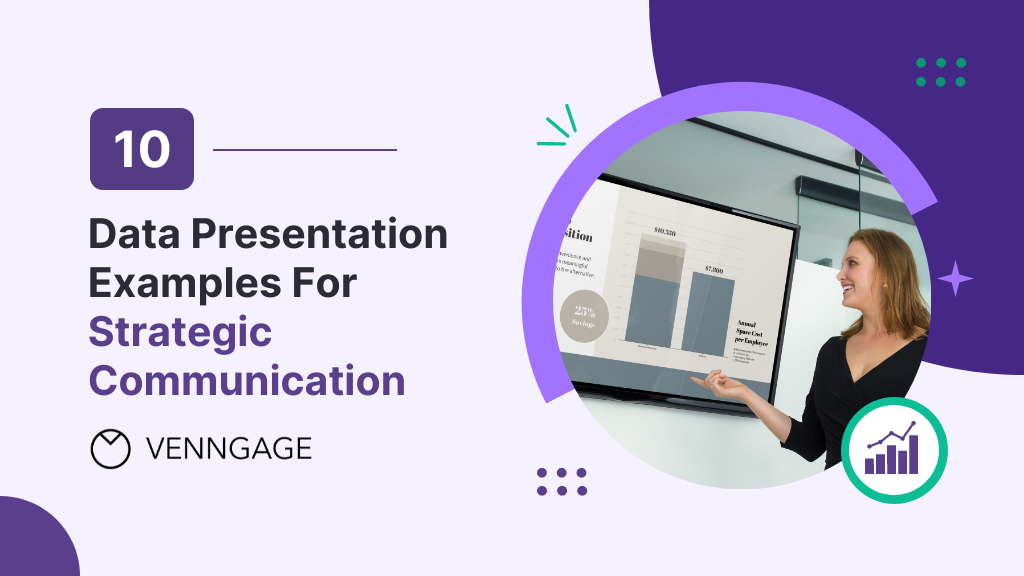
Knowing how to present data is like having a superpower.
Data presentation today is no longer just about numbers on a screen; it’s storytelling with a purpose. It’s about captivating your audience, making complex stuff look simple and inspiring action.
To help turn your data into stories that stick, influence decisions and make an impact, check out Venngage’s free chart maker or follow me on a tour into the world of data storytelling along with data presentation templates that work across different fields, from business boardrooms to the classroom and beyond. Keep scrolling to learn more!
Click to jump ahead:
10 Essential data presentation examples + methods you should know
What should be included in a data presentation, what are some common mistakes to avoid when presenting data, faqs on data presentation examples, transform your message with impactful data storytelling.
Data presentation is a vital skill in today’s information-driven world. Whether you’re in business, academia, or simply want to convey information effectively, knowing the different ways of presenting data is crucial. For impactful data storytelling, consider these essential data presentation methods:
1. Bar graph
Ideal for comparing data across categories or showing trends over time.
Bar graphs, also known as bar charts are workhorses of data presentation. They’re like the Swiss Army knives of visualization methods because they can be used to compare data in different categories or display data changes over time.
In a bar chart, categories are displayed on the x-axis and the corresponding values are represented by the height of the bars on the y-axis.
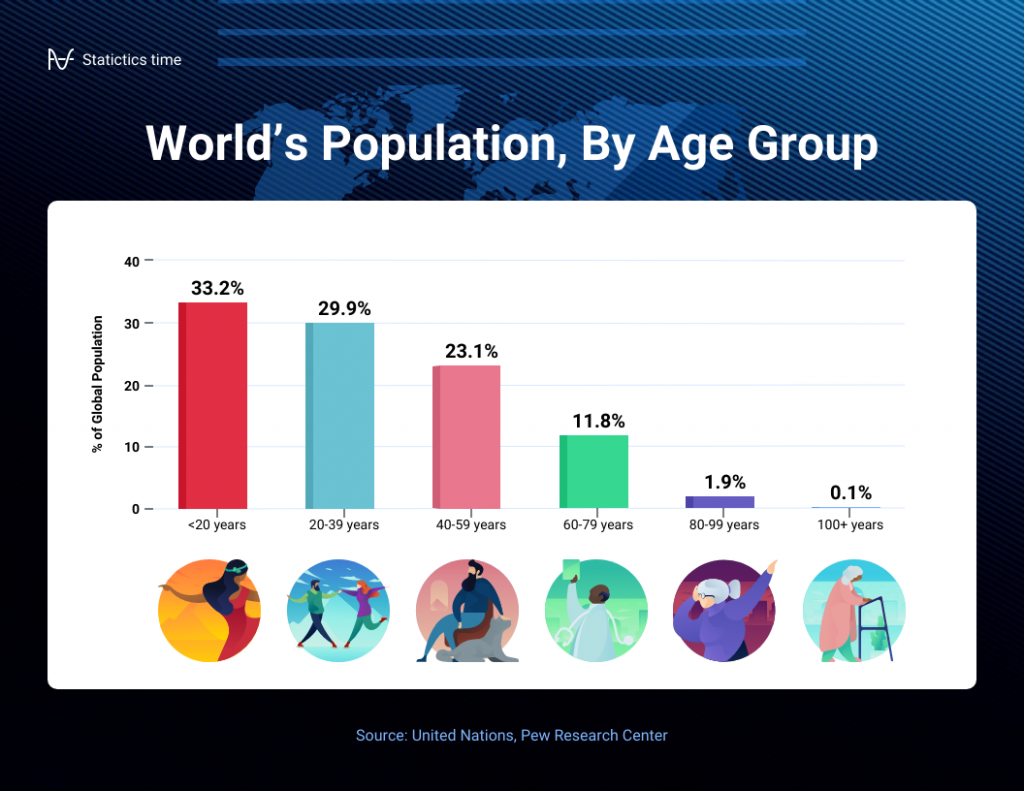
It’s a straightforward and effective way to showcase raw data, making it a staple in business reports, academic presentations and beyond.
Make sure your bar charts are concise with easy-to-read labels. Whether your bars go up or sideways, keep it simple by not overloading with too many categories.
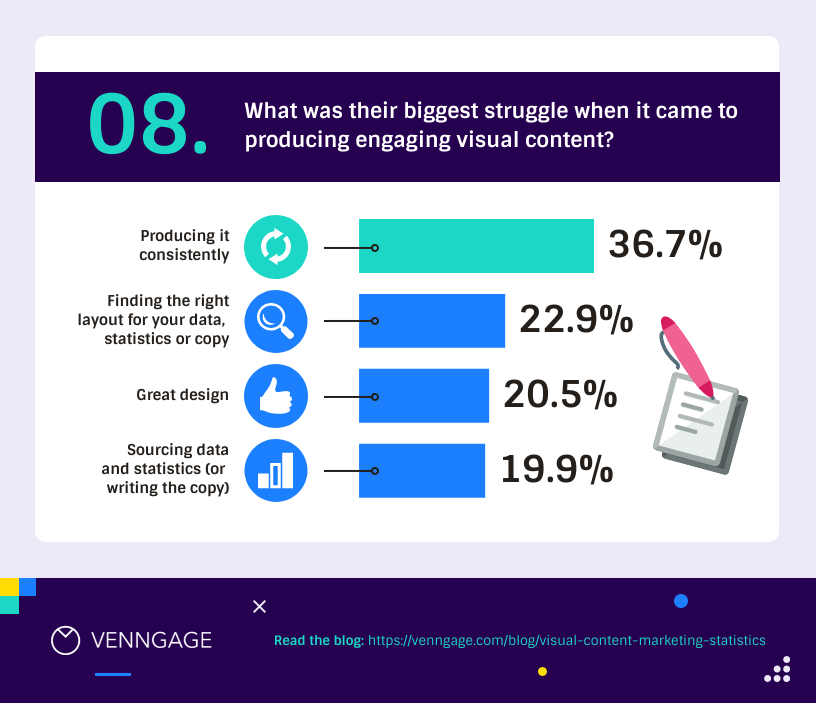
2. Line graph
Great for displaying trends and variations in data points over time or continuous variables.
Line charts or line graphs are your go-to when you want to visualize trends and variations in data sets over time.
One of the best quantitative data presentation examples, they work exceptionally well for showing continuous data, such as sales projections over the last couple of years or supply and demand fluctuations.
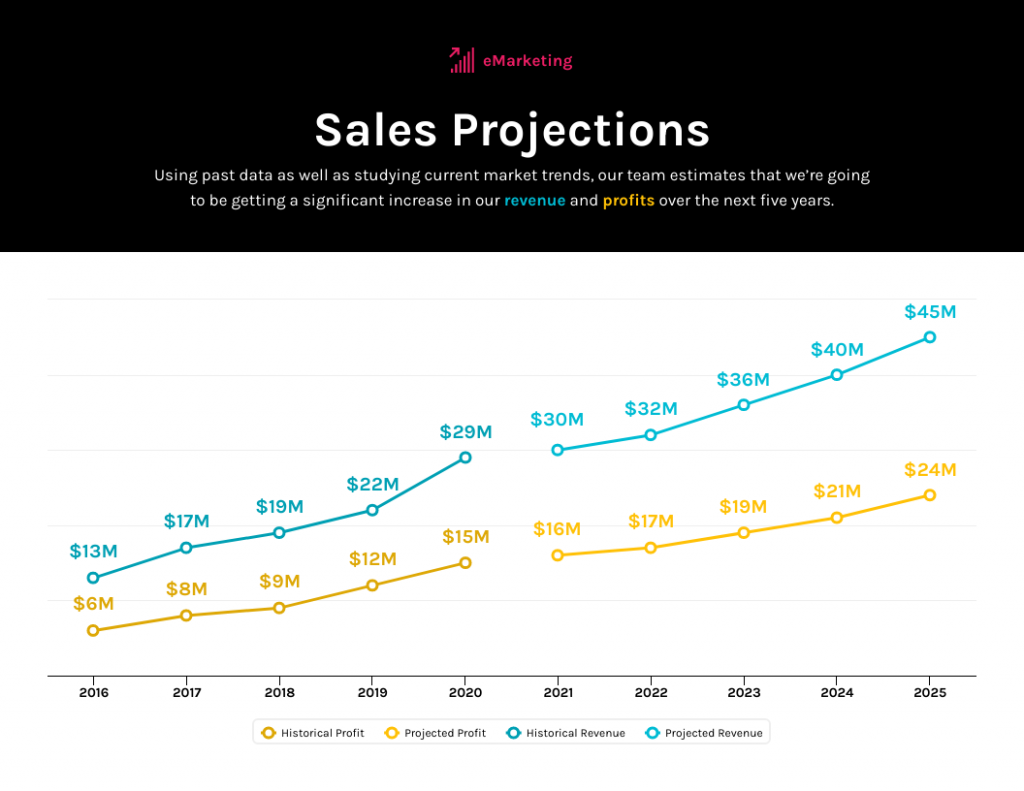
The x-axis represents time or a continuous variable and the y-axis represents the data values. By connecting the data points with lines, you can easily spot trends and fluctuations.
A tip when presenting data with line charts is to minimize the lines and not make it too crowded. Highlight the big changes, put on some labels and give it a catchy title.
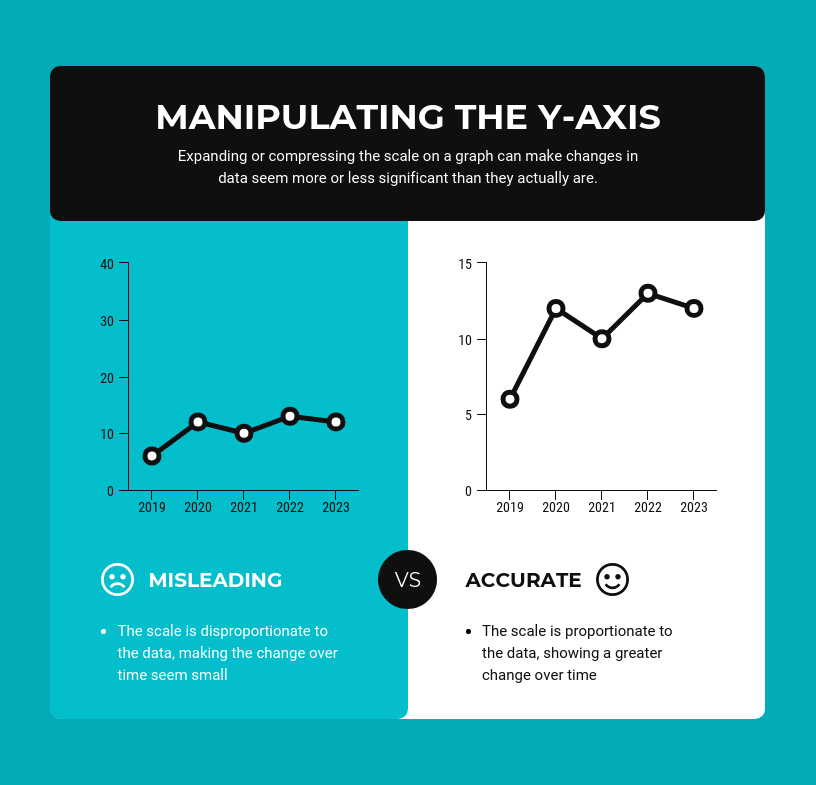
3. Pie chart
Useful for illustrating parts of a whole, such as percentages or proportions.
Pie charts are perfect for showing how a whole is divided into parts. They’re commonly used to represent percentages or proportions and are great for presenting survey results that involve demographic data.
Each “slice” of the pie represents a portion of the whole and the size of each slice corresponds to its share of the total.
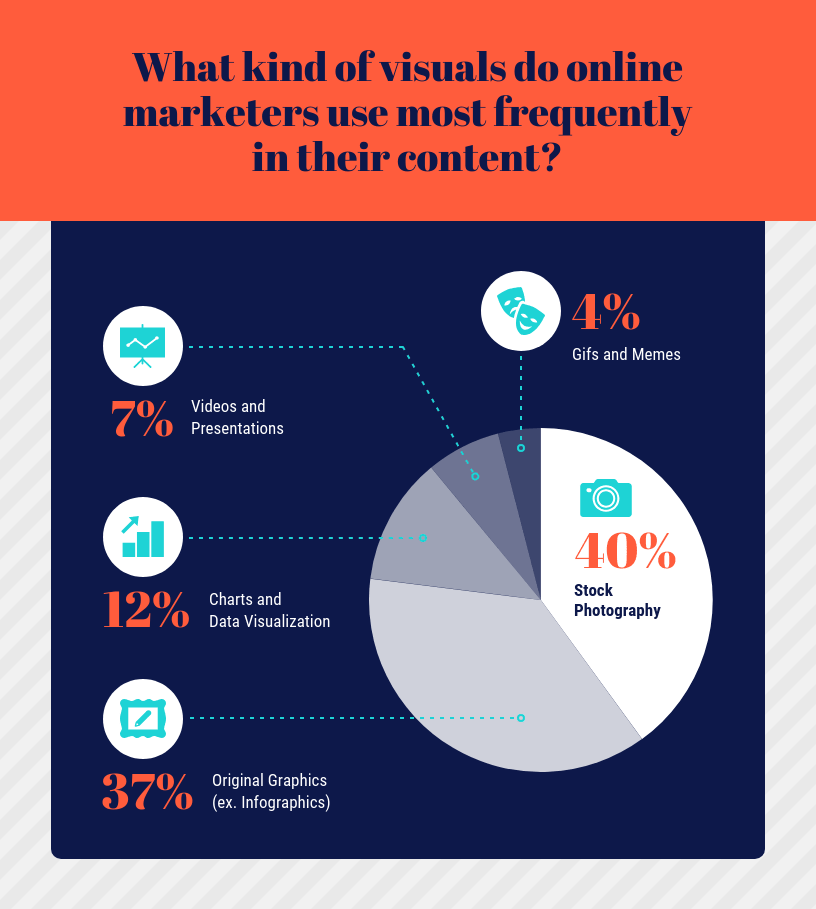
While pie charts are handy for illustrating simple distributions, they can become confusing when dealing with too many categories or when the differences in proportions are subtle.
