

Directional Hypothesis: Definition and 10 Examples
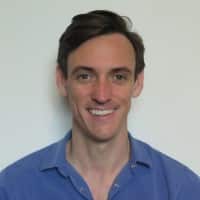
Chris Drew (PhD)
Dr. Chris Drew is the founder of the Helpful Professor. He holds a PhD in education and has published over 20 articles in scholarly journals. He is the former editor of the Journal of Learning Development in Higher Education. [Image Descriptor: Photo of Chris]
Learn about our Editorial Process
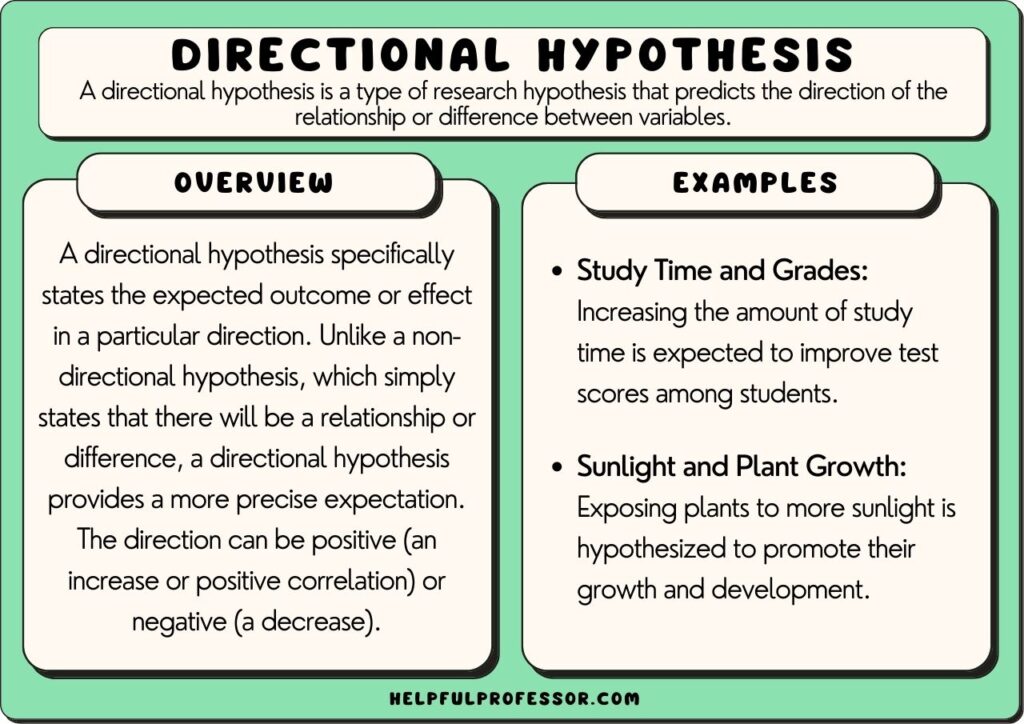
A directional hypothesis refers to a type of hypothesis used in statistical testing that predicts a particular direction of the expected relationship between two variables.
In simpler terms, a directional hypothesis is an educated, specific guess about the direction of an outcome—whether an increase, decrease, or a proclaimed difference in variable sets.
For example, in a study investigating the effects of sleep deprivation on cognitive performance, a directional hypothesis might state that as sleep deprivation (Independent Variable) increases, cognitive performance (Dependent Variable) decreases (Killgore, 2010). Such a hypothesis offers a clear, directional relationship whereby a specific increase or decrease is anticipated.
Global warming provides another notable example of a directional hypothesis. A researcher might hypothesize that as carbon dioxide (CO2) levels increase, global temperatures also increase (Thompson, 2010). In this instance, the hypothesis clearly articulates an upward trend for both variables.
In any given circumstance, it’s imperative that a directional hypothesis is grounded on solid evidence. For instance, the CO2 and global temperature relationship is based on substantial scientific evidence, and not on a random guess or mere speculation (Florides & Christodoulides, 2009).
Directional vs Non-Directional vs Null Hypotheses
A directional hypothesis is generally contrasted to a non-directional hypothesis. Here’s how they compare:
- Directional hypothesis: A directional hypothesis provides a perspective of the expected relationship between variables, predicting the direction of that relationship (either positive, negative, or a specific difference).
- Non-directional hypothesis: A non-directional hypothesis denotes the possibility of a relationship between two variables ( the independent and dependent variables ), although this hypothesis does not venture a prediction as to the direction of this relationship (Ali & Bhaskar, 2016). For example, a non-directional hypothesis might state that there exists a relationship between a person’s diet (independent variable) and their mood (dependent variable), without indicating whether improvement in diet enhances mood positively or negatively. Overall, the choice between a directional or non-directional hypothesis depends on the known or anticipated link between the variables under consideration in research studies.
Another very important type of hypothesis that we need to know about is a null hypothesis :
- Null hypothesis : The null hypothesis stands as a universality—the hypothesis that there is no observed effect in the population under study, meaning there is no association between variables (or that the differences are down to chance). For instance, a null hypothesis could be constructed around the idea that changing diet (independent variable) has no discernible effect on a person’s mood (dependent variable) (Yan & Su, 2016). This proposition is the one that we aim to disprove in an experiment.
While directional and non-directional hypotheses involve some integrated expectations about the outcomes (either distinct direction or a vague relationship), a null hypothesis operates on the premise of negating such relationships or effects.
The null hypotheses is typically proposed to be negated or disproved by statistical tests, paving way for the acceptance of an alternate hypothesis (either directional or non-directional).
Directional Hypothesis Examples
1. exercise and heart health.
Research suggests that as regular physical exercise (independent variable) increases, the risk of heart disease (dependent variable) decreases (Jakicic, Davis, Rogers, King, Marcus, Helsel, Rickman, Wahed, Belle, 2016). In this example, a directional hypothesis anticipates that the more individuals maintain routine workouts, the lesser would be their odds of developing heart-related disorders. This assumption is based on the underlying fact that routine exercise can help reduce harmful cholesterol levels, regulate blood pressure, and bring about overall health benefits. Thus, a direction – a decrease in heart disease – is expected in relation with an increase in exercise.
2. Screen Time and Sleep Quality
Another classic instance of a directional hypothesis can be seen in the relationship between the independent variable, screen time (especially before bed), and the dependent variable, sleep quality. This hypothesis predicts that as screen time before bed increases, sleep quality decreases (Chang, Aeschbach, Duffy, Czeisler, 2015). The reasoning behind this hypothesis is the disruptive effect of artificial light (especially blue light from screens) on melatonin production, a hormone needed to regulate sleep. As individuals spend more time exposed to screens before bed, it is predictably hypothesized that their sleep quality worsens.
3. Job Satisfaction and Employee Turnover
A typical scenario in organizational behavior research posits that as job satisfaction (independent variable) increases, the rate of employee turnover (dependent variable) decreases (Cheng, Jiang, & Riley, 2017). This directional hypothesis emphasizes that an increased level of job satisfaction would lead to a reduced rate of employees leaving the company. The theoretical basis for this hypothesis is that satisfied employees often tend to be more committed to the organization and are less likely to seek employment elsewhere, thus reducing turnover rates.
4. Healthy Eating and Body Weight
Healthy eating, as the independent variable, is commonly thought to influence body weight, the dependent variable, in a positive way. For example, the hypothesis might state that as consumption of healthy foods increases, an individual’s body weight decreases (Framson, Kristal, Schenk, Littman, Zeliadt, & Benitez, 2009). This projection is based on the premise that healthier foods, such as fruits and vegetables, are generally lower in calories than junk food, assisting in weight management.
5. Sun Exposure and Skin Health
The association between sun exposure (independent variable) and skin health (dependent variable) allows for a definitive hypothesis declaring that as sun exposure increases, the risk of skin damage or skin cancer increases (Whiteman, Whiteman, & Green, 2001). The premise aligns with the understanding that overexposure to the sun’s ultraviolet rays can deteriorate skin health, leading to conditions like sunburn or, in extreme cases, skin cancer.
6. Study Hours and Academic Performance
A regularly assessed relationship in academia suggests that as the number of study hours (independent variable) rises, so too does academic performance (dependent variable) (Nonis, Hudson, Logan, Ford, 2013). The hypothesis proposes a positive correlation , with an increase in study time expected to contribute to enhanced academic outcomes.
7. Screen Time and Eye Strain
It’s commonly hypothesized that as screen time (independent variable) increases, the likelihood of experiencing eye strain (dependent variable) also increases (Sheppard & Wolffsohn, 2018). This is based on the idea that prolonged engagement with digital screens—computers, tablets, or mobile phones—can cause discomfort or fatigue in the eyes, attributing to symptoms of eye strain.
8. Physical Activity and Stress Levels
In the sphere of mental health, it’s often proposed that as physical activity (independent variable) increases, levels of stress (dependent variable) decrease (Stonerock, Hoffman, Smith, Blumenthal, 2015). Regular exercise is known to stimulate the production of endorphins, the body’s natural mood elevators, helping to alleviate stress.
9. Water Consumption and Kidney Health
A common health-related hypothesis might predict that as water consumption (independent variable) increases, the risk of kidney stones (dependent variable) decreases (Curhan, Willett, Knight, & Stampfer, 2004). Here, an increase in water intake is inferred to reduce the risk of kidney stones by diluting the substances that lead to stone formation.
10. Traffic Noise and Sleep Quality
In urban planning research, it’s often supposed that as traffic noise (independent variable) increases, sleep quality (dependent variable) decreases (Muzet, 2007). Increased noise levels, particularly during the night, can result in sleep disruptions, thus, leading to poor sleep quality.
11. Sugar Consumption and Dental Health
In the field of dental health, an example might be stating as one’s sugar consumption (independent variable) increases, dental health (dependent variable) decreases (Sheiham, & James, 2014). This stems from the fact that sugar is a major factor in tooth decay, and increased consumption of sugary foods or drinks leads to a decline in dental health due to the high likelihood of cavities.
See 15 More Examples of Hypotheses Here
A directional hypothesis plays a critical role in research, paving the way for specific predicted outcomes based on the relationship between two variables. These hypotheses clearly illuminate the expected direction—the increase or decrease—of an effect. From predicting the impacts of healthy eating on body weight to forecasting the influence of screen time on sleep quality, directional hypotheses allow for targeted and strategic examination of phenomena. In essence, directional hypotheses provide the crucial path for inquiry, shaping the trajectory of research studies and ultimately aiding in the generation of insightful, relevant findings.
Ali, S., & Bhaskar, S. (2016). Basic statistical tools in research and data analysis. Indian Journal of Anaesthesia, 60 (9), 662-669. doi: https://doi.org/10.4103%2F0019-5049.190623
Chang, A. M., Aeschbach, D., Duffy, J. F., & Czeisler, C. A. (2015). Evening use of light-emitting eReaders negatively affects sleep, circadian timing, and next-morning alertness. Proceeding of the National Academy of Sciences, 112 (4), 1232-1237. doi: https://doi.org/10.1073/pnas.1418490112
Cheng, G. H. L., Jiang, D., & Riley, J. H. (2017). Organizational commitment and intrinsic motivation of regular and contractual primary school teachers in China. New Psychology, 19 (3), 316-326. Doi: https://doi.org/10.4103%2F2249-4863.184631
Curhan, G. C., Willett, W. C., Knight, E. L., & Stampfer, M. J. (2004). Dietary factors and the risk of incident kidney stones in younger women: Nurses’ Health Study II. Archives of Internal Medicine, 164 (8), 885–891.
Florides, G. A., & Christodoulides, P. (2009). Global warming and carbon dioxide through sciences. Environment international , 35 (2), 390-401. doi: https://doi.org/10.1016/j.envint.2008.07.007
Framson, C., Kristal, A. R., Schenk, J. M., Littman, A. J., Zeliadt, S., & Benitez, D. (2009). Development and validation of the mindful eating questionnaire. Journal of the American Dietetic Association, 109 (8), 1439-1444. doi: https://doi.org/10.1016/j.jada.2009.05.006
Jakicic, J. M., Davis, K. K., Rogers, R. J., King, W. C., Marcus, M. D., Helsel, D., … & Belle, S. H. (2016). Effect of wearable technology combined with a lifestyle intervention on long-term weight loss: The IDEA randomized clinical trial. JAMA, 316 (11), 1161-1171.
Khan, S., & Iqbal, N. (2013). Study of the relationship between study habits and academic achievement of students: A case of SPSS model. Higher Education Studies, 3 (1), 14-26.
Killgore, W. D. (2010). Effects of sleep deprivation on cognition. Progress in brain research , 185 , 105-129. doi: https://doi.org/10.1016/B978-0-444-53702-7.00007-5
Marczinski, C. A., & Fillmore, M. T. (2014). Dissociative antagonistic effects of caffeine on alcohol-induced impairment of behavioral control. Experimental and Clinical Psychopharmacology, 22 (4), 298–311. doi: https://psycnet.apa.org/doi/10.1037/1064-1297.11.3.228
Muzet, A. (2007). Environmental Noise, Sleep and Health. Sleep Medicine Reviews, 11 (2), 135-142. doi: https://doi.org/10.1016/j.smrv.2006.09.001
Nonis, S. A., Hudson, G. I., Logan, L. B., & Ford, C. W. (2013). Influence of perceived control over time on college students’ stress and stress-related outcomes. Research in Higher Education, 54 (5), 536-552. doi: https://doi.org/10.1023/A:1018753706925
Sheiham, A., & James, W. P. (2014). A new understanding of the relationship between sugars, dental caries and fluoride use: implications for limits on sugars consumption. Public health nutrition, 17 (10), 2176-2184. Doi: https://doi.org/10.1017/S136898001400113X
Sheppard, A. L., & Wolffsohn, J. S. (2018). Digital eye strain: prevalence, measurement and amelioration. BMJ open ophthalmology , 3 (1), e000146. doi: http://dx.doi.org/10.1136/bmjophth-2018-000146
Stonerock, G. L., Hoffman, B. M., Smith, P. J., & Blumenthal, J. A. (2015). Exercise as Treatment for Anxiety: Systematic Review and Analysis. Annals of Behavioral Medicine, 49 (4), 542–556. doi: https://doi.org/10.1007/s12160-014-9685-9
Thompson, L. G. (2010). Climate change: The evidence and our options. The Behavior Analyst , 33 , 153-170. Doi: https://doi.org/10.1007/BF03392211
Whiteman, D. C., Whiteman, C. A., & Green, A. C. (2001). Childhood sun exposure as a risk factor for melanoma: a systematic review of epidemiologic studies. Cancer Causes & Control, 12 (1), 69-82. doi: https://doi.org/10.1023/A:1008980919928
Yan, X., & Su, X. (2009). Linear regression analysis: theory and computing . New Jersey: World Scientific.
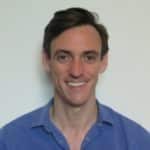
- Chris Drew (PhD) https://helpfulprofessor.com/author/chris-drew-phd-2/ 101 Hidden Talents Examples
- Chris Drew (PhD) https://helpfulprofessor.com/author/chris-drew-phd-2/ 15 Green Flags in a Relationship
- Chris Drew (PhD) https://helpfulprofessor.com/author/chris-drew-phd-2/ 15 Signs you're Burnt Out, Not Lazy
- Chris Drew (PhD) https://helpfulprofessor.com/author/chris-drew-phd-2/ 15 Toxic Things Parents Say to their Children
Leave a Comment Cancel Reply
Your email address will not be published. Required fields are marked *
- Skip to secondary menu
- Skip to main content
- Skip to primary sidebar
Statistics By Jim
Making statistics intuitive
One-Tailed and Two-Tailed Hypothesis Tests Explained
By Jim Frost 61 Comments
Choosing whether to perform a one-tailed or a two-tailed hypothesis test is one of the methodology decisions you might need to make for your statistical analysis. This choice can have critical implications for the types of effects it can detect, the statistical power of the test, and potential errors.
In this post, you’ll learn about the differences between one-tailed and two-tailed hypothesis tests and their advantages and disadvantages. I include examples of both types of statistical tests. In my next post, I cover the decision between one and two-tailed tests in more detail.
What Are Tails in a Hypothesis Test?
First, we need to cover some background material to understand the tails in a test. Typically, hypothesis tests take all of the sample data and convert it to a single value, which is known as a test statistic. You’re probably already familiar with some test statistics. For example, t-tests calculate t-values . F-tests, such as ANOVA, generate F-values . The chi-square test of independence and some distribution tests produce chi-square values. All of these values are test statistics. For more information, read my post about Test Statistics .
These test statistics follow a sampling distribution. Probability distribution plots display the probabilities of obtaining test statistic values when the null hypothesis is correct. On a probability distribution plot, the portion of the shaded area under the curve represents the probability that a value will fall within that range.
The graph below displays a sampling distribution for t-values. The two shaded regions cover the two-tails of the distribution.

Keep in mind that this t-distribution assumes that the null hypothesis is correct for the population. Consequently, the peak (most likely value) of the distribution occurs at t=0, which represents the null hypothesis in a t-test. Typically, the null hypothesis states that there is no effect. As t-values move further away from zero, it represents larger effect sizes. When the null hypothesis is true for the population, obtaining samples that exhibit a large apparent effect becomes less likely, which is why the probabilities taper off for t-values further from zero.
Related posts : How t-Tests Work and Understanding Probability Distributions
Critical Regions in a Hypothesis Test
In hypothesis tests, critical regions are ranges of the distributions where the values represent statistically significant results. Analysts define the size and location of the critical regions by specifying both the significance level (alpha) and whether the test is one-tailed or two-tailed.
Consider the following two facts:
- The significance level is the probability of rejecting a null hypothesis that is correct.
- The sampling distribution for a test statistic assumes that the null hypothesis is correct.
Consequently, to represent the critical regions on the distribution for a test statistic, you merely shade the appropriate percentage of the distribution. For the common significance level of 0.05, you shade 5% of the distribution.
Related posts : Significance Levels and P-values and T-Distribution Table of Critical Values
Two-Tailed Hypothesis Tests
Two-tailed hypothesis tests are also known as nondirectional and two-sided tests because you can test for effects in both directions. When you perform a two-tailed test, you split the significance level percentage between both tails of the distribution. In the example below, I use an alpha of 5% and the distribution has two shaded regions of 2.5% (2 * 2.5% = 5%).
When a test statistic falls in either critical region, your sample data are sufficiently incompatible with the null hypothesis that you can reject it for the population.
In a two-tailed test, the generic null and alternative hypotheses are the following:
- Null : The effect equals zero.
- Alternative : The effect does not equal zero.
The specifics of the hypotheses depend on the type of test you perform because you might be assessing means, proportions, or rates.
Example of a two-tailed 1-sample t-test
Suppose we perform a two-sided 1-sample t-test where we compare the mean strength (4.1) of parts from a supplier to a target value (5). We use a two-tailed test because we care whether the mean is greater than or less than the target value.
To interpret the results, simply compare the p-value to your significance level. If the p-value is less than the significance level, you know that the test statistic fell into one of the critical regions, but which one? Just look at the estimated effect. In the output below, the t-value is negative, so we know that the test statistic fell in the critical region in the left tail of the distribution, indicating the mean is less than the target value. Now we know this difference is statistically significant.

We can conclude that the population mean for part strength is less than the target value. However, the test had the capacity to detect a positive difference as well. You can also assess the confidence interval. With a two-tailed hypothesis test, you’ll obtain a two-sided confidence interval. The confidence interval tells us that the population mean is likely to fall between 3.372 and 4.828. This range excludes the target value (5), which is another indicator of significance.
Advantages of two-tailed hypothesis tests
You can detect both positive and negative effects. Two-tailed tests are standard in scientific research where discovering any type of effect is usually of interest to researchers.
One-Tailed Hypothesis Tests
One-tailed hypothesis tests are also known as directional and one-sided tests because you can test for effects in only one direction. When you perform a one-tailed test, the entire significance level percentage goes into the extreme end of one tail of the distribution.
In the examples below, I use an alpha of 5%. Each distribution has one shaded region of 5%. When you perform a one-tailed test, you must determine whether the critical region is in the left tail or the right tail. The test can detect an effect only in the direction that has the critical region. It has absolutely no capacity to detect an effect in the other direction.
In a one-tailed test, you have two options for the null and alternative hypotheses, which corresponds to where you place the critical region.
You can choose either of the following sets of generic hypotheses:
- Null : The effect is less than or equal to zero.
- Alternative : The effect is greater than zero.

- Null : The effect is greater than or equal to zero.
- Alternative : The effect is less than zero.

Again, the specifics of the hypotheses depend on the type of test you perform.
Notice how for both possible null hypotheses the tests can’t distinguish between zero and an effect in a particular direction. For example, in the example directly above, the null combines “the effect is greater than or equal to zero” into a single category. That test can’t differentiate between zero and greater than zero.
Example of a one-tailed 1-sample t-test
Suppose we perform a one-tailed 1-sample t-test. We’ll use a similar scenario as before where we compare the mean strength of parts from a supplier (102) to a target value (100). Imagine that we are considering a new parts supplier. We will use them only if the mean strength of their parts is greater than our target value. There is no need for us to differentiate between whether their parts are equally strong or less strong than the target value—either way we’d just stick with our current supplier.
Consequently, we’ll choose the alternative hypothesis that states the mean difference is greater than zero (Population mean – Target value > 0). The null hypothesis states that the difference between the population mean and target value is less than or equal to zero.

To interpret the results, compare the p-value to your significance level. If the p-value is less than the significance level, you know that the test statistic fell into the critical region. For this study, the statistically significant result supports the notion that the population mean is greater than the target value of 100.
Confidence intervals for a one-tailed test are similarly one-sided. You’ll obtain either an upper bound or a lower bound. In this case, we get a lower bound, which indicates that the population mean is likely to be greater than or equal to 100.631. There is no upper limit to this range.
A lower-bound matches our goal of determining whether the new parts are stronger than our target value. The fact that the lower bound (100.631) is higher than the target value (100) indicates that these results are statistically significant.
This test is unable to detect a negative difference even when the sample mean represents a very negative effect.
Advantages and disadvantages of one-tailed hypothesis tests
One-tailed tests have more statistical power to detect an effect in one direction than a two-tailed test with the same design and significance level. One-tailed tests occur most frequently for studies where one of the following is true:
- Effects can exist in only one direction.
- Effects can exist in both directions but the researchers only care about an effect in one direction. There is no drawback to failing to detect an effect in the other direction. (Not recommended.)
The disadvantage of one-tailed tests is that they have no statistical power to detect an effect in the other direction.
As part of your pre-study planning process, determine whether you’ll use the one- or two-tailed version of a hypothesis test. To learn more about this planning process, read 5 Steps for Conducting Scientific Studies with Statistical Analyses .
This post explains the differences between one-tailed and two-tailed statistical hypothesis tests. How these forms of hypothesis tests function is clear and based on mathematics. However, there is some debate about when you can use one-tailed tests. My next post explores this decision in much more depth and explains the different schools of thought and my opinion on the matter— When Can I Use One-Tailed Hypothesis Tests .
If you’re learning about hypothesis testing and like the approach I use in my blog, check out my Hypothesis Testing book! You can find it at Amazon and other retailers.

Share this:

