To read this content please select one of the options below:
Please note you do not have access to teaching notes, chatbots and virtual assistants: a bibliometric analysis.
Library Hi Tech
ISSN : 0737-8831
Article publication date: 8 July 2022
Issue publication date: 22 August 2022
This paper attempts to provide significant information on the increased growth of literature of chatbots and virtual assistants. Technological changes are dynamic and keep changing at regular intervals. Therefore, it becomes highly crucial to review the performance of chatbots and virtual assistants. This paper aims to review the literature by eminent researchers in the form of authors, keywords and major contributing organizations.

Design/methodology/approach
Systematic literature review and bibliometric analysis is used to analyze the growth of literature on chatbots. A sum of articles has been extracted from Scopus database with selected keywords and with certain filters. VOSviewer software is used for analysis of data. A total of 130 documents are extracted from Scopus database from 2017 to 2021 (31st August).
This study provides a significant contribution to an existing literature and provides the understanding of research in the area of chatbots and virtual assistants. The authors with maximum number of citations are Yan, Zaho, Bengio, Weizenbaum, Song, Zhou and Maedche with jointly 180 citations. Research is been contributed by different countries where the United States is the country with highest number of documents published. The United States contributes 17% of the total production in the area of chatbots and virtual assistants. The analysis shows that the area is gaining momentum as contribution in this area is been increasing in last few years.
Research limitations/implications
The study shows that several branches of chatbots are also in mainstream like natural language processing, e-learning, behavioural research, conversational agents, virtual assistants, human–computer interaction, natural language and so on. It provides a wider scope to authors and researchers to gain useful insights. The bibliometric study will provide a broader spectrum in this area.
Social implications
The developments in technology and also the effect of COVID-19 pandemic is boosting the adoption of technology in different sectors. Deployment of technology will uplift the economy and social infrastructure. Society can avail different services from their comfort area and also in real time. This will help in reducing wastage of resources like people visiting offices for routine jobs which can be easily availed from their workplace. Society may access better services without much human interaction.
Originality/value
This paper adds significant contribution to the existing literature by analysing the published papers from Scopus database. The study contains new and significant information as this study covers all industries where chatbots and virtual assistants are being applied whereas in previous literature only specific industry has been taken into consideration.
- Virtual assistants
- Systematic literature review
- Bibliometric analysis
Agarwal, S. , Agarwal, B. and Gupta, R. (2022), "Chatbots and virtual assistants: a bibliometric analysis", Library Hi Tech , Vol. 40 No. 4, pp. 1013-1030. https://doi.org/10.1108/LHT-09-2021-0330
Emerald Publishing Limited
Copyright © 2022, Emerald Publishing Limited
Related articles
All feedback is valuable.
Please share your general feedback
Report an issue or find answers to frequently asked questions
Contact Customer Support
- DOI: 10.5220/0009417600970103
- Corpus ID: 219317858
Virtual Assistants for Learning: A Systematic Literature Review
- R. Gubareva , Rui Pedro Lopes
- Published in International Conference on… 2020
- Education, Computer Science
Figures and Tables from this paper
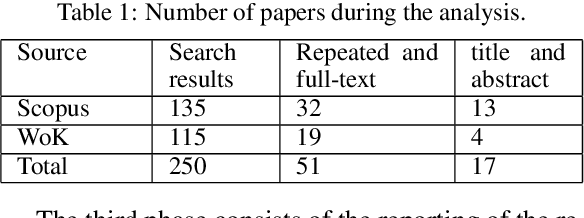
32 Citations
Interactive video virtual assistant framework with retrieval augmented generation for e-learning, a virtual companion for lifelong learning – design principles for motivation, social learning, and exam preparation, augmenting virtual labs with artificial intelligence for hybrid learning, integration of ai into the distance learning environment: enhancing soft skills, deriving design knowledge for elearning companions to support international students.
- Highly Influenced
A Service Perspective on Designing Learning Companions as Bonding and Mindful Time Managers in Further Education
Conversational agents in education – a systematic literature conversational agents in education – a systematic literature review review, a literature review on pedagogical conversational agent adaptation, towards pedagogical conversational agents as creativity drivers in virtual worlds, embracing the future: unveiling the revolution of human-ai interaction in the digital education era, 29 references, utilising a virtual learning assistant as a measurement and intervention tool for self-regulation in learning, céos: a collaborative web-based application for improving teaching-learning strategies, a virtual assistant for web-based training in engineering education, gamified learning in higher education: a systematic review of the literature, students’ learning autonomy: a systematic literature review, an intelligent assistant to support students and to prevent them from dropout, developing virtual teaching assistants for open e-learning platforms, tutoria no ensino superior: concepções e práticas, a multi-model and ai-based collegebot management system (aicms) for professional engineering colleges, an investigation into the efficacy of avatar-based systems for student advice, related papers.