Don’t get too carried away with slices — label those slices with percentages or values so people know what’s what and consider using a legend for more categories.
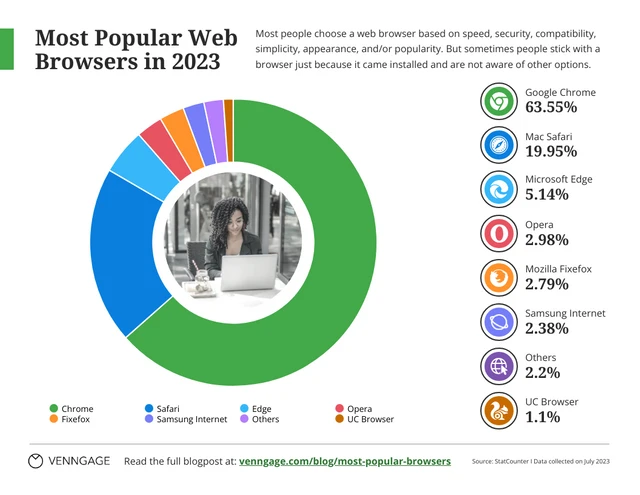
4. Scatter plot
Effective for showing the relationship between two variables and identifying correlations.
Scatter plots are all about exploring relationships between two variables. They’re great for uncovering correlations, trends or patterns in data.
In a scatter plot, every data point appears as a dot on the chart, with one variable marked on the horizontal x-axis and the other on the vertical y-axis.
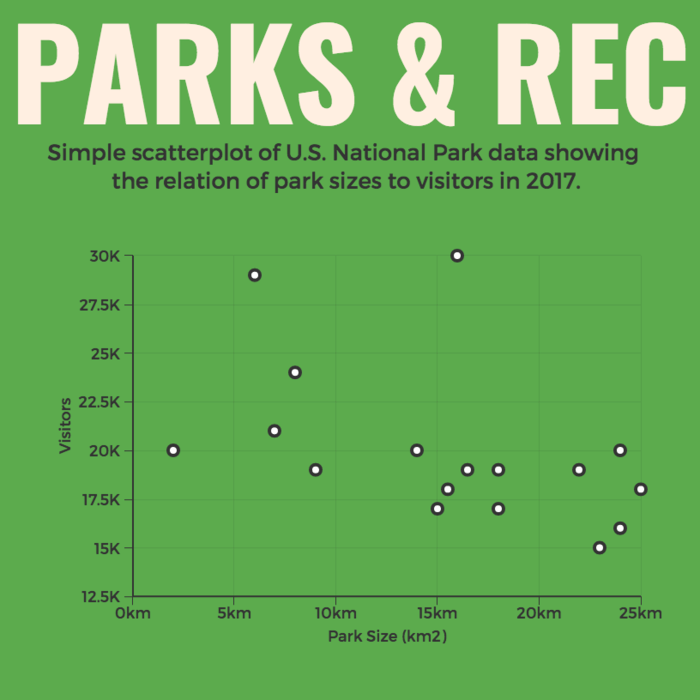
By examining the scatter of points, you can discern the nature of the relationship between the variables, whether it’s positive, negative or no correlation at all.
If you’re using scatter plots to reveal relationships between two variables, be sure to add trendlines or regression analysis when appropriate to clarify patterns. Label data points selectively or provide tooltips for detailed information.
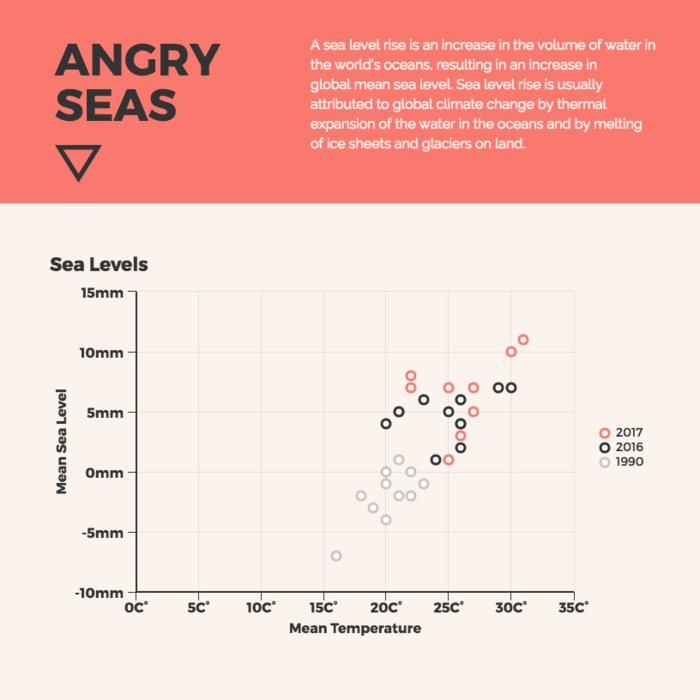
5. Histogram
Best for visualizing the distribution and frequency of a single variable.
Histograms are your choice when you want to understand the distribution and frequency of a single variable.
They divide the data into “bins” or intervals and the height of each bar represents the frequency or count of data points falling into that interval.
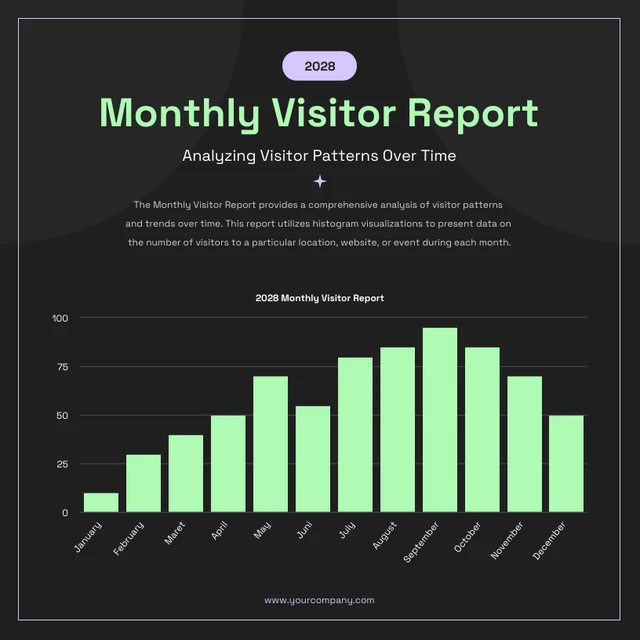
Histograms are excellent for helping to identify trends in data distributions, such as peaks, gaps or skewness.
Here’s something to take note of — ensure that your histogram bins are appropriately sized to capture meaningful data patterns. Using clear axis labels and titles can also help explain the distribution of the data effectively.
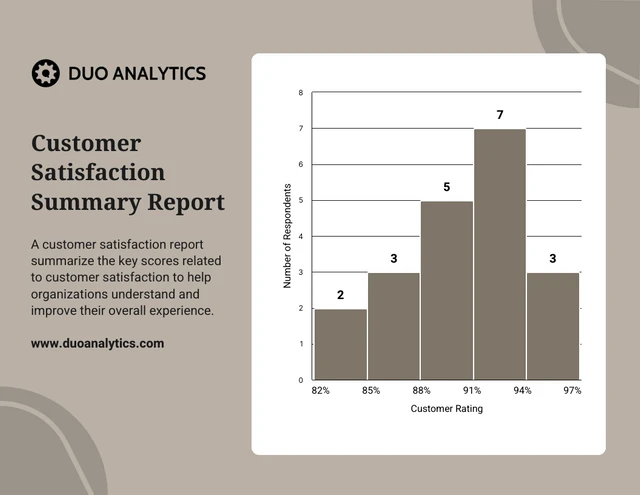
6. Stacked bar chart
Useful for showing how different components contribute to a whole over multiple categories.
Stacked bar charts are a handy choice when you want to illustrate how different components contribute to a whole across multiple categories.
Each bar represents a category and the bars are divided into segments to show the contribution of various components within each category.
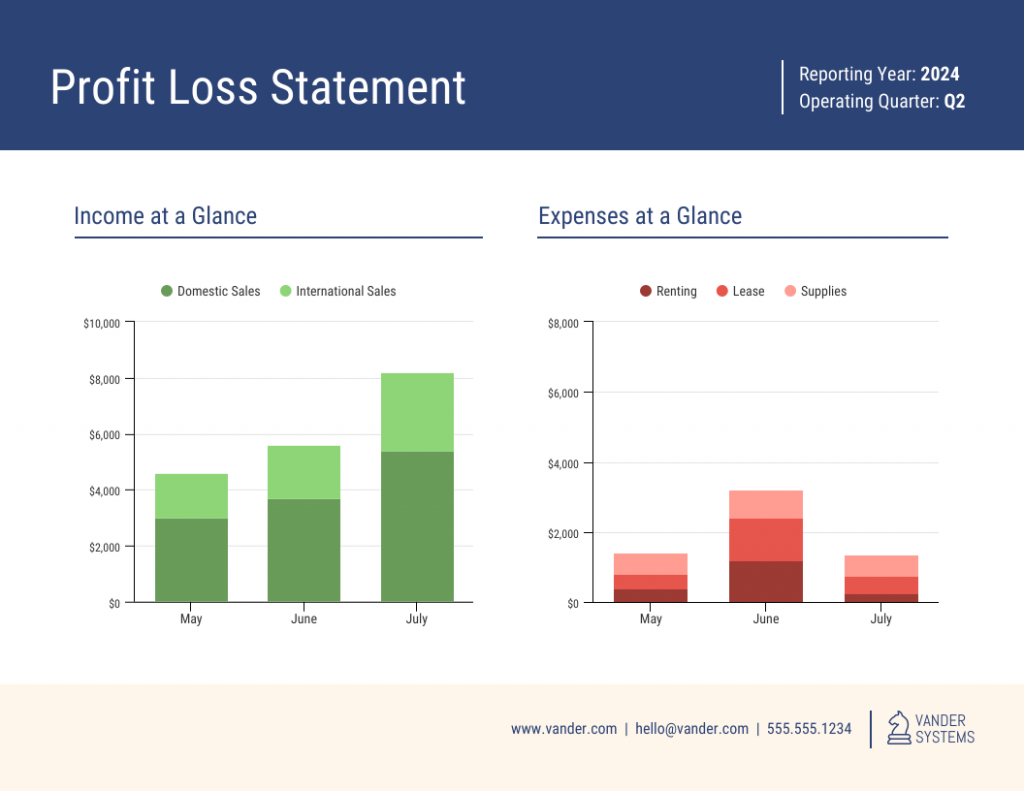
This method is ideal for highlighting both the individual and collective significance of each component, making it a valuable tool for comparative analysis.
Stacked bar charts are like data sandwiches—label each layer so people know what’s what. Keep the order logical and don’t forget the paintbrush for snazzy colors. Here’s a data analysis presentation example on writers’ productivity using stacked bar charts:
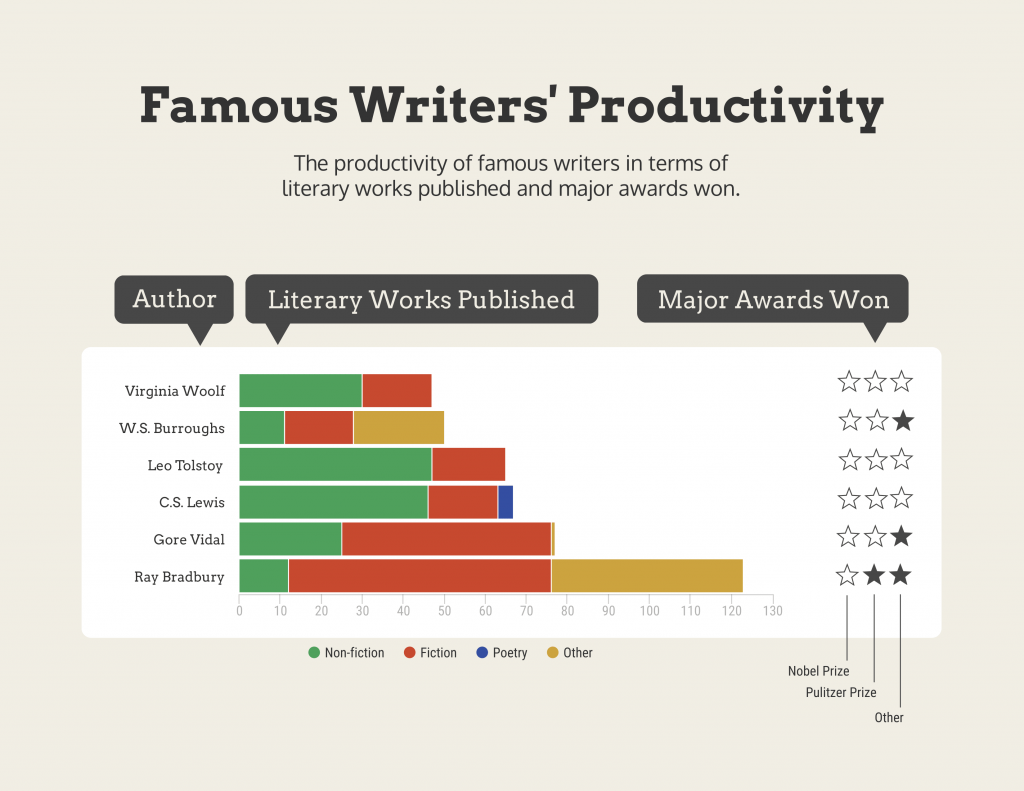
7. Area chart
Similar to line charts but with the area below the lines filled, making them suitable for showing cumulative data.
Area charts are close cousins of line charts but come with a twist.
Imagine plotting the sales of a product over several months. In an area chart, the space between the line and the x-axis is filled, providing a visual representation of the cumulative total.