Reader Interactions
August 23, 2024 at 1:28 pm
Thank so much. This is very helpfull
June 26, 2022 at 12:14 pm
Hi, Can help me with figuring out the null and alternative hypothesis of the following statement? Some claimed that the real average expenditure on beverage by general people is at least $10.
February 19, 2022 at 6:02 am
thank you for the thoroughly explanation, I’m still strugling to wrap my mind around the t-table and the relation between the alpha values for one or two tail probability and the confidence levels on the bottom (I’m understanding it so wrongly that for me it should be the oposite, like one tail 0,05 should correspond 95% CI and two tailed 0,025 should correspond to 95% because then you got the 2,5% on each side). In my mind if I picture the one tail diagram with an alpha of 0,05 I see the rest 95% inside the diagram, but for a one tail I only see 90% CI paired with a 5% alpha… where did the other 5% go? I tried to understand when you said we should just double the alpha for a one tail probability in order to find the CI but I still cant picture it. I have been trying to understand this. Like if you only have one tail and there is 0,05, shouldn’t the rest be on the other side? why is it then 90%… I know I’m missing a point and I can’t figure it out and it’s so frustrating…
February 23, 2022 at 10:01 pm
The alpha is the total shaded area. So, if the alpha = 0.05, you know that 5% of the distribution is shaded. The number of tails tells you how to divide the shaded areas. Is it all in one region (1-tailed) or do you split the shaded regions in two (2-tailed)?
So, for a one-tailed test with an alpha of 0.05, the 5% shading is all in one tail. If alpha = 0.10, then it’s 10% on one side. If it’s two-tailed, then you need to split that 10% into two–5% in both tails. Hence, the 5% in a one-tailed test is the same as a two-tailed test with an alpha of 0.10 because that test has the same 5% on one side (but there’s another 5% in the other tail).
It’s similar for CIs. However, for CIs, you shade the middle rather than the extremities. I write about that in one my articles about hypothesis testing and confidence intervals .
I’m not sure if I’m answering your question or not.
February 17, 2022 at 1:46 pm
I ran a post hoc Dunnett’s test alpha=0.05 after a significant Anova test in Proc Mixed using SAS. I want to determine if the means for treatment (t1, t2, t3) is significantly less than the means for control (p=pathogen). The code for the dunnett’s test is – LSmeans trt / diff=controll (‘P’) adjust=dunnett CL plot=control; I think the lower bound one tailed test is the correct test to run but I’m not 100% sure. I’m finding conflicting information online. In the output table for the dunnett’s test the mean difference between the control and the treatments is t1=9.8, t2=64.2, and t3=56.5. The control mean estimate is 90.5. The adjusted p-value by treatment is t1(p=0.5734), t2 (p=.0154) and t3(p=.0245). The adjusted lower bound confidence limit in order from t1-t3 is -38.8, 13.4, and 7.9. The adjusted upper bound for all test is infinity. The graphical output for the dunnett’s test in SAS is difficult to understand for those of us who are beginner SAS users. All treatments appear as a vertical line below the the horizontal line for control at 90.5 with t2 and t3 in the shaded area. For treatment 1 the shaded area is above the line for control. Looking at just the output table I would say that t2 and t3 are significantly lower than the control. I guess I would like to know if my interpretation of the outputs is correct that treatments 2 and 3 are statistically significantly lower than the control? Should I have used an upper bound one tailed test instead?
November 10, 2021 at 1:00 am
Thanks Jim. Please help me understand how a two tailed testing can be used to minimize errors in research
July 1, 2021 at 9:19 am
Hi Jim, Thanks for posting such a thorough and well-written explanation. It was extremely useful to clear up some doubts.
May 7, 2021 at 4:27 pm
Hi Jim, I followed your instructions for the Excel add-in. Thank you. I am very new to statistics and sort of enjoy it as I enter week number two in my class. I am to select if three scenarios call for a one or two-tailed test is required and why. The problem is stated:
30% of mole biopsies are unnecessary. Last month at his clinic, 210 out of 634 had benign biopsy results. Is there enough evidence to reject the dermatologist’s claim?
Part two, the wording changes to “more than of 30% of biopsies,” and part three, the wording changes to “less than 30% of biopsies…”
I am not asking for the problem to be solved for me, but I cannot seem to find direction needed. I know the elements i am dealing with are =30%, greater than 30%, and less than 30%. 210 and 634. I just don’t know what to with the information. I can’t seem to find an example of a similar problem to work with.
May 9, 2021 at 9:22 pm
As I detail in this post, a two-tailed test tells you whether an effect exists in either direction. Or, is it different from the null value in either direction. For the first example, the wording suggests you’d need a two-tailed test to determine whether the population proportion is ≠ 30%. Whenever you just need to know ≠, it suggests a two-tailed test because you’re covering both directions.
For part two, because it’s in one direction (greater than), you need a one-tailed test. Same for part three but it’s less than. Look in this blog post to see how you’d construct the null and alternative hypotheses for these cases. Note that you’re working with a proportion rather than the mean, but the principles are the same! Just plug your scenario and the concept of proportion into the wording I use for the hypotheses.
I hope that helps!
April 11, 2021 at 9:30 am
Hello Jim, great website! I am using a statistics program (SPSS) that does NOT compute one-tailed t-tests. I am trying to compare two independent groups and have justifiable reasons why I only care about one direction. Can I do the following? Use SPSS for two-tailed tests to calculate the t & p values. Then report the p-value as p/2 when it is in the predicted direction (e.g , SPSS says p = .04, so I report p = .02), and report the p-value as 1 – (p/2) when it is in the opposite direction (e.g., SPSS says p = .04, so I report p = .98)? If that is incorrect, what do you suggest (hopefully besides changing statistics programs)? Also, if I want to report confidence intervals, I realize that I would only have an upper or lower bound, but can I use the CI’s from SPSS to compute that? Thank you very much!
April 11, 2021 at 5:42 pm
Yes, for p-values, that’s absolutely correct for both cases.
For confidence intervals, if you take one endpoint of a two-side CI, it becomes a one-side bound with half the confidence level.
Consequently, to obtain a one-sided bound with your desired confidence level, you need to take your desired significance level (e.g., 0.05) and double it. Then subtract it from 1. So, if you’re using a significance level of 0.05, double that to 0.10 and then subtract from 1 (1 – 0.10 = 0.90). 90% is the confidence level you want to use for a two-sided test. After obtaining the two-sided CI, use one of the endpoints depending on the direction of your hypothesis (i.e., upper or lower bound). That’s produces the one-sided the bound with the confidence level that you want. For our example, we calculated a 95% one-sided bound.
March 3, 2021 at 8:27 am
Hi Jim. I used the one-tailed(right) statistical test to determine an anomaly in the below problem statement: On a daily basis, I calculate the (mapped_%) in a common field between two tables.
The way I used the t-test is: On any particular day, I calculate the sample_mean, S.D and sample_count (n=30) for the last 30 days including the current day. My null hypothesis, H0 (pop. mean)=95 and H1>95 (alternate hypothesis). So, I calculate the t-stat based on the sample_mean, pop.mean, sample S.D and n. I then choose the t-crit value for 0.05 from my t-ditribution table for dof(n-1). On the current day if my abs.(t-stat)>t-crit, then I reject the null hypothesis and I say the mapped_pct on that day has passed the t-test.
I get some weird results here, where if my mapped_pct is as low as 6%-8% in all the past 30 days, the t-test still gets a “pass” result. Could you help on this? If my hypothesis needs to be changed.
I would basically look for the mapped_pct >95, if it worked on a static trigger. How can I use the t-test effectively in this problem statement?
December 18, 2020 at 8:23 pm
Hello Dr. Jim, I am wondering if there is evidence in one of your books or other source you could provide, which supports that it is OK not to divide alpha level by 2 in one-tailed hypotheses. I need the source for supporting evidence in a Portfolio exercise and couldn’t find one.
I am grateful for your reply and for your statistics knowledge sharing!
November 27, 2020 at 10:31 pm
If I did a one directional F test ANOVA(one tail ) and wanted to calculate a confidence interval for each individual groups (3) mean . Would I use a one tailed or two tailed t , within my confidence interval .
November 29, 2020 at 2:36 am
Hi Bashiru,
F-tests for ANOVA will always be one-tailed for the reasons I discuss in this post. To learn more about, read my post about F-tests in ANOVA .
For the differences between my groups, I would not use t-tests because the family-wise error rate quickly grows out of hand. To learn more about how to compare group means while controlling the familywise error rate, read my post about using post hoc tests with ANOVA . Typically, these are two-side intervals but you’d be able to use one-sided.
November 26, 2020 at 10:51 am
Hi Jim, I had a question about the formulation of the hypotheses. When you want to test if a beta = 1 or a beta = 0. What will be the null hypotheses? I’m having trouble with finding out. Because in most cases beta = 0 is the null hypotheses but in this case you want to test if beta = 0. so i’m having my doubts can it in this case be the alternative hypotheses or is it still the null hypotheses?
Kind regards, Noa
November 27, 2020 at 1:21 am
Typically, the null hypothesis represents no effect or no relationship. As an analyst, you’re hoping that your data have enough evidence to reject the null and favor the alternative.
Assuming you’re referring to beta as in regression coefficients, zero represents no relationship. Consequently, beta = 0 is the null hypothesis.
You might hope that beta = 1, but you don’t usually include that in your alternative hypotheses. The alternative hypothesis usually states that it does not equal no effect. In other words, there is an effect but it doesn’t state what it is.
There are some exceptions to the above but I’m writing about the standard case.
November 22, 2020 at 8:46 am
Your articles are a help to intro to econometrics students. Keep up the good work! More power to you!
November 6, 2020 at 11:25 pm
Hello Jim. Can you help me with these please?
Write the null and alternative hypothesis using a 1-tailed and 2-tailed test for each problem. (In paragraph and symbols)
A teacher wants to know if there is a significant difference in the performance in MAT C313 between her morning and afternoon classes.
It is known that in our university canteen, the average waiting time for a customer to receive and pay for his/her order is 20 minutes. Additional personnel has been added and now the management wants to know if the average waiting time had been reduced.
November 8, 2020 at 12:29 am
I cover how to write the hypotheses for the different types of tests in this post. So, you just need to figure which type of test you need to use. In your case, you want to determine whether the mean waiting time is less than the target value of 20 minutes. That’s a 1-sample t-test because you’re comparing a mean to a target value (20 minutes). You specifically want to determine whether the mean is less than the target value. So, that’s a one-tailed test. And, you’re looking for a mean that is “less than” the target.
So, go to the one-tailed section in the post and look for the hypotheses for the effect being less than. That’s the one with the critical region on the left side of the curve.
Now, you need include your own information. In your case, you’re comparing the sample estimate to a population mean of 20. The 20 minutes is your null hypothesis value. Use the symbol mu μ to represent the population mean.
You put all that together and you get the following:
Null: μ ≥ 20 Alternative: μ 0 to denote the null hypothesis and H 1 or H A to denote the alternative hypothesis if that’s what you been using in class.
October 17, 2020 at 12:11 pm
I was just wondering if you could please help with clarifying what the hypothesises would be for say income for gamblers and, age of gamblers. I am struggling to find which means would be compared.
October 17, 2020 at 7:05 pm
Those are both continuous variables, so you’d use either correlation or regression for them. For both of those analyses, the hypotheses are the following:
Null : The correlation or regression coefficient equals zero (i.e., there is no relationship between the variables) Alternative : The coefficient does not equal zero (i.e., there is a relationship between the variables.)
When the p-value is less than your significance level, you reject the null and conclude that a relationship exists.
October 17, 2020 at 3:05 am
I was ask to choose and justify the reason between a one tailed and two tailed test for dummy variables, how do I do that and what does it mean?
October 17, 2020 at 7:11 pm
I don’t have enough information to answer your question. A dummy variable is also known as an indicator variable, which is a binary variable that indicates the presence or absence of a condition or characteristic. If you’re using this variable in a hypothesis test, I’d presume that you’re using a proportions test, which is based on the binomial distribution for binary data.
Choosing between a one-tailed or two-tailed test depends on subject area issues and, possibly, your research objectives. Typically, use a two-tailed test unless you have a very good reason to use a one-tailed test. To understand when you might use a one-tailed test, read my post about when to use a one-tailed hypothesis test .
October 16, 2020 at 2:07 pm
In your one-tailed example, Minitab describes the hypotheses as “Test of mu = 100 vs > 100”. Any idea why Minitab says the null is “=” rather than “= or less than”? No ASCII character for it?
October 16, 2020 at 4:20 pm
I’m not entirely sure even though I used to work there! I know we had some discussions about how to represent that hypothesis but I don’t recall the exact reasoning. I suspect that it has to do with the conclusions that you can draw. Let’s focus on the failing to reject the null hypothesis. If the test statistic falls in that region (i.e., it is not significant), you fail to reject the null. In this case, all you know is that you have insufficient evidence to say it is different than 100. I’m pretty sure that’s why they use the equal sign because it might as well be one.
Mathematically, I think using ≤ is more accurate, which you can really see when you look at the distribution plots. That’s why I phrase the hypotheses using ≤ or ≥ as needed. However, in terms of the interpretation, the “less than” portion doesn’t really add anything of importance. You can conclude that its equal to 100 or greater than 100, but not less than 100.
October 15, 2020 at 5:46 am
Thank you so much for your timely feedback. It helps a lot
October 14, 2020 at 10:47 am
How can i use one tailed test at 5% alpha on this problem?
A manufacturer of cellular phone batteries claims that when fully charged, the mean life of his product lasts for 26 hours with a standard deviation of 5 hours. Mr X, a regular distributor, randomly picked and tested 35 of the batteries. His test showed that the average life of his sample is 25.5 hours. Is there a significant difference between the average life of all the manufacturer’s batteries and the average battery life of his sample?
October 14, 2020 at 8:22 pm
I don’t think you’d want to use a one-tailed test. The goal is to determine whether the sample is significantly different than the manufacturer’s population average. You’re not saying significantly greater than or less than, which would be a one-tailed test. As phrased, you want a two-tailed test because it can detect a difference in either direct.
It sounds like you need to use a 1-sample t-test to test the mean. During this test, enter 26 as the test mean. The procedure will tell you if the sample mean of 25.5 hours is a significantly different from that test mean. Similarly, you’d need a one variance test to determine whether the sample standard deviation is significantly different from the test value of 5 hours.
For both of these tests, compare the p-value to your alpha of 0.05. If the p-value is less than this value, your results are statistically significant.
September 22, 2020 at 4:16 am
Hi Jim, I didn’t get an idea that when to use two tail test and one tail test. Will you please explain?
September 22, 2020 at 10:05 pm
I have a complete article dedicated to that: When Can I Use One-Tailed Tests .
Basically, start with the assumption that you’ll use a two-tailed test but then consider scenarios where a one-tailed test can be appropriate. I talk about all of that in the article.
If you have questions after reading that, please don’t hesitate to ask!
July 31, 2020 at 12:33 pm
Thank you so so much for this webpage.
I have two scenarios that I need some clarification. I will really appreciate it if you can take a look:
So I have several of materials that I know when they are tested after production. My hypothesis is that the earlier they are tested after production, the higher the mean value I should expect. At the same time, the later they are tested after production, the lower the mean value. Since this is more like a “greater or lesser” situation, I should use one tail. Is that the correct approach?
On the other hand, I have several mix of materials that I don’t know when they are tested after production. I only know the mean values of the test. And I only want to know whether one mean value is truly higher or lower than the other, I guess I want to know if they are only significantly different. Should I use two tail for this? If they are not significantly different, I can judge based on the mean values of test alone. And if they are significantly different, then I will need to do other type of analysis. Also, when I get my P-value for two tail, should I compare it to 0.025 or 0.05 if my confidence level is 0.05?
Thank you so much again.
July 31, 2020 at 11:19 pm
For your first, if you absolutely know that the mean must be lower the later the material is tested, that it cannot be higher, that would be a situation where you can use a one-tailed test. However, if that’s not a certainty, you’re just guessing, use a two-tail test. If you’re measuring different items at the different times, use the independent 2-sample t-test. However, if you’re measuring the same items at two time points, use the paired t-test. If it’s appropriate, using the paired t-test will give you more statistical power because it accounts for the variability between items. For more information, see my post about when it’s ok to use a one-tailed test .
For the mix of materials, use a two-tailed test because the effect truly can go either direction.
Always compare the p-value to your full significance level regardless of whether it’s a one or two-tailed test. Don’t divide the significance level in half.
June 17, 2020 at 2:56 pm
Is it possible that we reach to opposite conclusions if we use a critical value method and p value method Secondly if we perform one tail test and use p vale method to conclude our Ho, then do we need to convert sig value of 2 tail into sig value of one tail. That can be done just by dividing it with 2
June 18, 2020 at 5:17 pm
The p-value method and critical value method will always agree as long as you’re not changing anything about how the methodology.
If you’re using statistical software, you don’t need to make any adjustments. The software will do that for you.
However, if you calculating it by hand, you’ll need to take your significance level and then look in the table for your test statistic for a one-tailed test. For example, you’ll want to look up 5% for a one-tailed test rather than a two-tailed test. That’s not as simple as dividing by two. In this article, I show examples of one-tailed and two-tailed tests for the same degrees of freedom. The t critical value for the two-tailed test is +/- 2.086 while for the one-sided test it is 1.725. It is true that probability associated with those critical values doubles for the one-tailed test (2.5% -> 5%), but the critical value itself is not half (2.086 -> 1.725). Study the first several graphs in this article to see why that is true.
For the p-value, you can take a two-tailed p-value and divide by 2 to determine the one-sided p-value. However, if you’re using statistical software, it does that for you.
June 11, 2020 at 3:46 pm
Hello Jim, if you have the time I’d be grateful if you could shed some clarity on this scenario:
“A researcher believes that aromatherapy can relieve stress but wants to determine whether it can also enhance focus. To test this, the researcher selected a random sample of students to take an exam in which the average score in the general population is 77. Prior to the exam, these students studied individually in a small library room where a lavender scent was present. If students in this group scored significantly above the average score in general population [is this one-tailed or two-tailed hypothesis?], then this was taken as evidence that the lavender scent enhanced focus.”
Thank you for your time if you do decide to respond.
June 11, 2020 at 4:00 pm
It’s unclear from the information provided whether the researchers used a one-tailed or two-tailed test. It could be either. A two-tailed test can detect effects in both directions, so it could definitely detect an average group score above the population score. However, you could also detect that effect using a one-tailed test if it was set up correctly. So, there’s not enough information in what you provided to know for sure. It could be either.
However, that’s irrelevant to answering the question. The tricky part, as I see it, is that you’re not entirely sure about why the scores are higher. Are they higher because the lavender scent increased concentration or are they higher because the subjects have lower stress from the lavender? Or, maybe it’s not even related to the scent but some other characteristic of the room or testing conditions in which they took the test. You just know the scores are higher but not necessarily why they’re higher.
I’d say that, no, it’s not necessarily evidence that the lavender scent enhanced focus. There are competing explanations for why the scores are higher. Also, it would be best do this as an experiment with a control and treatment group where subjects are randomly assigned to either group. That process helps establish causality rather than just correlation and helps rules out competing explanations for why the scores are higher.
By the way, I spend a lot of time on these issues in my Introduction to Statistics ebook .
June 9, 2020 at 1:47 pm
If a left tail test has an alpha value of 0.05 how will you find the value in the table
April 19, 2020 at 10:35 am
Hi Jim, My question is in regards to the results in the table in your example of the one-sample T (Two-Tailed) test. above. What about the P-value? The P-value listed is .018. I assuming that is compared to and alpha of 0.025, correct?
In regression analysis, when I get a test statistic for the predictive variable of -2.099 and a p-value of 0.039. Am I comparing the p-value to an alpha of 0.025 or 0.05? Now if I run a Bootstrap for coefficients analysis, the results say the sig (2-tail) is 0.098. What are the critical values and alpha in this case? I’m trying to reconcile what I am seeing in both tables.
Thanks for your help.
April 20, 2020 at 3:24 am
Hi Marvalisa,
For one-tailed tests, you don’t need to divide alpha in half. If you can tell your software to perform a one-tailed test, it’ll do all the calculations necessary so you don’t need to adjust anything. So, if you’re using an alpha of 0.05 for a one-tailed test and your p-value is 0.04, it is significant. The procedures adjust the p-values automatically and it all works out. So, whether you’re using a one-tailed or two-tailed test, you always compare the p-value to the alpha with no need to adjust anything. The procedure does that for you!
The exception would be if for some reason your software doesn’t allow you to specify that you want to use a one-tailed test instead of a two-tailed test. Then, you divide the p-value from a two-tailed test in half to get the p-value for a one tailed test. You’d still compare it to your original alpha.
For regression, the same thing applies. If you want to use a one-tailed test for a cofficient, just divide the p-value in half if you can’t tell the software that you want a one-tailed test. The default is two-tailed. If your software has the option for one-tailed tests for any procedure, including regression, it’ll adjust the p-value for you. So, in the normal course of things, you won’t need to adjust anything.
March 26, 2020 at 12:00 pm
Hey Jim, for a one-tailed hypothesis test with a .05 confidence level, should I use a 95% confidence interval or a 90% confidence interval? Thanks
March 26, 2020 at 5:05 pm
You should use a one-sided 95% confidence interval. One-sided CIs have either an upper OR lower bound but remains unbounded on the other side.
March 16, 2020 at 4:30 pm
This is not applicable to the subject but… When performing tests of equivalence, we look at the confidence interval of the difference between two groups, and we perform two one-sided t-tests for equivalence..
March 15, 2020 at 7:51 am
Thanks for this illustrative blogpost. I had a question on one of your points though.
By definition of H1 and H0, a two-sided alternate hypothesis is that there is a difference in means between the test and control. Not that anything is ‘better’ or ‘worse’.
Just because we observed a negative result in your example, does not mean we can conclude it’s necessarily worse, but instead just ‘different’.
Therefore while it enables us to spot the fact that there may be differences between test and control, we cannot make claims about directional effects. So I struggle to see why they actually need to be used instead of one-sided tests.
What’s your take on this?
March 16, 2020 at 3:02 am
Hi Dominic,
If you’ll notice, I carefully avoid stating better or worse because in a general sense you’re right. However, given the context of a specific experiment, you can conclude whether a negative value is better or worse. As always in statistics, you have to use your subject-area knowledge to help interpret the results. In some cases, a negative value is a bad result. In other cases, it’s not. Use your subject-area knowledge!
I’m not sure why you think that you can’t make claims about directional effects? Of course you can!
As for why you shouldn’t use one-tailed tests for most cases, read my post When Can I Use One-Tailed Tests . That should answer your questions.
May 10, 2019 at 12:36 pm
Your website is absolutely amazing Jim, you seem like the nicest guy for doing this and I like how there’s no ulterior motive, (I wasn’t automatically signed up for emails or anything when leaving this comment). I study economics and found econometrics really difficult at first, but your website explains it so clearly its been a big asset to my studies, keep up the good work!
May 10, 2019 at 2:12 pm
Thank you so much, Jack. Your kind words mean a lot!
April 26, 2019 at 5:05 am
Hy Jim I really need your help now pls
One-tailed and two- tailed hypothesis, is it the same or twice, half or unrelated pls
April 26, 2019 at 11:41 am
Hi Anthony,
I describe how the hypotheses are different in this post. You’ll find your answers.
February 8, 2019 at 8:00 am
Thank you for your blog Jim, I have a Statistics exam soon and your articles let me understand a lot!
February 8, 2019 at 10:52 am
You’re very welcome! I’m happy to hear that it’s been helpful. Best of luck on your exam!
January 12, 2019 at 7:06 am
Hi Jim, When you say target value is 5. Do you mean to say the population mean is 5 and we are trying to validate it with the help of sample mean 4.1 using Hypo tests ?.. If it is so.. How can we measure a population parameter as 5 when it is almost impossible o measure a population parameter. Please clarify
January 12, 2019 at 6:57 pm
When you set a target for a one-sample test, it’s based on a value that is important to you. It’s not a population parameter or anything like that. The example in this post uses a case where we need parts that are stronger on average than a value of 5. We derive the value of 5 by using our subject area knowledge about what is required for a situation. Given our product knowledge for the hypothetical example, we know it should be 5 or higher. So, we use that in the hypothesis test and determine whether the population mean is greater than that target value.
When you perform a one-sample test, a target value is optional. If you don’t supply a target value, you simply obtain a confidence interval for the range of values that the parameter is likely to fall within. But, sometimes there is meaningful number that you want to test for specifically.
I hope that clarifies the rational behind the target value!
November 15, 2018 at 8:08 am
I understand that in Psychology a one tailed hypothesis is preferred. Is that so
November 15, 2018 at 11:30 am
No, there’s no overall preference for one-tailed hypothesis tests in statistics. That would be a study-by-study decision based on the types of possible effects. For more information about this decision, read my post: When Can I Use One-Tailed Tests?
November 6, 2018 at 1:14 am
I’m grateful to you for the explanations on One tail and Two tail hypothesis test. This opens my knowledge horizon beyond what an average statistics textbook can offer. Please include more examples in future posts. Thanks
November 5, 2018 at 10:20 am
Thank you. I will search it as well.
Stan Alekman
November 4, 2018 at 8:48 pm
Jim, what is the difference between the central and non-central t-distributions w/respect to hypothesis testing?
November 5, 2018 at 10:12 am
Hi Stan, this is something I will need to look into. I know central t-distribution is the common Student t-distribution, but I don’t have experience using non-central t-distributions. There might well be a blog post in that–after I learn more!
November 4, 2018 at 7:42 pm
this is awesome.
Comments and Questions Cancel reply
Directional and non-directional hypothesis: A Comprehensive Guide
Karolina Konopka
Customer support manager
Want to talk with us?
In the world of research and statistical analysis, hypotheses play a crucial role in formulating and testing scientific claims. Understanding the differences between directional and non-directional hypothesis is essential for designing sound experiments and drawing accurate conclusions. Whether you’re a student, researcher, or simply curious about the foundations of hypothesis testing, this guide will equip you with the knowledge and tools to navigate this fundamental aspect of scientific inquiry.
Understanding Directional Hypothesis
Understanding directional hypotheses is crucial for conducting hypothesis-driven research, as they guide the selection of appropriate statistical tests and aid in the interpretation of results. By incorporating directional hypotheses, researchers can make more precise predictions, contribute to scientific knowledge, and advance their fields of study.
Definition of directional hypothesis
Directional hypotheses, also known as one-tailed hypotheses, are statements in research that make specific predictions about the direction of a relationship or difference between variables. Unlike non-directional hypotheses, which simply state that there is a relationship or difference without specifying its direction, directional hypotheses provide a focused and precise expectation.
A directional hypothesis predicts either a positive or negative relationship between variables or predicts that one group will perform better than another. It asserts a specific direction of effect or outcome. For example, a directional hypothesis could state that “increased exposure to sunlight will lead to an improvement in mood” or “participants who receive the experimental treatment will exhibit higher levels of cognitive performance compared to the control group.”
Directional hypotheses are formulated based on existing theory, prior research, or logical reasoning, and they guide the researcher’s expectations and analysis. They allow for more targeted predictions and enable researchers to test specific hypotheses using appropriate statistical tests.
The role of directional hypothesis in research
Directional hypotheses also play a significant role in research surveys. Let’s explore their role specifically in the context of survey research:
- Objective-driven surveys : Directional hypotheses help align survey research with specific objectives. By formulating directional hypotheses, researchers can focus on gathering data that directly addresses the predicted relationship or difference between variables of interest.
- Question design and measurement : Directional hypotheses guide the design of survey question types and the selection of appropriate measurement scales. They ensure that the questions are tailored to capture the specific aspects related to the predicted direction, enabling researchers to obtain more targeted and relevant data from survey respondents.
- Data analysis and interpretation : Directional hypotheses assist in data analysis by directing researchers towards appropriate statistical tests and methods. Researchers can analyze the survey data to specifically test the predicted relationship or difference, enhancing the accuracy and reliability of their findings. The results can then be interpreted within the context of the directional hypothesis, providing more meaningful insights.
- Practical implications and decision-making : Directional hypotheses in surveys often have practical implications. When the predicted relationship or difference is confirmed, it informs decision-making processes, program development, or interventions. The survey findings based on directional hypotheses can guide organizations, policymakers, or practitioners in making informed choices to achieve desired outcomes.
- Replication and further research : Directional hypotheses in survey research contribute to the replication and extension of studies. Researchers can replicate the survey with different populations or contexts to assess the generalizability of the predicted relationships. Furthermore, if the directional hypothesis is supported, it encourages further research to explore underlying mechanisms or boundary conditions.
By incorporating directional hypotheses in survey research, researchers can align their objectives, design effective surveys, conduct focused data analysis, and derive practical insights. They provide a framework for organizing survey research and contribute to the accumulation of knowledge in the field.
Examples of research questions for directional hypothesis
Here are some examples of research questions that lend themselves to directional hypotheses:
- Does increased daily exercise lead to a decrease in body weight among sedentary adults?
- Is there a positive relationship between study hours and academic performance among college students?
- Does exposure to violent video games result in an increase in aggressive behavior among adolescents?
- Does the implementation of a mindfulness-based intervention lead to a reduction in stress levels among working professionals?
- Is there a difference in customer satisfaction between Product A and Product B, with Product A expected to have higher satisfaction ratings?
- Does the use of social media influence self-esteem levels, with higher social media usage associated with lower self-esteem?
- Is there a negative relationship between job satisfaction and employee turnover, indicating that lower job satisfaction leads to higher turnover rates?
- Does the administration of a specific medication result in a decrease in symptoms among individuals with a particular medical condition?
- Does increased access to early childhood education lead to improved cognitive development in preschool-aged children?
- Is there a difference in purchase intention between advertisements with celebrity endorsements and advertisements without, with celebrity endorsements expected to have a higher impact?
These research questions generate specific predictions about the direction of the relationship or difference between variables and can be tested using appropriate research methods and statistical analyses.
Definition of non-directional hypothesis
Non-directional hypotheses, also known as two-tailed hypotheses, are statements in research that indicate the presence of a relationship or difference between variables without specifying the direction of the effect. Instead of making predictions about the specific direction of the relationship or difference, non-directional hypotheses simply state that there is an association or distinction between the variables of interest.
Non-directional hypotheses are often used when there is no prior theoretical basis or clear expectation about the direction of the relationship. They leave the possibility open for either a positive or negative relationship, or for both groups to differ in some way without specifying which group will perform better or worse.
Advantages and utility of non-directional hypothesis
Non-directional hypotheses in survey s offer several advantages and utilities, providing flexibility and comprehensive analysis of survey data. Here are some of the key advantages and utilities of using non-directional hypotheses in surveys:
- Exploration of Relationships : Non-directional hypotheses allow researchers to explore and examine relationships between variables without assuming a specific direction. This is particularly useful in surveys where the relationship between variables may not be well-known or there may be conflicting evidence regarding the direction of the effect.
- Flexibility in Question Design : With non-directional hypotheses, survey questions can be designed to measure the relationship between variables without being biased towards a particular outcome. This flexibility allows researchers to collect data and analyze the results more objectively.
- Open to Unexpected Findings : Non-directional hypotheses enable researchers to be open to unexpected or surprising findings in survey data. By not committing to a specific direction of the effect, researchers can identify and explore relationships that may not have been initially anticipated, leading to new insights and discoveries.
- Comprehensive Analysis : Non-directional hypotheses promote comprehensive analysis of survey data by considering the possibility of an effect in either direction. Researchers can assess the magnitude and significance of relationships without limiting their analysis to only one possible outcome.
- S tatistical Validity : Non-directional hypotheses in surveys allow for the use of two-tailed statistical tests, which provide a more conservative and robust assessment of significance. Two-tailed tests consider both positive and negative deviations from the null hypothesis, ensuring accurate and reliable statistical analysis of survey data.
- Exploratory Research : Non-directional hypotheses are particularly useful in exploratory research, where the goal is to gather initial insights and generate hypotheses. Surveys with non-directional hypotheses can help researchers explore various relationships and identify patterns that can guide further research or hypothesis development.
It is worth noting that the choice between directional and non-directional hypotheses in surveys depends on the research objectives, existing knowledge, and the specific variables being investigated. Researchers should carefully consider the advantages and limitations of each approach and select the one that aligns best with their research goals and survey design.
- Share with others
- Twitter Twitter Icon
- LinkedIn LinkedIn Icon
Related posts
Microsurveys: complete guide, recurring surveys: the ultimate guide, close-ended questions: definition, types, and examples, top 10 typeform alternatives and competitors, 15 best google forms alternatives and competitors, top 10 qualtrics alternatives and competitors, get answers today.
- No credit card required
- No time limit on Free plan
You can modify this template in every possible way.
All templates work great on every device.
Have a language expert improve your writing
Run a free plagiarism check in 10 minutes, generate accurate citations for free.
- Knowledge Base
- Null and Alternative Hypotheses | Definitions & Examples
Null & Alternative Hypotheses | Definitions, Templates & Examples
Published on May 6, 2022 by Shaun Turney . Revised on June 22, 2023.
The null and alternative hypotheses are two competing claims that researchers weigh evidence for and against using a statistical test :
- Null hypothesis ( H 0 ): There’s no effect in the population .
- Alternative hypothesis ( H a or H 1 ) : There’s an effect in the population.
Table of contents
Answering your research question with hypotheses, what is a null hypothesis, what is an alternative hypothesis, similarities and differences between null and alternative hypotheses, how to write null and alternative hypotheses, other interesting articles, frequently asked questions.
The null and alternative hypotheses offer competing answers to your research question . When the research question asks “Does the independent variable affect the dependent variable?”:
- The null hypothesis ( H 0 ) answers “No, there’s no effect in the population.”
- The alternative hypothesis ( H a ) answers “Yes, there is an effect in the population.”
The null and alternative are always claims about the population. That’s because the goal of hypothesis testing is to make inferences about a population based on a sample . Often, we infer whether there’s an effect in the population by looking at differences between groups or relationships between variables in the sample. It’s critical for your research to write strong hypotheses .
You can use a statistical test to decide whether the evidence favors the null or alternative hypothesis. Each type of statistical test comes with a specific way of phrasing the null and alternative hypothesis. However, the hypotheses can also be phrased in a general way that applies to any test.
Receive feedback on language, structure, and formatting
Professional editors proofread and edit your paper by focusing on:
- Academic style
- Vague sentences
- Style consistency
See an example
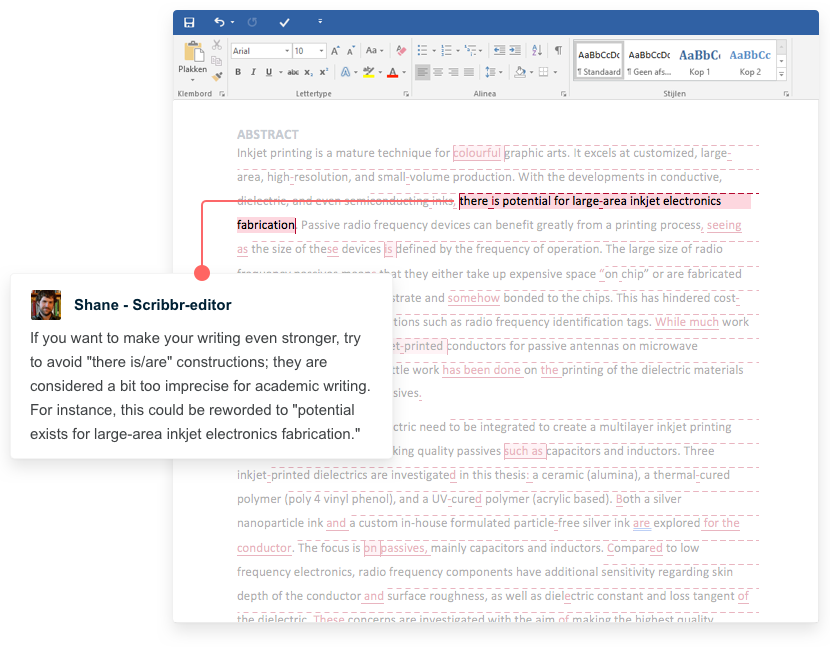
The null hypothesis is the claim that there’s no effect in the population.
If the sample provides enough evidence against the claim that there’s no effect in the population ( p ≤ α), then we can reject the null hypothesis . Otherwise, we fail to reject the null hypothesis.
Although “fail to reject” may sound awkward, it’s the only wording that statisticians accept . Be careful not to say you “prove” or “accept” the null hypothesis.
Null hypotheses often include phrases such as “no effect,” “no difference,” or “no relationship.” When written in mathematical terms, they always include an equality (usually =, but sometimes ≥ or ≤).
You can never know with complete certainty whether there is an effect in the population. Some percentage of the time, your inference about the population will be incorrect. When you incorrectly reject the null hypothesis, it’s called a type I error . When you incorrectly fail to reject it, it’s a type II error.
Examples of null hypotheses
The table below gives examples of research questions and null hypotheses. There’s always more than one way to answer a research question, but these null hypotheses can help you get started.
( ) | ||
Does tooth flossing affect the number of cavities? | Tooth flossing has on the number of cavities. | test: The mean number of cavities per person does not differ between the flossing group (µ ) and the non-flossing group (µ ) in the population; µ = µ . |
Does the amount of text highlighted in the textbook affect exam scores? | The amount of text highlighted in the textbook has on exam scores. | : There is no relationship between the amount of text highlighted and exam scores in the population; β = 0. |
Does daily meditation decrease the incidence of depression? | Daily meditation the incidence of depression.* | test: The proportion of people with depression in the daily-meditation group ( ) is greater than or equal to the no-meditation group ( ) in the population; ≥ . |
*Note that some researchers prefer to always write the null hypothesis in terms of “no effect” and “=”. It would be fine to say that daily meditation has no effect on the incidence of depression and p 1 = p 2 .
The alternative hypothesis ( H a ) is the other answer to your research question . It claims that there’s an effect in the population.
Often, your alternative hypothesis is the same as your research hypothesis. In other words, it’s the claim that you expect or hope will be true.
The alternative hypothesis is the complement to the null hypothesis. Null and alternative hypotheses are exhaustive, meaning that together they cover every possible outcome. They are also mutually exclusive, meaning that only one can be true at a time.
Alternative hypotheses often include phrases such as “an effect,” “a difference,” or “a relationship.” When alternative hypotheses are written in mathematical terms, they always include an inequality (usually ≠, but sometimes < or >). As with null hypotheses, there are many acceptable ways to phrase an alternative hypothesis.
Examples of alternative hypotheses
The table below gives examples of research questions and alternative hypotheses to help you get started with formulating your own.
Does tooth flossing affect the number of cavities? | Tooth flossing has an on the number of cavities. | test: The mean number of cavities per person differs between the flossing group (µ ) and the non-flossing group (µ ) in the population; µ ≠ µ . |
Does the amount of text highlighted in a textbook affect exam scores? | The amount of text highlighted in the textbook has an on exam scores. | : There is a relationship between the amount of text highlighted and exam scores in the population; β ≠ 0. |
Does daily meditation decrease the incidence of depression? | Daily meditation the incidence of depression. | test: The proportion of people with depression in the daily-meditation group ( ) is less than the no-meditation group ( ) in the population; < . |
Null and alternative hypotheses are similar in some ways:
- They’re both answers to the research question.
- They both make claims about the population.
- They’re both evaluated by statistical tests.
However, there are important differences between the two types of hypotheses, summarized in the following table.
A claim that there is in the population. | A claim that there is in the population. | |
| ||
Equality symbol (=, ≥, or ≤) | Inequality symbol (≠, <, or >) | |
Rejected | Supported | |
Failed to reject | Not supported |
To help you write your hypotheses, you can use the template sentences below. If you know which statistical test you’re going to use, you can use the test-specific template sentences. Otherwise, you can use the general template sentences.
General template sentences
The only thing you need to know to use these general template sentences are your dependent and independent variables. To write your research question, null hypothesis, and alternative hypothesis, fill in the following sentences with your variables:
Does independent variable affect dependent variable ?
- Null hypothesis ( H 0 ): Independent variable does not affect dependent variable.
- Alternative hypothesis ( H a ): Independent variable affects dependent variable.
Test-specific template sentences
Once you know the statistical test you’ll be using, you can write your hypotheses in a more precise and mathematical way specific to the test you chose. The table below provides template sentences for common statistical tests.
( ) | ||
test
with two groups | The mean dependent variable does not differ between group 1 (µ ) and group 2 (µ ) in the population; µ = µ . | The mean dependent variable differs between group 1 (µ ) and group 2 (µ ) in the population; µ ≠ µ . |
with three groups | The mean dependent variable does not differ between group 1 (µ ), group 2 (µ ), and group 3 (µ ) in the population; µ = µ = µ . | The mean dependent variable of group 1 (µ ), group 2 (µ ), and group 3 (µ ) are not all equal in the population. |
There is no correlation between independent variable and dependent variable in the population; ρ = 0. | There is a correlation between independent variable and dependent variable in the population; ρ ≠ 0. | |
There is no relationship between independent variable and dependent variable in the population; β = 0. | There is a relationship between independent variable and dependent variable in the population; β ≠ 0. | |
Two-proportions test | The dependent variable expressed as a proportion does not differ between group 1 ( ) and group 2 ( ) in the population; = . | The dependent variable expressed as a proportion differs between group 1 ( ) and group 2 ( ) in the population; ≠ . |
Note: The template sentences above assume that you’re performing one-tailed tests . One-tailed tests are appropriate for most studies.
If you want to know more about statistics , methodology , or research bias , make sure to check out some of our other articles with explanations and examples.
- Normal distribution
- Descriptive statistics
- Measures of central tendency
- Correlation coefficient
Methodology
- Cluster sampling
- Stratified sampling
- Types of interviews
- Cohort study
- Thematic analysis
Research bias
- Implicit bias
- Cognitive bias
- Survivorship bias
- Availability heuristic
- Nonresponse bias
- Regression to the mean
Hypothesis testing is a formal procedure for investigating our ideas about the world using statistics. It is used by scientists to test specific predictions, called hypotheses , by calculating how likely it is that a pattern or relationship between variables could have arisen by chance.
Null and alternative hypotheses are used in statistical hypothesis testing . The null hypothesis of a test always predicts no effect or no relationship between variables, while the alternative hypothesis states your research prediction of an effect or relationship.
The null hypothesis is often abbreviated as H 0 . When the null hypothesis is written using mathematical symbols, it always includes an equality symbol (usually =, but sometimes ≥ or ≤).
The alternative hypothesis is often abbreviated as H a or H 1 . When the alternative hypothesis is written using mathematical symbols, it always includes an inequality symbol (usually ≠, but sometimes < or >).
A research hypothesis is your proposed answer to your research question. The research hypothesis usually includes an explanation (“ x affects y because …”).
A statistical hypothesis, on the other hand, is a mathematical statement about a population parameter. Statistical hypotheses always come in pairs: the null and alternative hypotheses . In a well-designed study , the statistical hypotheses correspond logically to the research hypothesis.
Cite this Scribbr article
If you want to cite this source, you can copy and paste the citation or click the “Cite this Scribbr article” button to automatically add the citation to our free Citation Generator.
Turney, S. (2023, June 22). Null & Alternative Hypotheses | Definitions, Templates & Examples. Scribbr. Retrieved August 23, 2024, from https://www.scribbr.com/statistics/null-and-alternative-hypotheses/
Is this article helpful?
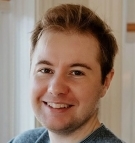
Shaun Turney
Other students also liked, inferential statistics | an easy introduction & examples, hypothesis testing | a step-by-step guide with easy examples, type i & type ii errors | differences, examples, visualizations, what is your plagiarism score.
What is a Directional Hypothesis? (Definition & Examples)
A statistical hypothesis is an assumption about a population parameter . For example, we may assume that the mean height of a male in the U.S. is 70 inches.
The assumption about the height is the statistical hypothesis and the true mean height of a male in the U.S. is the population parameter .
To test whether a statistical hypothesis about a population parameter is true, we obtain a random sample from the population and perform a hypothesis test on the sample data.
Whenever we perform a hypothesis test, we always write down a null and alternative hypothesis:
- Null Hypothesis (H 0 ): The sample data occurs purely from chance.
- Alternative Hypothesis (H A ): The sample data is influenced by some non-random cause.
A hypothesis test can either contain a directional hypothesis or a non-directional hypothesis:
- Directional hypothesis: The alternative hypothesis contains the less than (“”) sign. This indicates that we’re testing whether or not there is a positive or negative effect.
- Non-directional hypothesis: The alternative hypothesis contains the not equal (“≠”) sign. This indicates that we’re testing whether or not there is some effect, without specifying the direction of the effect.
Note that directional hypothesis tests are also called “one-tailed” tests and non-directional hypothesis tests are also called “two-tailed” tests.
Check out the following examples to gain a better understanding of directional vs. non-directional hypothesis tests.
Example 1: Baseball Programs
A baseball coach believes a certain 4-week program will increase the mean hitting percentage of his players, which is currently 0.285.
To test this, he measures the hitting percentage of each of his players before and after participating in the program.
He then performs a hypothesis test using the following hypotheses:
- H 0 : μ = .285 (the program will have no effect on the mean hitting percentage)
- H A : μ > .285 (the program will cause mean hitting percentage to increase)
This is an example of a directional hypothesis because the alternative hypothesis contains the greater than “>” sign. The coach believes that the program will influence the mean hitting percentage of his players in a positive direction.
Example 2: Plant Growth
A biologist believes that a certain pesticide will cause plants to grow less during a one-month period than they normally do, which is currently 10 inches.
To test this, she applies the pesticide to each of the plants in her laboratory for one month.
She then performs a hypothesis test using the following hypotheses:
- H 0 : μ = 10 inches (the pesticide will have no effect on the mean plant growth)
This is also an example of a directional hypothesis because the alternative hypothesis contains the less than “negative direction.
Example 3: Studying Technique
A professor believes that a certain studying technique will influence the mean score that her students receive on a certain exam, but she’s unsure if it will increase or decrease the mean score, which is currently 82.
To test this, she lets each student use the studying technique for one month leading up to the exam and then administers the same exam to each of the students.
- H 0 : μ = 82 (the studying technique will have no effect on the mean exam score)
- H A : μ ≠ 82 (the studying technique will cause the mean exam score to be different than 82)
This is an example of a non-directional hypothesis because the alternative hypothesis contains the not equal “≠” sign. The professor believes that the studying technique will influence the mean exam score, but doesn’t specify whether it will cause the mean score to increase or decrease.
Additional Resources
Introduction to Hypothesis Testing Introduction to the One Sample t-test Introduction to the Two Sample t-test Introduction to the Paired Samples t-test
How to Perform a Partial F-Test in Excel
4 examples of confidence intervals in real life, related posts, how to normalize data between -1 and 1, vba: how to check if string contains another..., how to interpret f-values in a two-way anova, how to create a vector of ones in..., how to find the mode of a histogram..., how to find quartiles in even and odd..., how to determine if a probability distribution is..., what is a symmetric histogram (definition & examples), how to calculate sxy in statistics (with example), how to calculate sxx in statistics (with example).
What is The Null Hypothesis & When Do You Reject The Null Hypothesis
Julia Simkus
Editor at Simply Psychology
BA (Hons) Psychology, Princeton University
Julia Simkus is a graduate of Princeton University with a Bachelor of Arts in Psychology. She is currently studying for a Master's Degree in Counseling for Mental Health and Wellness in September 2023. Julia's research has been published in peer reviewed journals.
Learn about our Editorial Process
Saul McLeod, PhD
Editor-in-Chief for Simply Psychology
BSc (Hons) Psychology, MRes, PhD, University of Manchester
Saul McLeod, PhD., is a qualified psychology teacher with over 18 years of experience in further and higher education. He has been published in peer-reviewed journals, including the Journal of Clinical Psychology.
Olivia Guy-Evans, MSc
Associate Editor for Simply Psychology
BSc (Hons) Psychology, MSc Psychology of Education
Olivia Guy-Evans is a writer and associate editor for Simply Psychology. She has previously worked in healthcare and educational sectors.
On This Page:
A null hypothesis is a statistical concept suggesting no significant difference or relationship between measured variables. It’s the default assumption unless empirical evidence proves otherwise.
The null hypothesis states no relationship exists between the two variables being studied (i.e., one variable does not affect the other).
The null hypothesis is the statement that a researcher or an investigator wants to disprove.
Testing the null hypothesis can tell you whether your results are due to the effects of manipulating the dependent variable or due to random chance.
How to Write a Null Hypothesis
Null hypotheses (H0) start as research questions that the investigator rephrases as statements indicating no effect or relationship between the independent and dependent variables.
It is a default position that your research aims to challenge or confirm.
For example, if studying the impact of exercise on weight loss, your null hypothesis might be:
There is no significant difference in weight loss between individuals who exercise daily and those who do not.
Examples of Null Hypotheses
Research Question | Null Hypothesis |
---|---|
Do teenagers use cell phones more than adults? | Teenagers and adults use cell phones the same amount. |
Do tomato plants exhibit a higher rate of growth when planted in compost rather than in soil? | Tomato plants show no difference in growth rates when planted in compost rather than soil. |
Does daily meditation decrease the incidence of depression? | Daily meditation does not decrease the incidence of depression. |
Does daily exercise increase test performance? | There is no relationship between daily exercise time and test performance. |
Does the new vaccine prevent infections? | The vaccine does not affect the infection rate. |
Does flossing your teeth affect the number of cavities? | Flossing your teeth has no effect on the number of cavities. |
When Do We Reject The Null Hypothesis?
We reject the null hypothesis when the data provide strong enough evidence to conclude that it is likely incorrect. This often occurs when the p-value (probability of observing the data given the null hypothesis is true) is below a predetermined significance level.
If the collected data does not meet the expectation of the null hypothesis, a researcher can conclude that the data lacks sufficient evidence to back up the null hypothesis, and thus the null hypothesis is rejected.
Rejecting the null hypothesis means that a relationship does exist between a set of variables and the effect is statistically significant ( p > 0.05).
If the data collected from the random sample is not statistically significance , then the null hypothesis will be accepted, and the researchers can conclude that there is no relationship between the variables.
You need to perform a statistical test on your data in order to evaluate how consistent it is with the null hypothesis. A p-value is one statistical measurement used to validate a hypothesis against observed data.
Calculating the p-value is a critical part of null-hypothesis significance testing because it quantifies how strongly the sample data contradicts the null hypothesis.
The level of statistical significance is often expressed as a p -value between 0 and 1. The smaller the p-value, the stronger the evidence that you should reject the null hypothesis.
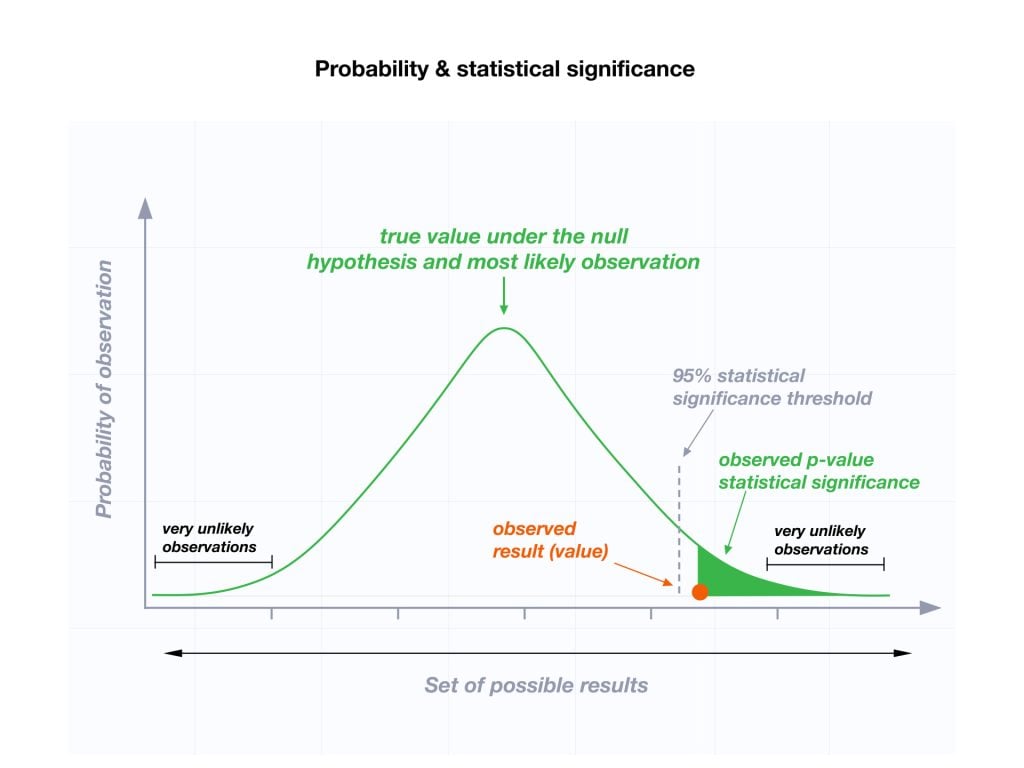
Usually, a researcher uses a confidence level of 95% or 99% (p-value of 0.05 or 0.01) as general guidelines to decide if you should reject or keep the null.
When your p-value is less than or equal to your significance level, you reject the null hypothesis.
In other words, smaller p-values are taken as stronger evidence against the null hypothesis. Conversely, when the p-value is greater than your significance level, you fail to reject the null hypothesis.
In this case, the sample data provides insufficient data to conclude that the effect exists in the population.
Because you can never know with complete certainty whether there is an effect in the population, your inferences about a population will sometimes be incorrect.
When you incorrectly reject the null hypothesis, it’s called a type I error. When you incorrectly fail to reject it, it’s called a type II error.
Why Do We Never Accept The Null Hypothesis?
The reason we do not say “accept the null” is because we are always assuming the null hypothesis is true and then conducting a study to see if there is evidence against it. And, even if we don’t find evidence against it, a null hypothesis is not accepted.
A lack of evidence only means that you haven’t proven that something exists. It does not prove that something doesn’t exist.
It is risky to conclude that the null hypothesis is true merely because we did not find evidence to reject it. It is always possible that researchers elsewhere have disproved the null hypothesis, so we cannot accept it as true, but instead, we state that we failed to reject the null.
One can either reject the null hypothesis, or fail to reject it, but can never accept it.
Why Do We Use The Null Hypothesis?
We can never prove with 100% certainty that a hypothesis is true; We can only collect evidence that supports a theory. However, testing a hypothesis can set the stage for rejecting or accepting this hypothesis within a certain confidence level.
The null hypothesis is useful because it can tell us whether the results of our study are due to random chance or the manipulation of a variable (with a certain level of confidence).
A null hypothesis is rejected if the measured data is significantly unlikely to have occurred and a null hypothesis is accepted if the observed outcome is consistent with the position held by the null hypothesis.
Rejecting the null hypothesis sets the stage for further experimentation to see if a relationship between two variables exists.
Hypothesis testing is a critical part of the scientific method as it helps decide whether the results of a research study support a particular theory about a given population. Hypothesis testing is a systematic way of backing up researchers’ predictions with statistical analysis.
It helps provide sufficient statistical evidence that either favors or rejects a certain hypothesis about the population parameter.
Purpose of a Null Hypothesis
- The primary purpose of the null hypothesis is to disprove an assumption.
- Whether rejected or accepted, the null hypothesis can help further progress a theory in many scientific cases.
- A null hypothesis can be used to ascertain how consistent the outcomes of multiple studies are.
Do you always need both a Null Hypothesis and an Alternative Hypothesis?
The null (H0) and alternative (Ha or H1) hypotheses are two competing claims that describe the effect of the independent variable on the dependent variable. They are mutually exclusive, which means that only one of the two hypotheses can be true.
While the null hypothesis states that there is no effect in the population, an alternative hypothesis states that there is statistical significance between two variables.
The goal of hypothesis testing is to make inferences about a population based on a sample. In order to undertake hypothesis testing, you must express your research hypothesis as a null and alternative hypothesis. Both hypotheses are required to cover every possible outcome of the study.
What is the difference between a null hypothesis and an alternative hypothesis?
The alternative hypothesis is the complement to the null hypothesis. The null hypothesis states that there is no effect or no relationship between variables, while the alternative hypothesis claims that there is an effect or relationship in the population.
It is the claim that you expect or hope will be true. The null hypothesis and the alternative hypothesis are always mutually exclusive, meaning that only one can be true at a time.
What are some problems with the null hypothesis?
One major problem with the null hypothesis is that researchers typically will assume that accepting the null is a failure of the experiment. However, accepting or rejecting any hypothesis is a positive result. Even if the null is not refuted, the researchers will still learn something new.
Why can a null hypothesis not be accepted?
We can either reject or fail to reject a null hypothesis, but never accept it. If your test fails to detect an effect, this is not proof that the effect doesn’t exist. It just means that your sample did not have enough evidence to conclude that it exists.
We can’t accept a null hypothesis because a lack of evidence does not prove something that does not exist. Instead, we fail to reject it.
Failing to reject the null indicates that the sample did not provide sufficient enough evidence to conclude that an effect exists.
If the p-value is greater than the significance level, then you fail to reject the null hypothesis.
Is a null hypothesis directional or non-directional?
A hypothesis test can either contain an alternative directional hypothesis or a non-directional alternative hypothesis. A directional hypothesis is one that contains the less than (“<“) or greater than (“>”) sign.
A nondirectional hypothesis contains the not equal sign (“≠”). However, a null hypothesis is neither directional nor non-directional.
A null hypothesis is a prediction that there will be no change, relationship, or difference between two variables.
The directional hypothesis or nondirectional hypothesis would then be considered alternative hypotheses to the null hypothesis.
Gill, J. (1999). The insignificance of null hypothesis significance testing. Political research quarterly , 52 (3), 647-674.
Krueger, J. (2001). Null hypothesis significance testing: On the survival of a flawed method. American Psychologist , 56 (1), 16.
Masson, M. E. (2011). A tutorial on a practical Bayesian alternative to null-hypothesis significance testing. Behavior research methods , 43 , 679-690.
Nickerson, R. S. (2000). Null hypothesis significance testing: a review of an old and continuing controversy. Psychological methods , 5 (2), 241.
Rozeboom, W. W. (1960). The fallacy of the null-hypothesis significance test. Psychological bulletin , 57 (5), 416.