Showing 1 through 3 of 0 Related Papers
Open Access is an initiative that aims to make scientific research freely available to all. To date our community has made over 100 million downloads. It’s based on principles of collaboration, unobstructed discovery, and, most importantly, scientific progression. As PhD students, we found it difficult to access the research we needed, so we decided to create a new Open Access publisher that levels the playing field for scientists across the world. How? By making research easy to access, and puts the academic needs of the researchers before the business interests of publishers.
We are a community of more than 103,000 authors and editors from 3,291 institutions spanning 160 countries, including Nobel Prize winners and some of the world’s most-cited researchers. Publishing on IntechOpen allows authors to earn citations and find new collaborators, meaning more people see your work not only from your own field of study, but from other related fields too.
Brief introduction to this section that descibes Open Access especially from an IntechOpen perspective
Want to get in touch? Contact our London head office or media team here
Our team is growing all the time, so we’re always on the lookout for smart people who want to help us reshape the world of scientific publishing.
Home > Books > Virtual Assistant
Introductory Chapter: Virtual Assistants
Submitted: 05 May 2021 Published: 13 October 2021
DOI: 10.5772/intechopen.100248
Cite this chapter
There are two ways to cite this chapter:
From the Edited Volume
Virtual Assistant
Edited by Ali Soofastaei
To purchase hard copies of this book, please contact the representative in India: CBS Publishers & Distributors Pvt. Ltd. www.cbspd.com | [email protected]
Chapter metrics overview
770 Chapter Downloads
Impact of this chapter
Total Chapter Downloads on intechopen.com

Total Chapter Views on intechopen.com
Author Information
Ali soofastaei *.
- Artificial Intelligence Center, Vale, Brisbane, Australia
*Address all correspondence to: [email protected]
1. Introduction
The application of Virtual Assistants (VAs) is growing fast in our personal and professional life. It has been predicted that 25% of households using a VA will have two or more devices by 2021 [ 1 ]. A virtual assistant is an intelligent application that can perform tasks or provide services for a person responding to orders or inquiries. Some VAs can understand and respond to human speech using synthesized voices. Users may use voice commands to request their VA to answer the questions, manage home appliances, control media playing, and handle other essential activities like email, creating the actions lists, and organize the meetings on calendars [ 2 ]. In the Internet of Things (IoT) world, an VA is a popular service to communicate with users based on voice command.
VA capabilities and usage are rapidly rising, thanks to new technologies reaching the people’s requirements and a robust focus on voice user interfaces. Samsung, Google, and Apple each have a considerable smartphone user base. Microsoft’s Windows-based personal computers, smartphones, and smart speakers have an intelligent VA installed base. On Amazon, smart speakers have a sizable installed base [ 3 ]. Over 100 million people have used Conversica’s short message and email interface Intelligent Virtual Assistants (IVAs) services in their companies.
Famous virtual assistants like Amazon Alexa and Google Assistant are typically cloud-based for maximum performance and data management. Many behavioral traces, including the user’s voice activity history with extensive descriptions, can be saved in a VA ecosystem’s remote cloud servers during this process.
The VAs story started in the 1910s, and the growth of technology has supported VAs’ improvement. The application of Artificial Intelligence (AI) also was a turning point in VAs journey. Using AI to develop the VAs was a great jump to increase the VAs’ capabilities. Currently, VAs use narrow AI with limited options. However, using general AI in the near future can be a revolution to improve the quality of VAs’ services.
2. Backgrounds
2.1 investigational years: 1910s: 1980s.
In 1922, an interesting toy named Radio Rex was introduced that was the first voice-activated doll [ 4 ]. A toy in the dog shape would appear from its den the moment it was given a name.
Bell Labs introduced the “Audrey,” which was an Automatic Digit Identification device in 1952. It took up a six-foot-high relay rack, used much power, had many wires, and had all of the issues that come with complicated vacuum-tube electronics. Despite this, Audrey was able to discriminate between phonemes, which are the basic components of speech. However, it was restricted to precise digit identification by assigned speakers. As a result, it may be utilized for voice dialing. However, push-button dialing was generally less expensive and faster than pronouncing the digits in order [ 5 ].
Another early gadget that could carry out digital language identification was Shoebox voice-activated calculator that IBM developed. It was revealed to the public for the period of the 1962 Seattle World’s Fair after its first market debut in 1961. This initial machine, which was built nearly twenty years earlier than the first Personal Computer made by IBM and debuted in 1981, was capable of detecting sixteen verbal phrases and the numbers 0 through 9.