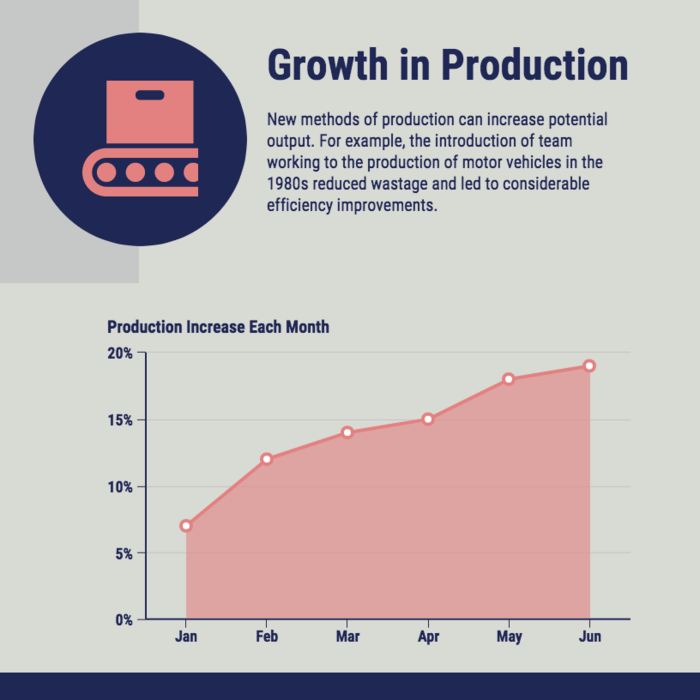
This makes it easy to see how values stack up over time, making area charts a valuable tool for tracking trends in data.
For area charts, use them to visualize cumulative data and trends, but avoid overcrowding the chart. Add labels, especially at significant points and make sure the area under the lines is filled with a visually appealing color gradient.
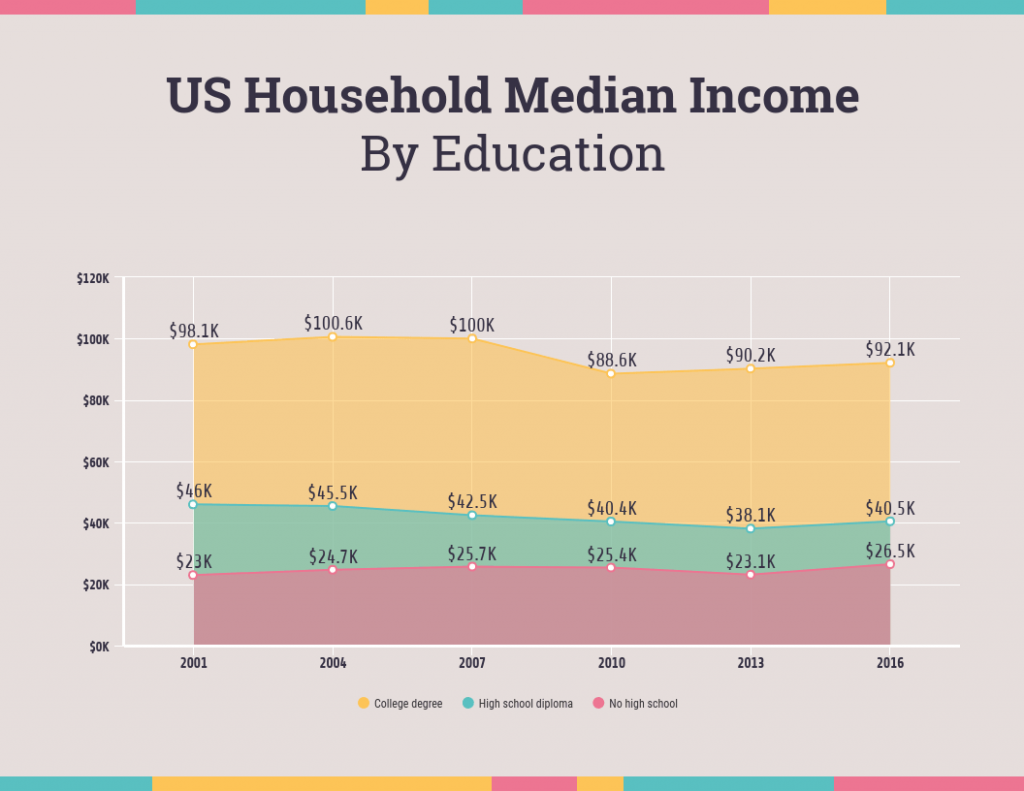
8. Tabular presentation
Presenting data in rows and columns, often used for precise data values and comparisons.
Tabular data presentation is all about clarity and precision. Think of it as presenting numerical data in a structured grid, with rows and columns clearly displaying individual data points.
A table is invaluable for showcasing detailed data, facilitating comparisons and presenting numerical information that needs to be exact. They’re commonly used in reports, spreadsheets and academic papers.
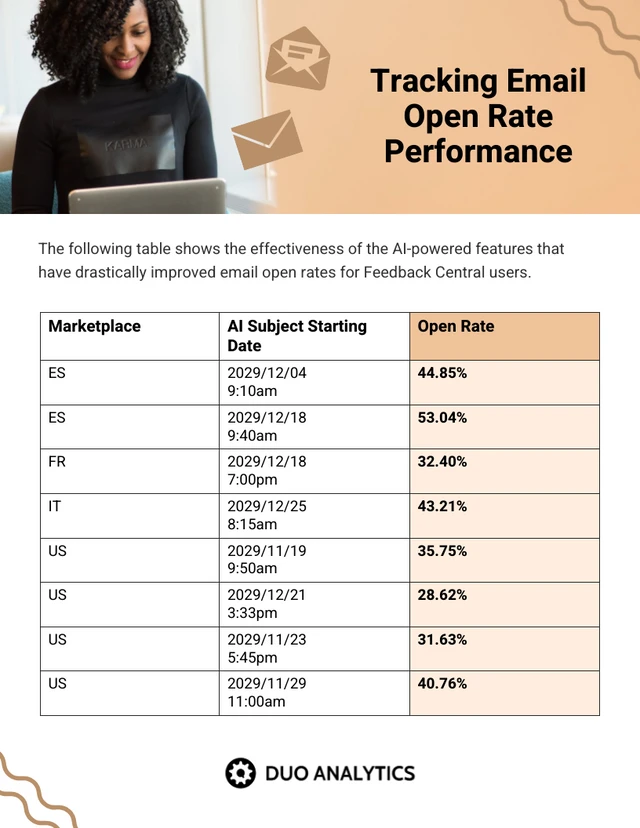
When presenting tabular data, organize it neatly with clear headers and appropriate column widths. Highlight important data points or patterns using shading or font formatting for better readability.
9. Textual data
Utilizing written or descriptive content to explain or complement data, such as annotations or explanatory text.
Textual data presentation may not involve charts or graphs, but it’s one of the most used qualitative data presentation examples.
It involves using written content to provide context, explanations or annotations alongside data visuals. Think of it as the narrative that guides your audience through the data.
Well-crafted textual data can make complex information more accessible and help your audience understand the significance of the numbers and visuals.
Textual data is your chance to tell a story. Break down complex information into bullet points or short paragraphs and use headings to guide the reader’s attention.
10. Pictogram
Using simple icons or images to represent data is especially useful for conveying information in a visually intuitive manner.
Pictograms are all about harnessing the power of images to convey data in an easy-to-understand way.
Instead of using numbers or complex graphs, you use simple icons or images to represent data points.
For instance, you could use a thumbs up emoji to illustrate customer satisfaction levels, where each face represents a different level of satisfaction.
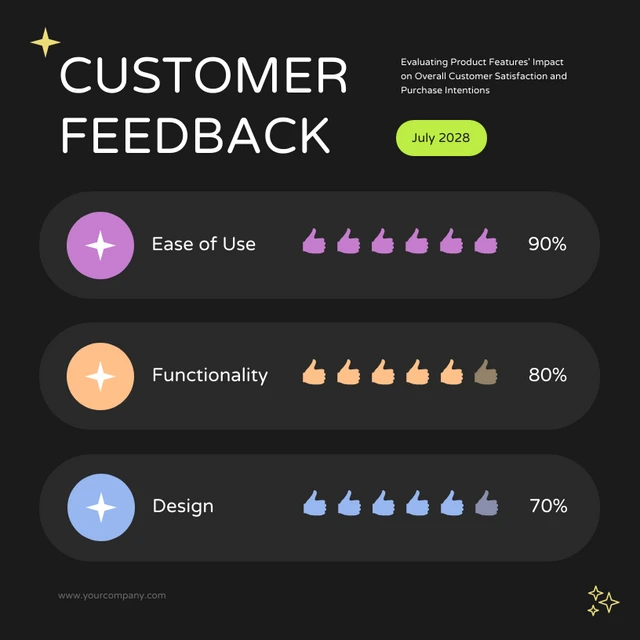
Pictograms are great for conveying data visually, so choose symbols that are easy to interpret and relevant to the data. Use consistent scaling and a legend to explain the symbols’ meanings, ensuring clarity in your presentation.
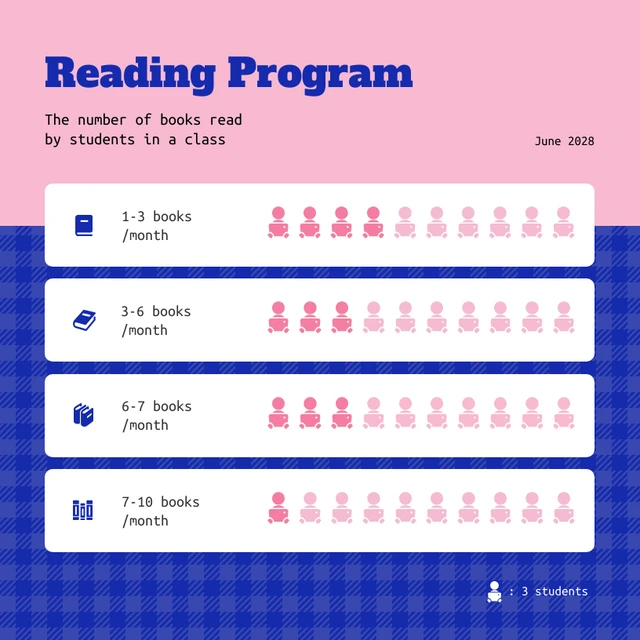
Looking for more data presentation ideas? Use the Venngage graph maker or browse through our gallery of chart templates to pick a template and get started!
A comprehensive data presentation should include several key elements to effectively convey information and insights to your audience. Here’s a list of what should be included in a data presentation:
1. Title and objective
- Begin with a clear and informative title that sets the context for your presentation.
- State the primary objective or purpose of the presentation to provide a clear focus.
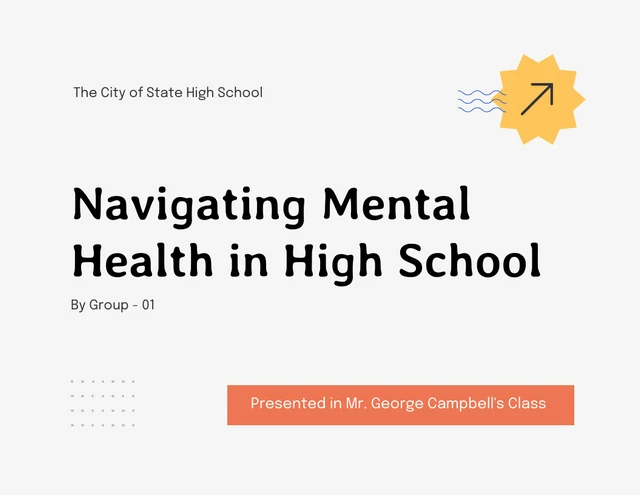
2. Key data points
- Present the most essential data points or findings that align with your objective.
- Use charts, graphical presentations or visuals to illustrate these key points for better comprehension.
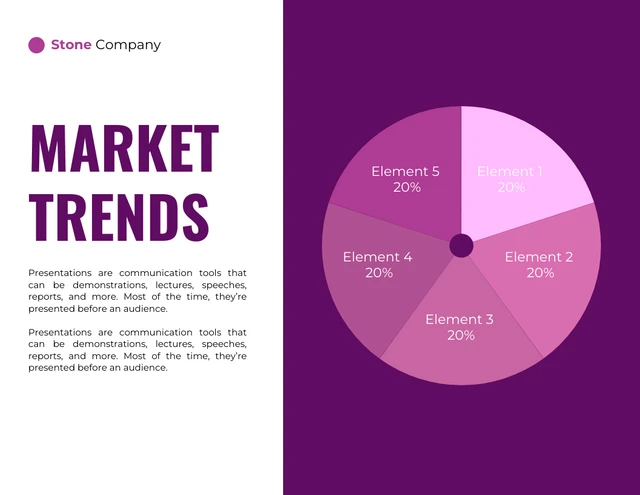
3. Context and significance
- Provide a brief overview of the context in which the data was collected and why it’s significant.
- Explain how the data relates to the larger picture or the problem you’re addressing.
4. Key takeaways
- Summarize the main insights or conclusions that can be drawn from the data.
- Highlight the key takeaways that the audience should remember.
5. Visuals and charts
- Use clear and appropriate visual aids to complement the data.
- Ensure that visuals are easy to understand and support your narrative.
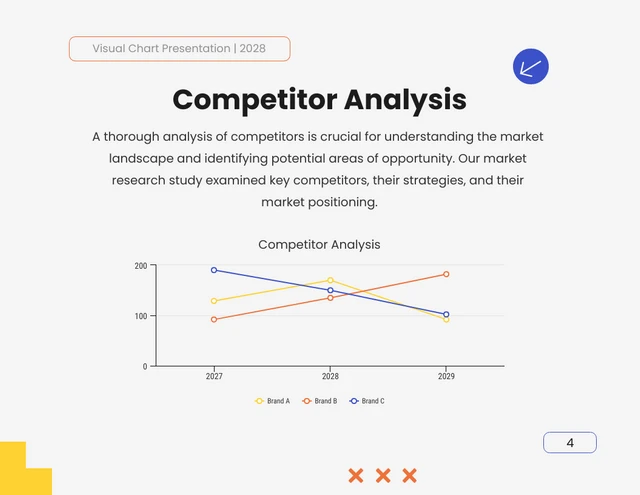
6. Implications or actions
- Discuss the practical implications of the data or any recommended actions.
- If applicable, outline next steps or decisions that should be taken based on the data.
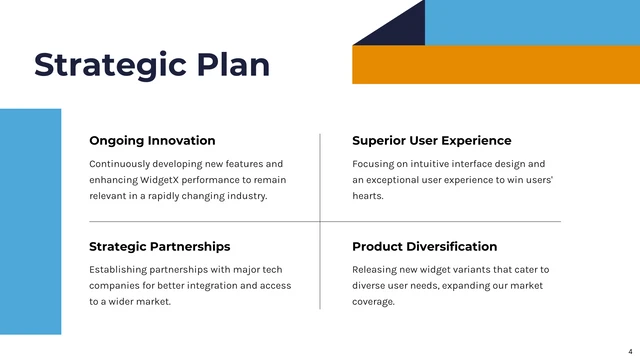
7. Q&A and discussion
- Allocate time for questions and open discussion to engage the audience.
- Address queries and provide additional insights or context as needed.
Presenting data is a crucial skill in various professional fields, from business to academia and beyond. To ensure your data presentations hit the mark, here are some common mistakes that you should steer clear of:
Overloading with data
Presenting too much data at once can overwhelm your audience. Focus on the key points and relevant information to keep the presentation concise and focused. Here are some free data visualization tools you can use to convey data in an engaging and impactful way.
Assuming everyone’s on the same page
It’s easy to assume that your audience understands as much about the topic as you do. But this can lead to either dumbing things down too much or diving into a bunch of jargon that leaves folks scratching their heads. Take a beat to figure out where your audience is coming from and tailor your presentation accordingly.