User Preferences
Content preview.
Arcu felis bibendum ut tristique et egestas quis:
- Ut enim ad minim veniam, quis nostrud exercitation ullamco laboris
- Duis aute irure dolor in reprehenderit in voluptate
- Excepteur sint occaecat cupidatat non proident
Keyboard Shortcuts
10.1 - setting the hypotheses: examples.
A significance test examines whether the null hypothesis provides a plausible explanation of the data. The null hypothesis itself does not involve the data. It is a statement about a parameter (a numerical characteristic of the population). These population values might be proportions or means or differences between means or proportions or correlations or odds ratios or any other numerical summary of the population. The alternative hypothesis is typically the research hypothesis of interest. Here are some examples.
Example 10.2: Hypotheses with One Sample of One Categorical Variable Section
About 10% of the human population is left-handed. Suppose a researcher at Penn State speculates that students in the College of Arts and Architecture are more likely to be left-handed than people found in the general population. We only have one sample since we will be comparing a population proportion based on a sample value to a known population value.
- Research Question : Are artists more likely to be left-handed than people found in the general population?
- Response Variable : Classification of the student as either right-handed or left-handed
State Null and Alternative Hypotheses
- Null Hypothesis : Students in the College of Arts and Architecture are no more likely to be left-handed than people in the general population (population percent of left-handed students in the College of Art and Architecture = 10% or p = .10).
- Alternative Hypothesis : Students in the College of Arts and Architecture are more likely to be left-handed than people in the general population (population percent of left-handed students in the College of Arts and Architecture > 10% or p > .10). This is a one-sided alternative hypothesis.
Example 10.3: Hypotheses with One Sample of One Measurement Variable Section
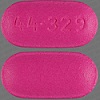
A generic brand of the anti-histamine Diphenhydramine markets a capsule with a 50 milligram dose. The manufacturer is worried that the machine that fills the capsules has come out of calibration and is no longer creating capsules with the appropriate dosage.
- Research Question : Does the data suggest that the population mean dosage of this brand is different than 50 mg?
- Response Variable : dosage of the active ingredient found by a chemical assay.
- Null Hypothesis : On the average, the dosage sold under this brand is 50 mg (population mean dosage = 50 mg).
- Alternative Hypothesis : On the average, the dosage sold under this brand is not 50 mg (population mean dosage ≠ 50 mg). This is a two-sided alternative hypothesis.
Example 10.4: Hypotheses with Two Samples of One Categorical Variable Section
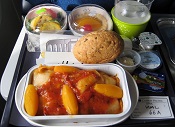
Many people are starting to prefer vegetarian meals on a regular basis. Specifically, a researcher believes that females are more likely than males to eat vegetarian meals on a regular basis.
- Research Question : Does the data suggest that females are more likely than males to eat vegetarian meals on a regular basis?
- Response Variable : Classification of whether or not a person eats vegetarian meals on a regular basis
- Explanatory (Grouping) Variable: Sex
- Null Hypothesis : There is no sex effect regarding those who eat vegetarian meals on a regular basis (population percent of females who eat vegetarian meals on a regular basis = population percent of males who eat vegetarian meals on a regular basis or p females = p males ).
- Alternative Hypothesis : Females are more likely than males to eat vegetarian meals on a regular basis (population percent of females who eat vegetarian meals on a regular basis > population percent of males who eat vegetarian meals on a regular basis or p females > p males ). This is a one-sided alternative hypothesis.
Example 10.5: Hypotheses with Two Samples of One Measurement Variable Section
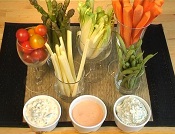
Obesity is a major health problem today. Research is starting to show that people may be able to lose more weight on a low carbohydrate diet than on a low fat diet.
- Research Question : Does the data suggest that, on the average, people are able to lose more weight on a low carbohydrate diet than on a low fat diet?
- Response Variable : Weight loss (pounds)
- Explanatory (Grouping) Variable : Type of diet
- Null Hypothesis : There is no difference in the mean amount of weight loss when comparing a low carbohydrate diet with a low fat diet (population mean weight loss on a low carbohydrate diet = population mean weight loss on a low fat diet).
- Alternative Hypothesis : The mean weight loss should be greater for those on a low carbohydrate diet when compared with those on a low fat diet (population mean weight loss on a low carbohydrate diet > population mean weight loss on a low fat diet). This is a one-sided alternative hypothesis.
Example 10.6: Hypotheses about the relationship between Two Categorical Variables Section
- Research Question : Do the odds of having a stroke increase if you inhale second hand smoke ? A case-control study of non-smoking stroke patients and controls of the same age and occupation are asked if someone in their household smokes.
- Variables : There are two different categorical variables (Stroke patient vs control and whether the subject lives in the same household as a smoker). Living with a smoker (or not) is the natural explanatory variable and having a stroke (or not) is the natural response variable in this situation.
- Null Hypothesis : There is no relationship between whether or not a person has a stroke and whether or not a person lives with a smoker (odds ratio between stroke and second-hand smoke situation is = 1).
- Alternative Hypothesis : There is a relationship between whether or not a person has a stroke and whether or not a person lives with a smoker (odds ratio between stroke and second-hand smoke situation is > 1). This is a one-tailed alternative.
This research question might also be addressed like example 11.4 by making the hypotheses about comparing the proportion of stroke patients that live with smokers to the proportion of controls that live with smokers.
Example 10.7: Hypotheses about the relationship between Two Measurement Variables Section
- Research Question : A financial analyst believes there might be a positive association between the change in a stock's price and the amount of the stock purchased by non-management employees the previous day (stock trading by management being under "insider-trading" regulatory restrictions).
- Variables : Daily price change information (the response variable) and previous day stock purchases by non-management employees (explanatory variable). These are two different measurement variables.
- Null Hypothesis : The correlation between the daily stock price change (\$) and the daily stock purchases by non-management employees (\$) = 0.
- Alternative Hypothesis : The correlation between the daily stock price change (\$) and the daily stock purchases by non-management employees (\$) > 0. This is a one-sided alternative hypothesis.
Example 10.8: Hypotheses about comparing the relationship between Two Measurement Variables in Two Samples Section
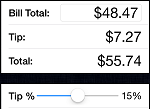
- Research Question : Is there a linear relationship between the amount of the bill (\$) at a restaurant and the tip (\$) that was left. Is the strength of this association different for family restaurants than for fine dining restaurants?
- Variables : There are two different measurement variables. The size of the tip would depend on the size of the bill so the amount of the bill would be the explanatory variable and the size of the tip would be the response variable.
- Null Hypothesis : The correlation between the amount of the bill (\$) at a restaurant and the tip (\$) that was left is the same at family restaurants as it is at fine dining restaurants.
- Alternative Hypothesis : The correlation between the amount of the bill (\$) at a restaurant and the tip (\$) that was left is the difference at family restaurants then it is at fine dining restaurants. This is a two-sided alternative hypothesis.
- +44 7897 053596
- [email protected]
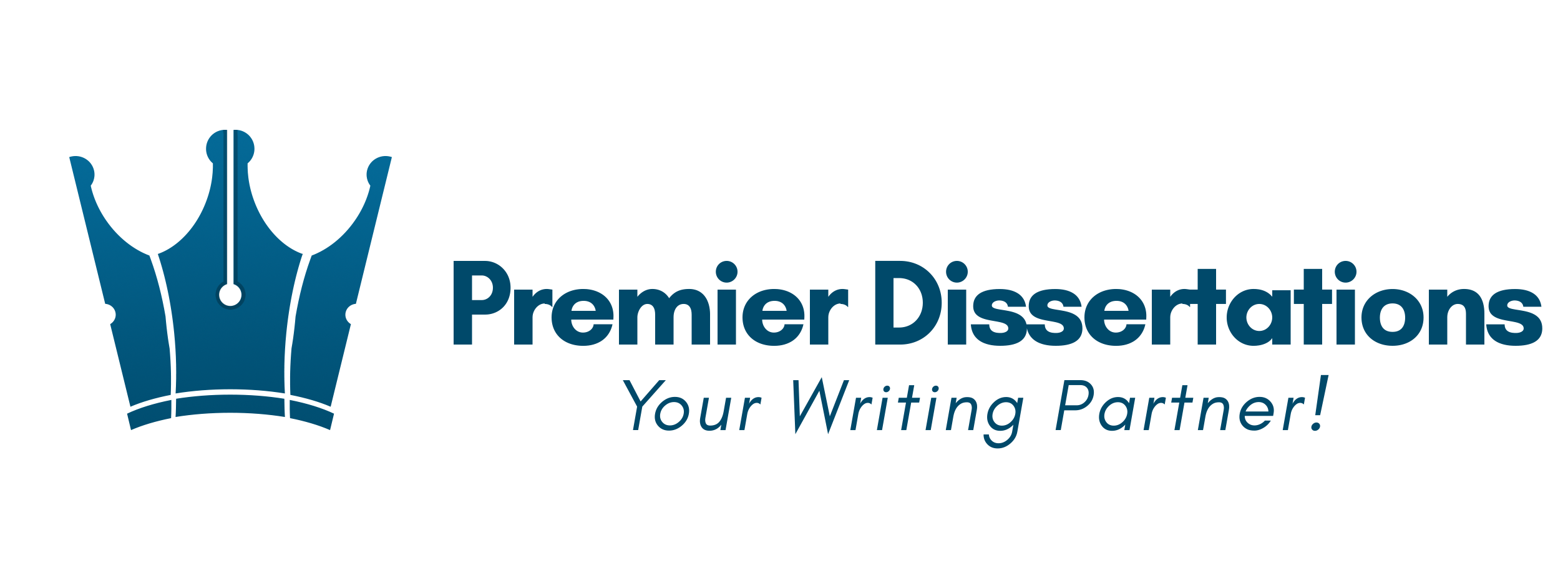
Get an experienced writer start working
Review our examples before placing an order, learn how to draft academic papers, research hypotheses: directional vs. non-directional hypotheses.
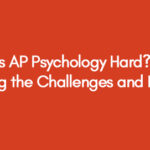
Is AP Psychology Hard? Exploring the Challenges and Rewards
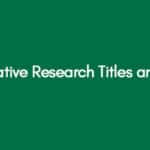
280+ Quantitative Research Titles and Topics
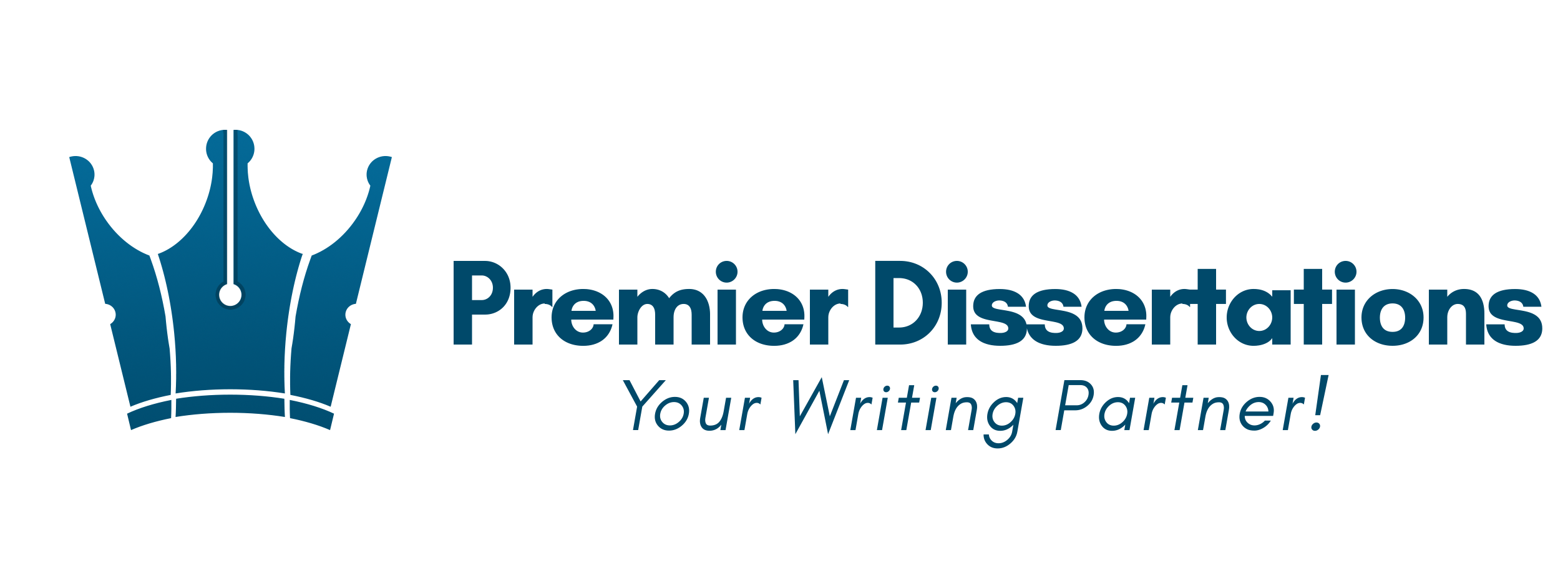
- Dissertation
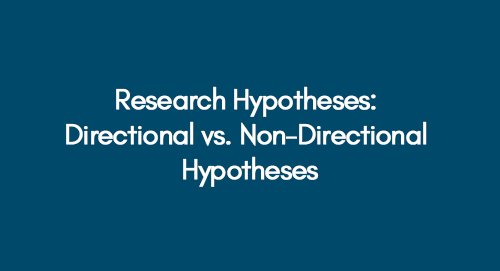
A research hypothesis is a statement that predicts or expects a relationship between variables, and it is tested through research. To create a hypothesis, researchers often review existing literature on the topic. This hypothesis is based on theories, observations, or empirical evidence. It guides the research process, including experiment design, data collection, and analysis. Ultimately, the hypothesis aims to predict the outcome of the study.
What is a Hypothesis in a Dissertation?
This article compares directional and non-directional hypotheses and provides guidelines for writing an effective hypothesis in research. The study explores the differences in predictions and research design implications between the two hypotheses.
3-Step Dissertation Process!

Get 3+ Topics

Dissertation Proposal

Get Final Dissertation
Types of hypothesis.
There are two main types of hypotheses in research:
Null Hypothesis (H0)
The null hypothesis is the default assumption in statistical analysis that there is no significant relationship or effect between the variables being studied. It suggests that any observed differences or relationships are due to chance.
Alternative Hypothesis (Ha or H1)
The alternative hypothesis proposes a significant relationship or effect between variables, contradicting the null hypothesis. It reflects the researcher's expectations based on existing theories or observations.
What is Directional Hypotheses?
A directional hypothesis is a type of hypothesis that is used to predict a specific change or outcome in a research study. It is typically used when researchers have a clear idea of the direction in which they expect their results to go, either an increase or decrease, and want to test this prediction. By making a directional hypothesis, researchers can focus their research efforts and design studies that are more likely to uncover meaningful results. In essence, a directional hypothesis is a statement that predicts the direction of the change that is expected to occur between two groups or variables that are being investigated.
Examples of Directional Hypothesis
Example 1: Online versus Traditional Classroom Learning
For instance, consider a study comparing the average study time of college students in online courses versus those in traditional classroom settings. Drawing on prior research indicating that online learning might lead to reduced engagement, a potential directional hypothesis could be: "Students enrolled in online classes will spend fewer weekly study hours than those in traditional classrooms."
In this scenario, our hypothesis presents a clear expectation—that the average number of weekly study hours among online learners will be lower than that of traditional learners. If the actual findings reveal no significant difference or even higher study times among online learners, then our hypothesis would be refuted.
Example 2: Carbon Dioxide Levels and Global Warming
A directional hypothesis in this scenario would propose a specific change in direction between these two variables. For instance, a directional hypothesis might state that as carbon dioxide levels increase, global temperatures will also rise. This hypothesis suggests a causal relationship between the increase in CO2 levels and the phenomenon of global warming, indicating a direction of change in global temperatures corresponding to changes in CO2 levels.
What is a Non-Directional Hypotheses?
In scientific research, a non-directional hypothesis, or null hypothesis, is a statement that suggests the absence of a relationship or difference between the variables being studied. This type of hypothesis is used to test the validity of a research question by assuming that there is no significant effect or relationship between the variables under investigation. The null hypothesis is typically tested against an alternative hypothesis, which proposes that there is a significant effect or relationship between the variables. If the null hypothesis is rejected, it means that there is enough evidence to suggest that the alternative hypothesis is true, and the variables are indeed related or different from each other.
Non-Directional Hypothesis Example
Example: Is there a difference in anxiety levels between students who receive traditional classroom instruction and those who participate in online learning?
In this non-directional hypothesis, researchers are interested in understanding if there's a disparity in anxiety levels between students who are taught in traditional classrooms versus those who learn online. The non-directional hypothesis posits that there won't be any notable variance in anxiety levels between the two groups. This means that the researchers are not predicting whether one group will have higher or lower anxiety levels; rather, they are exploring if there's any difference at all.
Testimonials
Very satisfied students
This is our reason for working. We want to make all students happy, every day. Review us on Sitejabber
Directional vs. Non-Directional Hypotheses in Research
Both directional and non directional hypothesis have their place in research, and choosing the appropriate type depends on the research question being investigated. Researchers can use directional or non-directional hypotheses in their studies, depending on their specific expectations about the relationship between variables. A directional hypothesis predicts a specific direction of change, while a non-directional hypothesis predicts that there will be a difference between groups or conditions without specifying the direction of that difference. It's important to understand the difference between these types of hypotheses to conduct rigorous and insightful research. Directional hypotheses are useful when researchers want to test a specific expectation about the relationship between variables, while non-directional hypotheses are more appropriate when researchers simply want to test if there is any difference between groups or conditions.
How to Write an Effective Hypothesis in Research?
Writing an effective hypothesis involves several key steps to ensure clarity, testability, and relevance to the research question. Here's a guide on how to write an effective hypothesis:
- Identify the Research Question: Start by clearly defining the research question or problem you want to investigate. Your hypothesis should directly address this question.
- State the Null Hypothesis: The null hypothesis (H0) is a statement that there is no relationship or effect between the variables being studied. It serves as the default assumption and is typically stated as the absence of an effect or difference.
- Formulate the Alternative Hypothesis: The alternative hypothesis (H1 or Ha) is the statement that contradicts the null hypothesis and suggests that there is a relationship or effect between the variables. It reflects what you expect to find in your research.
- Make it Testable: Your hypothesis should be testable through empirical observation or experimentation. This means that there must be a way to collect data or evidence to support or refute the hypothesis.
- Be Specific and Clear: Clearly state the variables involved and the expected relationship between them. Avoid vague or ambiguous language to ensure that your hypothesis is easy to understand and interpret.
- Use Quantifiable Terms: Whenever possible, use quantifiable terms or measurable variables in your hypothesis. This makes it easier to collect data and analyze results objectively.
- Consider the Scope: Ensure that your hypothesis is focused and specific to the research hypothesis at hand. Avoid making broad generalizations that are difficult to test or validate.
- Revise and Refine: Once you've drafted your hypothesis, review it carefully to ensure accuracy and coherence. Revise as needed to clarify any ambiguities or inconsistencies.
Need Help with Academic Writing? Get a Response within 24 Hours!
How Does It Work ?
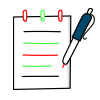
Fill the Form
Please fill the free topic form and share your requirements
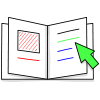
Writer Starts Working
The writer starts to find a topic for you (based on your requirements)
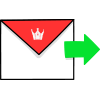
3+ Topics Emailed!
The writer shared custom topics with you within 24 hours
In conclusion, directional hypotheses predict whether variables will increase or decrease, providing a definite expectation about the direction of the relationship under investigation. Non-directional hypotheses, on the other hand, only claim that there is a difference between variables without specifying the direction of the change, leaving it open to any possibility. Both types of hypotheses play an important role in guiding research investigations and developing testable predictions.
Get 3+ Free Dissertation Topics within 24 hours?
Your Number
Academic Level Select Academic Level Undergraduate Masters PhD
Area of Research
Editor Arsalan
Related posts.
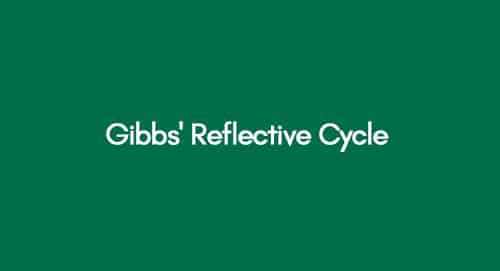
What is Gibbs’ Reflective Cycle and How Can It Benefit You? | Applications and Example
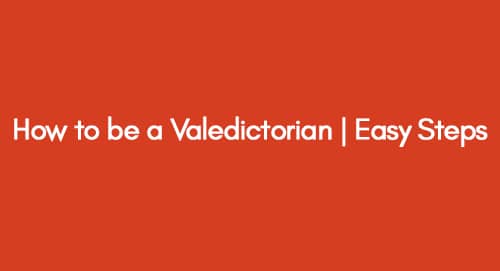
How to be a Valedictorian | Easy Steps
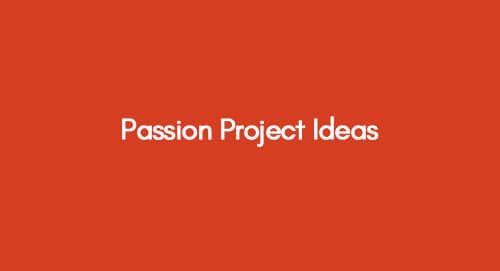
230 Passion Project Ideas for Students
Comments are closed.
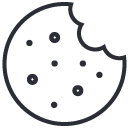
Null Hypothesis Examples
ThoughtCo / Hilary Allison
- Scientific Method
- Chemical Laws
- Periodic Table
- Projects & Experiments
- Biochemistry
- Physical Chemistry
- Medical Chemistry
- Chemistry In Everyday Life
- Famous Chemists
- Activities for Kids
- Abbreviations & Acronyms
- Weather & Climate
- Ph.D., Biomedical Sciences, University of Tennessee at Knoxville
- B.A., Physics and Mathematics, Hastings College
In statistical analysis, the null hypothesis assumes there is no meaningful relationship between two variables. Testing the null hypothesis can tell you whether your results are due to the effect of manipulating a dependent variable or due to chance. It's often used in conjunction with an alternative hypothesis, which assumes there is, in fact, a relationship between two variables.
The null hypothesis is among the easiest hypothesis to test using statistical analysis, making it perhaps the most valuable hypothesis for the scientific method. By evaluating a null hypothesis in addition to another hypothesis, researchers can support their conclusions with a higher level of confidence. Below are examples of how you might formulate a null hypothesis to fit certain questions.
What Is the Null Hypothesis?
The null hypothesis states there is no relationship between the measured phenomenon (the dependent variable ) and the independent variable , which is the variable an experimenter typically controls or changes. You do not need to believe that the null hypothesis is true to test it. On the contrary, you will likely suspect there is a relationship between a set of variables. One way to prove that this is the case is to reject the null hypothesis. Rejecting a hypothesis does not mean an experiment was "bad" or that it didn't produce results. In fact, it is often one of the first steps toward further inquiry.
To distinguish it from other hypotheses , the null hypothesis is written as H 0 (which is read as “H-nought,” "H-null," or "H-zero"). A significance test is used to determine the likelihood that the results supporting the null hypothesis are not due to chance. A confidence level of 95% or 99% is common. Keep in mind, even if the confidence level is high, there is still a small chance the null hypothesis is not true, perhaps because the experimenter did not account for a critical factor or because of chance. This is one reason why it's important to repeat experiments.
Examples of the Null Hypothesis
To write a null hypothesis, first start by asking a question. Rephrase that question in a form that assumes no relationship between the variables. In other words, assume a treatment has no effect. Write your hypothesis in a way that reflects this.
Are teens better at math than adults? | Age has no effect on mathematical ability. |
Does taking aspirin every day reduce the chance of having a heart attack? | Taking aspirin daily does not affect heart attack risk. |
Do teens use cell phones to access the internet more than adults? | Age has no effect on how cell phones are used for internet access. |
Do cats care about the color of their food? | Cats express no food preference based on color. |
Does chewing willow bark relieve pain? | There is no difference in pain relief after chewing willow bark versus taking a placebo. |