ELIZA, the first Natural Language Processing (NLP) application or chatbot, was invented by MIT in the 1960s. ELIZA was designed in order to “show that man-machine interaction is essentially superficial” [ 6 ]. It applied configuration matching and replacement procedures in written reactions to simulate conversation, creating the impression that the machine understood what was being said.
The ELIZA was designed by professor Joseph Weizenbaum. During the ELIZA development period, Joseph’s assistant has requested that he leave the room so that she and ELIZA can chat. Professor Weizenbaum later remarked, “I had no idea that brief exposures to a really simple computer software might cause serious delusional thinking in otherwise normal people [ 7 ].” The ELIZA impact, or the tendency to instinctively believe machine activities are equal to people’s behaviors, was called after this. Anthropomorphizing is a phenomenon that occurs in human interactions with VAs.
When DARPA funded a five-year Speech Understanding Research effort at Carnegie Mellon in the 1970s, the goal was to reach a vocabulary of 1,000 words. Participants included IBM, Carnegie Mellon University (CMU), and Stanford Research Institute, among many others.
The result was “Harpy,” a robot that could understand speech and knew around 1000 words, roughly equivalent to a three-year vocabulary. To reduce voice recognition failures, it could also analyze speech that followed pre-programmed vocabularies, pronunciations, and grammatical patterns to determine which word sequences made sense when spoken.
An improvement to the Shoebox was released in 1986 with the Tangora, a speech recognition typewriter. With a vocabulary of 20,000 words, it was able to anticipate the most likely outcome based on its information. Because of this, it was given the name “Fastest Typewriter. As part of its digital signal processing, IBM used a Hidden Markov model, which integrates statistics into the Using this strategy, you may anticipate which phonemes will follow a given phoneme. However, every speaker was responsible for training the typewriter to recognize his or her voice and halt in.
2.2 The beginning of intelligent virtual assistants: 1990s: Present
To compete for customers in the 1990s, companies such as IBM, Philips, and Lemont & Hauspie began integrating digital voice recognition into personal computers. The first smartphone introduced in 1994, the IBM Simon laid the groundwork for today’s smart virtual assistant.
In 1997, Dragon’s Biologically Talking application was able to detect and transcribe natural human speech at a pace of 100 words per minute, with no gaps between syllables. Biologically Talking is still accessible for download, and many doctors in the United States and the United Kingdom continue to use it to keep track of their medical records.
In 2001, Colloquies released Smarter Child on AIM and MSN Messenger, among other platforms. “Smarter Child” can play games and check the weather as well as seek up data. It can even speak with others to a certain extent, even if it is text.
Siri, which debuted on October 4, 2011, as an option of the iPhone 4S, was the first innovative digital VA to be placed on a smartphone [ 8 , 9 ]. Siri was built when Apple Inc. purchased Siri Inc. in 2010, a spin-off of SRI International, a research institute financed by DARPA and the US Department of Defense [ 10 ]. It was created to make texting, making phone calls, checking the weather, and setting the alarm easier. In addition, it can now make restaurant recommendations, perform Internet searches, and offer driving directions.
Amazon debuted Alexa alongside the Echo in November 2014. Later, in April 2017, Amazon launched a facility that allows users to create conversational interfaces for any VA or interface.
From 2017 till 2021, all the VAs mentioned above have been developed, and there are the more intelligent VAs using for individuals and professional activities. The companies in different areas use the VAs to improve the quality of their decisions at different levels, from operation to the high management level.
3. Virtual assistants - method of interaction
Text, including online chat, text messages, email, as well as other text-based modes of interaction, for instance, Conversica’s IVAs for enterprise [ 11 ].
Voice, for instance, with Siri on an iPhone (Apple products), Google Assistant on Android mobile smartphones, or Amazon Alexa [ 12 ] on the Amazon Echo device.
By shooting and uploading photos, as Bixby on the Samsung Galaxy does.
Various VAs, such as Google Assistant, are available in several ways, including chat on the Google Allo and Google Messages apps and voice on Google Home smart speakers.
VAs use NLP to translate text input from the user or voice input into executable. Furthermore, many people use AI techniques, such as machine learning, to learn continuously. Some of these assistants, such as Google Assistant (which includes Google Lens) and Samsung Bixby, can also perform image processing to distinguish things in the image, allowing users to obtain better results from the images they have clicked.
The awake phrase could be used to activate a voice-activated assistant. These words, for example, are “OK Google,” or “Hey Google,” “Hey Siri,” “Alexa,” and “Hey Microsoft” [ 13 ]. However, there are increasing legal dangers associated with VAs as they become more popular [ 14 ].