Misleading visuals
Using misleading visuals, such as distorted scales or inappropriate chart types can distort the data’s meaning. Pick the right data infographics and understandable charts to ensure that your visual representations accurately reflect the data.
Not providing context
Data without context is like a puzzle piece with no picture on it. Without proper context, data may be meaningless or misinterpreted. Explain the background, methodology and significance of the data.

Not citing sources properly
Neglecting to cite sources and provide citations for your data can erode its credibility. Always attribute data to its source and utilize reliable sources for your presentation.
Not telling a story
Avoid simply presenting numbers. If your presentation lacks a clear, engaging story that takes your audience on a journey from the beginning (setting the scene) through the middle (data analysis) to the end (the big insights and recommendations), you’re likely to lose their interest.
Infographics are great for storytelling because they mix cool visuals with short and sweet text to explain complicated stuff in a fun and easy way. Create one with Venngage’s free infographic maker to create a memorable story that your audience will remember.
Ignoring data quality
Presenting data without first checking its quality and accuracy can lead to misinformation. Validate and clean your data before presenting it.
Simplify your visuals
Fancy charts might look cool, but if they confuse people, what’s the point? Go for the simplest visual that gets your message across. Having a dilemma between presenting data with infographics v.s data design? This article on the difference between data design and infographics might help you out.
Missing the emotional connection
Data isn’t just about numbers; it’s about people and real-life situations. Don’t forget to sprinkle in some human touch, whether it’s through relatable stories, examples or showing how the data impacts real lives.
Skipping the actionable insights
At the end of the day, your audience wants to know what they should do with all the data. If you don’t wrap up with clear, actionable insights or recommendations, you’re leaving them hanging. Always finish up with practical takeaways and the next steps.
Can you provide some data presentation examples for business reports?
Business reports often benefit from data presentation through bar charts showing sales trends over time, pie charts displaying market share,or tables presenting financial performance metrics like revenue and profit margins.
What are some creative data presentation examples for academic presentations?
Creative data presentation ideas for academic presentations include using statistical infographics to illustrate research findings and statistical data, incorporating storytelling techniques to engage the audience or utilizing heat maps to visualize data patterns.
What are the key considerations when choosing the right data presentation format?
When choosing a chart format , consider factors like data complexity, audience expertise and the message you want to convey. Options include charts (e.g., bar, line, pie), tables, heat maps, data visualization infographics and interactive dashboards.
Knowing the type of data visualization that best serves your data is just half the battle. Here are some best practices for data visualization to make sure that the final output is optimized.
How can I choose the right data presentation method for my data?
To select the right data presentation method, start by defining your presentation’s purpose and audience. Then, match your data type (e.g., quantitative, qualitative) with suitable visualization techniques (e.g., histograms, word clouds) and choose an appropriate presentation format (e.g., slide deck, report, live demo).
For more presentation ideas , check out this guide on how to make a good presentation or use a presentation software to simplify the process.
How can I make my data presentations more engaging and informative?
To enhance data presentations, use compelling narratives, relatable examples and fun data infographics that simplify complex data. Encourage audience interaction, offer actionable insights and incorporate storytelling elements to engage and inform effectively.
The opening of your presentation holds immense power in setting the stage for your audience. To design a presentation and convey your data in an engaging and informative, try out Venngage’s free presentation maker to pick the right presentation design for your audience and topic.
What is the difference between data visualization and data presentation?
Data presentation typically involves conveying data reports and insights to an audience, often using visuals like charts and graphs. Data visualization , on the other hand, focuses on creating those visual representations of data to facilitate understanding and analysis.
Now that you’ve learned a thing or two about how to use these methods of data presentation to tell a compelling data story , it’s time to take these strategies and make them your own.
But here’s the deal: these aren’t just one-size-fits-all solutions. Remember that each example we’ve uncovered here is not a rigid template but a source of inspiration. It’s all about making your audience go, “Wow, I get it now!”
Think of your data presentations as your canvas – it’s where you paint your story, convey meaningful insights and make real change happen.
So, go forth, present your data with confidence and purpose and watch as your strategic influence grows, one compelling presentation at a time.
Discover popular designs
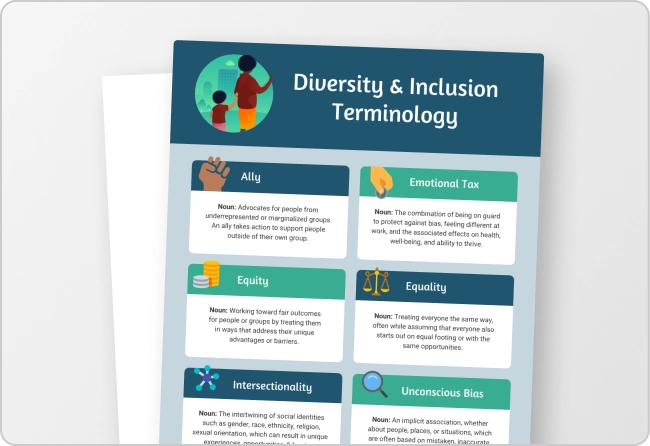
Infographic maker
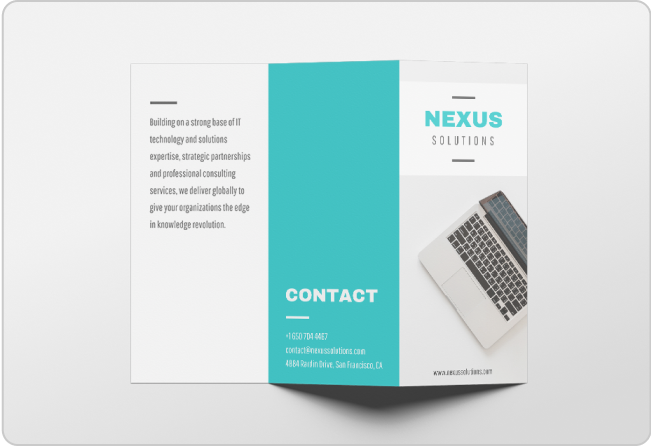
Brochure maker
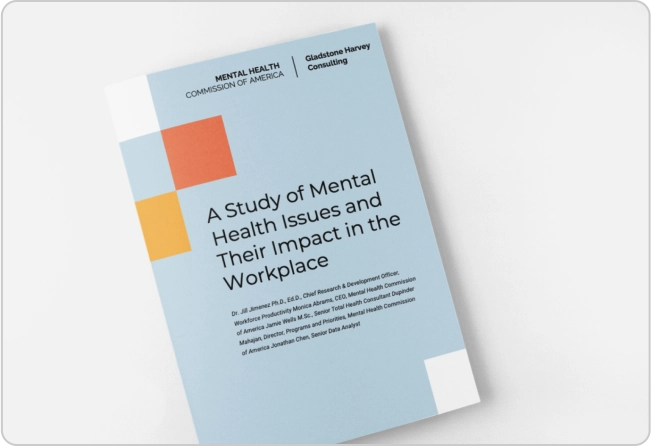
White paper online
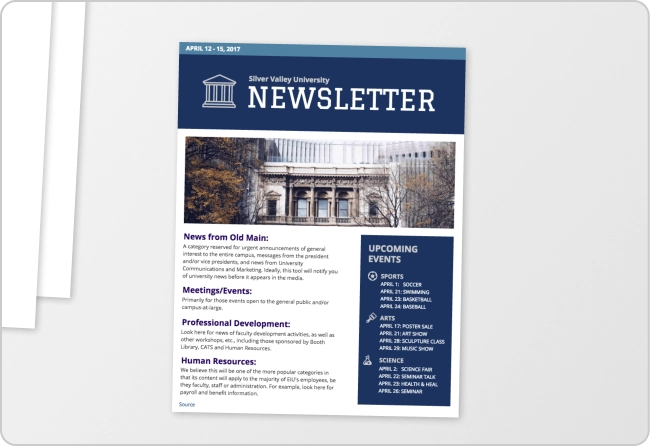
Newsletter creator
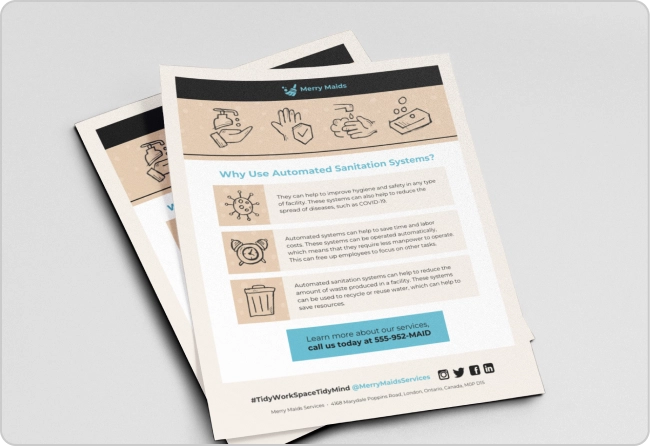
Flyer maker
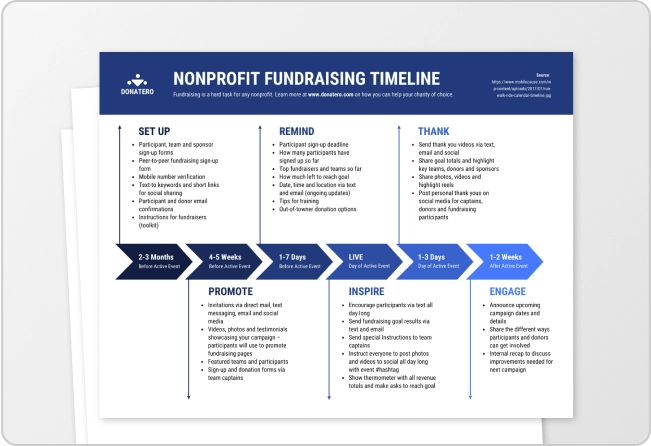
Timeline maker
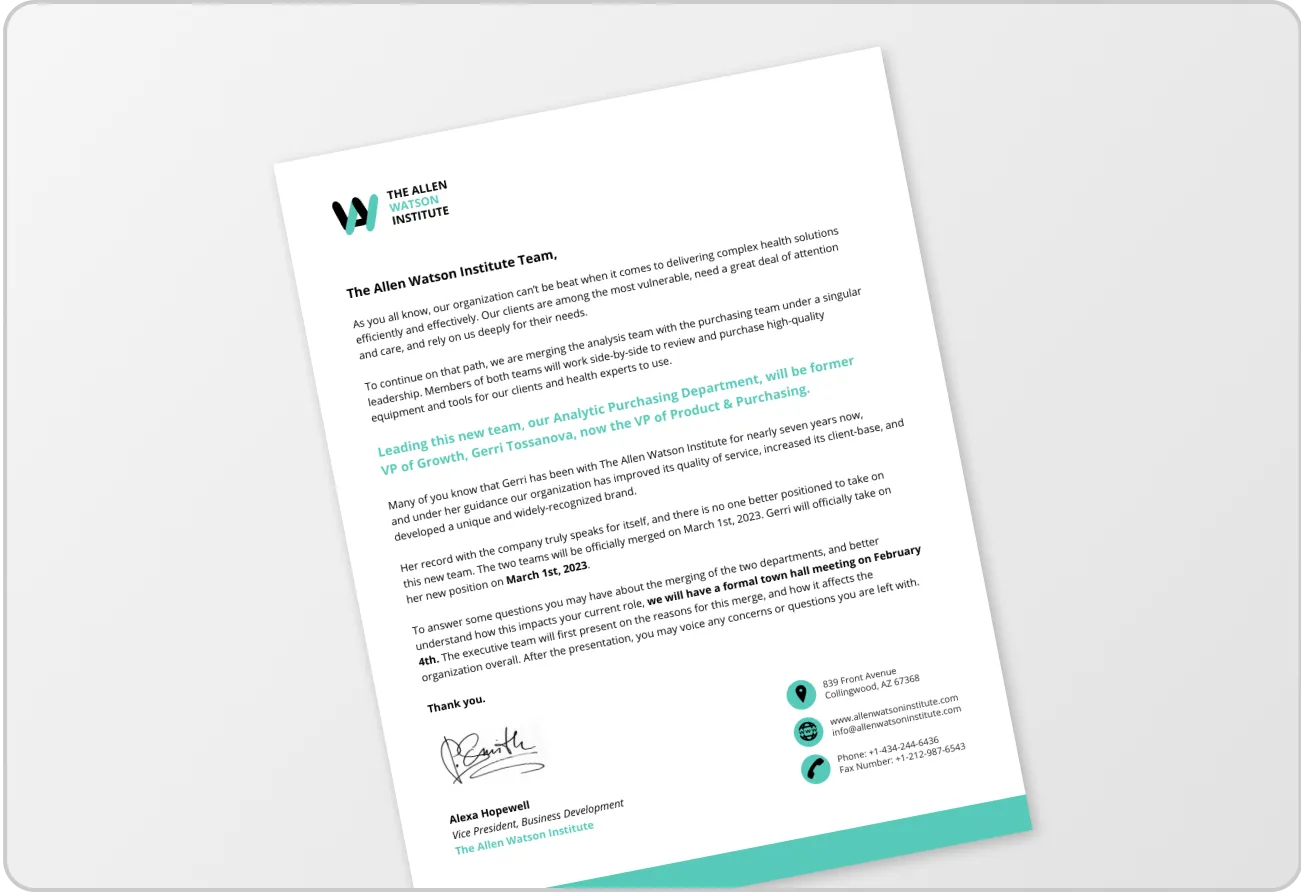
Letterhead maker
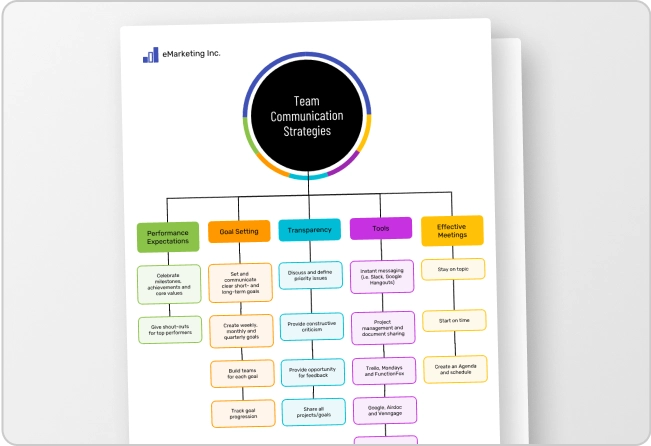
Mind map maker
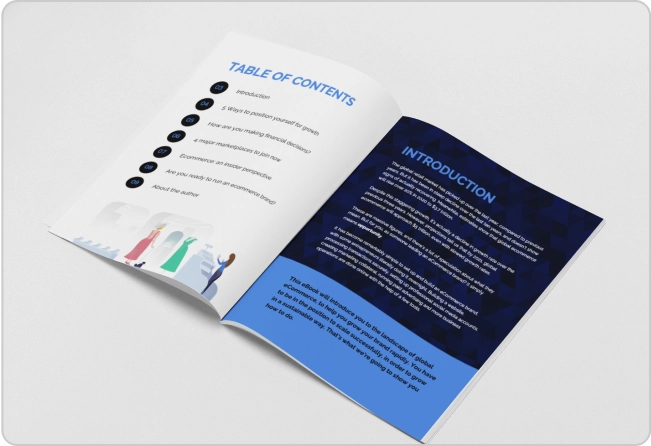
Ebook maker
A Step-by-Step Guide to the Data Analysis Process
Like any scientific discipline, data analysis follows a rigorous step-by-step process. Each stage requires different skills and know-how. To get meaningful insights, though, it’s important to understand the process as a whole. An underlying framework is invaluable for producing results that stand up to scrutiny.