Other Types of Hypotheses
In addition to the null hypothesis, the alternative hypothesis is also a staple in traditional significance tests . It's essentially the opposite of the null hypothesis because it assumes the claim in question is true. For the first item in the table above, for example, an alternative hypothesis might be "Age does have an effect on mathematical ability."
Key Takeaways
- In hypothesis testing, the null hypothesis assumes no relationship between two variables, providing a baseline for statistical analysis.
- Rejecting the null hypothesis suggests there is evidence of a relationship between variables.
- By formulating a null hypothesis, researchers can systematically test assumptions and draw more reliable conclusions from their experiments.
- What Are Examples of a Hypothesis?
- Random Error vs. Systematic Error
- Six Steps of the Scientific Method
- What Is a Hypothesis? (Science)
- Scientific Method Flow Chart
- What Are the Elements of a Good Hypothesis?
- Scientific Method Vocabulary Terms
- Understanding Simple vs Controlled Experiments
- The Role of a Controlled Variable in an Experiment
- What Is an Experimental Constant?
- What Is a Testable Hypothesis?
- Scientific Hypothesis Examples
- What Is the Difference Between a Control Variable and Control Group?
- DRY MIX Experiment Variables Acronym
- What Is a Controlled Experiment?
- Scientific Variable

An official website of the United States government
The .gov means it’s official. Federal government websites often end in .gov or .mil. Before sharing sensitive information, make sure you’re on a federal government site.
The site is secure. The https:// ensures that you are connecting to the official website and that any information you provide is encrypted and transmitted securely.
- Publications
- Account settings
Preview improvements coming to the PMC website in October 2024. Learn More or Try it out now .
- Advanced Search
- Journal List
- J Korean Med Sci
- v.37(16); 2022 Apr 25

A Practical Guide to Writing Quantitative and Qualitative Research Questions and Hypotheses in Scholarly Articles
Edward barroga.
1 Department of General Education, Graduate School of Nursing Science, St. Luke’s International University, Tokyo, Japan.
Glafera Janet Matanguihan
2 Department of Biological Sciences, Messiah University, Mechanicsburg, PA, USA.
The development of research questions and the subsequent hypotheses are prerequisites to defining the main research purpose and specific objectives of a study. Consequently, these objectives determine the study design and research outcome. The development of research questions is a process based on knowledge of current trends, cutting-edge studies, and technological advances in the research field. Excellent research questions are focused and require a comprehensive literature search and in-depth understanding of the problem being investigated. Initially, research questions may be written as descriptive questions which could be developed into inferential questions. These questions must be specific and concise to provide a clear foundation for developing hypotheses. Hypotheses are more formal predictions about the research outcomes. These specify the possible results that may or may not be expected regarding the relationship between groups. Thus, research questions and hypotheses clarify the main purpose and specific objectives of the study, which in turn dictate the design of the study, its direction, and outcome. Studies developed from good research questions and hypotheses will have trustworthy outcomes with wide-ranging social and health implications.
INTRODUCTION
Scientific research is usually initiated by posing evidenced-based research questions which are then explicitly restated as hypotheses. 1 , 2 The hypotheses provide directions to guide the study, solutions, explanations, and expected results. 3 , 4 Both research questions and hypotheses are essentially formulated based on conventional theories and real-world processes, which allow the inception of novel studies and the ethical testing of ideas. 5 , 6
It is crucial to have knowledge of both quantitative and qualitative research 2 as both types of research involve writing research questions and hypotheses. 7 However, these crucial elements of research are sometimes overlooked; if not overlooked, then framed without the forethought and meticulous attention it needs. Planning and careful consideration are needed when developing quantitative or qualitative research, particularly when conceptualizing research questions and hypotheses. 4
There is a continuing need to support researchers in the creation of innovative research questions and hypotheses, as well as for journal articles that carefully review these elements. 1 When research questions and hypotheses are not carefully thought of, unethical studies and poor outcomes usually ensue. Carefully formulated research questions and hypotheses define well-founded objectives, which in turn determine the appropriate design, course, and outcome of the study. This article then aims to discuss in detail the various aspects of crafting research questions and hypotheses, with the goal of guiding researchers as they develop their own. Examples from the authors and peer-reviewed scientific articles in the healthcare field are provided to illustrate key points.
DEFINITIONS AND RELATIONSHIP OF RESEARCH QUESTIONS AND HYPOTHESES
A research question is what a study aims to answer after data analysis and interpretation. The answer is written in length in the discussion section of the paper. Thus, the research question gives a preview of the different parts and variables of the study meant to address the problem posed in the research question. 1 An excellent research question clarifies the research writing while facilitating understanding of the research topic, objective, scope, and limitations of the study. 5
On the other hand, a research hypothesis is an educated statement of an expected outcome. This statement is based on background research and current knowledge. 8 , 9 The research hypothesis makes a specific prediction about a new phenomenon 10 or a formal statement on the expected relationship between an independent variable and a dependent variable. 3 , 11 It provides a tentative answer to the research question to be tested or explored. 4
Hypotheses employ reasoning to predict a theory-based outcome. 10 These can also be developed from theories by focusing on components of theories that have not yet been observed. 10 The validity of hypotheses is often based on the testability of the prediction made in a reproducible experiment. 8
Conversely, hypotheses can also be rephrased as research questions. Several hypotheses based on existing theories and knowledge may be needed to answer a research question. Developing ethical research questions and hypotheses creates a research design that has logical relationships among variables. These relationships serve as a solid foundation for the conduct of the study. 4 , 11 Haphazardly constructed research questions can result in poorly formulated hypotheses and improper study designs, leading to unreliable results. Thus, the formulations of relevant research questions and verifiable hypotheses are crucial when beginning research. 12
CHARACTERISTICS OF GOOD RESEARCH QUESTIONS AND HYPOTHESES
Excellent research questions are specific and focused. These integrate collective data and observations to confirm or refute the subsequent hypotheses. Well-constructed hypotheses are based on previous reports and verify the research context. These are realistic, in-depth, sufficiently complex, and reproducible. More importantly, these hypotheses can be addressed and tested. 13
There are several characteristics of well-developed hypotheses. Good hypotheses are 1) empirically testable 7 , 10 , 11 , 13 ; 2) backed by preliminary evidence 9 ; 3) testable by ethical research 7 , 9 ; 4) based on original ideas 9 ; 5) have evidenced-based logical reasoning 10 ; and 6) can be predicted. 11 Good hypotheses can infer ethical and positive implications, indicating the presence of a relationship or effect relevant to the research theme. 7 , 11 These are initially developed from a general theory and branch into specific hypotheses by deductive reasoning. In the absence of a theory to base the hypotheses, inductive reasoning based on specific observations or findings form more general hypotheses. 10
TYPES OF RESEARCH QUESTIONS AND HYPOTHESES
Research questions and hypotheses are developed according to the type of research, which can be broadly classified into quantitative and qualitative research. We provide a summary of the types of research questions and hypotheses under quantitative and qualitative research categories in Table 1 .
Quantitative research questions | Quantitative research hypotheses |
---|---|
Descriptive research questions | Simple hypothesis |
Comparative research questions | Complex hypothesis |
Relationship research questions | Directional hypothesis |
Non-directional hypothesis | |
Associative hypothesis | |
Causal hypothesis | |
Null hypothesis | |
Alternative hypothesis | |
Working hypothesis | |
Statistical hypothesis | |
Logical hypothesis | |
Hypothesis-testing | |
Qualitative research questions | Qualitative research hypotheses |
Contextual research questions | Hypothesis-generating |
Descriptive research questions | |
Evaluation research questions | |
Explanatory research questions | |
Exploratory research questions | |
Generative research questions | |
Ideological research questions | |
Ethnographic research questions | |
Phenomenological research questions | |
Grounded theory questions | |
Qualitative case study questions |
Research questions in quantitative research
In quantitative research, research questions inquire about the relationships among variables being investigated and are usually framed at the start of the study. These are precise and typically linked to the subject population, dependent and independent variables, and research design. 1 Research questions may also attempt to describe the behavior of a population in relation to one or more variables, or describe the characteristics of variables to be measured ( descriptive research questions ). 1 , 5 , 14 These questions may also aim to discover differences between groups within the context of an outcome variable ( comparative research questions ), 1 , 5 , 14 or elucidate trends and interactions among variables ( relationship research questions ). 1 , 5 We provide examples of descriptive, comparative, and relationship research questions in quantitative research in Table 2 .
Quantitative research questions | |
---|---|
Descriptive research question | |
- Measures responses of subjects to variables | |
- Presents variables to measure, analyze, or assess | |
What is the proportion of resident doctors in the hospital who have mastered ultrasonography (response of subjects to a variable) as a diagnostic technique in their clinical training? | |
Comparative research question | |
- Clarifies difference between one group with outcome variable and another group without outcome variable | |
Is there a difference in the reduction of lung metastasis in osteosarcoma patients who received the vitamin D adjunctive therapy (group with outcome variable) compared with osteosarcoma patients who did not receive the vitamin D adjunctive therapy (group without outcome variable)? | |
- Compares the effects of variables | |
How does the vitamin D analogue 22-Oxacalcitriol (variable 1) mimic the antiproliferative activity of 1,25-Dihydroxyvitamin D (variable 2) in osteosarcoma cells? | |
Relationship research question | |
- Defines trends, association, relationships, or interactions between dependent variable and independent variable | |
Is there a relationship between the number of medical student suicide (dependent variable) and the level of medical student stress (independent variable) in Japan during the first wave of the COVID-19 pandemic? |
Hypotheses in quantitative research
In quantitative research, hypotheses predict the expected relationships among variables. 15 Relationships among variables that can be predicted include 1) between a single dependent variable and a single independent variable ( simple hypothesis ) or 2) between two or more independent and dependent variables ( complex hypothesis ). 4 , 11 Hypotheses may also specify the expected direction to be followed and imply an intellectual commitment to a particular outcome ( directional hypothesis ) 4 . On the other hand, hypotheses may not predict the exact direction and are used in the absence of a theory, or when findings contradict previous studies ( non-directional hypothesis ). 4 In addition, hypotheses can 1) define interdependency between variables ( associative hypothesis ), 4 2) propose an effect on the dependent variable from manipulation of the independent variable ( causal hypothesis ), 4 3) state a negative relationship between two variables ( null hypothesis ), 4 , 11 , 15 4) replace the working hypothesis if rejected ( alternative hypothesis ), 15 explain the relationship of phenomena to possibly generate a theory ( working hypothesis ), 11 5) involve quantifiable variables that can be tested statistically ( statistical hypothesis ), 11 6) or express a relationship whose interlinks can be verified logically ( logical hypothesis ). 11 We provide examples of simple, complex, directional, non-directional, associative, causal, null, alternative, working, statistical, and logical hypotheses in quantitative research, as well as the definition of quantitative hypothesis-testing research in Table 3 .
Quantitative research hypotheses | |
---|---|
Simple hypothesis | |
- Predicts relationship between single dependent variable and single independent variable | |
If the dose of the new medication (single independent variable) is high, blood pressure (single dependent variable) is lowered. | |
Complex hypothesis | |
- Foretells relationship between two or more independent and dependent variables | |
The higher the use of anticancer drugs, radiation therapy, and adjunctive agents (3 independent variables), the higher would be the survival rate (1 dependent variable). | |
Directional hypothesis | |
- Identifies study direction based on theory towards particular outcome to clarify relationship between variables | |
Privately funded research projects will have a larger international scope (study direction) than publicly funded research projects. | |
Non-directional hypothesis | |
- Nature of relationship between two variables or exact study direction is not identified | |
- Does not involve a theory | |
Women and men are different in terms of helpfulness. (Exact study direction is not identified) | |
Associative hypothesis | |
- Describes variable interdependency | |
- Change in one variable causes change in another variable | |
A larger number of people vaccinated against COVID-19 in the region (change in independent variable) will reduce the region’s incidence of COVID-19 infection (change in dependent variable). | |
Causal hypothesis | |
- An effect on dependent variable is predicted from manipulation of independent variable | |
A change into a high-fiber diet (independent variable) will reduce the blood sugar level (dependent variable) of the patient. | |
Null hypothesis | |
- A negative statement indicating no relationship or difference between 2 variables | |
There is no significant difference in the severity of pulmonary metastases between the new drug (variable 1) and the current drug (variable 2). | |
Alternative hypothesis | |
- Following a null hypothesis, an alternative hypothesis predicts a relationship between 2 study variables | |
The new drug (variable 1) is better on average in reducing the level of pain from pulmonary metastasis than the current drug (variable 2). | |
Working hypothesis | |
- A hypothesis that is initially accepted for further research to produce a feasible theory | |
Dairy cows fed with concentrates of different formulations will produce different amounts of milk. | |
Statistical hypothesis | |
- Assumption about the value of population parameter or relationship among several population characteristics | |
- Validity tested by a statistical experiment or analysis | |
The mean recovery rate from COVID-19 infection (value of population parameter) is not significantly different between population 1 and population 2. | |
There is a positive correlation between the level of stress at the workplace and the number of suicides (population characteristics) among working people in Japan. | |
Logical hypothesis | |
- Offers or proposes an explanation with limited or no extensive evidence | |
If healthcare workers provide more educational programs about contraception methods, the number of adolescent pregnancies will be less. | |
Hypothesis-testing (Quantitative hypothesis-testing research) | |
- Quantitative research uses deductive reasoning. | |
- This involves the formation of a hypothesis, collection of data in the investigation of the problem, analysis and use of the data from the investigation, and drawing of conclusions to validate or nullify the hypotheses. |
Research questions in qualitative research
Unlike research questions in quantitative research, research questions in qualitative research are usually continuously reviewed and reformulated. The central question and associated subquestions are stated more than the hypotheses. 15 The central question broadly explores a complex set of factors surrounding the central phenomenon, aiming to present the varied perspectives of participants. 15
There are varied goals for which qualitative research questions are developed. These questions can function in several ways, such as to 1) identify and describe existing conditions ( contextual research question s); 2) describe a phenomenon ( descriptive research questions ); 3) assess the effectiveness of existing methods, protocols, theories, or procedures ( evaluation research questions ); 4) examine a phenomenon or analyze the reasons or relationships between subjects or phenomena ( explanatory research questions ); or 5) focus on unknown aspects of a particular topic ( exploratory research questions ). 5 In addition, some qualitative research questions provide new ideas for the development of theories and actions ( generative research questions ) or advance specific ideologies of a position ( ideological research questions ). 1 Other qualitative research questions may build on a body of existing literature and become working guidelines ( ethnographic research questions ). Research questions may also be broadly stated without specific reference to the existing literature or a typology of questions ( phenomenological research questions ), may be directed towards generating a theory of some process ( grounded theory questions ), or may address a description of the case and the emerging themes ( qualitative case study questions ). 15 We provide examples of contextual, descriptive, evaluation, explanatory, exploratory, generative, ideological, ethnographic, phenomenological, grounded theory, and qualitative case study research questions in qualitative research in Table 4 , and the definition of qualitative hypothesis-generating research in Table 5 .
Qualitative research questions | |
---|---|
Contextual research question | |
- Ask the nature of what already exists | |
- Individuals or groups function to further clarify and understand the natural context of real-world problems | |
What are the experiences of nurses working night shifts in healthcare during the COVID-19 pandemic? (natural context of real-world problems) | |
Descriptive research question | |
- Aims to describe a phenomenon | |
What are the different forms of disrespect and abuse (phenomenon) experienced by Tanzanian women when giving birth in healthcare facilities? | |
Evaluation research question | |
- Examines the effectiveness of existing practice or accepted frameworks | |
How effective are decision aids (effectiveness of existing practice) in helping decide whether to give birth at home or in a healthcare facility? | |
Explanatory research question | |
- Clarifies a previously studied phenomenon and explains why it occurs | |
Why is there an increase in teenage pregnancy (phenomenon) in Tanzania? | |
Exploratory research question | |
- Explores areas that have not been fully investigated to have a deeper understanding of the research problem | |
What factors affect the mental health of medical students (areas that have not yet been fully investigated) during the COVID-19 pandemic? | |
Generative research question | |
- Develops an in-depth understanding of people’s behavior by asking ‘how would’ or ‘what if’ to identify problems and find solutions | |
How would the extensive research experience of the behavior of new staff impact the success of the novel drug initiative? | |
Ideological research question | |
- Aims to advance specific ideas or ideologies of a position | |
Are Japanese nurses who volunteer in remote African hospitals able to promote humanized care of patients (specific ideas or ideologies) in the areas of safe patient environment, respect of patient privacy, and provision of accurate information related to health and care? | |
Ethnographic research question | |
- Clarifies peoples’ nature, activities, their interactions, and the outcomes of their actions in specific settings | |
What are the demographic characteristics, rehabilitative treatments, community interactions, and disease outcomes (nature, activities, their interactions, and the outcomes) of people in China who are suffering from pneumoconiosis? | |
Phenomenological research question | |
- Knows more about the phenomena that have impacted an individual | |
What are the lived experiences of parents who have been living with and caring for children with a diagnosis of autism? (phenomena that have impacted an individual) | |
Grounded theory question | |
- Focuses on social processes asking about what happens and how people interact, or uncovering social relationships and behaviors of groups | |
What are the problems that pregnant adolescents face in terms of social and cultural norms (social processes), and how can these be addressed? | |
Qualitative case study question | |
- Assesses a phenomenon using different sources of data to answer “why” and “how” questions | |
- Considers how the phenomenon is influenced by its contextual situation. | |
How does quitting work and assuming the role of a full-time mother (phenomenon assessed) change the lives of women in Japan? |
Qualitative research hypotheses | |
---|---|
Hypothesis-generating (Qualitative hypothesis-generating research) | |
- Qualitative research uses inductive reasoning. | |
- This involves data collection from study participants or the literature regarding a phenomenon of interest, using the collected data to develop a formal hypothesis, and using the formal hypothesis as a framework for testing the hypothesis. | |
- Qualitative exploratory studies explore areas deeper, clarifying subjective experience and allowing formulation of a formal hypothesis potentially testable in a future quantitative approach. |
Qualitative studies usually pose at least one central research question and several subquestions starting with How or What . These research questions use exploratory verbs such as explore or describe . These also focus on one central phenomenon of interest, and may mention the participants and research site. 15
Hypotheses in qualitative research
Hypotheses in qualitative research are stated in the form of a clear statement concerning the problem to be investigated. Unlike in quantitative research where hypotheses are usually developed to be tested, qualitative research can lead to both hypothesis-testing and hypothesis-generating outcomes. 2 When studies require both quantitative and qualitative research questions, this suggests an integrative process between both research methods wherein a single mixed-methods research question can be developed. 1
FRAMEWORKS FOR DEVELOPING RESEARCH QUESTIONS AND HYPOTHESES
Research questions followed by hypotheses should be developed before the start of the study. 1 , 12 , 14 It is crucial to develop feasible research questions on a topic that is interesting to both the researcher and the scientific community. This can be achieved by a meticulous review of previous and current studies to establish a novel topic. Specific areas are subsequently focused on to generate ethical research questions. The relevance of the research questions is evaluated in terms of clarity of the resulting data, specificity of the methodology, objectivity of the outcome, depth of the research, and impact of the study. 1 , 5 These aspects constitute the FINER criteria (i.e., Feasible, Interesting, Novel, Ethical, and Relevant). 1 Clarity and effectiveness are achieved if research questions meet the FINER criteria. In addition to the FINER criteria, Ratan et al. described focus, complexity, novelty, feasibility, and measurability for evaluating the effectiveness of research questions. 14
The PICOT and PEO frameworks are also used when developing research questions. 1 The following elements are addressed in these frameworks, PICOT: P-population/patients/problem, I-intervention or indicator being studied, C-comparison group, O-outcome of interest, and T-timeframe of the study; PEO: P-population being studied, E-exposure to preexisting conditions, and O-outcome of interest. 1 Research questions are also considered good if these meet the “FINERMAPS” framework: Feasible, Interesting, Novel, Ethical, Relevant, Manageable, Appropriate, Potential value/publishable, and Systematic. 14
As we indicated earlier, research questions and hypotheses that are not carefully formulated result in unethical studies or poor outcomes. To illustrate this, we provide some examples of ambiguous research question and hypotheses that result in unclear and weak research objectives in quantitative research ( Table 6 ) 16 and qualitative research ( Table 7 ) 17 , and how to transform these ambiguous research question(s) and hypothesis(es) into clear and good statements.
Variables | Unclear and weak statement (Statement 1) | Clear and good statement (Statement 2) | Points to avoid |
---|---|---|---|
Research question | Which is more effective between smoke moxibustion and smokeless moxibustion? | “Moreover, regarding smoke moxibustion versus smokeless moxibustion, it remains unclear which is more effective, safe, and acceptable to pregnant women, and whether there is any difference in the amount of heat generated.” | 1) Vague and unfocused questions |
2) Closed questions simply answerable by yes or no | |||
3) Questions requiring a simple choice | |||
Hypothesis | The smoke moxibustion group will have higher cephalic presentation. | “Hypothesis 1. The smoke moxibustion stick group (SM group) and smokeless moxibustion stick group (-SLM group) will have higher rates of cephalic presentation after treatment than the control group. | 1) Unverifiable hypotheses |
Hypothesis 2. The SM group and SLM group will have higher rates of cephalic presentation at birth than the control group. | 2) Incompletely stated groups of comparison | ||
Hypothesis 3. There will be no significant differences in the well-being of the mother and child among the three groups in terms of the following outcomes: premature birth, premature rupture of membranes (PROM) at < 37 weeks, Apgar score < 7 at 5 min, umbilical cord blood pH < 7.1, admission to neonatal intensive care unit (NICU), and intrauterine fetal death.” | 3) Insufficiently described variables or outcomes | ||
Research objective | To determine which is more effective between smoke moxibustion and smokeless moxibustion. | “The specific aims of this pilot study were (a) to compare the effects of smoke moxibustion and smokeless moxibustion treatments with the control group as a possible supplement to ECV for converting breech presentation to cephalic presentation and increasing adherence to the newly obtained cephalic position, and (b) to assess the effects of these treatments on the well-being of the mother and child.” | 1) Poor understanding of the research question and hypotheses |
2) Insufficient description of population, variables, or study outcomes |
a These statements were composed for comparison and illustrative purposes only.
b These statements are direct quotes from Higashihara and Horiuchi. 16
Variables | Unclear and weak statement (Statement 1) | Clear and good statement (Statement 2) | Points to avoid |
---|---|---|---|
Research question | Does disrespect and abuse (D&A) occur in childbirth in Tanzania? | How does disrespect and abuse (D&A) occur and what are the types of physical and psychological abuses observed in midwives’ actual care during facility-based childbirth in urban Tanzania? | 1) Ambiguous or oversimplistic questions |
2) Questions unverifiable by data collection and analysis | |||
Hypothesis | Disrespect and abuse (D&A) occur in childbirth in Tanzania. | Hypothesis 1: Several types of physical and psychological abuse by midwives in actual care occur during facility-based childbirth in urban Tanzania. | 1) Statements simply expressing facts |
Hypothesis 2: Weak nursing and midwifery management contribute to the D&A of women during facility-based childbirth in urban Tanzania. | 2) Insufficiently described concepts or variables | ||
Research objective | To describe disrespect and abuse (D&A) in childbirth in Tanzania. | “This study aimed to describe from actual observations the respectful and disrespectful care received by women from midwives during their labor period in two hospitals in urban Tanzania.” | 1) Statements unrelated to the research question and hypotheses |
2) Unattainable or unexplorable objectives |
a This statement is a direct quote from Shimoda et al. 17
The other statements were composed for comparison and illustrative purposes only.
CONSTRUCTING RESEARCH QUESTIONS AND HYPOTHESES
To construct effective research questions and hypotheses, it is very important to 1) clarify the background and 2) identify the research problem at the outset of the research, within a specific timeframe. 9 Then, 3) review or conduct preliminary research to collect all available knowledge about the possible research questions by studying theories and previous studies. 18 Afterwards, 4) construct research questions to investigate the research problem. Identify variables to be accessed from the research questions 4 and make operational definitions of constructs from the research problem and questions. Thereafter, 5) construct specific deductive or inductive predictions in the form of hypotheses. 4 Finally, 6) state the study aims . This general flow for constructing effective research questions and hypotheses prior to conducting research is shown in Fig. 1 .

Research questions are used more frequently in qualitative research than objectives or hypotheses. 3 These questions seek to discover, understand, explore or describe experiences by asking “What” or “How.” The questions are open-ended to elicit a description rather than to relate variables or compare groups. The questions are continually reviewed, reformulated, and changed during the qualitative study. 3 Research questions are also used more frequently in survey projects than hypotheses in experiments in quantitative research to compare variables and their relationships.
Hypotheses are constructed based on the variables identified and as an if-then statement, following the template, ‘If a specific action is taken, then a certain outcome is expected.’ At this stage, some ideas regarding expectations from the research to be conducted must be drawn. 18 Then, the variables to be manipulated (independent) and influenced (dependent) are defined. 4 Thereafter, the hypothesis is stated and refined, and reproducible data tailored to the hypothesis are identified, collected, and analyzed. 4 The hypotheses must be testable and specific, 18 and should describe the variables and their relationships, the specific group being studied, and the predicted research outcome. 18 Hypotheses construction involves a testable proposition to be deduced from theory, and independent and dependent variables to be separated and measured separately. 3 Therefore, good hypotheses must be based on good research questions constructed at the start of a study or trial. 12
In summary, research questions are constructed after establishing the background of the study. Hypotheses are then developed based on the research questions. Thus, it is crucial to have excellent research questions to generate superior hypotheses. In turn, these would determine the research objectives and the design of the study, and ultimately, the outcome of the research. 12 Algorithms for building research questions and hypotheses are shown in Fig. 2 for quantitative research and in Fig. 3 for qualitative research.