4. Virtual assistants: Services
Set the alarm, construct to-do and shopping lists, and support data such as weather;
Play TV shows, serials, and films on TVs, running from, e.g., Amazon Prime, YouTube;
Play music from the platforms such as Pandora, YouTube, Spotify, and; podcasts, and read journals and audiobooks;
Assist citizens in their dealings with the government;
Conversational business; and
Humans should be used to supplement and replace customer service [ 16 ]. For example, one study indicated that an automated online assistant reduced the burden of a human-staffed call center by 30% [ 17 ].
5. Virtual assistants - ethics implications
Generally, the consumers provide free data for the preparation and development of AI algorithms and VAs, which is often ethically disturbing. However, knowing how the applied intelligent models are developed using the consumers’ data and information could be more ethically troubling.
Most VAs on the market use Artificial Neural Networks (ANNs) to train AI algorithms, requiring many labeled data. In order to understand the increase in microwork over the past decade, however, this information must be categorized by a human being in order to understand the increase in microwork over the past decade. However, a human being must categorize this information. People worldwide are paid to perform repetitive and incredibly simple tasks, such as listening and copying down voice input from a virtual assistant for a few cents. Because of the insecurity it creates and the lack of control, microwork has been called out as a problem. The average hourly wage was 1.38 dollars [ 18 ], with no healthcare, sick pay, retirement benefits, or minimum salary. This has led to a dispute between VAs and their designers over employment insecurity, and the AIs they propose are still human in a way that would be impossible without millions of human workers micromanaging them [ 19 ].
A VA provider’s unencrypted access to voice commands raises privacy concerns since they can be shared with third parties and handled unlawfully or unexpectedly [ 20 ]. Along with language content, a user’s style of expression and voice features can provide information about his or her biometric identification, personality attributes, physical and mental health condition, sex and gender, moods and emotions, and socioeconomic status and geographic origin [ 21 ].
6. Comparison of notable virtual assistants
Different AI products work as VA in the market. Each product has been designed to provide the assistant service for the specific product. There also are different brands of VAs, and behind them are genius companies who annually are investing billion dollars in this field. Table 1 shows a shortlist of the most used VAs and their capabilities.
Virtual Assistant | Developer | IOT | Chromecast Integration | Smart Phone App |
---|---|---|---|---|
Alexa | Amazon | Yes | No | Yes |
Alice | Yandex | Yes | No | Yes |
AliGenie | Alibaba | Yes | No | Yes |
Assistant | Speaktoit | No | No | Yes |
Bixby | Samsung | No | No | Yes |
BlackBerry Assistant | BlackBerry | No | No | Yes |
Braina | Brain soft | No | No | Yes |
Clova | Naver | Yes | No | Yes |
Cortana | Microsoft | Yes | No | Yes |
Duer | Baidu | N/A | N/A | N/A |
Evi | Amazon | No | No | Yes |
Google Assistant | Yes | Yes | Yes | |
Google Now | Yes | Yes | Yes | |
M | N/A | N/A | N/A | |
Mycroft | Mycroft | Yes | Yes | Yes |
SILVIA | Cognitive Code | No | No | Yes |
Siri | Apple Inc. | Yes | No | Yes |
Viv | Samsung | Yes | No | Yes |
Xiaowei | Tencent | N/A | N/A | N/A |
Celia | Huawei | Yes | No | Yes |
Notable virtual assistants.
7. Virtual assistants – Financial importance
7.1 for persons.
Digital experiences facilitated by VAs are one of the most encouraging end-user trends in recent years. Specialists predict that digital practices would gain a prestige equivalent to ‘real’ ones, if not more sought-after and valued [ 22 ]. The development is supported by frequent users and a significant increase in the number of virtual digital assistant users worldwide. The number of people who use digital VAs regularly was predicted to be approximately 1 billion in mid-2017 [ 23 ]. Furthermore, virtual digital assistant technology is no longer limited to smartphone apps but is also found in many different industries [ 24 ]. There will be a 34.9 percent CAGR for speech recognition technology from 2016 to 2024, surpassing a global market size of US$7.5 billion by 2024 [ 25 ] as a result of considerable R&D expenditures of enterprises across all sectors and increasing use of mobile devices in speech recognition technology [ 24 ].
According to an Ovum estimate, by 2021, the “native digital assistant installed base” will outnumber the global population, with 7.5 billion active speech AI-capable devices [ 25 ]. “Google Assistant would dominate the speech AI–capable device market with 23.3 percent market share by that time,” according to Ovum, “followed by Samsung’s Bixby (14.5 percent), Apple’s Siri (13.1 percent), Amazon’s Alexa (3.9 percent), and Microsoft’s Cortana (2.3 percent)” [ 25 ].