In this post, we’ll explore the main steps in the data analysis process. This will cover how to define your goal, collect data, and carry out an analysis. Where applicable, we’ll also use examples and highlight a few tools to make the journey easier. When you’re done, you’ll have a much better understanding of the basics. This will help you tweak the process to fit your own needs.
Here are the steps we’ll take you through:
- Defining the question
- Collecting the data
- Cleaning the data
- Analyzing the data
- Sharing your results
- Embracing failure
On popular request, we’ve also developed a video based on this article. Scroll further along this article to watch that.
Ready? Let’s get started with step one.
1. Step one: Defining the question
The first step in any data analysis process is to define your objective. In data analytics jargon, this is sometimes called the ‘problem statement’.
Defining your objective means coming up with a hypothesis and figuring how to test it. Start by asking: What business problem am I trying to solve? While this might sound straightforward, it can be trickier than it seems. For instance, your organization’s senior management might pose an issue, such as: “Why are we losing customers?” It’s possible, though, that this doesn’t get to the core of the problem. A data analyst’s job is to understand the business and its goals in enough depth that they can frame the problem the right way.
Let’s say you work for a fictional company called TopNotch Learning. TopNotch creates custom training software for its clients. While it is excellent at securing new clients, it has much lower repeat business. As such, your question might not be, “Why are we losing customers?” but, “Which factors are negatively impacting the customer experience?” or better yet: “How can we boost customer retention while minimizing costs?”
Now you’ve defined a problem, you need to determine which sources of data will best help you solve it. This is where your business acumen comes in again. For instance, perhaps you’ve noticed that the sales process for new clients is very slick, but that the production team is inefficient. Knowing this, you could hypothesize that the sales process wins lots of new clients, but the subsequent customer experience is lacking. Could this be why customers don’t come back? Which sources of data will help you answer this question?
Tools to help define your objective
Defining your objective is mostly about soft skills, business knowledge, and lateral thinking. But you’ll also need to keep track of business metrics and key performance indicators (KPIs). Monthly reports can allow you to track problem points in the business. Some KPI dashboards come with a fee, like Databox and DashThis . However, you’ll also find open-source software like Grafana , Freeboard , and Dashbuilder . These are great for producing simple dashboards, both at the beginning and the end of the data analysis process.
2. Step two: Collecting the data
Once you’ve established your objective, you’ll need to create a strategy for collecting and aggregating the appropriate data. A key part of this is determining which data you need. This might be quantitative (numeric) data, e.g. sales figures, or qualitative (descriptive) data, such as customer reviews. All data fit into one of three categories: first-party, second-party, and third-party data. Let’s explore each one.
What is first-party data?
First-party data are data that you, or your company, have directly collected from customers. It might come in the form of transactional tracking data or information from your company’s customer relationship management (CRM) system. Whatever its source, first-party data is usually structured and organized in a clear, defined way. Other sources of first-party data might include customer satisfaction surveys, focus groups, interviews, or direct observation.
What is second-party data?
To enrich your analysis, you might want to secure a secondary data source. Second-party data is the first-party data of other organizations. This might be available directly from the company or through a private marketplace. The main benefit of second-party data is that they are usually structured, and although they will be less relevant than first-party data, they also tend to be quite reliable. Examples of second-party data include website, app or social media activity, like online purchase histories, or shipping data.
What is third-party data?
Third-party data is data that has been collected and aggregated from numerous sources by a third-party organization. Often (though not always) third-party data contains a vast amount of unstructured data points (big data). Many organizations collect big data to create industry reports or to conduct market research. The research and advisory firm Gartner is a good real-world example of an organization that collects big data and sells it on to other companies. Open data repositories and government portals are also sources of third-party data .
Tools to help you collect data
Once you’ve devised a data strategy (i.e. you’ve identified which data you need, and how best to go about collecting them) there are many tools you can use to help you. One thing you’ll need, regardless of industry or area of expertise, is a data management platform (DMP). A DMP is a piece of software that allows you to identify and aggregate data from numerous sources, before manipulating them, segmenting them, and so on. There are many DMPs available. Some well-known enterprise DMPs include Salesforce DMP , SAS , and the data integration platform, Xplenty . If you want to play around, you can also try some open-source platforms like Pimcore or D:Swarm .
Want to learn more about what data analytics is and the process a data analyst follows? We cover this topic (and more) in our free introductory short course for beginners. Check out tutorial one: An introduction to data analytics .
3. Step three: Cleaning the data
Once you’ve collected your data, the next step is to get it ready for analysis. This means cleaning, or ‘scrubbing’ it, and is crucial in making sure that you’re working with high-quality data . Key data cleaning tasks include:
- Removing major errors, duplicates, and outliers —all of which are inevitable problems when aggregating data from numerous sources.
- Removing unwanted data points —extracting irrelevant observations that have no bearing on your intended analysis.
- Bringing structure to your data —general ‘housekeeping’, i.e. fixing typos or layout issues, which will help you map and manipulate your data more easily.
- Filling in major gaps —as you’re tidying up, you might notice that important data are missing. Once you’ve identified gaps, you can go about filling them.
A good data analyst will spend around 70-90% of their time cleaning their data. This might sound excessive. But focusing on the wrong data points (or analyzing erroneous data) will severely impact your results. It might even send you back to square one…so don’t rush it! You’ll find a step-by-step guide to data cleaning here . You may be interested in this introductory tutorial to data cleaning, hosted by Dr. Humera Noor Minhas.
Carrying out an exploratory analysis
Another thing many data analysts do (alongside cleaning data) is to carry out an exploratory analysis. This helps identify initial trends and characteristics, and can even refine your hypothesis. Let’s use our fictional learning company as an example again. Carrying out an exploratory analysis, perhaps you notice a correlation between how much TopNotch Learning’s clients pay and how quickly they move on to new suppliers. This might suggest that a low-quality customer experience (the assumption in your initial hypothesis) is actually less of an issue than cost. You might, therefore, take this into account.
Tools to help you clean your data
Cleaning datasets manually—especially large ones—can be daunting. Luckily, there are many tools available to streamline the process. Open-source tools, such as OpenRefine , are excellent for basic data cleaning, as well as high-level exploration. However, free tools offer limited functionality for very large datasets. Python libraries (e.g. Pandas) and some R packages are better suited for heavy data scrubbing. You will, of course, need to be familiar with the languages. Alternatively, enterprise tools are also available. For example, Data Ladder , which is one of the highest-rated data-matching tools in the industry. There are many more. Why not see which free data cleaning tools you can find to play around with?
4. Step four: Analyzing the data
Finally, you’ve cleaned your data. Now comes the fun bit—analyzing it! The type of data analysis you carry out largely depends on what your goal is. But there are many techniques available. Univariate or bivariate analysis, time-series analysis, and regression analysis are just a few you might have heard of. More important than the different types, though, is how you apply them. This depends on what insights you’re hoping to gain. Broadly speaking, all types of data analysis fit into one of the following four categories.
Descriptive analysis
Descriptive analysis identifies what has already happened . It is a common first step that companies carry out before proceeding with deeper explorations. As an example, let’s refer back to our fictional learning provider once more. TopNotch Learning might use descriptive analytics to analyze course completion rates for their customers. Or they might identify how many users access their products during a particular period. Perhaps they’ll use it to measure sales figures over the last five years. While the company might not draw firm conclusions from any of these insights, summarizing and describing the data will help them to determine how to proceed.
Learn more: What is descriptive analytics?
Diagnostic analysis
Diagnostic analytics focuses on understanding why something has happened . It is literally the diagnosis of a problem, just as a doctor uses a patient’s symptoms to diagnose a disease. Remember TopNotch Learning’s business problem? ‘Which factors are negatively impacting the customer experience?’ A diagnostic analysis would help answer this. For instance, it could help the company draw correlations between the issue (struggling to gain repeat business) and factors that might be causing it (e.g. project costs, speed of delivery, customer sector, etc.) Let’s imagine that, using diagnostic analytics, TopNotch realizes its clients in the retail sector are departing at a faster rate than other clients. This might suggest that they’re losing customers because they lack expertise in this sector. And that’s a useful insight!
Predictive analysis
Predictive analysis allows you to identify future trends based on historical data . In business, predictive analysis is commonly used to forecast future growth, for example. But it doesn’t stop there. Predictive analysis has grown increasingly sophisticated in recent years. The speedy evolution of machine learning allows organizations to make surprisingly accurate forecasts. Take the insurance industry. Insurance providers commonly use past data to predict which customer groups are more likely to get into accidents. As a result, they’ll hike up customer insurance premiums for those groups. Likewise, the retail industry often uses transaction data to predict where future trends lie, or to determine seasonal buying habits to inform their strategies. These are just a few simple examples, but the untapped potential of predictive analysis is pretty compelling.
Prescriptive analysis
Prescriptive analysis allows you to make recommendations for the future. This is the final step in the analytics part of the process. It’s also the most complex. This is because it incorporates aspects of all the other analyses we’ve described. A great example of prescriptive analytics is the algorithms that guide Google’s self-driving cars. Every second, these algorithms make countless decisions based on past and present data, ensuring a smooth, safe ride. Prescriptive analytics also helps companies decide on new products or areas of business to invest in.
Learn more: What are the different types of data analysis?
5. Step five: Sharing your results
You’ve finished carrying out your analyses. You have your insights. The final step of the data analytics process is to share these insights with the wider world (or at least with your organization’s stakeholders!) This is more complex than simply sharing the raw results of your work—it involves interpreting the outcomes, and presenting them in a manner that’s digestible for all types of audiences. Since you’ll often present information to decision-makers, it’s very important that the insights you present are 100% clear and unambiguous. For this reason, data analysts commonly use reports, dashboards, and interactive visualizations to support their findings.
How you interpret and present results will often influence the direction of a business. Depending on what you share, your organization might decide to restructure, to launch a high-risk product, or even to close an entire division. That’s why it’s very important to provide all the evidence that you’ve gathered, and not to cherry-pick data. Ensuring that you cover everything in a clear, concise way will prove that your conclusions are scientifically sound and based on the facts. On the flip side, it’s important to highlight any gaps in the data or to flag any insights that might be open to interpretation. Honest communication is the most important part of the process. It will help the business, while also helping you to excel at your job!
Tools for interpreting and sharing your findings
There are tons of data visualization tools available, suited to different experience levels. Popular tools requiring little or no coding skills include Google Charts , Tableau , Datawrapper , and Infogram . If you’re familiar with Python and R, there are also many data visualization libraries and packages available. For instance, check out the Python libraries Plotly , Seaborn , and Matplotlib . Whichever data visualization tools you use, make sure you polish up your presentation skills, too. Remember: Visualization is great, but communication is key!
You can learn more about storytelling with data in this free, hands-on tutorial . We show you how to craft a compelling narrative for a real dataset, resulting in a presentation to share with key stakeholders. This is an excellent insight into what it’s really like to work as a data analyst!
6. Step six: Embrace your failures
The last ‘step’ in the data analytics process is to embrace your failures. The path we’ve described above is more of an iterative process than a one-way street. Data analytics is inherently messy, and the process you follow will be different for every project. For instance, while cleaning data, you might spot patterns that spark a whole new set of questions. This could send you back to step one (to redefine your objective). Equally, an exploratory analysis might highlight a set of data points you’d never considered using before. Or maybe you find that the results of your core analyses are misleading or erroneous. This might be caused by mistakes in the data, or human error earlier in the process.
While these pitfalls can feel like failures, don’t be disheartened if they happen. Data analysis is inherently chaotic, and mistakes occur. What’s important is to hone your ability to spot and rectify errors. If data analytics was straightforward, it might be easier, but it certainly wouldn’t be as interesting. Use the steps we’ve outlined as a framework, stay open-minded, and be creative. If you lose your way, you can refer back to the process to keep yourself on track.
In this post, we’ve covered the main steps of the data analytics process. These core steps can be amended, re-ordered and re-used as you deem fit, but they underpin every data analyst’s work:
- Define the question —What business problem are you trying to solve? Frame it as a question to help you focus on finding a clear answer.
- Collect data —Create a strategy for collecting data. Which data sources are most likely to help you solve your business problem?
- Clean the data —Explore, scrub, tidy, de-dupe, and structure your data as needed. Do whatever you have to! But don’t rush…take your time!
- Analyze the data —Carry out various analyses to obtain insights. Focus on the four types of data analysis: descriptive, diagnostic, predictive, and prescriptive.
- Share your results —How best can you share your insights and recommendations? A combination of visualization tools and communication is key.
- Embrace your mistakes —Mistakes happen. Learn from them. This is what transforms a good data analyst into a great one.
What next? From here, we strongly encourage you to explore the topic on your own. Get creative with the steps in the data analysis process, and see what tools you can find. As long as you stick to the core principles we’ve described, you can create a tailored technique that works for you.
To learn more, check out our free, 5-day data analytics short course . You might also be interested in the following:
- These are the top 9 data analytics tools
- 10 great places to find free datasets for your next project
- How to build a data analytics portfolio
What is Data Visualization? Definition, Examples, Best Practices
This guide provides an introduction to data visualization, including real-world examples, best practices and editable templates.
- Share on LinkedIn
- Share through Email
- Print this page
- Bookmark this page
Resource Details
June 5, 2020
Data visualization is the visual presentation of data or information. The goal of data visualization is to communicate data or information clearly and effectively to readers. Typically, data is visualized in the form of a chart, infographic, diagram, or map.
The field of data visualization combines both art and data science. While data visualization can be creative and pleasing to look at, it should also be functional in its visual communication of the data.
This resource explains the fundamentals of data visualization, including examples of different types of data visualizations and when and how to use them to illustrate findings and insights.
Do you have feedback on this resource?
Thank you for your feedback as we strive to curate and publish resources to help social impact organizations succeed with data.
Send us a note
Explore More
Related Guides & Resources
Getting started with data visualization.
Visualizing data is one of the most effective ways of communicating data. This can take many forms from live digital data dashboards to static charts shared in social media channels.
Communicating Results: Design your Visualization
Let’s get visual: nonprofit data visualization.
Data Collection, Presentation and Analysis
- First Online: 25 May 2023
Cite this chapter
- Uche M. Mbanaso 4 ,
- Lucienne Abrahams 5 &
- Kennedy Chinedu Okafor 6
809 Accesses
This chapter covers the topics of data collection, data presentation and data analysis. It gives attention to data collection for studies based on experiments, on data derived from existing published or unpublished data sets, on observation, on simulation and digital twins, on surveys, on interviews and on focus group discussions. One of the interesting features of this chapter is the section dealing with using measurement scales in quantitative research, including nominal scales, ordinal scales, interval scales and ratio scales. It explains key facets of qualitative research including ethical clearance requirements. The chapter discusses the importance of data visualization as key to effective presentation of data, including tabular forms, graphical forms and visual charts such as those generated by Atlas.ti analytical software.