EXAMPLES OF RESEARCH QUESTIONS FROM PUBLISHED ARTICLES
- EXAMPLE 1. Descriptive research question (quantitative research)
- - Presents research variables to be assessed (distinct phenotypes and subphenotypes)
- “BACKGROUND: Since COVID-19 was identified, its clinical and biological heterogeneity has been recognized. Identifying COVID-19 phenotypes might help guide basic, clinical, and translational research efforts.
- RESEARCH QUESTION: Does the clinical spectrum of patients with COVID-19 contain distinct phenotypes and subphenotypes? ” 19
- EXAMPLE 2. Relationship research question (quantitative research)
- - Shows interactions between dependent variable (static postural control) and independent variable (peripheral visual field loss)
- “Background: Integration of visual, vestibular, and proprioceptive sensations contributes to postural control. People with peripheral visual field loss have serious postural instability. However, the directional specificity of postural stability and sensory reweighting caused by gradual peripheral visual field loss remain unclear.
- Research question: What are the effects of peripheral visual field loss on static postural control ?” 20
- EXAMPLE 3. Comparative research question (quantitative research)
- - Clarifies the difference among groups with an outcome variable (patients enrolled in COMPERA with moderate PH or severe PH in COPD) and another group without the outcome variable (patients with idiopathic pulmonary arterial hypertension (IPAH))
- “BACKGROUND: Pulmonary hypertension (PH) in COPD is a poorly investigated clinical condition.
- RESEARCH QUESTION: Which factors determine the outcome of PH in COPD?
- STUDY DESIGN AND METHODS: We analyzed the characteristics and outcome of patients enrolled in the Comparative, Prospective Registry of Newly Initiated Therapies for Pulmonary Hypertension (COMPERA) with moderate or severe PH in COPD as defined during the 6th PH World Symposium who received medical therapy for PH and compared them with patients with idiopathic pulmonary arterial hypertension (IPAH) .” 21
- EXAMPLE 4. Exploratory research question (qualitative research)
- - Explores areas that have not been fully investigated (perspectives of families and children who receive care in clinic-based child obesity treatment) to have a deeper understanding of the research problem
- “Problem: Interventions for children with obesity lead to only modest improvements in BMI and long-term outcomes, and data are limited on the perspectives of families of children with obesity in clinic-based treatment. This scoping review seeks to answer the question: What is known about the perspectives of families and children who receive care in clinic-based child obesity treatment? This review aims to explore the scope of perspectives reported by families of children with obesity who have received individualized outpatient clinic-based obesity treatment.” 22
- EXAMPLE 5. Relationship research question (quantitative research)
- - Defines interactions between dependent variable (use of ankle strategies) and independent variable (changes in muscle tone)
- “Background: To maintain an upright standing posture against external disturbances, the human body mainly employs two types of postural control strategies: “ankle strategy” and “hip strategy.” While it has been reported that the magnitude of the disturbance alters the use of postural control strategies, it has not been elucidated how the level of muscle tone, one of the crucial parameters of bodily function, determines the use of each strategy. We have previously confirmed using forward dynamics simulations of human musculoskeletal models that an increased muscle tone promotes the use of ankle strategies. The objective of the present study was to experimentally evaluate a hypothesis: an increased muscle tone promotes the use of ankle strategies. Research question: Do changes in the muscle tone affect the use of ankle strategies ?” 23
EXAMPLES OF HYPOTHESES IN PUBLISHED ARTICLES
- EXAMPLE 1. Working hypothesis (quantitative research)
- - A hypothesis that is initially accepted for further research to produce a feasible theory
- “As fever may have benefit in shortening the duration of viral illness, it is plausible to hypothesize that the antipyretic efficacy of ibuprofen may be hindering the benefits of a fever response when taken during the early stages of COVID-19 illness .” 24
- “In conclusion, it is plausible to hypothesize that the antipyretic efficacy of ibuprofen may be hindering the benefits of a fever response . The difference in perceived safety of these agents in COVID-19 illness could be related to the more potent efficacy to reduce fever with ibuprofen compared to acetaminophen. Compelling data on the benefit of fever warrant further research and review to determine when to treat or withhold ibuprofen for early stage fever for COVID-19 and other related viral illnesses .” 24
- EXAMPLE 2. Exploratory hypothesis (qualitative research)
- - Explores particular areas deeper to clarify subjective experience and develop a formal hypothesis potentially testable in a future quantitative approach
- “We hypothesized that when thinking about a past experience of help-seeking, a self distancing prompt would cause increased help-seeking intentions and more favorable help-seeking outcome expectations .” 25
- “Conclusion
- Although a priori hypotheses were not supported, further research is warranted as results indicate the potential for using self-distancing approaches to increasing help-seeking among some people with depressive symptomatology.” 25
- EXAMPLE 3. Hypothesis-generating research to establish a framework for hypothesis testing (qualitative research)
- “We hypothesize that compassionate care is beneficial for patients (better outcomes), healthcare systems and payers (lower costs), and healthcare providers (lower burnout). ” 26
- Compassionomics is the branch of knowledge and scientific study of the effects of compassionate healthcare. Our main hypotheses are that compassionate healthcare is beneficial for (1) patients, by improving clinical outcomes, (2) healthcare systems and payers, by supporting financial sustainability, and (3) HCPs, by lowering burnout and promoting resilience and well-being. The purpose of this paper is to establish a scientific framework for testing the hypotheses above . If these hypotheses are confirmed through rigorous research, compassionomics will belong in the science of evidence-based medicine, with major implications for all healthcare domains.” 26
- EXAMPLE 4. Statistical hypothesis (quantitative research)
- - An assumption is made about the relationship among several population characteristics ( gender differences in sociodemographic and clinical characteristics of adults with ADHD ). Validity is tested by statistical experiment or analysis ( chi-square test, Students t-test, and logistic regression analysis)
- “Our research investigated gender differences in sociodemographic and clinical characteristics of adults with ADHD in a Japanese clinical sample. Due to unique Japanese cultural ideals and expectations of women's behavior that are in opposition to ADHD symptoms, we hypothesized that women with ADHD experience more difficulties and present more dysfunctions than men . We tested the following hypotheses: first, women with ADHD have more comorbidities than men with ADHD; second, women with ADHD experience more social hardships than men, such as having less full-time employment and being more likely to be divorced.” 27
- “Statistical Analysis
- ( text omitted ) Between-gender comparisons were made using the chi-squared test for categorical variables and Students t-test for continuous variables…( text omitted ). A logistic regression analysis was performed for employment status, marital status, and comorbidity to evaluate the independent effects of gender on these dependent variables.” 27
EXAMPLES OF HYPOTHESIS AS WRITTEN IN PUBLISHED ARTICLES IN RELATION TO OTHER PARTS
- EXAMPLE 1. Background, hypotheses, and aims are provided
- “Pregnant women need skilled care during pregnancy and childbirth, but that skilled care is often delayed in some countries …( text omitted ). The focused antenatal care (FANC) model of WHO recommends that nurses provide information or counseling to all pregnant women …( text omitted ). Job aids are visual support materials that provide the right kind of information using graphics and words in a simple and yet effective manner. When nurses are not highly trained or have many work details to attend to, these job aids can serve as a content reminder for the nurses and can be used for educating their patients (Jennings, Yebadokpo, Affo, & Agbogbe, 2010) ( text omitted ). Importantly, additional evidence is needed to confirm how job aids can further improve the quality of ANC counseling by health workers in maternal care …( text omitted )” 28
- “ This has led us to hypothesize that the quality of ANC counseling would be better if supported by job aids. Consequently, a better quality of ANC counseling is expected to produce higher levels of awareness concerning the danger signs of pregnancy and a more favorable impression of the caring behavior of nurses .” 28
- “This study aimed to examine the differences in the responses of pregnant women to a job aid-supported intervention during ANC visit in terms of 1) their understanding of the danger signs of pregnancy and 2) their impression of the caring behaviors of nurses to pregnant women in rural Tanzania.” 28
- EXAMPLE 2. Background, hypotheses, and aims are provided
- “We conducted a two-arm randomized controlled trial (RCT) to evaluate and compare changes in salivary cortisol and oxytocin levels of first-time pregnant women between experimental and control groups. The women in the experimental group touched and held an infant for 30 min (experimental intervention protocol), whereas those in the control group watched a DVD movie of an infant (control intervention protocol). The primary outcome was salivary cortisol level and the secondary outcome was salivary oxytocin level.” 29
- “ We hypothesize that at 30 min after touching and holding an infant, the salivary cortisol level will significantly decrease and the salivary oxytocin level will increase in the experimental group compared with the control group .” 29
- EXAMPLE 3. Background, aim, and hypothesis are provided
- “In countries where the maternal mortality ratio remains high, antenatal education to increase Birth Preparedness and Complication Readiness (BPCR) is considered one of the top priorities [1]. BPCR includes birth plans during the antenatal period, such as the birthplace, birth attendant, transportation, health facility for complications, expenses, and birth materials, as well as family coordination to achieve such birth plans. In Tanzania, although increasing, only about half of all pregnant women attend an antenatal clinic more than four times [4]. Moreover, the information provided during antenatal care (ANC) is insufficient. In the resource-poor settings, antenatal group education is a potential approach because of the limited time for individual counseling at antenatal clinics.” 30
- “This study aimed to evaluate an antenatal group education program among pregnant women and their families with respect to birth-preparedness and maternal and infant outcomes in rural villages of Tanzania.” 30
- “ The study hypothesis was if Tanzanian pregnant women and their families received a family-oriented antenatal group education, they would (1) have a higher level of BPCR, (2) attend antenatal clinic four or more times, (3) give birth in a health facility, (4) have less complications of women at birth, and (5) have less complications and deaths of infants than those who did not receive the education .” 30
Research questions and hypotheses are crucial components to any type of research, whether quantitative or qualitative. These questions should be developed at the very beginning of the study. Excellent research questions lead to superior hypotheses, which, like a compass, set the direction of research, and can often determine the successful conduct of the study. Many research studies have floundered because the development of research questions and subsequent hypotheses was not given the thought and meticulous attention needed. The development of research questions and hypotheses is an iterative process based on extensive knowledge of the literature and insightful grasp of the knowledge gap. Focused, concise, and specific research questions provide a strong foundation for constructing hypotheses which serve as formal predictions about the research outcomes. Research questions and hypotheses are crucial elements of research that should not be overlooked. They should be carefully thought of and constructed when planning research. This avoids unethical studies and poor outcomes by defining well-founded objectives that determine the design, course, and outcome of the study.
Disclosure: The authors have no potential conflicts of interest to disclose.
Author Contributions:
- Conceptualization: Barroga E, Matanguihan GJ.
- Methodology: Barroga E, Matanguihan GJ.
- Writing - original draft: Barroga E, Matanguihan GJ.
- Writing - review & editing: Barroga E, Matanguihan GJ.
Stack Exchange Network
Stack Exchange network consists of 183 Q&A communities including Stack Overflow , the largest, most trusted online community for developers to learn, share their knowledge, and build their careers.
Q&A for work
Connect and share knowledge within a single location that is structured and easy to search.
Directional research hypotheses vs. directional hypothesis testing
When I took a methods course, I was told to avoid making a directional hypothesis. I understand the reason. Now however, I often see hypotheses like these: "We hypothesized that reaction time will decrease due to the treatment". It's rare to read research papers that have non-directional hypotheses (at least in my limited readings). If the researcher makes a directional hypothesis, I would assume that he will need to run a one-tailed test. From what I remember, if the results go the opposite direction--i.e., reaction time increases--our researcher failed to reject the null.
What if an ANOVA is used instead? Does that negate the directionality of the hypothesis? From my understanding, the ANOVA is a non-directional test.
On a related note, would rewording the hypothesis to "we hypothesized that reaction time may decrease due to the treatment" make it non-directional? Do researchers "change" their hypothesis after the fact to make it non-directional because they found an opposite effect? Sounds rather unethical to me.
- hypothesis-testing

2 Answers 2
The connection between the research hypothesis and the choice of null an alternative is not writ in stone. I can't see any particular reason why one could not say (just casting your phrase in plain English because that way I won't get tangled up):
"We think the treatment should reduce reaction time" ...
... but then formulate a two-sided alternative, if that was appropriate. I don't think any great song and dance is required to use a two-tailed test if you're clear that you want your hypothesis test to have power in both tails.
That is, I see no problem with discussing the properties of the hypothesis test as if the alternative were not the same thing as your research hypothesis, and then simply interpreting the results of the test back in terms of the research hypothesis.
Of course, I don't control how pointlessly dogmatic any particular journal, editor or referee may be. [Indeed, in my experience, my thoughts seems rarely to influence people whose mind is set on something being the case.]
The same attitude carries through to ANOVA; it's not 'saving' you, since a multigroup test can be made "directional" (in an ANOVA-like situation, whether or not you still call it ANOVA) --
With one-factor comparisons ($k$ groups), you have $k!$ possible orderings of the means. If you are interested in some particular ordered alternative, you can specify it clearly up front and simply use a test sensitive to that alternative (you could specify a contrast, for example, though there are other approaches to ordered alternatives).
So if a research hypothesis was "forcing" you to do one tailed, it would, I think, equally "force" you to do some equivalent with more groups, since that's possible.