Businesses in North America (such as Nuance Communications and IBM) are projected to dominate the sector over the next few years due to BYOD (Bring Your Own Device) and enterprise mobility business strategies. Furthermore, the growing demand for smartphone-assisted platforms will likely propel IVA’s further growth in North America. On the other hand, even though it is smaller than the North American market [ 24 ], the intelligent VA sector in the Asia-Pacific area is predicted to expand at a 40 percent annual growth rate (above the world average) between 2016 and 2024, with its primary players located in India and China.
7.2 For companies
VAs should not be viewed solely as a tool for individuals, as they may have genuine economic value for businesses. A virtual assistant, for example, can serve as an always-available aide with encyclopedia knowledge. Furthermore, it can organize meetings, checking inventories, and verifying data. VAs, on the other hand, are so significant that their integration into small and medium-sized businesses is frequently a simple first step towards a more worldwide adaption and use of IoT. Small and medium-sized enterprises regard IoT technologies as critical technologies that are difficult, risky, or expensive to employ [ 26 ].
8. Virtual assistants: Protection
To demonstrate how audio commands can be directly integrated into music or spoken text, researchers from the University of California, Berkeley, published a study in May 2018. The publication showed that VAs could perform specified actions without the user’s knowledge. The researchers altered audio files to eliminate the sound patterns that speech recognition algorithms are designed to recognize. Instead, noises were used to direct the system to dial numbers on the phone, launch webpages, or even move money [ 27 ]. Since 2016 [ 27 ], this has been a possibility, and it impacts Apple, Amazon, and Google devices [ 28 ].
Security and privacy concerns with IVAs are not limited to unwanted actions or voice recording. For example, when a person pretends to be someone else, he or she uses malevolent voice commands to gain illegal access to their home or garage, such as unlocking a smart door or shopping items online without their The system may have trouble distinguishing between similar sounds, even though some IVAs have a voice-training feature to prevent imitating. In addition, the system may be fooled into believing that the user is the real owner if a malicious individual has access [ 29 ] to an IVA-enabled device.
9. Virtual assistants: A new definition
From 2020 when the world faced the COVID-19 pandemic, and most people had to work remotely from home, the VA found the new definition. A human VA, also known as a virtual office assistant, is a self-employed person who works remotely from a home office to give clients professional administrative, technical, or creative (social) help [ 30 ]. Unless these indirect costs are included in the VA fees, clients are not liable for employee-related taxes and insurance or benefits because VAs are independent contractors rather than employees. They also avoid the logistical nightmare of providing additional office space, equipment, or supplies to a third party. A virtual assistant (VA) is a person who does a specific task for a client. The client pays only for work, that is. VAs typically serve other small companies [ 31 , 32 ], but they can also assist busy executives.
VAs use the Internet, email, phone-call conferences and online workplaces, as well as fax machines, to communicate and exchange data with each other There is also a growing use of Skype and Zoom, Slack, or Google Voice by virtual assistants (VAs Because the professionals in this field operate on contract, it is believed that they will work together for a long Ten years of office experience is required for executive assistants, office managers/supervisors, secretaries, paralegal assistants, legal secretaries, real estate assistants, and information technology.
Voice Over Internet Protocol (VOIP) services like Skype, Microsoft Teams, Google Meet, and Zoom have made it possible to have a virtual assistant (VA) answer the phone without the end user’s knowledge in recent years, and VAs have made their way into many mainstream organizations. With today’s technology, many firms may personalize their receptionists without having to pay for an additional receptionist.
A VA is a person or company who works remotely as an independent professional, supplying a wide range of products to businesses and customers. When it comes to the typical secretarial tasks such as website editing, social media marketing, and customer care, and data input and accounting (MYOB and QuickBooks), virtual assistants excel. In the virtual world, the industry has changed tremendously as more people join.
VAs come from various professional backgrounds, but the majority have at least a few years of experience working in the “real” (non-virtual) corporate sector or working online or remotely. The modern world is a place for VAs, where the next generation is increasingly relying on intelligent technology to improve their personal and professional lives.