This is a preview of subscription content, log in via an institution to check access.
Access this chapter
Subscribe and save.
- Get 10 units per month
- Download Article/Chapter or eBook
- 1 Unit = 1 Article or 1 Chapter
- Cancel anytime
- Available as PDF
- Read on any device
- Instant download
- Own it forever
- Available as EPUB and PDF
- Compact, lightweight edition
- Dispatched in 3 to 5 business days
- Free shipping worldwide - see info
- Durable hardcover edition
Tax calculation will be finalised at checkout
Purchases are for personal use only
Institutional subscriptions
Bibliography
Abdullah, M. F., & Ahmad, K. (2013). The mapping process of unstructured data to structured data. Proceedings of the 2013 International Conference on Research and Innovation in Information Systems (ICRIIS) , Malaysia , 151–155. https://doi.org/10.1109/ICRIIS.2013.6716700
Adnan, K., & Akbar, R. (2019). An analytical study of information extraction from unstructured and multidimensional big data. Journal of Big Data, 6 , 91. https://doi.org/10.1186/s40537-019-0254-8
Article Google Scholar
Alsheref, F. K., & Fattoh, I. E. (2020). Medical text annotation tool based on IBM Watson Platform. Proceedings of the 2020 6th international conference on advanced computing and communication systems (ICACCS) , India , 1312–1316. https://doi.org/10.1109/ICACCS48705.2020.9074309
Cinque, M., Cotroneo, D., Della Corte, R., & Pecchia, A. (2014). What logs should you look at when an application fails? Insights from an industrial case study. Proceedings of the 2014 44th Annual IEEE/IFIP International Conference on Dependable Systems and Networks , USA , 690–695. https://doi.org/10.1109/DSN.2014.69
Gideon, L. (Ed.). (2012). Handbook of survey methodology for the social sciences . Springer.
Google Scholar
Leedy, P., & Ormrod, J. (2015). Practical research planning and design (12th ed.). Pearson Education.
Madaan, A., Wang, X., Hall, W., & Tiropanis, T. (2018). Observing data in IoT worlds: What and how to observe? In Living in the Internet of Things: Cybersecurity of the IoT – 2018 (pp. 1–7). https://doi.org/10.1049/cp.2018.0032
Chapter Google Scholar
Mahajan, P., & Naik, C. (2019). Development of integrated IoT and machine learning based data collection and analysis system for the effective prediction of agricultural residue/biomass availability to regenerate clean energy. Proceedings of the 2019 9th International Conference on Emerging Trends in Engineering and Technology – Signal and Information Processing (ICETET-SIP-19) , India , 1–5. https://doi.org/10.1109/ICETET-SIP-1946815.2019.9092156 .
Mahmud, M. S., Huang, J. Z., Salloum, S., Emara, T. Z., & Sadatdiynov, K. (2020). A survey of data partitioning and sampling methods to support big data analysis. Big Data Mining and Analytics, 3 (2), 85–101. https://doi.org/10.26599/BDMA.2019.9020015
Miswar, S., & Kurniawan, N. B. (2018). A systematic literature review on survey data collection system. Proceedings of the 2018 International Conference on Information Technology Systems and Innovation (ICITSI) , Indonesia , 177–181. https://doi.org/10.1109/ICITSI.2018.8696036
Mosina, C. (2020). Understanding the diffusion of the internet: Redesigning the global diffusion of the internet framework (Research report, Master of Arts in ICT Policy and Regulation). LINK Centre, University of the Witwatersrand. https://hdl.handle.net/10539/30723
Nkamisa, S. (2021). Investigating the integration of drone management systems to create an enabling remote piloted aircraft regulatory environment in South Africa (Research report, Master of Arts in ICT Policy and Regulation). LINK Centre, University of the Witwatersrand. https://hdl.handle.net/10539/33883
QuestionPro. (2020). Survey research: Definition, examples and methods . https://www.questionpro.com/article/survey-research.html
Rajanikanth, J. & Kanth, T. V. R. (2017). An explorative data analysis on Bangalore City Weather with hybrid data mining techniques using R. Proceedings of the 2017 International Conference on Current Trends in Computer, Electrical, Electronics and Communication (CTCEEC) , India , 1121-1125. https://doi/10.1109/CTCEEC.2017.8455008
Rao, R. (2003). From unstructured data to actionable intelligence. IT Professional, 5 , 29–35. https://www.researchgate.net/publication/3426648_From_Unstructured_Data_to_Actionable_Intelligence
Schulze, P. (2009). Design of the research instrument. In P. Schulze (Ed.), Balancing exploitation and exploration: Organizational antecedents and performance effects of innovation strategies (pp. 116–141). Gabler. https://doi.org/10.1007/978-3-8349-8397-8_6
Usanov, A. (2015). Assessing cybersecurity: A meta-analysis of threats, trends and responses to cyber attacks . The Hague Centre for Strategic Studies. https://www.researchgate.net/publication/319677972_Assessing_Cyber_Security_A_Meta-analysis_of_Threats_Trends_and_Responses_to_Cyber_Attacks
Van de Kaa, G., De Vries, H. J., van Heck, E., & van den Ende, J. (2007). The emergence of standards: A meta-analysis. Proceedings of the 2007 40th Annual Hawaii International Conference on Systems Science (HICSS’07) , USA , 173a–173a. https://doi.org/10.1109/HICSS.2007.529
Download references
Author information
Authors and affiliations.
Centre for Cybersecurity Studies, Nasarawa State University, Keffi, Nigeria
Uche M. Mbanaso
LINK Centre, University of the Witwatersrand, Johannesburg, South Africa
Lucienne Abrahams
Department of Mechatronics Engineering, Federal University of Technology, Owerri, Nigeria
Kennedy Chinedu Okafor
You can also search for this author in PubMed Google Scholar
Rights and permissions
Reprints and permissions
Copyright information
© 2023 The Author(s), under exclusive license to Springer Nature Switzerland AG
About this chapter
Mbanaso, U.M., Abrahams, L., Okafor, K.C. (2023). Data Collection, Presentation and Analysis. In: Research Techniques for Computer Science, Information Systems and Cybersecurity. Springer, Cham. https://doi.org/10.1007/978-3-031-30031-8_7
Download citation
DOI : https://doi.org/10.1007/978-3-031-30031-8_7
Published : 25 May 2023
Publisher Name : Springer, Cham
Print ISBN : 978-3-031-30030-1
Online ISBN : 978-3-031-30031-8
eBook Packages : Engineering Engineering (R0)
Share this chapter
Anyone you share the following link with will be able to read this content:
Sorry, a shareable link is not currently available for this article.
Provided by the Springer Nature SharedIt content-sharing initiative
- Publish with us
Policies and ethics
- Find a journal
- Track your research

What is data analytics?
Data analytics is how organizations turn raw data into business value. By analyzing large datasets, organizations can uncover patterns that yield insights, inform decision-making, and lead to better business outcomes.
Understanding data analytics
Data analytics definition.
Data analytics is the process of analyzing, interpreting, and visualizing large, complex datasets to derive meaningful insights and make informed decisions. It encompasses a variety of techniques from statistical analysis to machine learning, aimed at analyzing past and current data to predict future trends.
Why is data analytics important?
In its raw form, data is little more than a list of facts and figures. This is why it is often compared to oil, a resource whose value is latent until it’s processed into something useful. Data analytics is important because it helps extract value from the raw material we call data.
Imagine running a global supply chain with no insight into your data—or building a budget for your next product release based on gut feelings and intuition. Without data analytics, the goal of becoming a data-driven enterprise—with optimized operations and continuously improving customer experiences—can hardly be achieved. For organizations seeking to establish and maintain competitive advantage, data analytics is key for ongoing success.
How does data analytics work?
Data analytics is a multifaceted process involving various stages to extract meaningful insights from raw data. Specialized data analytics tools and teams play an essential role in this sequence, meticulously gathering, processing, and analyzing data to derive actionable intelligence.
The following five steps help to ensure that data is accurately analyzed, leading to actionable insights that drive strategic decision-making:
Step 1: Data collection
This initial step involves gathering data from various sources, such as databases, sensors, and online transactions. Collecting diverse datasets is crucial to gaining comprehensive insights.
Step 2: Data cleansing
Once collected, the data often contains errors, duplicates, or missing values. Data cleansing involves identifying and correcting these issues to ensure accuracy and reliability in subsequent analyses.
Step 3: Data transformation
Transforming data includes normalizing, aggregating, and formatting it into a consistent and usable structure. This step prepares the data for efficient analysis by converting it into suitable formats and structures.
Step 4: Data modeling
In this phase, statistical models and algorithms are applied to the data to identify patterns, correlations, and trends. Modeling helps predict future outcomes and provides a basis for making informed decisions.
Step 5: Data visualization
Finally, the analyzed data is presented through charts, graphs, and dashboards. Visualization makes complex data understandable and highlights key insights, enabling stakeholders to make strategic decisions quickly.

Data visualization of top 100 customers overdue on payments
Data analytics types and techniques
Types of data analytics.
As data volumes grow, successfully navigating vast oceans of information requires different navigational tools depending on your destination. Increasingly, organizations approach data analytics by tailoring their strategies to answer targeted questions and achieve specific goals. Whether deciphering what happened in the past, uncovering the reasons behind those events, forecasting future possibilities, or recommending the best course of action, each type of data analytics offers unique insights and solutions. Here's a closer look at the four main types of data analytics: descriptive, diagnostic, predictive, and prescriptive.
Descriptive analytics
Descriptive analytics interrogates historical data to identify trends and patterns. It answers the "What happened?" question using techniques like data aggregation and data mining for insights into past performance. Examples include monthly sales performance reports, results from customer satisfaction surveys, and web traffic analyses that measure engagement rates.
Diagnostic analytics
Diagnostic analytics delves deeper into data to understand the causes of past events. It addresses the "Why did it happen?" question using techniques such as drill-down, data discovery, data mining, and correlations. For example, a retailer might use diagnostic analytics to understand why sales dropped in a particular month by examining influencing factors.
Predictive analytics
Predictive analytics uses statistical models and machine learning techniques to forecast future outcomes based on historical data. It answers the "What could happen?" question by identifying trends and predicting future events. One example would be a bank using predictive analytics to forecast the likelihood of a customer defaulting on a loan.
Prescriptive analytics
Prescriptive analytics recommends actions based on data analyses. It addresses the "What should we do?" question using techniques like optimization and simulation algorithms. For instance, a logistics company might use prescriptive analytics to find the most efficient route for delivery trucks.

The four types of data analytics
Data analytics techniques
Data analytics is a continuously evolving business discipline. The techniques employed can be as diverse as the datasets being examined and the goals organizations aim to achieve. To succeed with data analytics, a familiarity with the full range data analytics techniques is a must. These include:
Data mining
Data mining uses algorithms and statistical methods to extract patterns and knowledge from large datasets. This technique helps organizations discover hidden patterns and relationships in data for use with various predictive models.
Text analytics
Text analytics parses unstructured text data from emails, social media posts, customer reviews, and other types of content to derive meaning, context, and insights. It is particularly useful for sentiment analysis, entity recognition, topic modeling, and natural language processing.
Statistical analysis
Statistical analysis uses statistical tests and models to understand relationships and trends within data. This technique includes hypothesis testing, regression analysis, and variance analysis to make inferences about the data.
Machine learning
Machine learning uses algorithms that learn from and make predictions on data, improving performance over time with more data. Common methods include supervised learning, unsupervised learning, and reinforcement learning, applied in areas like recommendation systems and image recognition.
Data visualization
Data visualization is the visual representations of data through charts, graphs, and dashboards to make complex data more understandable. Effective visualization helps organizations identify trends, patterns, and outliers quickly, aiding better decision-making.
Big Data analytics
Big Data analytics focuses on large volumes of data that traditional methods cannot manage effectively. It often involves distributed computing and storage solutions to process vast amounts of information, enabling organizations to leverage Big Data for strategic insights.
Examples and applications for data analytics
Data analytics examples.
Organizations use data analytics for a range of purposes. Some use it to foresee equipment failures before they happen or tailor marketing strategies to individual preferences. Others use it to streamline delivery routes for maximum efficiency or tap into the collective mood of social media users. Here are some of the most common examples of how data analytics helps to make these possibilities a reality:
Predictive maintenance
Data analytics is regularly used for predictive maintenance, where equipment or vehicle performance data is analyzed to predict component failures. This helps organizations schedule timely maintenance and reduce unexpected breakdowns.
Customer segmentation
E-commerce platforms use data analytics for customer segmentation, analyzing browsing and purchasing behavior to group customers based on their preferences. This enables targeted marketing campaigns.
Supply chain optimization
Organizations running logistics operations analyze shipment data to optimize delivery routes, reduce transportation costs, and improve delivery times. This helps ensure efficient operations and enhance customer satisfaction.
Sentiment analysis
Social media platforms use sentiment analysis to gauge public opinion on various topics. Analyzing posts and comments enables companies to understand customer sentiment and adjust strategies accordingly.
Data analytics applications
Across various sectors, data analytics is not just a tool but a transformative force that drives progress and addresses unique challenges. Depending on the industry, data analytics can reveal critical insights that lead to greater efficiency, strategic advancements, and even life-changing improvements. Here are some compelling applications of data analytics across different fields:
In the healthcare industry, data analytics is used to predict disease outbreaks, improve patient care, and optimize hospital operations. Analyzing patient data helps in early diagnosis and personalized treatment plans, improving overall health outcomes.
Financial institutions use data analytics for fraud detection, risk management, and personalized banking services. By analyzing transaction patterns, banks can identify fraudulent activities and assess credit risk more accurately.
Retailers use data analytics to optimize inventory management, enhance the customer experience, and personalize marketing messages. The analysis of customer purchase data helps retailers predict trends and tailor their offerings to meet consumer demand.
Manufacturing
Manufacturers use data analytics to improve production efficiency, predict maintenance needs, and enhance supply chain management. Analyzing machine performance data helps manufacturers reduce downtime and maintain consistent product quality.
Telecommunications
Telecom companies use data analytics to enhance network performance, reduce churn, and provide personalized services. By analyzing usage patterns, they can optimize network resources and improve customer satisfaction.
Modern data analytics use cases
The data analytics landscape is constantly shifting and evolving, with organizations using it to stay ahead of the curve and drive innovation. Today, data analytics has become an indispensable practice, offering solutions that were once unimaginable. As companies strive for competitive advantage, they explore a myriad of modern use cases that demonstrate the transformative potential of analyzing vast amounts of data. These contemporary applications of data analytics include the following:
IoT data analysis
The Internet of Things (IoT) generates massive amounts of data from connected devices. Analyzing this data helps in monitoring device performance, predicting failures, and enhancing user experiences in smart homes and cities.
AI and machine learning integration
Modern data analytics increasingly integrates with AI and machine learning to automate decision-making processes. These technologies analyze vast datasets to provide real-time insights and recommendations.
Real-time analytics
Industries such as finance and retail are adopting real-time analytics to make instantaneous decisions. This involves analyzing streaming data to detect fraud, manage stock levels, and personalize customer interactions on the fly.
Blockchain data analytics
In sectors like finance and supply chain, blockchain analytics is used to verify transactions, track assets, and ensure transparency. Analyzing blockchain data helps in detecting anomalies and ensuring the integrity of transactions.