It is usual to run two tailed tests even if your hypothesis is pretty much directional, at least in psychology.
For one thing, running a one tailed test limits your ability to be surprised.
Changing your hypothesis after the data has been collected is cheating.
Your Answer
Sign up or log in, post as a guest.
Required, but never shown
By clicking “Post Your Answer”, you agree to our terms of service and acknowledge you have read our privacy policy .
Not the answer you're looking for? Browse other questions tagged hypothesis-testing anova or ask your own question .
- Featured on Meta
- We've made changes to our Terms of Service & Privacy Policy - July 2024
- Bringing clarity to status tag usage on meta sites
Hot Network Questions
- Would it take less thrust overall to put an object into higher orbit?
- Passport validity when entering Israel
- C - mini string lib
- Can a "sharp turn" on a trace with an SMD resistor also present a risk of reflection?
- One IO to control two LEDs. When one is lit, the other is not
- How do we know for sure that the dedekind axiom is logically independent of the other axioms?
- Visualizing histogram of data on unit circle?
- Reference request: "Higher order eigentuples" as generalized eigenvectors?
- Looking for source of story about returning a lost object
- GPLv3 obligations
- Who said "If you don't do politics, politics will do you"?
- Fitting 10 pieces of pizza in a box
- Is it possible to add custom Social Media accounts to contacts in the Contacts app?
- Does my AC vent air from the room to outside?
- \includegraphics not reading \newcommand
- python stats.spearmanr and R cor.test(method='spearman') don't return the same p-value?
- Home water pressure higher than city water pressure?
- Can you successfully substitute pickled onions for baby onions in Coq Au Vin?
- Ethics application: secondary analysis of anonymous data without "future use" consent
- Direct product of direct sum of a flat module
- I am a fifteen-year-old from India planning to fly to Germany alone (without my parents accompanying me) to see my relatives.What documents do I need?
- Does the overall mAh of the battery add up when batteries are parallel?
- Is it possible for a company to dilute my shares to the point they are insignificant
- Calculate the sum of numbers in a rectangle
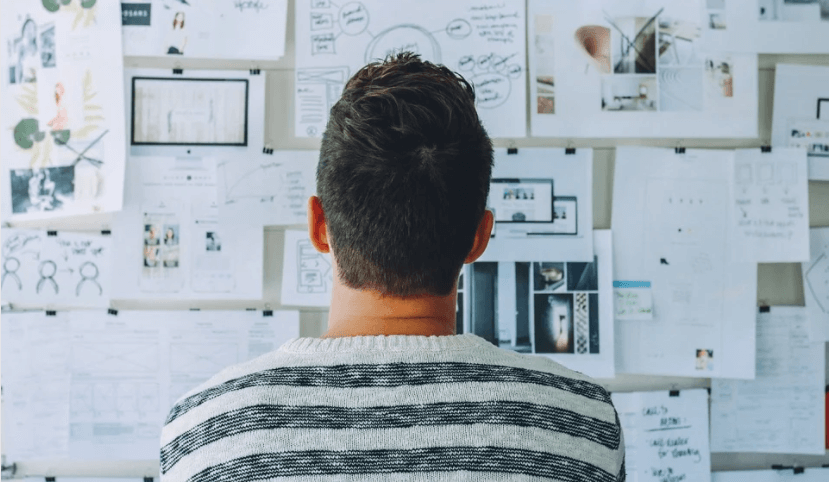
Directional vs Non-Directional Hypothesis – Collect Feedback More Effectively
To conduct a perfect survey, you should know the basics of good research . That’s why in Startquestion we would like to share with you our knowledge about basic terms connected to online surveys and feedback gathering . Knowing the basis you can create surveys and conduct research in more effective ways and thanks to this get meaningful feedback from your customers, employees, and users. That’s enough for the introduction – let’s get to work. This time we will tell you about the hypothesis .
What is a Hypothesis?
A Hypothesis can be described as a theoretical statement built upon some evidence so that it can be tested as if it is true or false. In other words, a hypothesis is a speculation or an idea, based on insufficient evidence that allows it further analysis and experimentation.
The purpose of a hypothetical statement is to work like a prediction based on studied research and to provide some estimated results before it ha happens in a real position. There can be more than one hypothesis statement involved in a research study, where you need to question and explore different aspects of a proposed research topic. Before putting your research into directional vs non-directional hypotheses, let’s have some basic knowledge.
Most often, a hypothesis describes a relation between two or more variables. It includes:
An Independent variable – One that is controlled by the researcher
Dependent Variable – The variable that the researcher observes in association with the Independent variable.
Try one of the best survey tools for free!
Start trial period without any credit card or subscription. Easily conduct your research and gather feedback via link, social media, email, and more.
Create first survey
No credit card required · Cancel any time · GDRP Compilant
How to write an effective Hypothesis?
To write an effective hypothesis follow these essential steps.
- Inquire a Question
The very first step in writing an effective hypothesis is raising a question. Outline the research question very carefully keeping your research purpose in mind. Build it in a precise and targeted way. Here you must be clear about the research question vs hypothesis. A research question is the very beginning point of writing an effective hypothesis.
Do Literature Review
Once you are done with constructing your research question, you can start the literature review. A literature review is a collection of preliminary research studies done on the same or relevant topics. There is a diversified range of literature reviews. The most common ones are academic journals but it is not confined to that. It can be anything including your research, data collection, and observation.
At this point, you can build a conceptual framework. It can be defined as a visual representation of the estimated relationship between two variables subjected to research.
Frame an Answer
After a collection of literature reviews, you can find ways how to answer the question. Expect this stage as a point where you will be able to make a stand upon what you believe might have the exact outcome of your research. You must formulate this answer statement clearly and concisely.
Build a Hypothesis
At this point, you can firmly build your hypothesis. By now, you knew the answer to your question so make a hypothesis that includes:
- Applicable Variables
- Particular Group being Studied (Who/What)
- Probable Outcome of the Experiment
Remember, your hypothesis is a calculated assumption, it has to be constructed as a sentence, not a question. This is where research question vs hypothesis starts making sense.
Refine a Hypothesis
Make necessary amendments to the constructed hypothesis keeping in mind that it has to be targeted and provable. Moreover, you might encounter certain circumstances where you will be studying the difference between one or more groups. It can be correlational research. In such instances, you must have to testify the relationships that you believe you will find in the subject variables and through this research.
Build Null Hypothesis
Certain research studies require some statistical investigation to perform a data collection. Whenever applying any scientific method to construct a hypothesis, you must have adequate knowledge of the Null Hypothesis and an Alternative hypothesis.
Null Hypothesis:
A null Hypothesis denotes that there is no statistical relationship between the subject variables. It is applicable for a single group of variables or two groups of variables. A Null Hypothesis is denoted as an H0. This is the type of hypothesis that the researcher tries to invalidate. Some of the examples of null hypotheses are:
– Hyperactivity is not associated with eating sugar.
– All roses have an equal amount of petals.
– A person’s preference for a dress is not linked to its color.
Alternative Hypothesis:
An alternative hypothesis is a statement that is simply inverse or opposite of the null hypothesis and denoted as H1. Simply saying, it is an alternative statement for the null hypothesis. The same examples will go this way as an alternative hypothesis:
– Hyperactivity is associated with eating sugar.
– All roses do not have an equal amount of petals.
– A person’s preference for a dress is linked to its color.
Start your research right now: use professional survey templates
- Brand Awareness Survey
- Survey for the thesis
- Website Evaluation Survey
See more templates
Types of Hypothesis
Apart from null and alternative hypotheses, research hypotheses can be categorized into different types. Let’s have a look at them:
Simple Hypothesis:
This type of hypothesis is used to state a relationship between a particular independent variable and only a dependent variable.
Complex Hypothesis:
A statement that states the relationship between two or more independent variables and two or more dependent variables, is termed a complex hypothesis.
Associative and Causal Hypothesis:
This type of hypothesis involves predicting that there is a point of interdependency between two variables. It says that any kind of change in one variable will cause a change in the other one. Similarly, a casual hypothesis says that a change in the dependent variable is due to some variations in the independent variable.
Directional vs non-directional hypothesis
Directional hypothesis:.
A hypothesis that is built upon a certain directional relationship between two variables and constructed upon an already existing theory, is called a directional hypothesis. To understand more about what is directional hypothesis here is an example, Girls perform better than boys (‘better than’ shows the direction predicted)
Non-directional Hypothesis:
It involves an open-ended non-directional hypothesis that predicts that the independent variable will influence the dependent variable; however, the nature or direction of a relationship between two subject variables is not defined or clear.
For Example, there will be a difference in the performance of girls & boys (Not defining what kind of difference)
As a professional, we suggest you apply a non-directional alternative hypothesis when you are not sure of the direction of the relationship. Maybe you’re observing potential gender differences on some psychological test, but you don’t know whether men or women would have the higher ratio. Normally, this would say that you are lacking practical knowledge about the proposed variables. A directional test should be more common for tests.
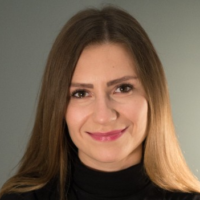
Author: Ula Kamburov-Niepewna
Updated: 18 November 2022
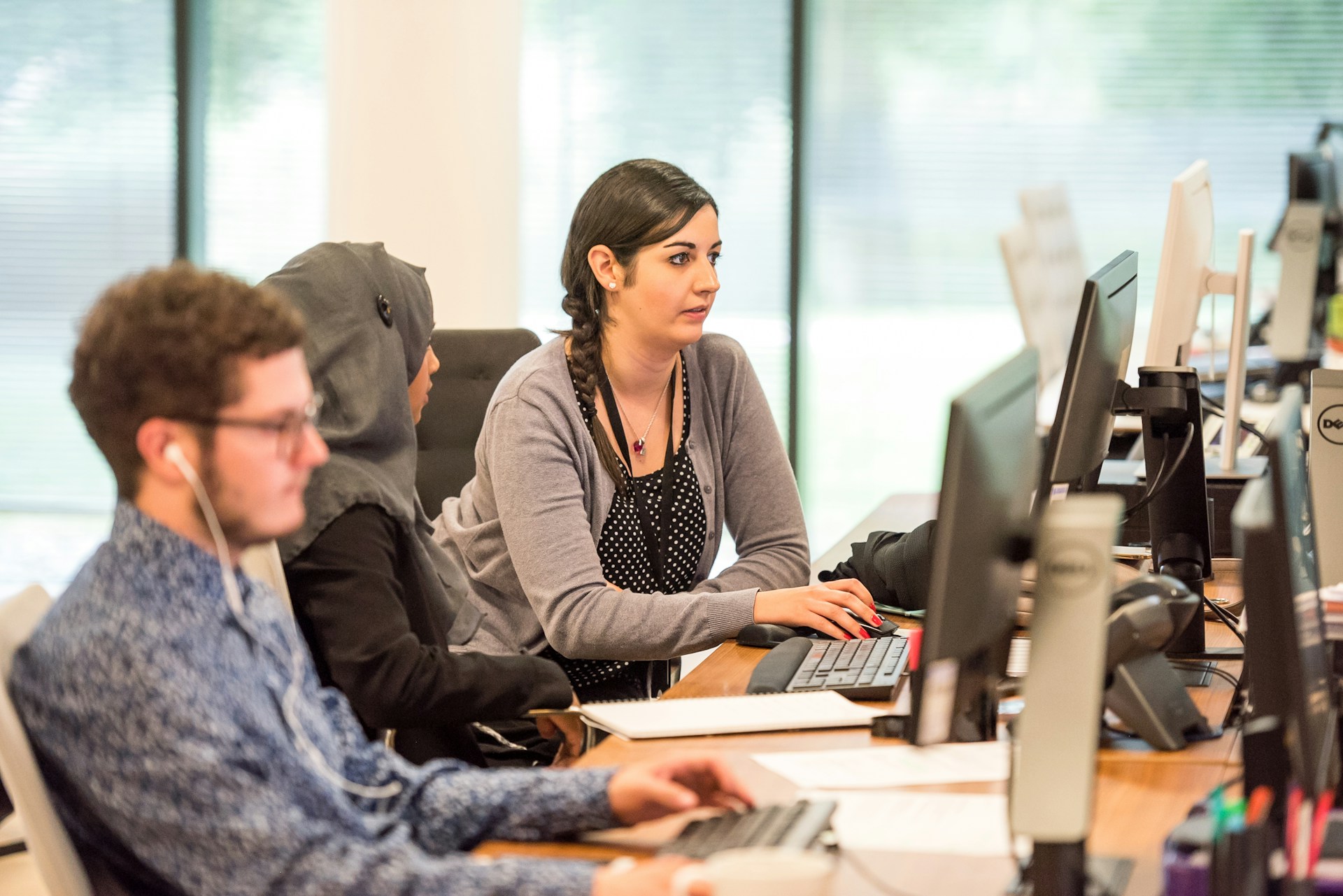
How to Build a Successful Closed-Loop Feedback System
A successful closed-loop system enhances the customer experience, drives innovation, strengthens brand loyalty, and boosts the bottom line. Learn how to harness the power of feedback, close the loop, and unlock your business's full potential.
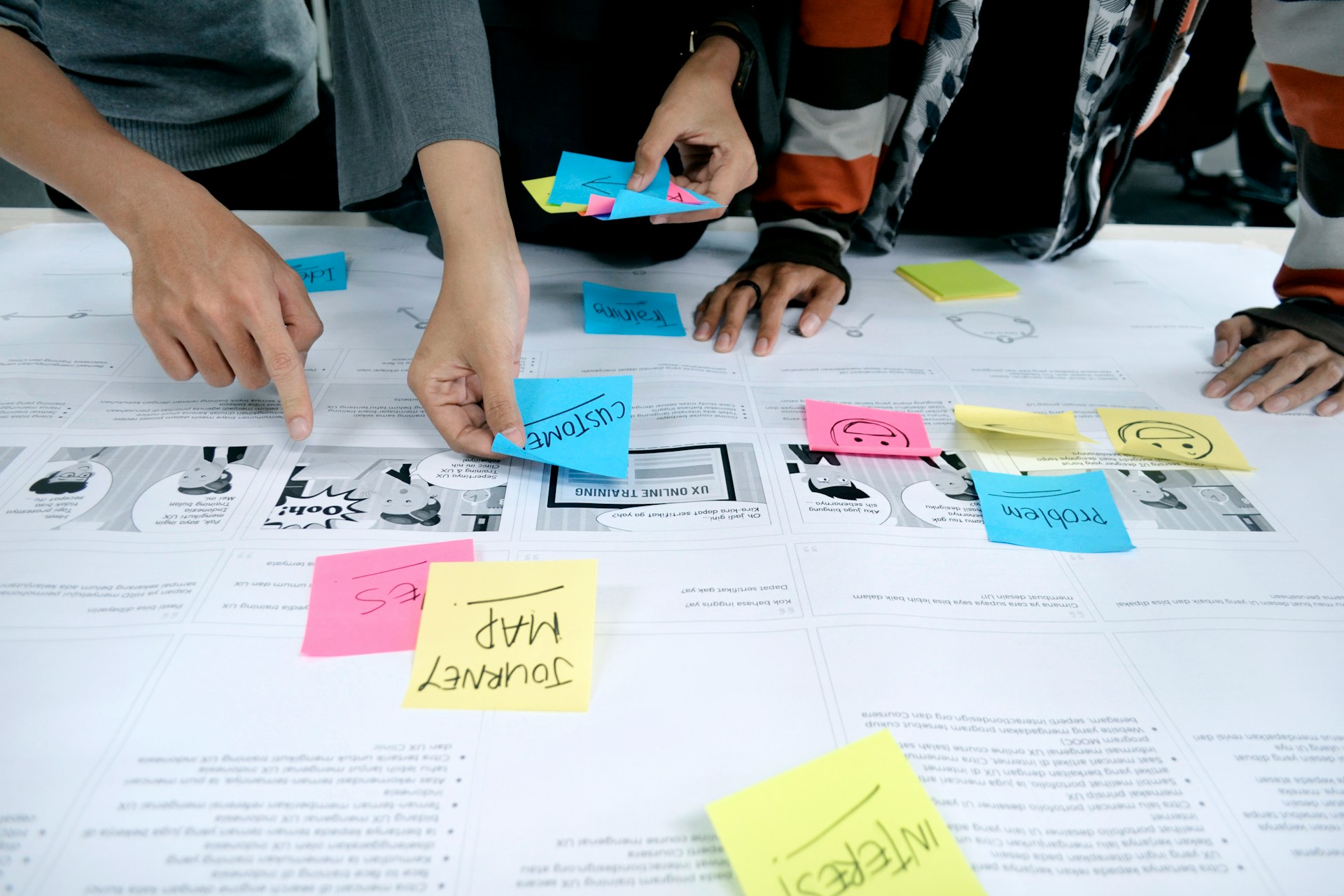
Customer Journey Map: How to Create Them Easily?
Understanding your customers' experiences can elevate your business from good to great. Customer journey mapping, a powerful visual tool, uncovers every interaction customers have with your brand, highlighting pain points and opportunities for improvement.
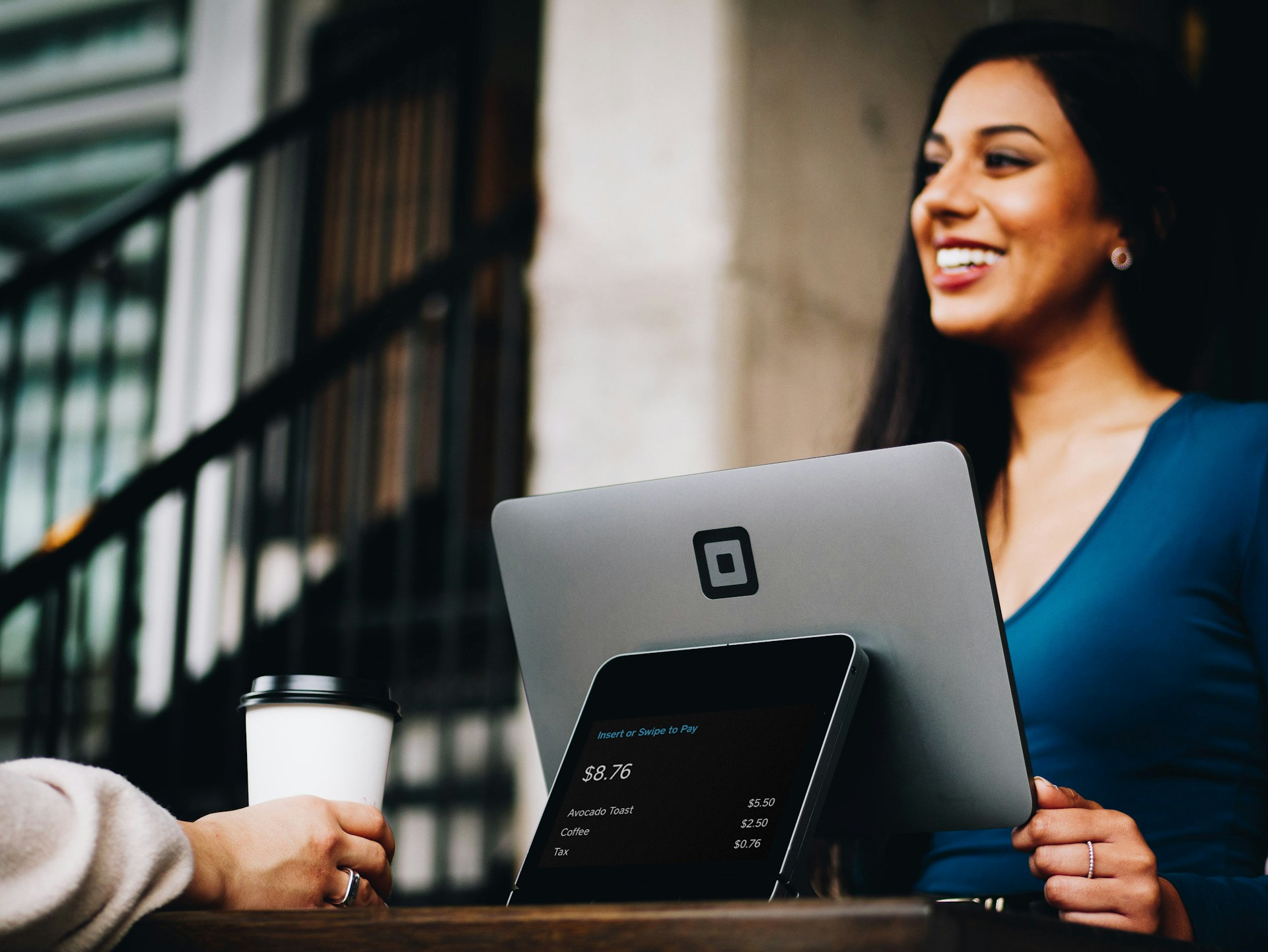
Importance and Methodology of the Voice of the Customer
Voice of the Customer (VoC) is more than just collecting feedback—it's the foundation of a client-focused strategy that can drive innovation, improve customer satisfaction, and gain a competitive advantage.
Thank you for visiting nature.com. You are using a browser version with limited support for CSS. To obtain the best experience, we recommend you use a more up to date browser (or turn off compatibility mode in Internet Explorer). In the meantime, to ensure continued support, we are displaying the site without styles and JavaScript.
- View all journals
- Explore content
- About the journal
- Publish with us
- Sign up for alerts
- Open access
- Published: 22 August 2024
Genome-scale quantification and prediction of pathogenic stop codon readthrough by small molecules
- Ignasi Toledano 1 , 2 ,
- Fran Supek ORCID: orcid.org/0000-0002-7811-6711 1 , 3 , 4 &
- Ben Lehner ORCID: orcid.org/0000-0002-8817-1124 2 , 4 , 5 , 6
Nature Genetics ( 2024 ) Cite this article
41 Altmetric
Metrics details
- Genetics research
- Clinical trial design
Premature termination codons (PTCs) cause ~10–20% of inherited diseases and are a major mechanism of tumor suppressor gene inactivation in cancer. A general strategy to alleviate the effects of PTCs would be to promote translational readthrough. Nonsense suppression by small molecules has proven effective in diverse disease models, but translation into the clinic is hampered by ineffective readthrough of many PTCs. Here we directly tackle the challenge of defining drug efficacy by quantifying the readthrough of ~5,800 human pathogenic stop codons by eight drugs. We find that different drugs promote the readthrough of complementary subsets of PTCs defined by local sequence context. This allows us to build interpretable models that accurately predict drug-induced readthrough genome-wide, and we validate these models by quantifying endogenous stop codon readthrough. Accurate readthrough quantification and prediction will empower clinical trial design and the development of personalized nonsense suppression therapies.
Similar content being viewed by others
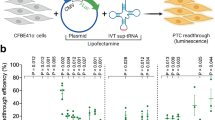
Translation velocity determines the efficacy of engineered suppressor tRNAs on pathogenic nonsense mutations
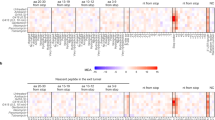
Extended stop codon context predicts nonsense codon readthrough efficiency in human cells
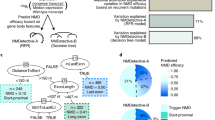
The impact of nonsense-mediated mRNA decay on genetic disease, gene editing and cancer immunotherapy
Premature termination codons (PTCs) are the cause of 10% 1 to 20% 2 of inherited diseases and an important mechanism of tumor suppressor gene inactivation in cancer. PTCs cause the production of truncated versions of proteins, which are typically loss-of-function and sometimes gain-of-function or dominant negatives. Many, but not all, PTCs also cause the degradation of mRNA transcripts by a process called nonsense-mediated mRNA decay (NMD), strongly reducing the production of the truncated protein 3 , 4 .
A general therapeutic strategy to alleviate the effects of PTCs would be to promote translational readthrough (RT) of the stop codon (Fig. 1a ). Effective nonsense suppression therapy would increase the expression of full-length proteins, reduce the production of pathological protein fragments and inhibit NMD 5 .
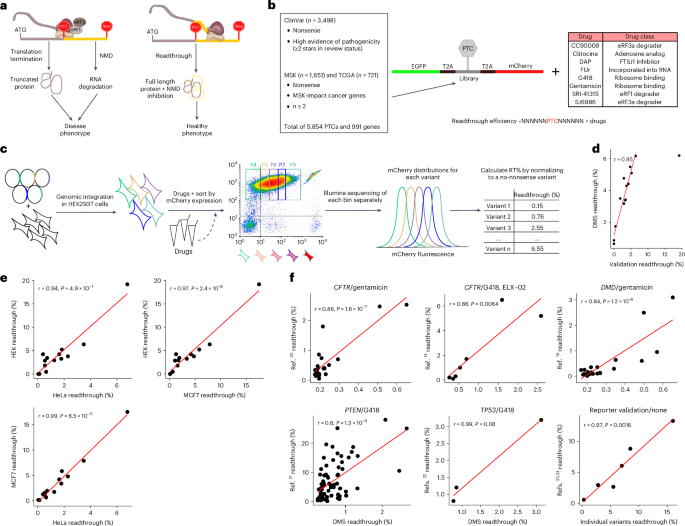
a , Readthrough drugs stimulate full-length protein synthesis and decrease NMD-mediated transcript degradation. b , Experimental design, ~5,800 nonsense variants in human genetic diseases and cancer were retrieved from ClinVar, TCGA and MSK-IMPACT datasets, cloned in a readthrough reporter, integrated into the genome of HEK293T_LP human cell line and treated with eight readthrough compounds. A readthrough efficiency value was obtained for each variant–drug pair. c , Sort-sequencing overview. Each cell integrates one copy of one variant, cells are sorted based on mCherry fluorescence ( x -axis), bins are sequenced and readthrough percentages are calculated from the mCherry distribution of reads of each variant normalized to the distribution of a no-nonsense variant. d , Deep mutational scanning (DMS) versus individual measurements Pearson’s correlation ( r = 0.95), where 15 variants spanning the whole readthrough range under SRI treatment were individually measured (Spearman correlation ( ρ ) = 0.86). e , The same 15 variants shown in d were episomally transfected in MCF7 and HeLa cells, and their readthrough percentages were correlated with HEK293T_LP’s. Pearson’s correlation and P values are shown. f , DMS Pearson’s correlation (and corresponding P values) with measurements from previous studies 14 , 16 , 20 , 21 , 22 , 23 , 24 (Spearman’s correlation ( ρ ) = 0.56, 0.93, 0.71, 0.59, 1, 0.94, from top-left to bottom-right plots). Titles indicate the gene for which nonsense variants were tested and the drug used to stimulate readthrough. The bottom-right plot does not show DMS estimates, but measurements of individual variants also tested in refs. 23 , 24 , which were used to validate the readthrough reporter. Note that the readthrough scales differ across some of the studies, illustrating how differences in the assay, conditions and reporter influence the absolute readthrough.
Source data
Multiple small-molecule drugs that promote PTC-readthrough have been discovered, with diverse mechanisms of action (MOAs) promoting the recognition of stop codons by near-cognate tRNAs rather than translation termination factors 6 . For multiple disease genes, even modest readthrough can be sufficient to alleviate disease symptoms in animal models 7 , 8 , 9 , 10 .
The extent of readthrough promoted by small molecules varies extensively for different stop codons, with most drugs increasing the readthrough of UGA more effectively than UAG and UAA PTCs 11 , 12 , 13 . Testing small numbers of mutations has identified sequence features that influence the readthrough of particular stops, for example, the presence of a cytosine in position +1 after the PTC 14 and the presence of an adenine at position −1 (ref. 15 ). To date, the largest survey of drug-induced readthrough tested the compound TLN468 on 40 variants 16 .
Here we deploy a deep mutational scanning (DMS) approach to generate much richer datasets quantifying nonsense suppression by different drugs. We measure the readthrough of ~5,800 human disease-causing PTCs for eight different readthrough-promoting compounds (henceforth referred to as drugs). We find that the drugs vary substantially in their efficacy and also in the identity of the PTCs that they most effectively promote readthrough. We identify multiple local sequence determinants that predict PTC-readthrough efficacy and show that these determinants differ across drugs. Using these sequence determinants, we are able to build models that predict readthrough efficacy by the best-performing drugs with very good performance genome-wide ( r 2 = 0.83). We make these models available as a resource to allow these drugs to be profiled for all possible PTCs in the human genome. Our data and models suggest that the design of clinical trials of nonsense suppression therapies could be improved by using patient–drug combinations that are predicted to be effective.
Quantifying readthrough of thousands of pathogenic PTCs
To quantify drug-induced readthrough of diverse PTCs, we constructed a library containing 3,498 PTCs that cause Mendelian diseases reported in ClinVar 1 , 2,372 recurrent somatic PTCs in cancer genes (721 from The Cancer Genome Atlas (TCGA) 17 plus 1,651 from MSK-IMPACT 18 ) and a TP53 control no-nonsense variant ( n = 5,871; Fig. 1b ; Methods ). We cloned each PTC with 144 nucleotides (nts) of surrounding sequence context into a dual fluorescent protein reporter, where an upstream green fluorescent protein (EGFP) controls for variable expression and readthrough causes expression of a downstream mCherry protein, and performed single-copy genomic integration into HEK293T landing pad (LP) cell line 19 . We combined fluorescence sorting and Illumina sequencing to obtain readthrough efficiencies (Fig. 1c and Extended Data Fig. 1a ), which were highly correlated across replicates (Extended Data Fig. 1b ), with individual measurements of 15 variants spanning the full dynamic range of the assay in HEK293T_LP cells ( r = 0.95, ρ = 0.86; up to the measurement saturation limit of ~6%; Fig. 1d , Extended Data Fig. 1h , Supplementary Table 1 and Supplementary Note 1 ) and in two other cell lines (MCF7 and HeLa; Fig. 1e ). Readthrough is highly correlated across variants in the three cell types ( r = 0.94–0.99), but absolute readthrough levels are about twofold lower in HeLa cells. Our measurements also correlate very well with quantifications performed in other laboratories comprising varied genes and drugs 14 , 16 , 20 , 21 , 22 , 23 , 24 (Fig. 1f ).
Readthrough varies extensively across drugs and PTCs
We tested four to six concentrations for 20 drugs reported to induce readthrough, of which eight induced reproducible readthrough in our assay (Extended Data Fig. 1c,d and Supplementary Table 2 ; Methods ). We quantified the readthrough of the library in untreated conditions and under the effect of the following eight drugs: CC90009 (refs. 25 , 26 ), clitocine 27 , 2,6-diaminopurine (DAP) 12 , gentamicin 28 , 29 , G418 (refs. 28 , 29 ), SJ6986 (ref. 25 ), SRI-41315 (refs. 30 , 31 ; henceforth: SRI) and 5-fluorouridine (FUr) 8 , which comprise different classes of small molecules spanning different MOAs (Fig. 1b , Methods ). Considering all PTCs in the library, the median readthrough varied across drugs from 0.08% (gentamicin) to 1.32% (SJ6986; Supplementary Table 3 ). However, each drug promoted a stronger readthrough of a subset of PTCs, with the median readthrough of the top 10% of variants varying from 0.51% (gentamicin) to 4.28% (DAP). Readthrough distributions were unimodal with a long upper tail for seven drugs, whereas clitocine treatment resulted in a bimodal distribution (Fig. 2a and Extended Data Fig. 1i ). In the absence of drugs, only a very small number of PTCs ( n = 17) gave >1% readthrough. Additionally, by quantifying readthrough for three SJ6986 concentrations (0.5 μM, 5 μM and 20 μM), we observed that sequence effects are preserved across drug concentrations (Extended Data Fig. 2k,l and Supplementary Note 2 ).
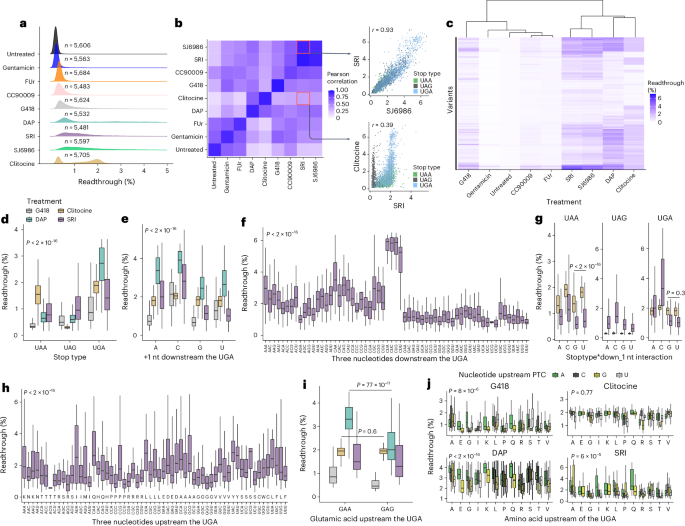
a , Readthrough distributions across drugs. The number of high-confidence variants (≥10 reads) recovered for each treatment and for which readthrough percentages were quantified is shown. b , Interdrug correlation. Correlation values between the same drug represent the inter-replicate correlation. Examples of high-correlated (SRI and SJ6986) and low-correlated (SRI and clitocine) drug pairs are shown, colored by stop type. c , Readthrough efficiencies for all variant–drug combinations. d – j , Effect of the sequence feature ( x axis) on readthrough efficiency ( y axis) in HEK293T_LP cells, colored by the drug. The top and bottom sides of the box are the upper and lower quartiles, respectively. The box covers the interquartile interval, where 50% of the data are found. The horizontal line that splits the box in two is the median. Only variants where the stop codon is UGA are shown (except for d and g , where all stop codon variants are shown). The sequence features are stop codon identity ( n = 22,342, P < 2 × 10 −16 , Kruskal–Wallis test; d ), the nucleotide in position +1 downstream of the PTC ( n = 10,602, P < 2 × 10 −16 ; e ), the nucleotides in +1, +2 and +3 positions downstream of the PTC ( n = 2,589, P < 2 × 10 −16 ; f ), same as e but stratified by stop codon (in clitocine samples U>G for UAA stops, n = 614, adjusted P < 2 × 10 −16 ; U=G for UGA stops, n = 1,395, adjusted P = 0.3; one-sided Wilcoxon signed-rank test; g ), the nucleotides in −1, −2 and −3 positions upstream of the PTC together with the amino acid encoded by each codon ( n = 2,589, P < 2 × 10 − 16 , Kruskal–Wallis test; h ) and same as h but only for variants with a glutamic acid upstream of the PTC (GAA>GAG for DAP, n = 155, adjusted P = 7 × 10 −11 ; GAA=GAG for clitocine, n = 158, adjusted P = 0.6; one-sided Wilcoxon signed-rank test; i ). Finally, the effect of amino acids encoded by A-ending codons on readthrough efficiency across drugs, where codons ended in A display higher readthrough compared to the rest of the codons ( n = 7,989, adjusted P < 6 × 10 −5 for DAP, G418 and SRI, one-sided Wilcoxon signed-rank test). The nucleotide upstream of the PTC is colored ( j ).
The readthrough profiles of the different drugs are, in most cases, only moderately correlated (Fig. 2b and Extended Data Fig. 1e ). One exception is SRI and SJ6986, which both inhibit eRF1/eRF3 (refs. 25 , 30 ) and induce readthrough of a highly correlated set of PTCs ( r = 0.93; Fig. 2b and Supplementary Note 3 ). The effects of other drugs are much more distinct. Clitocine and SRI, for example, both elicit high readthrough of many PTCs, but their effects are only weakly correlated ( r = 0.39, in comparison to the inter-replicate correlations of r = 0.94 and r = 0.96 for the two drugs). Hierarchical clustering of the readthrough profiles of all 5,837 PTCs identifies sets of PTCs with strong readthrough induced by multiple drugs as well as PTCs strongly affected by only one drug (Fig. 2c ).
Stop type and downstream sequence modulate readthrough
To better understand why the readthrough of particular PTCs is promoted by particular drugs, we quantified the association between readthrough and 47 sequence features for all drugs. These included the stop codon type, the adjacent downstream and upstream nucleotides (up to eight nucleotides away), several codon-related metrics and general features such as G + C content and RNA secondary structure propensity (Extended Data Fig. 1f and Supplementary Table 4 ).
Figure 2 shows data for one drug representative of each MOA, with the remaining drugs presented in Extended Data Fig. 2 . Consistent with previous observations 23 , 32 , 33 , drug-induced readthrough is much stronger for particular types of stop codon ( P < 2 × 10 −16 , Kruskal–Wallis test). However, this varies extensively across drugs. For example, for G418 and SRI, the efficiency of readthrough is UGA>UAG>UAA, whereas for clitocine it is UGA>UAA>>UAG, and for DAP, it is UGA>>UAG~UAA ( P < 1.7 × 10 −15 for all comparisons, one-sided Wilcoxon signed-rank test; Fig. 2d and Extended Data Fig. 2c ). Drugs with the same direction of effect can also have different magnitudes of effects. For instance, both DAP and SRI stimulate UGA>UAG, but the fold change is different (4.65-fold and 1.64-fold, respectively).
To control for the strong effect of the stop codon types, in the following sections, we focus on UGA variants because they trigger the highest readthrough across all drugs (conclusions for UAG and UAA are similar, and all data are included in Supplementary Table 3 , with main differences pointed out in the text). The three nucleotides immediately after a stop codon have been previously reported to modulate readthrough efficiency in the absence of drugs 23 , 34 . Consistent with this, we see a strong effect of the downstream sequence (+1, +2 and +3 nts) on drug-induced readthrough ( P < 2 × 10 −16 , Kruskal–Wallis test). However, as for stop codon preferences, how the downstream sequence modulates readthrough is drug-specific. Readthrough by all drugs is modulated by the nucleotide immediately after the stop codon (Fig. 2e and Extended Data Fig. 2d ), with C consistently being the most efficient nucleotide. However, the rest of the nucleotides show distinct preferences across drugs.
Readthrough is also modulated by the +2 and +3 positions, and the effects differ across drugs (Fig. 2f and Extended Data Fig. 2a ). We identified a stop codon-dependent effect of the downstream nucleotides (Fig. 2g and Extended Data Fig. 2e ), indicating genetic interactions between neighboring nucleotides. A detailed analysis of nucleotide contexts can be found in Supplementary Note 4 .
Upstream sequence modulates readthrough
Previous studies in bacteria 35 , yeast 36 , 37 and mammalian cells 38 , 39 have shown that the codons upstream of a stop codon can also modulate readthrough under drug-free conditions. Clustering sequences in our library by the upstream codon revealed upstream preferences for each of the drugs ( P < 2 × 10 −16 , Kruskal–Wallis test; Fig. 2h and Extended Data Fig. 2b ). For instance, under SRI treatment, the codons encoding the amino acids P, G and I ( n = 193) display low readthrough, as opposed to Y- and Q-encoding codons ( n = 459), which drive high readthrough (1.8-fold in Q and Y versus P, G and I; P < 2 × 10 −16 , one-sided Wilcoxon signed-rank test; Extended Data Fig. 2f ). Note that codons encoding the same amino acid might display different readthrough in a drug-specific fashion (Fig. 2i and Extended Data Fig. 2g,h ).
To gain more insight into the effect of the upstream sequence, we clustered the codons by the identity of the third nucleotide (Fig. 2j and Extended Data Fig. 2i ). Codons ending in A ( n = 538 versus n = 1,457) tend to be the top-readthrough-promoting codons for all readthrough drugs, except for clitocine, although the effect differs across amino acids (1.1- to 1.3-fold change, adjusted P < 1 × 10 −4 for DAP, G418, SRI, SJ6986 and CC90009). Additional analyses of nucleotide contexts can be found in Supplementary Note 4 .
We found little association between readthrough and GC-content or codon bias indexes (codon adaptation index (CAI) 40 , tRNA adaptation index (tAI) 41 , 42 ; Methods ; Extended Data Fig. 3a–c ). Controlling for nucleotide sequence does suggest an additional effect of the encoded amino acid (Extended Data Fig. 2j ).
Multistop variants
Our library comprised a total of 240 genomic positions with two variants representing different stop types (named ‘multistop variants’). The correlation of readthrough between pairs of stop variants ranges from ~0 to almost 0.85, depending on the drug and stop types being compared (Extended Data Fig. 3d ). For instance, in SRI and SJ6986, the UGA variants correlate well in readthrough efficiency with the UAA variants, but their readthrough is two times higher. For G418, UGA variants are three times more readthrough sensitive than UAA variants. Other comparisons show very different behavior across stop types (for example, UAA versus UAG for DAP and clitocine). Examples of different stop codon variants in the same genomic position under different treatments are shown in Fig. 3a . For instance, DMD _S622X_UGA responds efficiently to DAP, but DMD _S622X_UAA responds poorly. Other examples include PTEN _Y88X in clitocine, IFNGR1 _S306X in DAP/G418/SRI and APC _S583X in DAP/SRI.
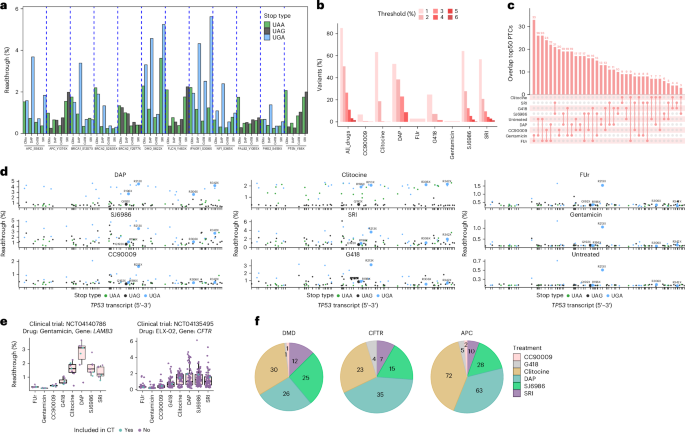
a , Readthrough efficiency for 12 multistop variants across four drugs. Each multistop variant comprises two different nonsense mutations (different stop codon identities) observed in the same genomic locus. b , Percentage of variants with readthrough over different thresholds for each drug separately and when considering all eight drugs together (All_drugs). c , All pairwise overlaps of each drug’s top 50 readthrough-sensitive variants. The number indicates how many variants overlap in the top 50 readthrough-sensitive variant sets of the two compared drugs. d , Readthrough efficiency across drugs, for 102 nonsense TP53 mutations colored by stop codon type. The top five most recurrent nonsense mutations in the human tumor genomes are highlighted. e , Our observed readthrough efficiencies of the nonsense variants tested in two clinical trials (CTs) (blue), together with the rest of the nonsense variants in the same gene tested in our assay (purple). Clinical trial identifier, drug and gene tested are specified in the titles. The top and bottom sides of the box are the lower and upper quartiles. The box covers the interquartile interval, where 50% of the data are found. The horizontal line that splits the box in two is the median. f , Number of variants for which each drug displays the highest readthrough efficiency across the top three genes commonly tested in clinical trials for nonsense suppression therapies, considering all variants in our dataset.
Effective readthrough drugs for pathogenic variants
The drug-specific readthrough of different variants increases the number of patients potentially treatable by a genetically informed choice of drug. Considering all 5,837 PTCs in our library, readthrough >2% can be achieved for 50.3% by using the best drug for each mutation. This is higher than for any individual drug, with >2% readthrough for 38%, 28%, 21%, 19%, 7% and 0.6% of PTCs with DAP, SJ6986, SRI, clitocine, G418 and CC90009, respectively (Fig. 3b ). By applying genetically informed drug selection, many variants display even higher readthrough—>3% for 27% of PTCs, >4% for 11%, >5% for 3.2% and >6% for 1.6% (Fig. 3b ).
However, clinical trials usually test one drug—one gene association; hence, knowing which drug maximizes readthrough across all observed PTCs of a gene is important. The highly represented genes in our library (>20 mutations, n = 33) revealed that DAP, SJ6986 and clitocine are the most efficient drugs for most genes, but their relative order is different (Extended Data Fig. 3e ). As expected, it is strongly associated with the stop type prevalence in the gene. In general, clitocine emerges as the best drug for UAA-rich genes ( APC and BRCA2 ), DAP for UGA-rich ( ATRX and FAT1 ) and SJ6986 for UAG-rich ( MYBPC3 and TSC2 ). Additionally, we asked whether some genes are overall more readthrough sensitive than others. The average readthrough when pooling all drugs together is normally distributed and varies between 0.9% and 1.5% across genes (>20 mutations in our library, n = 33).
Readthrough of tumor suppressor and disease genes
As evidenced above, there is considerable drug-specific variability in readthrough at the variant level (Fig. 2c ). Comparing the top 50 most sensitive variants for each drug shows that each drug maximizes readthrough of a different set of variants (an average of 13 variants overlap across all pairwise comparisons; Fig. 3c ). Thus, the best drug to apply would strongly depend on the particular nonsense mutation causing the disease in each patient.
As an example of how different drugs promote the readthrough of different PTCs, we consider the commonly mutated tumor suppressors TP53 and PTEN (Fig. 3d , Extended Data Fig. 3f and Supplementary Table 5 ). The five most recurrent TP53 PTCs constitute 44% of all the TP53 nonsense mutations in the MSK-IMPACT and TCGA datasets (102 different TP53 nonsense mutations) and are carried by 3% of all MSK-IMPACT and TCGA patients 17 , 18 . These PTCs show promising readthrough therapy potential. Readthrough of the most prevalent TP53 nonsense mutation R213X_UGA can be substantial—4.5% with DAP and 3.6% with SRI and SJ6986. Interestingly, readthrough stimulation of TP53 _R213X_UGA bearing mice was reported to decrease tumor growth 8 . Readthrough of the second most frequent PTC, R342X_UGA, is strong with clitocine, DAP, SRI and SJ6986 (all > 2%). Q192X_UAG, the fifth most recurrent mutation, is the only variant in this set insensitive to all treatments (readthrough < 1%). In total, readthrough >2% can be achieved by at least one drug for 43/102 PTCs in TP53 . Considering all 102 PTCs, SJ6986 is the most effective drug for 36 PTCs, DAP for 25 PTCs, clitocine for 21 PTCs, SRI for 14 PTCs and G418 for 6 PTCs in TP53 . Encouragingly, clitocine treatment was shown to impede tumor growth in mice bearing the Q136X_UAA mutation 27 , which displays a 2.1% readthrough in our dataset. R196X_UGA, R213X_UGA, R306X_UGA and R342X_UGA have similar readthrough efficiencies (1.9%, 2.1%, 2.1% and 2.1%, respectively), suggesting that clitocine could also have similar therapeutic potential for these four TP53 mutations.
For PTEN , the four most frequent PTCs constitute 36% of PTCs reported in MSK-IMPACT (97 different PTEN nonsense mutations were considered here). Of these, the two more prevalent mutations (R130X_UGA and R233X_UGA) show very similar readthrough profiles with high DAP-induced readthrough (3.4% and 3.1%) and are also effectively stimulated by SJ6986 (2.0% and 2.0%), clitocine (1.7% and 1.8%) and SRI (1.8% and 1.3%; Extended Data Fig. 3f ), in line with previous observations that these mutations drive restoration of functional PTEN under readthrough treatment 21 . In contrast, PTEN Q171X_UAG and Q245X_UAG do not respond to any of the readthrough drugs tested here (readthrough <1%). In total, readthrough >2% can be achieved for 35/97 of all the PTCs reported in PTEN in MSK-IMPACT with at least one drug. Considering all 97 PTCs, DAP is the most effective drug for 32 PTCs, clitocine for 31 PTCs, SJ6986 for 22 PTCs and SRI for 12 PTCs.
Residual WT protein levels that partially rescue phenotypes were also reported for mucopolysaccharidosis type I-Hurler disease (>0.5% expression of IDUA gene) 7 and ataxia-telangiectasia (2–5% expression of ATM gene) 43 , 44 . Our dataset shows that 10/10 IDUA mutations and 28/83 ATM mutations display readthrough >0.5% and >2%, respectively, for at least one treatment, suggesting that patients harboring those mutations could be promising candidates for readthrough therapy (Extended Data Fig. 3g ).
Genetics-informed patient stratification for clinical trials
Our data highlight the highly variable efficacy of readthrough-inducing drugs across different PTCs. However, to our knowledge, only 1 of 42 phase II–IV clinical trials using readthrough-promoting drugs 45 used the genetic context of a PTC as an inclusion criterion (ClinicalTrials.gov registration: NCT04135495 ; Supplementary Table 6 and Extended Data Fig. 3h ). Furthermore, only five trials made the identity of patient PTCs available 46 , 47 , 48 .
We used our data to evaluate the optimal match between drugs and PTCs in two of these trials (Fig. 3e ). Clinical trials NCT04140786 used gentamicin and NCT04135495 used a gentamicin derivative, ELX-02. However, our data show that effective readthrough of the PTCs present in patients included in these trials is likely to have been very limited. The average readthrough of these PTCs by gentamicin is only 0.2%. In contrast, average readthrough by clitocine and DAP would be 1.8% and 2.9%, respectively, and other PTCs in the same gene would be better choices for a gentamicin trial (Fig. 3e ). In the two other trials with available patient data, the most effective drug also varies across patients' PTCs and PTCs display high-readthrough variability within each drug, too (Extended Data Fig. 3i ). Patient response data shows a non-significant but positive correlation with our readthrough measurements (Supplementary Note 5 ).
We next considered the following three genes most frequently targeted in nonsense suppression clinical trials: DMD , CFTR and APC . Our data shows that the most effective readthrough drugs for these genes are clitocine and DAP, but that, in all three cases, a combination of drugs matched to patient PTCs would prompt the highest readthrough rates (Fig. 3f ). For example, of the 95 pathogenic PTCs in DMD , the most effective readthrough is obtained with clitocine for 30 PTCs, DAP for 26, SJ6986 for 25, SRI for 12 and G418 and CC90009 for 1 PTC each. To our knowledge, none of the best three drugs (clitocine, DAP and SJ6986) have yet been evaluated in clinical trials.
Accurate prediction of readthrough efficiency
Our extensive and quantitative dataset of drug-induced PTC readthroughs provides an opportunity to train and evaluate computational models to predict drug-induced readthrough. We focused on the six drugs that triggered readthrough >1% for >3% of PTCs and used logistic regression to train sequence-based genotype–phenotype models (Supplementary Note 6 ). Models for the remaining three conditions (FUr, gentamicin and untreated cells) had poor predictive performance ( r 2 = 0.37, 0.38 and 0.02, respectively) due to the very small proportion of PTCs undergoing any notable amount of readthrough in these conditions (153, 31 and 17 PTCs with >1% readthrough, respectively; Extended Data Fig. 4a,b and Supplementary Note 6 ).
After model optimization (Supplementary Note 6 and Extended Data Fig. 4c–e ), we found that a simple model using four sequence feature groups showed good performance across all six drugs (Fig. 4a and Supplementary Table 7 ). The four feature groups included are as follows: (1) stop codon type; (2) the three nucleotides downstream of the PTC and their interactions; (3) the three nucleotides upstream of the PTC and their interactions and (4) the interaction between the stop type and the three nucleotides downstream of the PTC (formula 4 in Supplementary Note 8 ). Increasing the downstream sequence context up to +8 nts didn’t improve predictive performance for any of the drugs (Fig. 4b and formula 5 in Supplementary Note 8 ). The correlation between predicted and observed readthrough evaluated by ten rounds of cross-validation (90–10% training-testing split) was r 2 = 0.89 (clitocine), 0.87 (DAP), 0.76 (SRI), 0.76 (G418), 0.71 (SJ6986) and 0.55 (CC90009). Of note, CC90009 is the dataset with the highest technical noise ( r 2 = 0.62 inter-replicate correlation), likely hindering model performance. Training a single model with the data from all six drugs (pan-drug model) provided poor performance ( r 2 = 0.39) unless the drug identity was included as a predictive feature with interaction terms between the drug and the additional features ( r 2 = 0.83 for the pan-drug model; Fig. 4c , Supplementary Table 8 and formula 6 in Supplementary Note 8 ). This model explains 94% of the explainable readthrough variance (maximum achievable r 2 = 0.89 calculated via inter-replicate correlation across all variants and drugs).
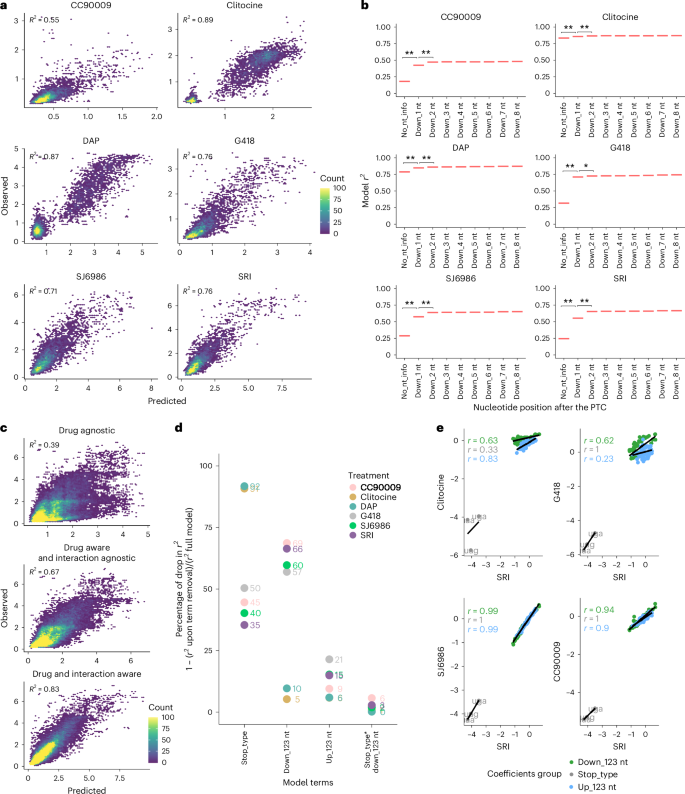
a , Drug-specific models cross-validated predictive performance for CC90009, clitocine, DAP, G418, SJ6986 and SRI conditions. b , Contribution to model performance of the eight nucleotides downstream of the PTC (by adding one at a time). The fixed predictive variables present in all models are the stop codon type and the three nucleotides upstream of the PTC. T test over 20 cross-validation rounds comparing each model (column) to the previous one was used to determine significance (adjusted * P < 0.05, adjusted ** P < 0.01, one-sided t test). c , Pan-drug models cross-validated predictive performance—drug-agnostic (top), drug-aware but sequence × drug interaction-agnostic (middle), drug and sequence × drug interaction-aware (bottom). d , Contribution of each sequence feature to the drug-specific models. Y axis shows the percentage drop in r 2 when each term is removed from the model and normalized to the full model (1 − ( r 2 on term removal/ r 2 full model)). e , Correlation of drug-specific model coefficients (note that for the sake of coefficient interpretability, we ran the models without the interaction term stop_type × down_123 nts, which incurs only a small decrease of r 2 , ranging between 1% and 3% depending on the drug). Coefficients are colored by the model feature they belong to—stop codon type, down_123 nt and up_123 nt. Drugs displaying high correlations respond similarly to the sequence features and, consequently, trigger readthrough of similar subsets of PTCs.
To identify features important for model performance, we removed one variable at a time and calculated the drop in cross-validated r 2 normalized to the full model r 2 , showing that feature contributions quantitatively differ across drug models (Fig. 4d , Extended Data Fig. 4f and Supplementary Note 7 ). We also compared the model coefficients for each feature (in a simplified model without the interaction term to aid coefficient interpretability; Fig. 4e , Extended Data Fig. 4g–j , Supplementary Note 6 and Supplementary Tables 9 and 10 ), which allowed us to capture similarities and differences of individual sequence elements across drugs.
Readthrough prediction for all PTCs in the human genome
The accurate prediction of drug-induced readthrough by our interpretable model allows us to provide readthrough predictions for every possible PTC in every transcript of the human genome (Fig. 5a ). In total, one to three nucleotide substitutions can introduce 32.7 million stop codons in the 19,061 human protein-coding transcripts (Ensembl v107 genes, hg38 assembly), and we made readthrough predictions for six drugs, available as a resource named RTDetective that can be visualized along the human genome using the UCSC browser (Fig. 5b and Extended Data Fig. 5a,b ; https://doi.org/10.6084/m9.figshare.23708901 ).
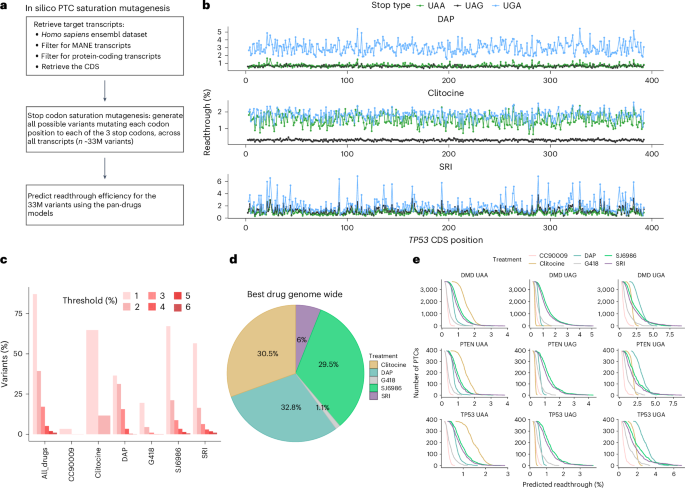
a , Generation of the comprehensive in silico dataset with all possible nonsense mutations in human coding genes. b , Readthrough predictions along the coding sequence (CDS) of TP53 for each stop codon type. Each panel represents a drug-specific readthrough prediction—DAP (top), clitocine (middle) and SRI (bottom). c , Percentage of variants genome-wide with readthrough over a given threshold (color legend) for each drug separately and when considering all eight drugs together (All_drugs). d , Percentage of the number of variants across all possible variants in the human exome for which each drug is predicted to display the highest readthrough efficiency. e , Cumulative histograms showing the number of variants as a function of readthrough efficiency for the genes DMD (top), PTEN (middle) and TP53 (bottom), stratified by stop codon type as UAA (left), UAG (center) and UGA (right).
We estimate that by using these six drugs, a readthrough of >2% can be achieved for 13 million of 32.7 million (39.6%) possible stops in the human genome, with a readthrough of >1% possible for 28.6 million stops (87.3%; Fig. 5c ). The individual drugs are predicted to result in >2% readthrough for 31.4%, 21.3%, 16.2%, 11.7%, 4.3% and 0.02% of PTCs for DAP, SJ6986, SRI, clitocine, G418 and CC90009, respectively. Clitocine is a mid-intensity readthrough drug but spans UAA and UGA stops, inducing 1.5–2% readthrough for many variants but higher readthrough for only a few. Considering all 32.7 million possible PTCs, the most effective drug in 32.8% of cases is DAP, followed by clitocine (30.5%), SJ6986 (29.5%), SRI (6%) and G418 (1.1%; Fig. 5d ).
Some drug-stop codon type combinations show promising potential, as illustrated for DMD , PTEN and TP53 (Fig. 5e ). DAP stimulates readthrough >2% for almost 100% of the UGA variants across the three genes. For UAA mutations, clitocine emerges as the best candidate (readthrough >1.5% for ~50% of PTCs). For UAG mutants, the recently reported eRF1/eRF3 inhibitors SRI-41315 and SJ6986 (refs. 25 , 30 ) show promise (across DMD , PTEN and TP53 , readthrough > 1.5% for 33% and 25% of UAG PTCs for SJ6986 and SRI, respectively, whereas readthrough > 1.5% for <0.1% of UAG PTCs for clitocine and DAP). Thus, even for UAG variants that have been considered particularly difficult to suppress, drug-induced readthrough provides a promising therapeutic strategy, provided that the correct drugs are matched to each PTC.
Readthrough of natural termination codons
Drug-induced readthrough over natural termination codons (NTCs) has been postulated as the main cause of toxicity observed in patients 49 . Notably, translation termination of NTCs differs from that of PTCs, with additional elements involved, including the proximity to Poly(A) tails 50 , readthrough peptide targeting pathways 51 and in-frame downstream 3′-UTR stops that together reduce the generation of readthrough peptides at NTCs. Here we focus on the contribution of local sequence context to NTC readthrough, while the additional elements listed above remain to be addressed in future work.
We leveraged our system to assess the readthrough stimulation of five high-readthrough drugs (clitocine, DAP, G418, SJ6986 and SRI) over NTCs from ~18.8k human protein-coding genes, preserving the upstream and downstream (3′ UTR) 66 nts, but selectively removing the in-frame stops downstream (to uniquely assess the role of the stop codon proximal sequence to NTC readthrough). Readthrough measurements were highly correlated across replicates ( r = 0.88–0.99), and on average, 5 million cells were sorted (~277 cells per variant) and 17,590 high-confidence variants (≥10 reads) were recovered in each experiment (Supplementary Table 11 ). Distributions resembled their PTC counterparts with somewhat lower readthrough (except for SJ8986), with the largest PTC–NTC differences for DAP (1.9-fold, adjusted P < 2 × 10 −16 ) and SRI (1.5-fold, adjusted P < 2 × 10 −16 ) drugs (Fig. 6a ). This suggests a modest readthrough-protective effect of the NTC-surrounding sequence, even after removing additional downstream stops. Artificially setting the readthrough of variants whose endogenous gene has downstream in-frame stops to 0% (assuming that readthrough over two stops is ~0%), shows a strong drop in readthrough of the NTC population (Fig. 6b ).
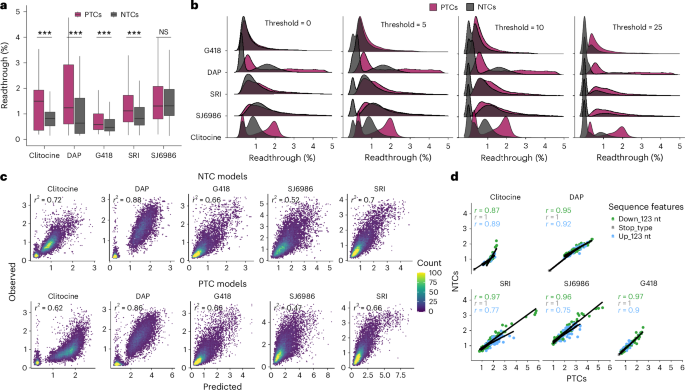
a , Readthrough distributions across drugs for the PTC and NTC libraries (two-sided Wilcoxon test, *** P < 2 × 10 −16 and n = 23,459, n = 23,096, n = 22,905, n = 22,989 for clitocine, DAP, G418 and SRI, respectively, whereas P = NS and n = 23,201 for SJ6986). The top and bottom sides of the box are the lower and upper quartiles. The box covers the interquartile interval, where 50% of the data are found. The horizontal line that splits the box in two is the median. b , Readthrough distributions across drugs for the PTC and NTC libraries. The threshold indicates the number of amino acids downstream of the NTC considered for the analysis. NTC variants with a 3′-UTR in-frame stop codon more proximal than the threshold are assumed to have a readthrough of 0%. Increasing the threshold increases the number of readthrough-insensitive variants. The number of NTC high-confidence variants (≥10 reads) recovered for each treatment and for which readthrough percentages were quantified are 17,812, 17,654, 17,382, 17,661 and 17,587 for clitocine, DAP, G418, SJ6986 and SRI, respectively. c , Drug-specific models predictive performance on the NTC dataset using NTC-trained tenfold cross-validated models (top) or PTC-trained models (bottom). d , Correlation of the mean readthrough for each sequence context between PTCs and NTCs, colored by the sequence feature. NS, not significant.
Finally, we used the drug-induced readthrough of the NTC library as an additional test of our PTC-trained predictive models (Fig. 6c ). Performance was good across drugs ( r = 0.47–0.86) and similar to that for new models trained on the NTC data itself ( r = 0.52–0.88 by tenfold cross-validation; Supplementary Table 12 ). Indeed, the mean readthrough for each sequence feature correlates very well in PTCs versus NTCs, supporting a similar sequence context role in both translation termination scenarios (Fig. 6d ).
We have presented here a systematic quantification of drug-induced stop codon suppression comprising >140,000 readthrough measurements in human cells made using eight drugs. Our massively parallel assay represents a substantial scaling-up of data production compared to previous studies 14 , 16 , generating datasets of sufficient size to train models to accurately predict drug-induced nonsense suppression genome-wide.
Our results show that each drug only induces the readthrough of a subset of pathogenic PTCs, and our models use the sequence context to predict the readthrough specificity of each drug. This diversity of drug responses means that for any particular disease gene, there is no single drug that triggers a strong readthrough of all pathogenic PTCs. Rather, effective clinical nonsense suppression will require a panel of drugs, with the appropriate drug selected for each patient according to the identity of the PTC that they carry.
The models that we have trained are deliberately interpretable and relatively simple, and yet they explain 94% of the variance in our dataset (excluding FUr and gentamicin). It is possible that black box machine learning models may further improve predictive performance, but model interpretability both aids mechanistic understanding and is desirable for models to be used in clinical decision-making 52 .
We envisage that accurate genome-scale prediction of drug-induced readthrough will improve clinical trial design and the development of personalized nonsense suppression therapies. To date, trial designs have nearly all ignored the large variation in readthrough efficacy across PTCs 45 , resulting in suboptimal matching between patient PTCs and drugs. The use of the right drugs but in the wrong patients is, in retrospect, likely to have been an important cause of trial failure.
Our general approach can be used to rapidly quantify the specificities of new nonsense suppression therapeutics, allowing their clinical efficacy to then be tested in the subset of patients in which they are likely to be most effective.