- 1. IDC. (2015). Explosive Internet of Things Spending to Reach$1.7 trillion in 2020 According to IDC. http://www.businesswire.com/news/home/20150602005329/en/
- 2. Klüwer, Tina. “From chatbots to dialog systems.” Conversational agents and natural language interaction: Techniques and Effective Practices. IGI Global, 2011. 1-22
- 3. Daniel B. Kline (January 30, 2017). “ Alexa, How Big Is Amazon’s Echo ”Alexa, How Big Is Amazon’s Echo ?”. The Motley Fool
- 4. Markowitz, Judith. “Toys That Have a Voice.” Speech Tech Mag
- 5. Moskvitch, Katia. “The machines that learned to listen.” BBC.com . Retrieved May 5, 2020
- 6. Epstein, J; Klinkenberg, W. D (1 May 2001). “From Eliza to Internet: a brief history of computerized assessment.” Computers in Human Behaviour. 17 (3): 295-314. ISSN 0747-5632
- 7. Weizenbaum, Joseph (1976). Computer power and human reason: from judgment to calculation. Oliver Wendell Holmes Library Phillips Academy. San Francisco: W. H. Freeman
- 8. “Smartphone: your new personal assistant – Orange Pop.” July 10, 2017. Archived from the original on July 10, 2017. Retrieved May 5, 2020
- 9. Darren Murph (October 4, 2011). “iPhone 4S hands-on!”. Retrieved December 10, 2017
- 10. “Feature: Von IBM Shoebox bis Siri: 50 Jahre Spracher kennung – WELT” [From IBM Shoebox to Siri: 50 years of speech recognition]. Die Welt (in German). Welt.de. April 20, 2012. Retrieved December 10, 2017
- 11. “Conversica Raises $31 Million in Series C Funding to Fuel Expansion of Conversational AI for Business”. Bloomberg.com . October 30, 2018. Retrieved October 23, 2020
- 12. Herrera, Sebastian. “Amazon Extends Alexa’s Reach into Wearables.” The Wall Street Journal. Retrieved September 26, 2019
- 13. “S7617 – Developing Your Wake Word Engine Just Like ‘Alexa’ and ‘OK Google”. GPU Technology Conference. Retrieved July 17, 2017
- 14. Van Loo, Rory (March 1, 2019). “Digital Market Perfection.” Michigan Law Review. 117 (5): 815
- 15. Taylor Martin; David Priest (September 10, 2017). “The complete list of Alexa commands so far.” CNET. Retrieved December 10, 2017
- 16. Kongthon, Alisa; Sangkeettrakarn, Chat chawal; Kong young, Sara woot; Haruechaiyasak, Choo chart (1 January 2009). Implementing an Online Help Desk System Based on Conversational Agent. Proceedings of the International Conference on Management of Emergent Digital EcoSystems. MEDES ‘09. New York, NY, USA: ACM. pp. 69:450-69:451
- 17. Anthony O’Donnell (June 3, 2010). “Aetna’s new “virtual online assistant.” Insurance & Technology. Archived from the original on June 7, 2010
- 18. Horton, John Joseph; Chilton, Lydia B. (2010). “The labor economics of paid crowdsourcing.” Proceedings of the 11th ACM Conference on Electronic Commerce – EC '10. New York, New York, USA: ACM Press: 209
- 19. Casilli, Antonio A. (2019). En attendant les robots. Enquête sur le travail du clic. Editions Seuil. ISBN 978-2-02-140188-2
- 20. Apple, Google, and Amazon May Have Violated Your Privacy by Reviewing Digital Assistant Commands”. Fortune. August 5, 2019. Retrieved May 13, 2020
- 21. Kröger, Jacob Leon; Lutz, Otto Hans-Martin; Reschke, Philip (2020). “Privacy Implications of Voice and Speech Analysis – Information Disclosure by Inference.” 576: 242-258
- 22. “5 Consumer Trends for 2017”. Trend Watching. October 31, 2016. Retrieved December 10, 2017
- 23. Felix Richter (August 26, 2016). “Chart: Digital Assistants – Always at Your Service.” Statista. Retrieved December 10, 2017
- 24. “Virtual Assistant Industry Statistics « Global Market Insights, Inc.” Gminsights.wordpress.com . January 30, 2017. Retrieved December 10, 2017
- 25. “Virtual digital assistants to overtake world population by 2021”. ovum.informa.com . Retrieved May 11, 2018
- 26. Jones, Nory B.; Graham, C. Matt (February 2018). “Can the IoT Help Small Businesses?”. Bulletin of Science, Technology & Society. 38 (1-2): 3-12
- 27. “Alexa and Siri Can Hear This Hidden Command. You Cannot”. The New York Times. May 10, 2018. ISSN 0362-4331. Retrieved May 11, 2018
- 28. “As voice assistants go mainstream, researchers warn of vulnerabilities.” CNET. May 10, 2018. Retrieved May 11, 2018
- 29. Chung, H.; Iorga, M.; Voas, J.; Lee, S. (2017). “Alexa, Can I Trust You?”. Computer. 50 (9): 100-104
- 30. Starks, Misty (July–August 2006). “Helping Entrepreneurs, virtually” (PDF). D-MARS. Archived from the original (PDF) on 2008-09-21. Retrieved 2008-07-27
- 31. Finkelstein, Brad (February–March 2005). “Virtual Assistants A Reality.” Broker Magazine. 7 (1): 44-46
- 32. Johnson, Tory (2007-07-23). “Work-From-Home Tips: Job Opportunities for Everyone.” ABC News. Retrieved 2008-07-28
© 2021 The Author(s). Licensee IntechOpen. This chapter is distributed under the terms of the Creative Commons Attribution 3.0 License , which permits unrestricted use, distribution, and reproduction in any medium, provided the original work is properly cited.