Comparative insights
Data analytics vs. data science.
While the fields of data analytics and data science certainly overlap, the two are separate disciplines. Picture two skilled artisans at work in different fields, using unique tools and techniques to create something of value from the raw materials they work with. In a similar way, practitioners of data analytics and data science diverge in their methods and goals even while they may share certain foundational elements. Here we highlight the distinct differences between the two.
Data analytics:
- Focus : Primarily concerned with examining datasets to draw conclusions and identify patterns. It focuses on analyzing data to understand past behavior, predict future outcomes, and inform decision-making.
- Tools and techniques : Utilizes statistical analysis, data visualization, and business intelligence tools. Common techniques include data mining, text analytics, and sentiment analysis.
- Objective : To provide actionable insights that help improve business performance, optimize processes, and support decision-making. It’s more application-driven, focusing on specific business questions and problems.
Data science:
- Focus : Encompasses a broader scope, including data analytics, but also involves developing algorithms, building predictive models, and creating new data products. It integrates aspects of computer science, statistics, and domain expertise.
- Tools and techniques : Employs advanced techniques like machine learning, artificial intelligence, and Big Data technologies. Data scientists often use programming languages such as Python and R, along with frameworks like TensorFlow and PyTorch.
- Objective : To uncover new insights through exploratory analysis, create predictive and prescriptive models, and innovate through data-driven solutions. It aims to solve complex problems and generate new questions from data exploration.
Benefits and challenges for data analytics
Benefits of data analytics.
In the face of ever-increasing market complexity, businesses turn to data analytics as a guiding light that can help illuminate the path toward improved performance and success. And as organizations increasingly tap the power and value of data analytics, they’re able to reap a range of benefits, including:
Improved decision-making
Data analytics provides organizations with actionable insights, enabling them to make informed decisions. By analyzing data trends and patterns, businesses can optimize strategies and better anticipate market changes.
Enhanced customer experience
Understanding customer behavior through data analytics helps businesses personalize their services and products. This leads to better customer satisfaction and loyalty, as businesses tailor their offerings to meet specific needs and preferences.
Operational efficiency
Data analytics identifies bottlenecks and inefficiencies within business processes. By addressing these issues, organizations can streamline operations, reduce costs, and increase productivity. The result is a more efficient use of resources and improved profit margins.
Competitive advantage
Data analytics enables businesses to stay ahead of competitors by identifying new market opportunities and trends. This empowers companies to innovate and adapt more quickly to changing market conditions, ensuring they remain competitive in their industry.
Challenges in data analytics
While organizations continue to invest in data analytics due to its clear benefits, certain challenges remain, particularly in the area of data management. Before embarking on your data analytics journey, it is useful to keep in mind some of these challenges so that you’re prepared to overcome them on the path to greater insight and success. These challenges include:
Data quality
Ensuring the accuracy, completeness, and consistency of data is a significant challenge. Poor data quality can lead to incorrect insights and decisions, negatively impacting business outcomes.
Data integration
Combining data from various sources can be complex and time-consuming. Inconsistent formats, structures, and standards across datasets make it difficult to create a unified view, hindering effective analysis.
Data security and privacy
Protecting sensitive data from breaches and ensuring compliance with regulations such as GDPR is critical. Organizations must implement robust security measures and maintain strict data governance to safeguard their data assets.
Scalability
As data volumes grow, managing and analyzing large datasets becomes increasingly challenging. Organizations need scalable solutions that can handle Big Data efficiently without compromising performance or accuracy.
The shortage of skilled data professionals poses a significant hurdle. Businesses often struggle to find and retain talent with the necessary expertise in data analytics, which can slow down their analytics initiatives and impact overall effectiveness.
Future trends in data analytics
The landscape of data analytics is continually evolving, driven by technological advancements and the increasing demand for data-driven insights. Moving forward, the field is expected to be shaped by advancements in artificial intelligence, machine learning, and Big Data technologies.
Meanwhile, organizations will continue to invest in advanced analytical tools and platforms to gain deeper insights from their data and make more informed business decisions. Organizations will increasingly focus on real-time analytics, enabling faster response times to changing market conditions and customer demands. And as data continues to grow exponentially, there will be a greater emphasis on data governance and privacy regulations to ensure that data is securely managed and used ethically.
Finally, the proliferation of generative AI and the Internet of Things (IoT) devices will lead to an increase in the volume and variety of data sources, driving the need for more sophisticated analytics techniques to extract meaningful insights. The future of data analytics is poised to be dynamic and innovative, continually evolving to meet the demands of a data-driven world.
Become a data-driven organization with SAP
The future belongs to data-driven organizations. SAP offers a range of solutions for data analytics, designed to help you and your organization maximize the value of all your data across SAP and third-party systems with an open and multi-cloud architecture. Start your data analytics journey with SAP today.
https://main--builder-prospect-prod--sapudex.hlx.page/fragments/blog/article-details
Maximize your data
The future belongs to data-driven organizations. Start your data analytics journey with SAP today.
https://www.sap.com/resources/what-is-data-fabric
https://www.sap.com/resources/story-beyond-spreadsheet-data-blindness
https://www.sap.com/resources/digital-transformation-strategy-road-map
Tool Induced Biases? Misleading Data Presentation as a Biasing Source in Digital Forensic Analysis
10 Pages Posted: 7 Sep 2024
Daniel Bing Andersen
affiliation not provided to SSRN
Norwegian Police University College
Kyle Porter
Pattern of life analysis has gained ground in the digital forensics field due to the widespread use of smart devices and systems. At the core of pattern of life analysis are the activity-level traces, from which requires expertise to draw valid inferences. Complex tasks also increase the risks of bias and error. The contextual biases have been examined in a digital forensic context, however, the flaws and misinterpretations related to the interplay between the practitioner and the presented data from various software have not been examined through research. This study advances this knowledge, by examining the flaws or misinterpretations that may occur during such interactions in digital forensic casework. Further, the study examines how biases/misinterpretations may be mitigated by traditional quality measures in digital forensics, such as the dual tool approach.
Keywords: Pattern of Life, Activity-Level Traces, Human Factor, Digital Forensics, Digital Investigation, Bias
Suggested Citation: Suggested Citation
affiliation not provided to SSRN ( email )
No Address Available
Norwegian Police University College ( email )
Kyle porter (contact author), do you have a job opening that you would like to promote on ssrn, paper statistics, related ejournals, cybersecurity, privacy, & networks ejournal.
Subscribe to this fee journal for more curated articles on this topic
Forensic Science eJournal
Forensic evidence, criminal procedures & ethics ejournal, digital forensics, instruments & software ejournal, criminalistics ejournal.
2023 EU wide transparency exercise
The transparency exercise is part of the EBA's ongoing efforts to foster transparency and market discipline in the EU financial market, and complements banks' own Pillar 3 disclosures, as laid down in the EU's capital requirements directive (CRD). Along with the dataset, the EBA also provides a wide range of interactive tools that allow users to compare and to visualise data across time and at a country and a bank-by-bank level. In 2023, EBA is going to carry out in autumn an annual Transparency exercise which will disclose capital positions, financial assets, risk exposure amounts, sovereign exposures and asset quality information of the EU banking sector in the second half of 2022 and in the first half of 2023. The exercise is going to be exclusively based on supervisory reporting data, therefore it will not require any additional reporting burden for banks.
The EU-wide Transparency exercise is a pivotal initiative in the European Banking Authority's continuous commitment to fostering transparency and market discipline in the EU financial sector. It complements banks' Pillar 3 disclosures outlined in the EU Capital Requirements Directive (CRD). This year’s disclosure includes detailed bank-by-bank data for reference dates of 30 September 2022, 31 December 2022, 31 March 2023, and 30 June 2023. Information is disclosed for 123 banks across 26 countries in the European Union (EU) and the European Economic Area (EEA), providing information at the highest level of consolidation. The exercise results are derived from supervisory data submitted to the EBA via the European Centralised Infrastructure of Data (EUCLID) platform.
- 2023 EU-wide Transparency exercise and RAR presentation
Transparency exercise documents
- Risk Assessment Report December 2023
- Risk Assessment Report 2023 Annex
- Mapping with COREP/FINREP
- Press release
Interactive tools
Main indicators.
This web tool provides some key figures, for each country and bank-by-bank. |
Key Metrics, Capital, Leverage, P&L, RWAs, Assets and Liabilities
This tool shows individual and countries' data reported in the following templates: Key Metrics, Capital, Leverage, P&L, RWAs, Assets and Liabilities. |
- Credit risk
Data aggregated by countries of banks and Individual banks' data | This tool provides credit risk exposures for a specific country of counterparty (US, DE, ...) broken down by the country of the banks exposed to it (AT, DE,..) for all regulatory portfolios (IRB/SA) and exposure classes (corporates, retail, ...). |
Asset quality and NFC Loans by NACE
Data aggregated by countries of banks and Individual banks' data | This tool shows countries' data, reported in the following templates: non-performing and forborne exposures. Figures are aggregated by country of the bank. The tools allows also to visualise individual banks' data reported under the same templates. |
Data aggregated by countries of banks and Individual banks' data | This tool shows countries' data, reported in the following templates: breakdown of loans and advances to non-financial corporations other than held for trading. Figures are aggregated by country of the bank. The tools allows also to visualise individual banks' data reported under the same templates. |
Sovereign exposures
Data aggregated by countries of banks, by sovereign issuer and Individual banks' data | This tool provides country aggregated figures of sovereign exposures held by all the banks of a specific country (AT, DE, ...) towards each sovereign country (AT, US,..) as well as country aggregated amounts of sovereign exposures issued by a specific country (AT, US,..), broken down by the country of the banks exposed (AT, DE,..). The tools reports also the bank-by-bank sovereign exposures, broken down by the sovereign country issuers (AT, US,..). |
Data refreshed in March 2024. After the publication of the results for EBA’s Transparency exercise, one bank of the sample had to resubmit ITS supervisory data to the EBA. As a result, the full database and the Visual exploration tools have been updated.
Full database
- Market risk
- Sovereign debt exposures
- Other templates
- Data dictionary
- CSV and Tools guide Transparency 2023
Banks individual results
BANK | LEI CODE |
---|---|
529900S9YO2JHTIIDG38 | |
PQOH26KWDF7CG10L6792 | |
9ZHRYM6F437SQJ6OUG95 | |
529900XSTAE561178282 | |
AT0000000000043000VB |
BANK | LEI CODE |
---|---|
A5GWLFH3KM7YV2SFQL84 | |
549300DYPOFMXOR7XM56 | |
5493008QOCP58OLEN998 | |
213800X3Q9LSAKRUWY91 | |
MMYX0N4ZEZ13Z4XCG897 |
BANK | LEI CODE |
---|---|
635400L14KNHZXPUZM19 | |
CXUHEGU3MADZ2CEV7C11 |
BANK | LEI CODE |
---|---|
MAES062Z21O4RZ2U7M96 | |
3M5E1GQGKL17HI6CPN30 | |
LIU16F6VZJSD6UKHD557 |
BANK | LEI CODE |
---|---|
529900JG015JC10LED24 | |
213800RZWHE5EUX9R444 |
BANK | LEI CODE |
---|---|
529900HEKOENJHPNN480 | |
529900ODI3047E2LIV03 | |
7437003B5WFBOIEFY714 |
BANK | LEI CODE |
---|---|
R0MUWSFPU8MPRO8K5P83 | |
549300FH0WJAPEHTIQ77 | |
969500STN7T9MRUMJ267 | |
9695000CG7B84NLR5984 | |
FR9695005MSX1OYEMGDF | |
FR969500TJ5KRTCJQWXH | |
F0HUI1NY1AZMJMD8LP67 | |
96950066U5XAAIRCPA78 | |
96950001WI712W7PQG45 | |
549300HFEHJOXGE4ZE63 | |
O2RNE8IBXP4R0TD8PU41 |
BANK | LEI CODE |
---|---|
254900RNFMDM0P11YR84 | |
VDYMYTQGZZ6DU0912C88 | |
851WYGNLUQLFZBSYGB56 | |
6TJCK1B7E7UTXP528Y04 | |
5299007S3UH5RKUYDA52 | |
7LTWFZYICNSX8D621K86 | |
529900HNOAA1KXQJUQ27 | |
0W2PZJM8XOY22M4GG883 | |
DZZ47B9A52ZJ6LT6VV95 | |
391200EEGLNXBBCVKC73 | |
8IBZUGJ7JPLH368JE346 | |
529900JZTYE3W7WQH904 | |
TUKDD90GPC79G1KOE162 | |
549300ZK53CNGEEI6A29 | |
B81CK4ESI35472RHJ606 | |
DIZES5CFO5K3I5R58746 | |
549300C9KPZR0VZ16R05 | |
529900GM944JT8YIRL63 | |
DSNHHQ2B9X5N6OUJ1236 | |
529900V3O1M5IHMOSF46 | |
5299007QVIQ7IO64NX37 | |
529900GJD3OQLRZCKW37 | |
529900S1KHKOEQL5CK20 |
BANK | LEI CODE |
---|---|
5299009N55YRQC69CN08 | |
JEUVK5RWVJEN8W0C9M24 | |
5UMCZOEYKCVFAW8ZLO05 | |
M6AD1Y1KW32H8THQ6F76 |
BANK | LEI CODE |
---|---|
3H0Q3U74FVFED2SHZT16 | |
529900W3MOO00A18X956 |
BANK | LEI CODE |
---|---|
RIL4VBPDB0M7Z3KXSF19 | |
549300TLZPT6JELDWM92 | |
549300PZMFIQR79Q0T97 |
BANK | LEI CODE |
---|---|
635400AKJBGNS5WNQL34 | |
EQYXK86SF381Q21S3020 | |
635400C8EK6DRI12LJ39 | |
2G5BKIC2CB69PRJH1W31 | |
549300K7L8YW8M215U46 |
BANK | LEI CODE |
---|---|
7LVZJ6XRIE7VNZ4UBX81 | |
J48C8PCSJVUBR8KCW529 | |
815600E4E6DCD2D25E30 | |
N747OI7JINV7RUUH6190 | |
J4CP7MHCXR8DAQMKIL78 | |
815600AD83B2B6317788 | |
LOO0AWXR8GF142JCO404 | |
549300L7YCATGO57ZE10 | |
NNVPP80YIZGEY2314M97 | |
2W8N8UU78PMDQKZENC08 | |
PSNL19R2RXX5U3QWHI44 | |
549300TRUWO2CD2G5692 |
BANK | LEI CODE |
---|---|
2138009Y59EAR7H1UO97 |
Liechtenstein
BANK | LEI CODE |
---|---|
5493009EIBTCB1X12G89 | |
529900OE1FOAM50XLP72 |
BANK | LEI CODE |
---|---|
549300TK038P6EV4YU51 | |
485100FX5Y9YLAQLNP12 |
BANK | LEI CODE |
---|---|
9CZ7TVMR36CYD5TZBS50 | |
R7CQUF1DQM73HUTV1078 | |
KHCL65TP05J1HUW2D560 |
BANK | LEI CODE |
---|---|
529900RWC8ZYB066JF16 | |
213800TC9PZRBHMJW403 |
Netherlands
BANK | LEI CODE |
---|---|
BFXS5XCH7N0Y05NIXW11 | |
529900GGYMNGRQTDOO93 | |
DG3RU1DBUFHT4ZF9WN62 | |
549300NYKK9MWM7GGW15 | |
JLP5FSPH9WPSHY3NIM24 | |
724500A1FNICHSDF2I11 |
BANK | LEI CODE |
---|---|
549300GKFG0RYRRQ1414 | |
549300Q3OIWRHQUQM052 | |
7V6Z97IO7R1SEAO84Q32 |
BANK | LEI CODE |
---|---|
5493000LKS7B3UTF7H35 | |
P4GTT6GF1W40CVIMFR43 |
BANK | LEI CODE |
---|---|
JU1U6S0DG9YLT7N8ZV32 | |
TO822O0VT80V06K0FH57 | |
222100K6QL2V4MLHWQ08 |
BANK | LEI CODE |
---|---|
549300RG3H390KEL8896 |
BANK | LEI CODE |
---|---|
213800HDJ876ACJXXD05 | |
5493001BABFV7P27OW30 |
BANK | LEI CODE |
---|---|
54930056IRBXK0Q1FP96 | |
K8MS7FD7N5Z2WQ51AZ71 | |
5493006QMFDDMYWIAM13 | |
95980020140005881190 | |
SI5RG2M0WQQLZCXKRM20 | |
VWMYAEQSTOPNV0SUGU82 | |
7CUNS533WID6K7DGFI87 | |
549300OLBL49CW8CT155 | |
549300U4LIZV0REEQQ46 | |
5493007SJLLCTM6J6M37 |
BANK | LEI CODE |
---|---|
EV2XZWMLLXF2QRX0CD47 | |
549300C6TUMDXNOVXS82 | |
H0YX5LBGKDVOWCXBZ594 | |
F3JS33DEI6XQ4ZBPTN86 | |
NHBDILHZTYCNBV5UYZ31 | |
M312WZV08Y7LYUC71685 |
BANK | LEI CODE |
---|---|
xxxxxxxxxxxxxxxxxxxx |
More From Forbes
The richest cities in new hampshire as revealed by latest census data.