Our study has some important limitations. First, readthrough was not measured in the endogenous genomic context, and long-distance features may also affect readthrough. Second, not all readthrough translation products will be functional proteins. Third, the level of readthrough required for clinical benefit will vary across proteins and diseases. Finally, the clinical efficacy of readthrough-promoting drugs will depend on additional parameters such as pharmacokinetics, pharmacodynamics and drug toxicity.
Taken together, our results show that the specificities of nonsense suppression therapies differ extensively across drugs, and these specificities can be rapidly learned using high-throughput experiments to allow accurate prediction of drug responses. Looking forward, we believe that the goal should be to develop an expanding portfolio of readthrough drugs with defined and complementary specificities such that effective and specific nonsense suppression therapy can be achieved for any pathogenic stop codon in the human genome.
Ethics and consent
The study did not require any specific ethics approval. It builds on publicly available data provided by ClinVar, TCGA and MSK-IMPACT. The patient data obtained from two clinical trials were already released.
Library design
For the PTCs library, genetic disease and cancer germline variants were retrieved ( n = 3498) from the ClinVar database, where all pathogenic nonsense variants whose review status was two or more stars were included (a final filtering step was carried out to decrease the penetrance of overrepresented genes such as BRCA2). Somatic cancer variants were obtained from MSK-IMPACT and TCGA databases with at least two entries in either dataset ( n = 2,372). Finally, a control no-nonsense TP53 variant (c.541C-c.687T nucleotides from ENST00000269305 transcript) was included to use as a 100% readthrough control to normalize the expression of the PTC variants and get estimates for the percentage of WT protein expression for each variant. The 147 nt spliced-mRNA context of each nonsense variant (MANE transcript isoform) was retrieved from Ensembl (v104) to preserve a large sequence context (72 nts upstream and 72 nts downstream).
For the NTCs library, the 66 nts upstream and downstream (3′ UTR) for the spliced MANE isoform of all 18,824 human protein-coding genes were retrieved from Ensembl (v104). We artificially performed 1-Hamming distance substitutions to remove all in-frame 3′-UTR stop codons (TAA->TAC, TAG, TGA->TGG) to assess the role of sequence context on readthrough without the confounding influence of tandem stops. The no-nonsense TP53 variant (c.541C-c.687T nucleotides from ENST00000269305 transcript) was included to use as a 100% readthrough control.
No statistical methods were used to predetermine sample sizes of the libraries, but our sample sizes are 100–1,000 folds larger than those reported in previous publications 11 , 12 , 13 , 14 , 16 .
Readthrough reporter
We designed a double fluorescent reporter plasmid (pIT092) to quantify readthrough. The plasmid encodes a single transcript that contains the open reading frames (ORFs) of EGFP, T2A (2×) and mCherry from 5′–3′, respectively. The library oligo pool was cloned in-frame between the two T2A sequences. T2As allow the independent folding of the fluorescent proteins and prevent undesired effects of the variable sequence on their folding and stability. In a normal translation event, termination occurs in the PTC of the library, protecting mCherry from translation. However, if readthrough occurs, the ribosome extends elongation until the mCherry stop codon translating the mCherry protein along the way. Hence, mCherry fluorescence is proportional to readthrough efficiency, and we used it as our assay readout. EGFP is used to filter out those cells that either do not have EGFP or have unexpectedly high levels of EGFP. These cells are likely to have either aberrant cloning, out-of-frame integration, promoter mutations, promoter silencing, transcript-stability mutations, etc., and might be misleading if included in the assay. For some of the treatments, we detect a slight EGFP increase in the mCherry + population, suggesting a readthrough-mediated transcript stabilization either via NMD inhibition or translation-mediated mRNA protection. In those cases, the mCherry increase (in mCherry + versus mCherry − populations) is higher than the EGFP increase, proving that the drugs are increasing the mCherry signal via readthrough. pIT092 is suitable for genomic integration using the HEK293T landing pad (LP) system 19 , which ensures that each cell integrates only one variant providing a direct genotype–phenotype linkage. The vector contains BxBI-compatible attB sites that allow recombination into the genomic LP of HEK293T_LP cells. After genomic integration into the LP locus, the ORF sequence is placed right downstream of a tetracycline induction cassette, allowing its expression when doxycycline is added to the media.
Library cloning
Oligos were ordered as an oligo pool to Twist Biosciences containing the variable part (library) and two constant sequencesfor PCR amplification and subcloning. The oligo pool was PCR-amplified for 14 cycles using primers oIT204 and oIT340 (Supplementary Table 14 ). The oligo pool was cloned between the EGFP-T2A and T2A-mCherry ORFs of pIT092 using Gibson Assembly. The library was electrotransformed using Neb10 electrocompetent bacteria and grown in 100 ml overnight culture. Library complexity and representativity of the variants were estimated by plating a small amount of the transformation reaction and extrapolating the total number of transformants. Individual clones were Sanger sequenced to confirm the expected structure and diversity.
Stable cell line generation
To generate the cell lines, we used the HEK293T_LP cell line generated in ref. 19 (TetBxB1BFP-iCasp-Blast Clone 12 HEK293T cells), which allows the stable single-copy integration of variants in the genome. Mutational libraries cloned into the LP compatible construct (pIT092) are cotransfected (1:1) with a BxBI expression construct (pCAG-NLS-Bxb1) into the HEK293T_LP cell line using lipofectamine 3000 according to the manufacturer’s instructions in three T150 cm 2 flasks. This cell line has a genetically integrated tetracycline induction cassette, followed by a BxBI recombination site and a split rapalog-inducible dimerizable Casp-9. Cells were maintained in DMEM supplemented with 10% FBS tetracycline-free without antibiotics. Two days after transfection, doxycycline (2 μg ml −1 ; Sigma-Aldrich) was added to induce expression of the library (recombined cells) or the iCasp-9 protein (no recombination). Twenty-four hours later, 10 nM rimiducid (Selleckchem, AP1903) was added to the cells. Successful recombination frameshifts the iCasp-9 out of frame. However, nonrecombined cells express iCasp-9, which dimerizes in the presence of rimiducid and induces apoptosis. One day after rimiducid treatment, the media was changed back to DMEM + doxycycline, and cells were maintained in culture for the following 5 days to obtain a large volume of cells for downstream experiments and cryostorage.
Readthrough compounds
We tested a panel of 20 compounds reported to have readthrough activity (Supplementary Table 2 ), in our library-integrated HEK293T_LP cells. If a drug induces readthrough, the fluorescence-activated cell sorting (FACS) profile would be different than the untreated cells; specifically, we would observe an increase in the mCherry + population (Extended Data Fig. 1a ). Readthrough was calculated as the (mCherry + and EGFP + )/(EGFP + ) cell ratio multiplied by the mean mCherry intensity of the mCherry + population and normalized to the readthrough of the no-nonsense variant (Extended Data Fig. 1d ). All drugs were tested at four or more different concentrations ranging along orders of magnitude, to ensure that a negative result was not due to a concentration-related problem. The 4 × 10 5 library-integrated HEK293T_LP cells were seeded in six-well plates and treated with 2 μg ml −1 doxy to induce the expression of the transcript, and after 24 h, the drug was added to the medium. Readthrough was measured 48 h after treatment with the BD LSRFortessa Cell Analyzer as described above. Eight drugs, namely SRI, clitocine, SJ6986, DAP, G418, gentamicin, CC90009 and FUr, were validated, whereas the remaining 12 did not trigger detectable readthrough in our system at the tested concentrations. We did toxicity titrations for the eight positive drugs (Extended Data Fig. 1c ). In total, 2 × 10 4 cells were plated in 96-well plates, and the CellTiter-Glo Luminescent Cell Viability Assay (Promega) was used to quantify cell viability 48 h after drug or vehicle treatment using a Tecan Infinite M Plex plate reader (Tecan). For each drug, the concentration that didn’t decrease cell viability by more than 25% and exhibited the highest readthrough was selected (Extended Data Fig. 1c,d ). For G418, we chose a concentration that dropped cell viability by a slightly different quantity of 30%, because the increase in readthrough was substantial, compared to the concentration that reduced viability by 25%. Also, note that very high concentrations of gentamicin and G418 were used to counteract the effect of the aminoglycoside-resistance cassette of the HEK293T_LP cells 19 .
The validated drugs comprise different classes of small molecules. G418 and gentamicin bind to the decoding center of the small ribosomal subunit; SRI, SJ6986 and CC90009 are eRF1/eRF3 inhibitors; DAP interferes with the activity of a tRNA-specific 2′-O-methyltransferase (FTSJ1); and clitocine and FUr are nucleotide analogs that get incorporated into the mRNA. Some were reported decades ago, and their readthrough potential is supported by extensive literature (gentamicin, G418) and tested in several clinical trials (most of them with disappointing and confusing outcomes). In contrast, others have been recently described as drugs, and little is known about their readthrough stimulatory potential.
Cells were grown on standard culture plates in tetracycline-free DMEM supplemented with 10% FBS, and without antibiotics. They were split before reaching confluency to maintain cell health. Cells were detached with trypsin, spun down and washed with PBS. For the sort-seq experiments 53 , cells were treated with 2 μg ml −1 doxy to induce the expression of the transcript, and after 24 h, the drug was added to the medium for 48 h more. We used high volumes of cells to ensure that each variant was represented >100 times in the cell population.
Cells were sorted on a BD Influx Cell Sorter and analyzed with BD FACS Software (1.0.0.650). Cells were gated by forward scattering area and by side scattering area to retain whole cells, forward scattering width and height to discard aggregates, and by DAPI staining to retain only recombined and alive cells. EGFP and mCherry fluorescence were excited with 488 nm and 561 nm lasers and recorded with 530/40 bandpass (BP) and 593/40 BP channels, respectively. EGFP + cells were sorted based on mCherry expression into three to five populations (Extended Data Fig. 1a ), depending on how stretched the library was across the mCherry axis. The top-right population in the Extended Data Fig. 1a plots represents the no-nonsense variant and was sequenced for one drug condition (gentamicin) of the natural stops library. In total, 30% of the reads in that population indeed map to the no-nonsense TP53 variant, in contrast to <0.01% in the other populations (Extended Data Fig. 1g ). For most of the populations, 400k cells were sorted. However, for some minor populations representing <2% of the total population, we sorted less cells (100–200k). The percentage of cells in each bin population was used for normalization during sequencing analysis (see below). Experiments were performed in biological duplicates, and, on average, 1.6 million cells were sorted in each drug experiment (~272 cells per variant).
DNA extraction
Sorted cells were centrifuged at 1,200 rpm for 3 min, and the pellet was used to conduct genomic DNA extraction following the DNeasy Blood & Tissue Kit (Qiagen) and resuspended in 80 μl of Milli-Q water.
Sequencing library preparation
The sequencing libraries were constructed in three consecutive PCR reactions. The first PCR intends to amplify the library fragment from the genomic DNA pool without amplifying the remaining plasmid from transfection. It uses a forward (oIT314) primer annealing in the LP outside of the recombined sequence and a reverse (oIT205) primer annealing at the 3′ end of the library fragment. This ensures that plasmid DNA is not amplified because it lacks the annealing site for the forward primer. The second PCR (PCR2) was designed to insert part of the Illumina adapters and to increase the nucleotide complexity of the first sequenced bases by introducing frame-shift bases between the adapters and the sequencing region of interest. The third PCR (PCR3) was necessary to add the remainder of the Illumina adapter and the demultiplexing indexes. All PCRs were run using Q5 Hot Start High-Fidelity DNA Polymerase (New England Biolabs) according to the manufacturer’s protocol.
All genomic DNA extracted from each bin was used as a template for PCR1 and amplified using 25 pmol of primers oIT205 and oIT314. The annealing temperature was set to 66 °C, extension time to 1 min and number of cycles to 25. Because high volumes of genomic DNA inhibit PCR reactions, we aliquoted each sample in eight PCRs and ran them in 96-well plates. Excess primers were removed by adding 0.04 μl of ExoSAP-IT (Affymetrix) per microliter of PCR1 reaction and incubated for 20 min at 37 °C followed by an inactivation for 15 min at 80 °C. Then, the PCRs of each sample were pooled together and purified using the MinElute PCR Purification Kit (Qiagen) according to the manufacturer’s protocol. DNA was eluted in Milli-Q water to a volume of 20 μl.
In total, 2 μl of PCR1 product were used as template for PCR2, together with 25 pmol of pooled frame-shift primers (oIT_ILL_204_mix and oIT_ILL_205_mix; Supplementary Table 14 ). The PCR reactions were set to an annealing temperature of 66 ºC, 15 s of extension time and were run for eight cycles. Excess primers were removed by adding 0.04 μl of ExoSAP-IT (Affymetrix) per microliter of PCR1 reaction and incubated for 20 min at 37 °C followed by an inactivation for 15 min at 80 °C. The PCRs of each sample were purified using the MinElute PCR Purification Kit (Qiagen) according to the manufacturer’s protocol. DNA was eluted in Milli-Q water to a volume of 10 μl.
In total, 2 μl of PCR2 products were used as template for PCR3. In PCR3, the remaining parts of the Illumina adapters were added to the library amplicon. The forward primer (oIT_GJJ_1J) was the same for all samples, while the reverse primer (oIT_GJJ_2J) differed by the barcode index, to allow pooling of all samples together and demultiplexing after deep sequencing (Supplementary Table 14 ). Eight cycles of PCR3s were run at 62 °C of annealing temperature and 25 s of extension time. All reactions from the same sample were pooled together, and an aliquot was run on a 2% agarose gel to be quantified. After quantification, samples with different Illumina indexes that were sequenced together in the same flow cell were pooled in an equimolar ratio, run on a gel and purified using the QIAEX II Gel Extraction Kit. The purified amplicon library pools were subjected to 150-bp paired-end NextSeq 500 sequencing (Illumina) at the CRG Genomics Core Facility.
Sequencing data processing
FastQ files from paired-end sequencing of all experiments were processed with DiMSum (v.1.3; https://github.com/lehner-lab/DiMSum ) to obtain the read counts for each variant. DimSum applies stringent quality filters to discard low-quality reads, reads with sequencing errors, etc., to ensure that only high-quality reads are used for downstream analysis.
The DimSum output read count tables were used to calculate readthrough estimates for each variant as follows: the read count table provides the distribution of each variant among the different sorting gates. Because gates harbored different percentages of the general population, they had to be sorted at different times to get the same number of cells in each bin, forcing us to calculate the mCherry distributions in relative numbers (formula 1 in Supplementary Note 8 ). The distribution of each variant is generated by calculating its proportion of reads in each sorting gate ( j ), with m being the total number of sorting gates. All reads of a given variant in a given sorting gate ( r j ) are (1) divided by the total number of reads of that gate ( R j ) yielding a normalized reads value, (2) multiplied by a fixed value ( pc ) corresponding to the percentage of cells of the total population that belong to that gate and (3) and multiplied by a second fixed value ( fv ) corresponding to the mean mCherry signal of the gate. Finally, (4) it is averaged by the total number of normalized reads across gates ( N ). In steps (1) and (2), we are simply calculating the percentage of reads of the total population belonging to each variant in each gate ( \(\frac{{r}_{j}}{{R}_{j}}{{\mathrm{pc}}}_{j}\) ). In steps (3) and (4) we obtain a value corresponding to a normalized mean mCherry value for each variant. Then, by dividing this mCherry value of each variant by the mCherry value of the untreated no-nonsense variant (which represents the amount of WT protein in basal conditions), the readthrough percentage for each variant (RTp, percentage of WT protein expression) is calculated (formula 2 in Supplementary Note 8 ). The no-nonsense population does not undergo readthrough and displays the same mCherry value across treatments, as expected. However, under clitocine conditions, its mCherry signal is 1.6-fold higher, suggesting an RNA stabilization effect of clitocine. The normalization with the no-nonsense variant in the untreated condition sets all the treatments’ readthrough distributions on the same scale and allows direct comparison across drugs. The EGFP − /mCherry − population was used to calibrate the voltage of the sorting instrument across treatments and replicates. The standard deviation (s.d.) from the two replicates was used as the error measure. Readthrough percentage efficiencies for all variants and drugs are included in Supplementary Tables 3 (PTCs) and 11 (NTCs). Experiments were performed in biological duplicates, and, on average, 5,602 high-confidence variants (≥10 reads) were recovered in each experiment. We retained variants with ≥10 reads. Shapiro–Wilk test was used to determine the non-normality of the sample, and accordingly, nonparametric tests (Kruskal–Wallis and Wilcoxon signed-rank tests) were used to assess significance. Note that the two clitocine replicates were sigmoidally related due to a difference in the voltage setting of the flow cytometer between experiments. A LOESS model was fit and used to predict replicate 2 based on replicate 1. This transformation yielded a linear relation between the two replicates.
Single-variant validation experiments
To validate the assay, we set out to individually measure the readthrough of 15 variants. We selected 15 variants spanning the whole dynamic range when treated with SRI plus the no-nonsense control and individually cloned and integrated them into HEK293T_LP, yielding 16 stable cell lines each expressing a different variant. In parallel, we conducted the same measurements in MCF7 and HeLa cell lines (cells were maintained in tetracycline-free DMEM supplemented with 10% FBS without antibiotics). Because the LP is unique to HEK293T cells, we used transient transfection to express the readthrough variants in MCF7 and HeLa cell lines. Constructs harboring the 16 variants were cloned out from pIT092 and cloned in a mammalian CMV-expression vector (pIT075).
HEK293T_LP were treated with 2 μg ml −1 doxy, and 50 ng of pIT075 plasmids were transfected into MCF7 and HeLa with lipofectamine 3000. Twenty-four hours later, cells were treated with SRI at the same concentration than used in the DMS assay (7.5 μM). 48 h after treatment, EGFP and mCherry fluorescence were quantified using 530/40 BP and 593/40 BP channels in the BD LSRFortessa Cell Analyzer. EGFP + cells were used to calculate the readthrough by multiplying the percentage of mCherry + cells by its mean mCherry intensity and finally normalizing to the no-nonsense variant (formula 3 in Supplementary Note 8 ). We termed these readthrough values as ‘readthrough P individual ’ because they refer to individual measurements of readthrough percentages of each variant. Readthrough P individual were correlated against the readthrough P DMS estimates of the 15 variants to calculate the correlation coefficient between our DMS assay and individually measured readthrough (Supplementary Table 1 ).
Sequence features
We listed a large set of sequence features to test their contribution to readthrough variability. Features were chosen based on literature and preliminary results, but we also listed several features for which we had no evidence of their participation in readthrough. All features tested in the predictive models can be found in Supplementary Table 4 . See Supplementary Note 6 for extended information on model design.
The tAI is a measure of translational efficiency that takes into account the intracellular concentration of tRNA molecules and the efficiencies of each codon–anticodon pairing 41 , 42 . The pairing affinity of each codon–anticodon is specific to each species. The human-specific tAI indexes were downloaded from the STADIUM database as of January 2023 (ref. 54 ). tAI for a given sequence was calculated as the mean tAI across all codons of the sequence.
The CAI is an estimate of translational efficiency based on the similarity of codon usage of one sequence with regard to the genome codon usage 40 . The human codon usage table was downloaded from the Codon/Codon Pair Usage Tables project release as of January 2023 (ref. 55 ). CAI for a given sequence was calculated as the mean CAI across all codons of the sequence.
In silico saturation mutagenesis
We used the general drug model to perform an in silico prediction of the readthrough efficiency of all possible nonsense mutations in the human exome, resulting in 32.7 × 10 6 predictions for the 19,061 protein-coding transcripts (Ensembl v107 genes, hg38 assembly) for each drug. For each codon position of each protein-coding transcript, a readthrough efficiency value was estimated for each drug.
Statistics and reproducibility
Statistical tests were performed in R (v4.3.1) using RStudio (v2023.03.0+386). Kruskal–Wallis tests were used to assess the general association of sequence features and readthrough, whereas Wilcoxon tests were used for pairwise comparisons of specific levels of the sequence features. Wilcoxon tests were either one- or two-sided, and this information is always provided in the figure legend. P values were adjusted using the p.adjust function in R (Benjamini–Hochberg). No statistical method was used to predetermine the sample size. Variants with <10 reads were not used for analyses, and analyses included all other variants unless explicitly indicated. No blinding or randomization was performed.
Reporting summary
Further information on research design is available in the Nature Portfolio Reporting Summary linked to this article.
Data availability
All DNA sequencing data have been deposited in the Sequence Read Archive with accessions PRJNA996618 (PTCs) and PRJNA1073909 (NTCs). The readthrough efficiency predictions have been made available through the Figshare repository at https://figshare.com/articles/dataset/Readthrough_predictions/23708901 and via a digital object identifier ( https://doi.org/10.6084/m9.figshare.23708901 ). All readthrough measurements are provided in Supplementary Tables 3 and 11 .
The MSK-IMPACT and TCGA datasets were downloaded from cBioPortal ( https://www.cbioportal.org/ ) on 2 June 2021. The ClinVar dataset was downloaded from https://ftp.ncbi.nlm.nih.gov/pub/clinvar/vcf_GRCh38/ on 3 June 2021. Source data are provided with this paper.
Code availability
Source code used to perform all analyses and to reproduce all figures in this work is available at GitHub ( https://github.com/lehner-lab/Stop_codon_readthrough ) and has been archived to Zenodo ( https://zenodo.org/records/12698349 ) 56 .
Landrum, M. J. et al. ClinVar: improving access to variant interpretations and supporting evidence. Nucleic Acids Res. 46 , D1062–D1067 (2018).
Article CAS PubMed Google Scholar
Stenson, P. D. et al. The Human Gene Mutation Database (HGMD®): optimizing its use in a clinical diagnostic or research setting. Hum. Genet. 139 , 1197–1207 (2020).
Article PubMed PubMed Central Google Scholar
Supek, F., Lehner, B. & Lindeboom, R. G. H. To NMD or not to NMD: nonsense-mediated mRNA decay in cancer and other genetic diseases. Trends Genet. 37 , 657–668 (2021).
Lykke-Andersen, S. & Jensen, T. H. Nonsense-mediated mRNA decay: an intricate machinery that shapes transcriptomes. Nat. Rev. Mol. Cell Biol. 16 , 665–677 (2015).
Lombardi, S., Testa, M. F., Pinotti, M. & Branchini, A. Molecular insights into determinants of translational readthrough and implications for nonsense suppression approaches. Int. J. Mol. Sci. 21 , 9449 (2020).
Article CAS PubMed PubMed Central Google Scholar
Dabrowski, M., Bukowy-Bieryllo, Z. & Zietkiewicz, E. Advances in therapeutic use of a drug-stimulated translational readthrough of premature termination codons. Mol. Med. 24 , 25 (2018).
Gunn, G. et al. Long-term nonsense suppression therapy moderates MPS I-H disease progression. Mol. Genet. Metab. 111 , 374–381 (2014).
Palomar-Siles, M. et al. Translational readthrough of nonsense mutant TP53 by mRNA incorporation of 5-fluorouridine. Cell Death Dis. 13 , 997 (2022).
Sarkar, C., Zhang, Z. & Mukherjee, A. B. Stop codon read-through with PTC124 induces palmitoyl-protein thioesterase-1 activity, reduces thioester load and suppresses apoptosis in cultured cells from INCL patients. Mol. Genet. Metab. 104 , 338–345 (2011).
Ramalho, A. S. et al. Five percent of normal cystic fibrosis transmembrane conductance regulator mRNA ameliorates the severity of pulmonary disease in cystic fibrosis. Am. J. Respir. Cell Mol. Biol. 27 , 619–627 (2002).
Welch, E. M. et al. PTC124 targets genetic disorders caused by nonsense mutations. Nature 447 , 87–91 (2007).
Trzaska, C. et al. 2,6-Diaminopurine as a highly potent corrector of UGA nonsense mutations. Nat. Commun. 11 , 1509 (2020).
Floquet, C., Hatin, I., Rousset, J.-P. & Bidou, L. Statistical analysis of readthrough levels for nonsense mutations in mammalian cells reveals a major determinant of response to gentamicin. PLoS Genet. 8 , e1002608 (2012).
Pranke, I. M. et al. The U UGA C sequence provides a favorable context to ELX-02 induced CFTR readthrough. J. Cyst. Fibros. 22 , 560–563 (2023).
Dabrowski, M., Bukowy-Bieryllo, Z. & Zietkiewicz, E. Translational readthrough potential of natural termination codons in eucaryotes—the impact of RNA sequence. RNA Biol. 12 , 950–958 (2015).
Bidou, L. et al. 2-Guanidino-quinazoline promotes the readthrough of nonsense mutations underlying human genetic diseases. Proc. Natl Acad. Sci. USA 119 , e2122004119 (2022).
Cancer Genome Atlas Research Network, et al. The Cancer Genome Atlas Pan-Cancer analysis project. Nat. Genet. 45 , 1113–1120 (2013).
Article Google Scholar
Cheng, D. T. et al. Memorial Sloan Kettering-Integrated Mutation Profiling of Actionable Cancer Targets (MSK-IMPACT): a hybridization capture-based next-generation sequencing clinical assay for solid tumor molecular oncology. J. Mol. Diagn. 17 , 251–264 (2015).
Matreyek, K. A., Stephany, J. J., Chiasson, M. A., Hasle, N. & Fowler, D. M. An improved platform for functional assessment of large protein libraries in mammalian cells. Nucleic Acids Res. 48 , e1 (2020).
CAS PubMed Google Scholar
Pranke, I. et al. Factors influencing readthrough therapy for frequent cystic fibrosis premature termination codons. ERJ Open Res. 4 , 00080-02017 (2018).
Article PubMed Google Scholar
Luna, S. et al. A global analysis of the reconstitution of PTEN function by translational readthrough of PTEN pathogenic premature termination codons. Hum. Mutat. 42 , 551–566 (2021).
Floquet, C., Deforges, J., Rousset, J.-P. & Bidou, L. Rescue of non-sense mutated p53 tumor suppressor gene by aminoglycosides. Nucleic Acids Res. 39 , 3350–3362 (2011).
Loughran, G. et al. Evidence of efficient stop codon readthrough in four mammalian genes. Nucleic Acids Res. 42 , 8928–8938 (2014).
Green, L. & Goff, S. P. Translational readthrough-promoting drugs enhance pseudoknot-mediated suppression of the stop codon at the Moloney murine leukemia virus gag–pol junction. J. Gen. Virol. 96 , 3411–3421 (2015).
Lee, R. E. et al. Small-molecule eRF3a degraders rescue CFTR nonsense mutations by promoting premature termination codon readthrough. J. Clin. Invest. 132 , e154571 (2022).
Baradaran-Heravi, A. et al. Effect of small molecule eRF3 degraders on premature termination codon readthrough. Nucleic Acids Res. 49 , 3692–3708 (2021).
Friesen, W. J. et al. The nucleoside analog clitocine is a potent and efficacious readthrough agent. RNA 23 , 567–577 (2017).
Howard, M., Frizzell, R. A. & Bedwell, D. M. Aminoglycoside antibiotics restore CFTR function by overcoming premature stop mutations. Nat. Med. 2 , 467–469 (1996).
Bedwell, D. M. et al. Suppression of a CFTR premature stop mutation in a bronchial epithelial cell line. Nat. Med. 3 , 1280–1284 (1997).
Sharma, J. et al. A small molecule that induces translational readthrough of CFTR nonsense mutations by eRF1 depletion. Nat. Commun. 12 , 4358 (2021).
2024 A molecular glue degrader of eRF1 on the ribosome Nat. Chem. Biol . 20 810 811.
Cridge, A. G., Crowe-McAuliffe, C., Mathew, S. F. & Tate, W. P. Eukaryotic translational termination efficiency is influenced by the 3′ nucleotides within the ribosomal mRNA channel. Nucleic Acids Res. 46 , 1927–1944 (2018).
Wangen, J. R. & Green, R. Stop codon context influences genome-wide stimulation of termination codon readthrough by aminoglycosides. eLife 9 , e52611 (2020).
Namy, O., Hatin, I. & Rousset, J. P. Impact of the six nucleotides downstream of the stop codon on translation termination. EMBO Rep. 2 , 787–793 (2001).
Mottagui-Tabar, S. & Isaksson, L. A. Only the last amino acids in the nascent peptide influence translation termination in Escherichia coli genes. FEBS Lett. 414 , 165–170 (1997).
Mottagui-Tabar, S., Tuite, M. F. & Isaksson, L. A. The influence of 5′ codon context on translation termination in Saccharomyces cerevisiae . Eur. J. Biochem. 257 , 249–254 (1998).
Tork, S., Hatin, I., Rousset, J.-P. & Fabret, C. The major 5′ determinant in stop codon read-through involves two adjacent adenines. Nucleic Acids Res. 32 , 415–421 (2004).
Cassan, M. & Rousset, J. P. UAG readthrough in mammalian cells: effect of upstream and downstream stop codon contexts reveal different signals. BMC Mol. Biol. 2 , 3 (2001).
Arkov, A. L., Korolev, S. V. & Kisselev, L. L. 5′ contexts of Escherichia coli and human termination codons are similar. Nucleic Acids Res. 23 , 4712–4716 (1995).
Sharp, P. M. & Li, W. H. The codon adaptation index—a measure of directional synonymous codon usage bias, and its potential applications. Nucleic Acids Res. 15 , 1281–1295 (1987).
dos Reis, M., Wernisch, L. & Savva, R. Unexpected correlations between gene expression and codon usage bias from microarray data for the whole Escherichia coli K-12 genome. Nucleic Acids Res. 31 , 6976–6985 (2003).
dos Reis, M., Savva, R. & Wernisch, L. Solving the riddle of codon usage preferences: a test for translational selection. Nucleic Acids Res. 32 , 5036–5044 (2004).
Du, L. et al. Nonaminoglycoside compounds induce readthrough of nonsense mutations. J. Exp. Med. 206 , 2285–2297 (2009).
Gilad, S. et al. Genotype–phenotype relationships in ataxia-telangiectasia and variants. Am. J. Hum. Genet. 62 , 551–561 (1998).
Spelier, S., van Doorn, E. P. M., van der Ent, C. K., Beekman, J. M. & Koppens, M. A. J. Readthrough compounds for nonsense mutations: bridging the translational gap. Trends Mol. Med. 29 , 297–314 (2023).
Sermet-Gaudelus, I. et al. Ataluren (PTC124) induces cystic fibrosis transmembrane conductance regulator protein expression and activity in children with nonsense mutation cystic fibrosis. Am. J. Respir. Crit. Care Med. 182 , 1262–1272 (2010).
Mosallaei, D. et al. Molecular and clinical outcomes after intravenous gentamicin treatment for patients with junctional epidermolysis bullosa caused by nonsense variants. JAMA Dermatol. 158 , 366–374 (2022).
Finkel, R. S. et al. Phase 2a study of ataluren-mediated dystrophin production in patients with nonsense mutation Duchenne muscular dystrophy. PLoS ONE 8 , e81302 (2013).
Li, S. et al. Pharmaceuticals promoting premature termination codon readthrough: progress in development. Biomolecules 13 , 988 (2023).
Wu, C., Roy, B., He, F., Yan, K. & Jacobson, A. Poly(A)-binding protein regulates the efficiency of translation termination. Cell Rep. 33 , 108399 (2020).
Müller, M. B. D., Kasturi, P., Jayaraj, G. G. & Hartl, F. U. Mechanisms of readthrough mitigation reveal principles of GCN1-mediated translational quality control. Cell 186 , 3227–3244 (2023).
Sathyan, A., Weinberg, A. I. & Cohen, K. Interpretable AI for bio-medical applications. Complex Eng. Syst. 2 , 18 (2022).
Peterman, N. & Levine, E. Sort-seq under the hood: implications of design choices on large-scale characterization of sequence-function relations. BMC Genomics 17 , 206 (2016).
Yoon, J., Chung, Y.-J. & Lee, M. STADIUM: species-specific tRNA adaptive index compendium. Genomics Inform. 16 , e28 (2018).
Alexaki, A. et al. Codon and codon-pair usage tables (CoCoPUTs): facilitating genetic variation analyses and recombinant gene design. J. Mol. Biol. 431 , 2434–2441 (2019).
lehner-lab/Stop_codon_readthrough: v1.0. Zenodo zenodo.org/records/12698349 (2024).
Download references
Acknowledgements
Work in the Supek laboratory was supported by an ERC StG ‘HYPER-INSIGHT’ (757700) and ERC CoG ‘STRUCTOMATIC’ (101088342), Horizon2020 project ‘DECIDER’ (965193), Horizon Europe project ‘LUCIA’ (101096473), Spanish government project ‘REPAIRSCAPE’, CaixaResearch project ‘POTENT-IMMUNO’ (HR22-00402), an ICREA professorship to F.S., the SGR funding from the Catalan government and a Novo Nordisk Fonden starting package. Work in the Lehner laboratory was funded by European Research Council (ERC) Advanced (grant 883742) and Consolidator (grant 616434), the Spanish Ministry of Science and Innovation (BFU2017-89488-P, EMBL Partnership, Severo Ochoa Center of Excellence), the Bettencourt Schueller Foundation, the AXA Research Fund, Agencia de Gestio d’Ajuts Universitaris i de Recerca (AGAUR, 2017 SGR 1322) and the CERCA Program/Generalitat de Catalunya. The authors would like to thank the four members E. Ramírez, A. Bote, E. Julià and Ò. Fornàs of the Flow Cytometry CRG Core Unit for their support and time, together with G. Palou (IRB) for his help in retrieving the transcript sequences from the Ensembl database and all members of the Supek and Lehner laboratories for helpful discussions and suggestions.
Author information
Authors and affiliations.
Institute for Research in Biomedicine (IRB Barcelona), The Barcelona Institute of Science and Technology (BIST), Barcelona, Spain
Ignasi Toledano & Fran Supek
Centre for Genomic Regulation (CRG), The Barcelona Institute of Science and Technology (BIST), Barcelona, Spain
Ignasi Toledano & Ben Lehner
Biotech Research and Innovation Centre (BRIC), University of Copenhagen, Copenhagen, Denmark
Institució Catalana de Recerca i Estudis Avançats (ICREA), Barcelona, Spain
Fran Supek & Ben Lehner
University Pompeu Fabra (UPF), Barcelona, Spain
Wellcome Sanger Institute, Wellcome Genome Campus, Hinxton, UK
You can also search for this author in PubMed Google Scholar
Contributions
I.T., F.S. and B.L. conceived the project and designed the experiments. I.T. performed the experiments and analyzed the data. I.T., F.S. and B.L. wrote the manuscript.
Corresponding authors
Correspondence to Fran Supek or Ben Lehner .
Ethics declarations
Competing interests.
The authors declare no competing interests.
Peer review
Peer review information.
Nature Genetics thanks the anonymous reviewer(s) for their contribution to the peer review of this work.
Additional information
Publisher’s note Springer Nature remains neutral with regard to jurisdictional claims in published maps and institutional affiliations.
Extended data
Extended data fig. 1 experimental setup and overview of the drug’s datasets..
a , FACS profiles (BD Influx Cell Sorter instrument) of the PTC library under the different treatments sorted by EGFP (y-axis) and mCherry (x-axis). Binned populations are indicated together with the control population harboring the no-nonsense TP53 variant. b Inter-replicate correlations for the nine conditions. c , d , Cell viability ( c ) and readthrough ( d ) titration curves for each drug, where error bars represent the standard deviation across three biological replicates. Four to six drug concentrations were tested for each drug, and the concentration displaying the highest readthrough and reducing cell viability less than 25% (blue) was used for the assay. Very toxic concentrations were not tested for readthrough stimulation. In d , readthrough was calculated as the (mCherry + and EGFP + )/(EGFP + ) cell ratio multiplied by the mean mCherry intensity of the mCherry + population and normalized to the readthrough of the no-nonsense variant. e , All pairwise inter-drug correlations. f , Sequence features association with readthrough efficiency: showing Pearson correlations (continuous variables) and Kruskal–Wallis chi-squared statistics (discrete variables). g , Percentage of reads mapping to the no-nonsense variant across sorting populations of the natural stops library under gentamicin treatment. The variant is almost exclusively found in the no-nonsense population, where it represents 30% of the cells. h , DMS vs individual measurements Pearson’s correlation (r = 0.77). It represents an extension of Fig. 1d to specifically test the upper ceiling of the assay, with ten more variants spanning high-readthrough estimates included. A loess curve was fit to model the non-linearities triggered by the upper limit of the assay (see Supplementary Note 1 for more information on the assay saturation limit. Variants above the dashed line (1.3% of the library) have >90% reads in the highest sorting gate. i , Readthrough distributions across drugs colored by the stop type.
Extended Data Fig. 2 Sequence features explain the readthrough variability across PTCs and drugs.
a – i , Effect of the sequence feature ( x -axis) on readthrough efficiency ( y -axis) colored by drug. The top and bottom sides of the box are the lower and upper quartiles. The box covers the interquartile interval, where 50% of the data are found. The horizontal line that splits the box in two is the median. Only variants where the stop codon is UGA are shown (except for c and e , where all stop codon variants are shown). The sequence features are the three nucleotides downstream of the PTC (n = 10645, p < 2e−16, Kruskal–Wallis test) ( a ), the three nucleotides upstream of the PTC (n = 10645, p < 2e−16) ( b ), the stop type (n = 22227, p < 2e−16) ( c ), the nucleotide in position +1 downstream of the PTC (n = 10502, p < 2e−16) ( d ), same as d but stratified by stop codon (n = 16753) ( e ), the amino acid upstream of the PTC (n = 10602, p < 2e−16) ( f ), variants with a glutamic acid upstream of the PTC stratified by the codon (n = 613) ( g ), variants with an arginine upstream of the PTC stratified by the codon (n = 1040, p = 3e−6) ( h ), and the effect of amino acids encoded by A-ending codons on readthrough efficiency for FUr, gentamicin, CC90009 and SJ6986, where codons ended in A display higher readthrough efficiencies compared to the rest of the codons (n = 7902, adjusted p < 1e−3, one-sided Wilcoxon signed-rank test). The nucleotide in position +3 of the codon is denoted with colors ( i ). j , Mean readthrough difference between pairs of codons with Hamming distance of 1 (that is, single nucleotide difference) that encode for the same amino acid, or pairs that encode a different amino acid across drugs. P value of the two-sided t-test between the same amino acid and different amino acid groups is shown. k , l , Readthrough distributions ( k ) and pairwise correlations ( l ) for the three SJ6986 concentrations tested (0.5 μM, 5 μM and 20 μM).
Extended Data Fig. 3 Codon-related features, multistop variants and overview of PTEN nonsense mutations and clinical trials.
a – c , Correlation of tAI ( a ), CAI ( b ) and GC% ( c ) of the 5aas upstream of the PTC with readthrough efficiency for each drug. d , Correlation of multistop variants across drugs. Each data point belongs to a mutation in the same genomic position but with a different stop type. e , Drug preferences for the highly represented genes in our dataset (>20 variants, n = 33). Y-axis shows the percentage of mutations for which each drug displays the highest readthrough. f , Readthrough efficiency across drugs for 97 nonsense PTEN mutations colored by stop codon type. The top 4 most recurrent nonsense mutations in human tumors are highlighted. g , Percentage of IDUA and ATM mutations in our dataset displaying higher readthrough levels than the phenotypic threshold reported in refs. 7 , 43 , 44 across drugs. h , All past and current (n = 42) phases II–IV clinical trials testing readthrough drugs, obtained from ref. 45 . i , Our readthrough efficiencies of the nonsense variants tested in two clinical trials (CTs) (blue), together with the rest of nonsense variants in the same gene tested in our assay (purple). Clinical trial identifier, drug (ataluren) and gene tested are specified in the titles. Many variants included in clinical trials are unresponsive to drugs, likely hindering their performance. The top and bottom sides of the box are the lower and upper quartiles. The box covers the interquartile interval, where 50% of the data are found. The horizontal line that splits the box in two is the median.
Extended Data Fig. 4 Predictive models overview and optimization.
a , Drug-specific models cross-validated predictive performance for FUr, gentamicin and untreated conditions. b , Downsampling the number of readthrough variants yields decreased model performance for the high-performing drug models (Supplementary Note 6 ). X -axis shows the number of variants with readthrough >1% retained and used to rerun the models (gray). We used control models randomly removing the same number of variants to control for the effect of smaller training sizes in model performance (black). Models with 20, 50 and 150 variants retained intend to represent similar scenarios to untreated, gentamicin and FUr datasets. The r 2 s shown are the average over 10 cross-validation rounds. c , Comparison of the r 2 values for the drug-specific models when using stop type, the three nucleotides downstream and upstream of the PTC and the interaction between stop type and three nucleotides downstream versus when using ElasticNet regularization on 47 sequence features (Extended Data Fig. 1f and Supplementary Table 4 ). d , Performance for three different model formulations across drugs (Supplementary Note 6 ): using only stop type and the three nucleotides downstream and upstream of the PTC, adding the stop type and three nucleotides upstream interaction or adding the stop type and three nucleotides downstream interaction. Only the latter consistently improves model performance across drugs. The r 2 s shown are the average over 10 cross-validation rounds. e , Performance for three different model formulations across drugs: encoding the three nucleotides downstream and upstream of the PTC as nucleotide triplets (m1), encoding the upstream sequence as a nucleotide triplet and the three nucleotides downstream as three different terms (one for each position, no interaction among them, m2) and encoding the downstream sequence as a nucleotide triplet and the three nucleotides upstream as three different terms (one for each position, no interaction among them, m3). m1 consistently yields higher r 2 across drugs. The r 2 s shown are the average over 10 cross-validation rounds. f , Contribution of each sequence feature to the pan-drug model. The y-axis shows the relative drop in r 2 when each term is removed from the model and normalized to the full model (1 − (r 2 upon term removal/r 2 full model)). g – j , Model coefficients of the following predictive models: CC90009 and clitocine ( g ), DAP and SJ6986 ( h ), G418 and SRI ( i ) and the down_123 nt (top) and up_123 nt (bottom) coefficients for the pan-drug model ( j ). Mean, 95% confidence intervals and significance (two-sided Student’s t-test) of the coefficient estimates across 10 cross-validation rounds are shown. Asterisks represent an adjusted p-value < 0.01.
Extended Data Fig. 5 In silico PTC saturation mutagenesis.
a , Overview of the readthrough predictions along the coding sequence of TP53 for each stop codon type. Each panel represents a drug-specific prediction: G418 (top), CC90009 (middle) and SJ6986 (bottom). b , Same as a , but drugs are represented as colors and each panel belongs to a different stop type.
Supplementary information
Supplementary information.
Supplementary Notes 1–8 and Supplementary Fig. 1.
Reporting Summary
Supplementary data.
Supporting data for Supplementary Fig. 1.
Supplementary Tables
Supplementary Table 1: Single-variant validation experiments. DMS and individual SRI-stimulated readthrough measurements for the 25 variants used for validation experiments. The s.d. across two replicates is provided. The ‘upper_limit_variant’ column indicates if the variant was tested on the first validation round or on the second round (to specifically define the upper limit of the assay). Supplementary Table 2: Readthrough compounds. All readthrough compounds were tested, along with the concentration and solvent used and the company/lab they were obtained from. For the compounds whose reported readthrough stimulation could not be reproduced in our assay (validated = ‘no’), the range of concentrations tested is provided. Supplementary Table 3: PTC dataset. Readthrough measurements for all PTC variants across all drugs and the untreated condition. Supplementary Table 4: Sequence features tested for readthrough association. All 47 sequence features whose role in readthrough regulation was tested during model generation. The first column shows the feature short name used throughout the paper, whereas the second column provides a more detailed explanation. Supplementary Table 5: TP53 and PTEN mutants. Readthrough measurements across all conditions for the nine highly prevalent PTEN ( n = 4) and TP53 ( n = 5) mutants addressed in Fig. 3d and Extended Data Fig. 3f . Supplementary Table 6: Clinical trials. A list of the 42 clinical trials testing readthrough compounds is revised in this manuscript. Relevant information such as the drug tested, the patient’s pathology, the number, age and gender of patients, the clinical phase and the availability of mutational data are provided. Supplementary Table 7: Predictions for the PTC drug-specific models. Observed and predicted readthrough measurements obtained across the ten rounds of cross-validation (90–10% training-testing data) during model training. The ‘treatment’ column indicates the PTC drug-specific model. Supplementary Table 8: Predictions for the PTC pan-drug model. Observed and predicted readthrough measurements obtained across the ten rounds of cross-validation (90–10% training-testing data) during model training for the PTC pan-drug model. Supplementary Table 9: PTC drug-specific model coefficients. Model coefficients for the PTC drug-specific models. The ‘fold’ columns show the coefficients obtained in each cross-validation round. The ‘mean’, ‘s.d.’ and ‘ci95’ columns show the mean, s.d. and 95% confidence intervals across the tenfolds. A two-sided t test was performed to interrogate the null hypothesis that the coefficient equals 0. P values and adjusted P values (false discovery rate (FDR) = 0.05) are shown. The ‘feature’ and ‘terms’ columns indicate the sequence feature and specific feature level, respectively. Supplementary Table 10: PTC pan-drug model coefficients. Model coefficients for the PTC pan-drug model. The ‘fold’ columns show the coefficients obtained in each cross-validation round. The ‘mean’, ‘s.d.’ and ‘ci95’ columns show the mean, s.d. and 95% confidence intervals across the tenfolds. A two-sided t test was performed to interrogate the null hypothesis that the coefficient equals 0. P values and adjusted P values (FDR = 0.05) are shown. The ‘feature’ and ‘terms’ columns indicate the sequence feature and specific feature level, respectively. Supplementary Table 11: NTC dataset. Readthrough measurements for all NTC variants across all drugs and the untreated condition. Supplementary Table 12: Predictions for the NTC drug-specific models. Observed and predicted readthrough measurements obtained across the ten rounds of cross-validation (90–10% training-testing data) during model training. The ‘treatment’ column indicates the NTC drug-specific model. Supplementary Table 13: NTC drug-specific model coefficients. Model coefficients for the NTC drug-specific models. The ‘fold’ columns show the coefficients obtained in each cross-validation round. The ‘mean’, ‘s.d.’ and ‘ci95’ columns show the mean, s.d. and 95% confidence intervals across the tenfolds. A two-sided t test was performed to interrogate the null hypothesis that the coefficient equals 0. P values and adjusted P values (FDR = 0.05) are shown. The ‘feature’ and ‘terms’ columns indicate the sequence feature and specific feature level, respectively. Supplementary Table 14: Primers. List of primers used for plasmid generation and library preparation.
Source Data Fig. 1
Statistical source data.
Source Data Fig. 2
Source data fig. 3, source data fig. 4, source data fig. 5, source data fig. 6, source data extended data fig. 1, source data extended data fig. 2, source data extended data fig. 3, source data extended data fig. 4, source data extended data fig. 5, rights and permissions.
Open Access This article is licensed under a Creative Commons Attribution 4.0 International License, which permits use, sharing, adaptation, distribution and reproduction in any medium or format, as long as you give appropriate credit to the original author(s) and the source, provide a link to the Creative Commons licence, and indicate if changes were made. The images or other third party material in this article are included in the article’s Creative Commons licence, unless indicated otherwise in a credit line to the material. If material is not included in the article’s Creative Commons licence and your intended use is not permitted by statutory regulation or exceeds the permitted use, you will need to obtain permission directly from the copyright holder. To view a copy of this licence, visit http://creativecommons.org/licenses/by/4.0/ .
Reprints and permissions
About this article
Cite this article.
Toledano, I., Supek, F. & Lehner, B. Genome-scale quantification and prediction of pathogenic stop codon readthrough by small molecules. Nat Genet (2024). https://doi.org/10.1038/s41588-024-01878-5
Download citation
Received : 01 August 2023
Accepted : 23 July 2024
Published : 22 August 2024
DOI : https://doi.org/10.1038/s41588-024-01878-5
Share this article
Anyone you share the following link with will be able to read this content:
Sorry, a shareable link is not currently available for this article.
Provided by the Springer Nature SharedIt content-sharing initiative
Quick links
- Explore articles by subject
- Guide to authors
- Editorial policies
Sign up for the Nature Briefing: Translational Research newsletter — top stories in biotechnology, drug discovery and pharma.