Continue reading from the same book
Published: 13 October 2021
By Adriana Stan and Beáta Lőrincz
673 downloads
By Abhishek Kaul
913 downloads
By Aik Beng Ng, Simon See, Zhangsheng Lai and Shaowei...
486 downloads
IntechOpen Author/Editor? To get your discount, log in .
Discounts available on purchase of multiple copies. View rates
Local taxes (VAT) are calculated in later steps, if applicable.
Support: [email protected]
A Systematic Review of Voice Assistant Usability: An ISO 9241–11 Approach
- Original Research
- Published: 03 May 2022
- Volume 3 , article number 267 , ( 2022 )
Cite this article
- Faruk Lawal Ibrahim Dutsinma ORCID: orcid.org/0000-0003-0108-5782 1 ,
- Debajyoti Pal ORCID: orcid.org/0000-0002-7305-1015 1 ,
- Suree Funilkul ORCID: orcid.org/0000-0003-0352-8647 2 &
- Jonathan H. Chan ORCID: orcid.org/0000-0001-6939-9943 1
9015 Accesses
27 Citations
Explore all metrics
Voice assistants (VA) are an emerging technology that have become an essential tool of the twenty-first century. The VA ease of access and use has resulted in high usability curiosity in voice assistants. Usability is an essential aspect of any emerging technology, with every technology having a standardized usability measure. Despite the high acceptance rate on the use of VA, to the best of our knowledge, not many studies were carried out on voice assistants’ usability. We reviewed studies that used voice assistants for various tasks in this context. Our study highlighted the usability measures currently used for voice assistants. Moreover, our study also highlighted the independent variables used and their context of use. We employed the ISO 9241-11 framework as the measuring tool in our study. We highlighted voice assistant’s usability measures currently used; both within the ISO 9241-11 framework, as well as outside of it to provide a comprehensive view. A range of diverse independent variables are identified that were used to measure usability. We also specified that the independent variables still not used to measure some usability experience. We currently concluded what was carried out on voice assistant usability measurement and what research gaps were present. We also examined if the ISO 9241-11 framework can be used as a standard measurement tool for voice assistants.
Similar content being viewed by others
Understanding Task Differences to Leverage the Usability and Adoption of Voice Assistants (VAs)
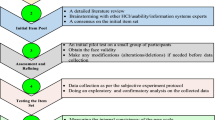
Usability Evaluation of Artificial Intelligence-Based Voice Assistants: The Case of Amazon Alexa
A Survey-Based Study to Identify User Annoyances of German Voice Assistant Users
Explore related subjects.
- Artificial Intelligence
Avoid common mistakes on your manuscript.
Introduction
Voice assistants (VAs) which are also called intelligent personal assistants are computer programs capable of understanding and responding to users using synthetic voices. Voice assistants have been integrated into different technological devices, including smartphones and smart speakers [ 1 ]. The voice modality is the central mode of communication used by these devices, rendering the graphic user interface (GUI) inapplicable or less meaningful [ 2 ]. People use VA technology in different aspects of their lives, such as for simple tasks like getting the weather report [ 3 ] or managing emails [ 4 ]. In addition, the VA can perform complex tasks like client representative tasks [ 5 ] and controllers in autonomous vehicles [ 6 ]. In other words, VA’s can revolutionize the way people interact with computing systems [ 7 ]. Currently, there is a massive global adoption of voice assistants. A report in [ 8 ] indicates that 4.2 billion VA’s were adopted and used in 2020 alone, with a projected increase to 8.4 billion by 2024. The popularity of VA’s has led to a greater research attention to its usability and user experience aspect.