- Share to Facebook
- Share to Twitter
- Share to Linkedin
Hanover, New Hampshire, is home to Dartmouth University, and is among the top five richest cities in ... [+] New Hampshire.
In recent studies, we’ve analyzed and examined the richest cities in Deep South states, such as Mississippi , Georgia , and Alabama . Here, we’re going to take a look at another region — New England. And the first state to be analyzed is New Hampshire.
The median household income in New Hampshire is quite high, at $90,485 per year. By comparison, the median household income for the United States overall is $75,149. Depending on the city, however, incomes in New Hampshire can be much higher.
Read on and find out what the richest city in New Hampshire is, as well as the top 20 wealthiest cities in the state.
What Is the Richest City in New Hampshire?
To define the “richest city in New Hampshire,” we sourced data from the Census Bureau’s 2022 American Community Survey 5-Year Estimates (which is the latest data available), including median household income, mean household income, median home value, and median property taxes paid, and then analyzed and scored each one of these factors, assembling a list of the top 20 richest cities in New Hampshire.
In order to fully grasp the figures, here are some important notes about Census data. The Census Bureau measures median household incomes up to $250,000, with households earning more being denoted as “$250,000+”. Similarly, the Census does this with median home value, which goes up to $2 million, with everything above that being expressed as “$2,000,000+”. Lastly, the same limits apply to measuring median property taxes paid, which go up to $10,000 and any amount above that is denoted as “$10,000+”.
Google Is Deleting Gmail Accounts—3 Steps Needed To Keep Yours
Used tesla cybertruck price continues to crash, new and dangerous android attack warning issued.
This is what makes mean household income particularly useful in this analysis of the wealthiest cities in New Hampshire. Unlike median household income, the Census Bureau provides mean (or average) household income with exact values for every city in New Hampshire. All four of these metrics were scored, added up, and then ranked by the cities’ combined scores.
Below you’ll find a table detailing the top 20 richest cities in New Hampshire and their respective dollar figures for each metric:
Amherst, New Hampshire
Based on this four-factor metric of median household income, average household income, median home value, and median property taxes paid, the richest city in New Hampshire is Amherst. This city consists of only 253 households, and is northwest of Nashua. Located in Hillsborough County, Amherst is a wealthy city whose population works in some interesting fields. For instance, more than a fifth (21%) of the workforce in Amherst works in Architecture & Engineering Occupations, according to DataUSA . Another 11.1% work in Education Instruction & Library Occupations.
Amherst has the second highest median household income in New Hampshire (of the cities that had complete Census data). Its median household income is $144,940, which is almost double the national figure. Equally significantly, the average household income in Amherst is the highest in the state, at $304,728, implying that a minority of top-earners pull the mean income figure upward, away from the median. According to Zillow , the median home value in Amherst is over $630,000, while the median property taxes households pay in Amherst exceeds $10,000 a year.
Hanover, New Hampshire
The No. 2 richest city in New Hampshire is small (in the grand scheme of things), but much larger than No. 1 Amherst, having approximately 1,971 total households versus Amherst’s 253 households. Hanover lies along the Vermont border, east of Interstate 91. Hanover is home to Dartmouth College, the U.S. Army Corps of Engineers Cold Regions Research and Engineering Laboratory, and Hanover High School. Not surprisingly, its occupational breakdown fits that of a college town: Educational Services account for 42.3% of the workforce, Health Care & Social Assistance accounts for 22.8%, and Professional, Scientific, & Technical Services account for 10.2%, according to DataUSA .
The median household income in Hanover is $111,958, but its average household income is more than $80,000 higher, at $193,451. Home values in Hanover are high, north of $965,7000 according to Zillow . It’s, therefore, not surprising that the median property taxes paid per year in Hanover are in excess of $10,000 per household.
Newfields, New Hampshire
No. 3 Newfields is much smaller than the No. 2 richest city, and actually less than half the size of the already-small Amherst. Newfields has an estimated 107 total households. Located in the part of New Hampshire that forms a wedge between Massachusetts and Maine, Newfields boasts the highest median household income in New Hampshire, at $173,295 per year. Interestingly, its average household income is actually lower, at $158,428. The price of homes in Newfields has escalated, as it has pretty much everywhere. According to Zillow , the current median home value in Newfields is $796,168. Not coincidentally, the median property taxes paid by homeowners is well over $9,000 a year.
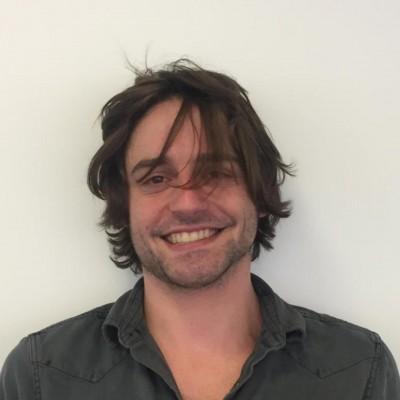
- Editorial Standards
- Reprints & Permissions
Join The Conversation
One Community. Many Voices. Create a free account to share your thoughts.
Forbes Community Guidelines
Our community is about connecting people through open and thoughtful conversations. We want our readers to share their views and exchange ideas and facts in a safe space.
In order to do so, please follow the posting rules in our site's Terms of Service. We've summarized some of those key rules below. Simply put, keep it civil.
Your post will be rejected if we notice that it seems to contain:
- False or intentionally out-of-context or misleading information
- Insults, profanity, incoherent, obscene or inflammatory language or threats of any kind
- Attacks on the identity of other commenters or the article's author
- Content that otherwise violates our site's terms.
User accounts will be blocked if we notice or believe that users are engaged in:
- Continuous attempts to re-post comments that have been previously moderated/rejected
- Racist, sexist, homophobic or other discriminatory comments
- Attempts or tactics that put the site security at risk
- Actions that otherwise violate our site's terms.
So, how can you be a power user?
- Stay on topic and share your insights
- Feel free to be clear and thoughtful to get your point across
- ‘Like’ or ‘Dislike’ to show your point of view.
- Protect your community.
- Use the report tool to alert us when someone breaks the rules.
Thanks for reading our community guidelines. Please read the full list of posting rules found in our site's Terms of Service.

An official website of the United States government
Here's how you know
Official websites use .gov A .gov website belongs to an official government organization in the United States.
Secure .gov websites use HTTPS A lock ( Lock Locked padlock ) or https:// means you’ve safely connected to the .gov website. Share sensitive information only on official, secure websites.

News Release FHFA Releases Mortgage Loan and Natural Disaster Dashboard
Washington, D.C. – The Federal Housing Finance Agency (FHFA) released an online risk analysis tool that provides geographic estimates for physical risks from various types of natural disasters as well as nationwide data on housing and the mortgage market.
The tool — known as the Mortgage Loan and Natural Disaster Dashboard — is intended to give property owners, community leaders, financial institutions, policymakers, and other stakeholders better insight into which areas of the country are most likely to incur greater damages from hurricanes, flooding, wildfires, and other types of natural hazards.
Users can combine FHFA’s Public Use Database (PUDB) with data on previous disasters and other analysis from the Federal Emergency Management Agency (FEMA). They can identify areas of the country with elevated disaster risk based on several factors, and which of those areas have concentrations of properties financed with loans acquired by Fannie Mae, Freddie Mac, and the Federal Home Loan Banks.
Scientists widely attribute more intense storm seasons — along with higher human and financial costs — in recent years to climate risks. Tools such as FHFA’s new dashboard provide stakeholders with greater visibility of communities already facing economic challenges that are susceptible to natural disasters.
“Climate risks, especially natural disasters, pose a serious threat to housing and other critical infrastructure, particularly in vulnerable communities,” said FHFA Director Sandra L. Thompson. “Providing geographic information on disasters as well as concentrated exposures of loans acquired by our regulated entities can help policymakers and the industry develop solutions to better safeguard those communities from the impact of future catastrophes.”
The dashboard utilizes data from three publicly available sources. The PUDB provides a geographic breakdown of loans acquired by FHFA’s regulated entities. FEMA’s National Risk Index identifies communities most at risk for 18 types of natural hazards. The third source, FHFA’s Duty to Serve High-Needs Rural Areas data , pinpoints rural areas in the country that are characterized by a high concentration of poverty and substandard housing conditions.
The data on mortgages were updated as of 2022 and the data on past natural disasters reflected in the online tool were updated as of 2023, while the Census tracts were drawn from the 2020 U.S. Census. Dashboard users can view nationwide mortgage data at the Census-tract level overlaid with expected annual damages for 18 different types of natural disasters.
Additional layers measure other factors affecting an area’s susceptibility to certain disasters, including a social vulnerability index, a community resilience index, and an additional layer enabling users to analyze FHFA’s Duty to Serve High-Needs Rural areas.
FHFA released this dashboard along with a blog post and answers to frequently asked questions (FAQs), outlining potential uses of the new data tool and how disaster assistance resources developed by federal agencies and the Enterprises can assist homeowners affected by natural disasters.
Related Resources:
Mortgage Loan and Natural Disaster Dashboard
FHFA Mortgage Loan and Natural Disaster Dashboard Blog: A Case Study of Hurricane Beryl
Mortgage Loan and Natural Disaster Dashboard Instructions and FAQs
The Federal Housing Finance Agency regulates Fannie Mae, Freddie Mac, and the 11 Federal Home Loan Banks. These government-sponsored enterprises provide more than $8.4 trillion in funding for the U.S. mortgage markets and financial institutions. Additional information is available at www.FHFA.gov , on Twitter @FHFA , YouTube , Facebook, and LinkedIn .
Contacts: MediaInq[email protected]

COMMENTS
Understanding Data Presentations (Guide Examples)
What is Data Analysis? An Expert Guide With Examples
Data Presentation - Types & Its Importance
Data Presentation: A Comprehensive Guide
What Is Data Analysis? (With Examples)
See a slide-by-slide example of a presentation deck for a data analytics report. Connor, a Marketing Analytics Manager at Google Cloud, walks you through exa...
Key Objectives of Data Presentation. Here are some key objectives to think about when presenting financial analysis: Visual communication. Audience and context. Charts, graphs, and images. Focus on important points. Design principles. Storytelling. Persuasiveness.
Present Your Data Like a Pro
Storytelling with data is a highly valued skill in the workforce today and translating data and insights for a non-technical audience is rare to see than it is expected. Here's my five-step routine to make and deliver your data presentation right where it is intended —. 1. Understand Your Data & Make It Seen.
What Is Data Presentation? (Definition, Types And How-To)
Data Analytics: Definition, Uses, Examples, and More
10 Data Presentation Examples For Strategic Communication
A Step-by-Step Guide to the Data Analysis Process [2024]
A data-driven approach encourages the exploration of new ideas and the creation of new products, services, and business models—ultimately driving growth and progress. Boost security and minimize risks: When it comes to managing risks, data analysis is a reliable and valuable tool to have on your side. You can spot potential threats and craft ...
Overview. Data visualization is the visual presentation of data or information. The goal of data visualization is to communicate data or information clearly and effectively to readers. Typically, data is visualized in the form of a chart, infographic, diagram, or map. The field of data visualization combines both art and data science.
What Is Data Visualization? Definition & Examples
What Is Data Analysis? (With Examples)
analysis to use on a set of data and the relevant forms of pictorial presentation or data display. The decision is based on the scale of measurement of the data. These scales are nominal, ordinal and numerical. Nominal scale A nominal scale is where: the data can be classified into a non-numerical or named categories, and
Data Analysis and Presentation Skills: the PwC Approach ...
Abstract. This chapter covers the topics of data collection, data presentation and data analysis. It gives attention to data collection for studies based on experiments, on data derived from existing published or unpublished data sets, on observation, on simulation and digital twins, on surveys, on interviews and on focus group discussions.
Data analytics is the process of analyzing, interpreting, and visualizing large, complex datasets to derive meaningful insights and make informed decisions. It encompasses a variety of techniques from statistical analysis to machine learning, aimed at analyzing past and current data to predict future trends. Why is data analytics important?
Pattern of life analysis has gained ground in the digital forensics field due to the widespread use of smart devices and systems. At the core of pattern of life. ... Misleading Data Presentation as a Biasing Source in Digital Forensic Analysis. 10 Pages Posted: 7 Sep 2024. See all articles by Daniel Bing Andersen
1 Introduction. Irritable Bowel Syndrome (IBS) is one of the most common disorders of gut-brain interaction and one that considerably reduces quality of life, [] affecting roughly 11% of the global population. [] IBS is classified into three major clinical subtypes: IBS with predominant constipation (IBS-C), IBS predominantly with diarrhea (IBS-D), and mixed IBS (IBS-M). []
The tools allows also to visualise individual banks' data reported under the same templates. NACE. Data aggregated by countries of banks and Individual banks' data. This tool shows countries' data, reported in the following templates: breakdown of loans and advances to non-financial corporations other than held for trading.
Data Visualization: Definition, Benefits, and Examples
To define the "richest city in New Hampshire," we sourced data from the Census Bureau's 2022 American Community Survey 5-Year Estimates (which is the latest data available), including median ...
The Federal Housing Finance Agency (FHFA) released an online risk analysis tool that provides geographic estimates for physical risks from various types of natural disasters as well as nationwide data on housing and the mortgage market. The tool — known as the Mortgage Loan and Natural Disaster Dashboard— is intended to give property owners, community leaders, financial institutions ...