- Future Perfect
The staggering death toll of scientific lies
Scientific fraud kills people. Should it be illegal?
by Kelsey Piper

You probably haven’t heard of cardiologist Don Poldermans, but experts who study scientific misconduct believe that thousands of people may be dead because of him.
Poldermans was a prolific medical researcher at Erasmus Medical Center in the Netherlands, where he analyzed the standards of care for cardiac surgery, publishing a series of definitive studies from 1999 until the early 2010s.
One crucial question he studied: Should you give patients a beta blocker, which lowers blood pressure, before certain heart surgeries? Poldermans’s research said yes. European medical guidelines (and to a lesser extent US guidelines) recommended it accordingly .
The problem? Poldermans’s data was reportedly fake . A 2012 inquiry by Erasmus Medical School, his employer, into allegations of misconduct found that he “used patient data without written permission, used fictitious data and… submitted to conferences [reports] which included knowingly unreliable data.” Poldermans admitted the allegations and apologized , while stressing that the use of fictitious data was accidental.
This story was first featured in the Future Perfect newsletter .
Sign up here to explore the big, complicated problems the world faces and the most efficient ways to solve them. Sent twice a week.
After the revelations, a new meta-analysis was published in 2014, evaluating whether to use beta blockers before cardiac surgery. It found that a course of beta blockers made it 27 percent more likely that someone would die within 30 days of their heart surgery. That is, the policy which Poldermans had recommended using falsified data, adopted in Europe on the basis of his research, was actually dramatically increasing the odds people would die in heart surgery.
Tens of millions of heart surgeries were conducted across the US and Europe during the years from 2009 to 2013 when those misguided guidelines were in place. One provocative analysis from cardiologists Graham Cole and Darrel Francis estimated that there were 800,000 deaths compared to if the best practices had been established five years sooner. While that exact number is hotly contested, a 27 percent increase in mortality for a common procedure for years on end can add up to an extraordinary death toll.
I learned about the Poldermans case when I reached out to some scientific misconduct researchers, asking them a provocative question: Should scientific fraud be prosecuted?
Unfortunately, fraud and misconduct in the scientific community isn’t nearly as rare as one might like to believe. We also know that the consequences of being caught are frequently underwhelming. It can take years to get a bad paper retracted, even if the flaws are readily apparent. Sometimes, scientists alleged to have falsified their data file frivolous lawsuits against their peers who point it out, further silencing anyone who would speak out about bad data. And we know that this behavior can have high stakes, and can dramatically affect treatment options for patients .
In cases where research dishonesty is literally killing people, shouldn’t it be appropriate to resort to the criminal justice system?
The question of whether research fraud should be a crime
In some cases, research misconduct may be hard to distinguish from carelessness.
If a researcher fails to apply the appropriate statistical correction for multiple hypothesis testing, they will probably get some spurious results. In some cases, researchers are heavily incentivized to be careless in these ways by an academic culture that puts non-null results above all else (that is, rewarding researchers for finding an effect even if it is not a methodologically sound one, while being unwilling to publish sound research if it finds no effect).
But I’d argue it’s a bad idea to prosecute such behavior. It would produce a serious chilling effect on research, and likely make the scientific process slower and more legalistic — which also results in more deaths that could be avoided if science moved more freely.
So the conversation about whether to criminalize research fraud tends to focus on the most clear-cut cases: intentional falsification of data. Elisabeth Bik, a scientific researcher who studies fraud, made a name for herself by demonstrating that photographs of test results in many medical journals were clearly altered . That’s not the kind of thing that can be an innocent mistake, so it represents something of a baseline for how often manipulated data is published.
While technically some scientific fraud could fall under existing statutes that prohibit lying on, say, a grant application, in practice scientific fraud is more or less never prosecuted. Poldermans eventually lost his job in 2011, but most of his papers weren’t even retracted, and he faced no further consequences.
But in response to growing awareness of fraud’s frequency and its harms, some scientists and scientific-fraud watchdogs have proposed changing that . A new statute, narrowly tailored to scientific fakery, could make it clearer where to draw the line between carelessness and fraud.
The question is whether legal consequences would actually help with our fraud problem. I asked Bik what she thought about proposals to criminalize the misconduct that she studied.
Her reaction was that, while it’s not clear whether criminalization is the right approach, people should understand that currently there are almost no consequences for wrongdoers. “It’s maddening when you see people cheat,” she told me, “And even if it involves grant money from the NIH, there’s very little punishment. Even with people who have been caught cheating, the punishment is super light. You are not eligible to apply for new grants for the next year or sometimes three years. It’s very rare that people lose jobs over it.”
Why is that? Fundamentally, it’s a problem of incentives. It is embarrassing for institutions when one of their researchers commits misconduct, so they’d rather impose a mild penalty and not keep digging. There’s little incentive for anyone to get to the bottom of misconduct. “If the most serious consequence for speeding was a police officer saying ‘Don’t do that again,’ everyone would be speeding,” Bik told me. “This is the situation we have in science. Do whatever you want. If you get caught, it’ll take years to investigate.”
In some ways, a legal statute isn’t the ideal solution. Courts are also guilty of taking years to deliver justice in complex cases. They also aren’t well suited to answering detailed scientific questions, and would almost certainly be relying on scientific institutions that conduct investigations — so what really matters is those institutions, not whether they’re attached to a court, a nonprofit, or to the NIH.
But in sufficiently severe cases of misconduct, it does seem to me that it’d be a major advantage to have an institution outside academia at work on getting to the bottom of these cases. If well designed, a statute that allowed prosecution for scientific fraud could shift the overwhelming incentives to let misconduct go unpunished and move on.
If there were ongoing investigations conducted by an outside agency (like a prosecutor), it would no longer be easiest for institutions to maintain their reputation by sweeping incidents of fraud under the rug. But the outside agency would not actually have to be a prosecutor; an independent scientific review board would probably also suffice, Bik said.
Ultimately, prosecution is a blunt tool. It might help provide accountability in cases where no one is incentivized to provide it — and I do think in cases of misconduct that lead to thousands of deaths, it would be a matter of justice. But it’s neither the only way to solve our fraud problem nor necessarily the best one.
So far, however, efforts to build institutions within the scientific community that police misconduct have had only limited success. At this point, I’d consider it a positive if there were efforts to allow external institutions to police misconduct as well.
Most Popular
- Michelle Obama articulated something Democrats have been afraid to say
- The massive Social Security number breach is actually a good thing
- The Supreme Court decides not to disenfranchise thousands of swing state voters
- Kamala Harris just revealed her formula for taking down Trump
- The moment when Kamala Harris’s speech came alive
Today, Explained
Understand the world with a daily explainer plus the most compelling stories of the day.
This is the title for the native ad
More in Future Perfect

Organ companies are getting up to pancreas hijinks.

Even OpenAI warns that chatting with an AI voice can breed “emotional reliance.”

However current companies do financially, the big AI safety challenges remain.

But there might be global consequences.

Researchers wildly underestimated how many people don’t have safe drinking water.

A first case outside of the continent has been detected in Sweden. What’s next?

IMAGES
COMMENTS
A hypothesis test can either contain a directional hypothesis or a non-directional hypothesis: Directional hypothesis: The alternative hypothesis contains the less than ("<") or greater than (">") sign. This indicates that we're testing whether or not there is a positive or negative effect. Non-directional hypothesis: The alternative ...
Directional Hypothesis Examples. 1. Exercise and Heart Health. Research suggests that as regular physical exercise (independent variable) increases, the risk of heart disease (dependent variable) decreases (Jakicic, Davis, Rogers, King, Marcus, Helsel, Rickman, Wahed, Belle, 2016). In this example, a directional hypothesis anticipates that the ...
This null hypothesis can be written as: H0: X¯ = μ H 0: X ¯ = μ. For most of this textbook, the null hypothesis is that the means of the two groups are similar. Much later, the null hypothesis will be that there is no relationship between the two groups. Either way, remember that a null hypothesis is always saying that nothing is different.
HYPOTHESIS TESTING. A clinical trial begins with an assumption or belief, and then proceeds to either prove or disprove this assumption. In statistical terms, this belief or assumption is known as a hypothesis. Counterintuitively, what the researcher believes in (or is trying to prove) is called the "alternate" hypothesis, and the opposite ...
One-tailed hypothesis tests are also known as directional and one-sided tests because you can test for effects in only one direction. When you perform a one-tailed test, the entire significance level percentage goes into the extreme end of one tail of the distribution. In the examples below, I use an alpha of 5%.
Two-tailed tests consider both positive and negative deviations from the null hypothesis, ensuring accurate and reliable statistical analysis of survey data. Exploratory Research: Non-directional hypotheses are particularly useful in exploratory research, where the goal is to gather initial insights and generate hypotheses. Surveys with non ...
5.2 - Writing Hypotheses. The first step in conducting a hypothesis test is to write the hypothesis statements that are going to be tested. For each test you will have a null hypothesis ( H 0) and an alternative hypothesis ( H a ). When writing hypotheses there are three things that we need to know: (1) the parameter that we are testing (2) the ...
Abstract. Statistical hypothesis testing is common in research, but a conventional understanding sometimes leads to mistaken application and misinterpretation. The logic of hypothesis testing presented in this article provides for a clearer understanding, application, and interpretation. Key conclusions are that (a) the magnitude of an estimate ...
When the research question asks "Does the independent variable affect the dependent variable?": The null hypothesis ( H0) answers "No, there's no effect in the population.". The alternative hypothesis ( Ha) answers "Yes, there is an effect in the population.". The null and alternative are always claims about the population.
Null Hypothesis (H 0): The sample data occurs purely from chance. Alternative Hypothesis (H A): The sample data is influenced by some non-random cause. A hypothesis test can either contain a directional hypothesis or a non-directional hypothesis: Directional hypothesis: The alternative hypothesis contains the less than ("") sign. This ...
Types of research hypotheses include inductive and deductive, directional and non-directional, and null and alternative hypotheses. Rejecting the null hypothesis and accepting the alternative hypothesis is the basis for building a good research study. This work reviews the most important aspects of organizing and establishing an efficient and ...
Examples. A research hypothesis, in its plural form "hypotheses," is a specific, testable prediction about the anticipated results of a study, established at its outset. It is a key component of the scientific method. Hypotheses connect theory to data and guide the research process towards expanding scientific understanding.
A tutorial on a practical Bayesian alternative to null-hypothesis significance testing. Behavior research methods, 43, 679-690. Nickerson, R. S. (2000). Null hypothesis significance testing: a review of an old and continuing controversy. Psychological methods, 5(2), 241. Rozeboom, W. W. (1960). The fallacy of the null-hypothesis significance test.
The actual test begins by considering two hypotheses.They are called the null hypothesis and the alternative hypothesis.These hypotheses contain opposing viewpoints. \(H_0\): The null hypothesis: It is a statement of no difference between the variables—they are not related. This can often be considered the status quo and as a result if you cannot accept the null it requires some action.
There are seven different types of research hypotheses. Simple Hypothesis. A simple hypothesis predicts the relationship between a single dependent variable and a single independent variable. Complex Hypothesis. A complex hypothesis predicts the relationship between two or more independent and dependent variables. Directional Hypothesis.
10.1 - Setting the Hypotheses: Examples. A significance test examines whether the null hypothesis provides a plausible explanation of the data. The null hypothesis itself does not involve the data. It is a statement about a parameter (a numerical characteristic of the population). These population values might be proportions or means or ...
In scientific research, a non-directional hypothesis, or null hypothesis, is a statement that suggests the absence of a relationship or difference between the variables being studied. This type of hypothesis is used to test the validity of a research question by assuming that there is no significant effect or relationship between the variables ...
To distinguish it from other hypotheses, the null hypothesis is written as H 0 (which is read as "H-nought," "H-null," or "H-zero"). A significance test is used to determine the likelihood that the results supporting the null hypothesis are not due to chance. A confidence level of 95% or 99% is common. Keep in mind, even if the confidence level is high, there is still a small chance the ...
INTRODUCTION. Scientific research is usually initiated by posing evidenced-based research questions which are then explicitly restated as hypotheses.1,2 The hypotheses provide directions to guide the study, solutions, explanations, and expected results.3,4 Both research questions and hypotheses are essentially formulated based on conventional theories and real-world processes, which allow the ...
If the researcher makes a directional hypothesis, I would assume that he will need to run a one-tailed test. From what I remember, if the results go the opposite direction--i.e., reaction time increases--our researcher failed to reject the null. ... The connection between the research hypothesis and the choice of null an alternative is not writ ...
Note that this is a directional hypothesis since we are posting a negative relationship. Typically, a directional hypothesis implies a one-tailed test where the critical value is 0.05 on one side of the distribution. A non-directional hypothesis, β≠0β≠0 does not imply a particular direction, it only implies that there is a relationship ...
In this video, I have discussed about1. Hypothesis, 2. Types of hypothesis (null hypothesis, alternative hypothesis, non directional hypothesis, and directio...
A Null Hypothesis is denoted as an H0. This is the type of hypothesis that the researcher tries to invalidate. Some of the examples of null hypotheses are: - Hyperactivity is not associated with eating sugar. - All roses have an equal amount of petals. - A person's preference for a dress is not linked to its color.
A two-sided t test was performed to interrogate the null hypothesis that the coefficient equals 0. P values and adjusted P values (FDR = 0.05) are shown. The 'feature' and 'terms' columns ...
From Don Poldersmans and Francesca Gino to faked Alzheimer's data, the scientific field needs a better handle on allegations and consequences of fraudulent research.
Tests of general relativity serve to establish observational evidence for the theory of general relativity.The first three tests, proposed by Albert Einstein in 1915, concerned the "anomalous" precession of the perihelion of Mercury, the bending of light in gravitational fields, and the gravitational redshift.The precession of Mercury was already known; experiments showing light bending in ...