Usability is a critical factor in the adoption of voice assistants [ 9 ]. A study by Zwakman et al. [ 10 ] highlighted the importance of usability in voice assistants [ 9 ]. An additional study by Coronado et al. [ 11 ] reiterated the importance of usability in human–computer interaction tools. Numerous studies have been carried out on the usability heuristics used in a VA, each study adopting a unique approach. A study by Maguire [ 12 ] used the Nielsen and Molich versions of Voice User Interface (VUI), and the heuristic Voice User Interface (VUI), to evaluate the ease of use of the VA’s. The study affirmed both the two heuristics were appropriate. However, the study noted that one was less problematic to use than the other [ 12 ]. A further study tested VUI heuristics to measure VA efficacy [ 13 ]. However, a critical factor that prevents the VA from adopting the heuristic currently available is the absence of a graphical user interface (GUI). Despite numerous studies on heuristics, the level of satisfaction is still low [ 14 ]. Furthermore, heuristics cannot be used as a standardized approach because they are approximate strategies or empirical rules for decision-making and problem-solving that do not ensure a correct solution. According to a study by Murad [ 16 ], the absence of standardized usability guidelines when developing VA interface presents a challenge in the development of an effective VA [ 15 ]. Another report from Budi & Leipheimer [ 17 ] also suggests that the usability of the VA’s requires improvements and standardization [ 16 ]. To create a standard tool a globally recognized and well-known organization is critical in the process because it eliminates bias and promotes neutrality [ 17 ]. The International Organization for Standardization (ISO) 9241-11 framework is one of the standard usability frameworks widely used for measuring technology acceptance.
According to the ISO 9241–11 framework, usability is defined as “the degree to which a program may be utilized to achieve measurable objectives with effectiveness, efficiency, and satisfaction in a specific context of usage” [ 18 ]. ISO 9241-11 provides a framework for understanding and applying the concept of usability in an interactive system and environment [ 19 ]. The main advantage of using the ISO standard is that industries and developers do not need to build different design measurement tools. This standard is intended to create compatibility with new and existing technologies, and also create trust [ 20 ]. Currently, the system developers do not have any standardized tool created specifically for the measurement of VA usability, consequently, the measures are decentralized, causing confusion among developers. The lack of in-depth assessment of the current heuristics used in the VA design affects the trust and adaptability of their users [ 15 ]. Other emerging technologies such as virtual reality [ 21 ] and game design [ 22 ] have understood the importance of creating an acceptable standardized measurement tool when designing new interfaces. Therefore, VA technology could also benefit significantly from the same concept. As evident from the above discussion, there is little to no focus on VA standardization.
Our study presents a systematic literature review comprising works carried out on the usability of voice assistants. In addition, we use the ISO 9241-11 framework as a standardized measurement tool to analyze the findings from the studies we collected. We chose the ACM and IEEE databases for the selection of our articles because both contain a variety of studies dealing with the usability aspects of VA’s. The following are the contributions of this literature review to the Human--Computer Interaction (HCI) community:
Our work highlights the studies currently carried out on VA usability. This includes the independent and dependent variables currently used.
Our study highlights the factors that affect the voice assistants' acceptance and impact the user’s total experience.
We identify and explain some attributes unique to only voice assistants, such as machine voice.
We also highlight the evaluation techniques used in previous studies to measure usability.
Finally, our study tries to compare the existing usability studies with the ISO 9241-11 framework. The decentralized approach of the VA usability measurement makes it vague to understand if the ISO 9241-11 framework is being adhered to whilst developing the usability metrics.
We hope that our input will highlight the integration of the current existing VA usability measures with the ISO 9241-11 framework. This will also verify whether the ISO 9241-11 framework can serve as a standard measure of usability in voice assistants. In conclusion, our study tries to answer the following four research questions:
RQ 1 : Can the ISO 9241–11 framework be used to measure the usability of the VA’s?
RQ 2 : What are the independent variables used when dealing with the usability of VA’s?
RQ 3 : What current measures serve as the dependent variables when evaluating the usability of VA’s?
RQ 4 : What is the relationship between the independent and dependent variables?
The remaining work is structured as follows. The second section presents the related work. This highlights what previous literature review studies had been carried out on voice agents’ usability; furthermore, the section also highlights the emergent technology that employed the ISO 9241-11 framework as a usability measuring tool. This is followed by the methodology section, which presents the inclusion and exclusion criteria used together with the review protocol. Furthermore, the query created for the database search is presented, and the database to be used is also selected. The fourth section presents the result and analysis. In this phase, the article used for this study is listed. Also, the research questions are answered. The fifth section contains discussion on the result analysis. This includes a more detailed explanation of the relationships between independent and dependent variables. Our insights and observations are included in this section as well.
Literature Review
Previous systematic reviews.
There have been a number of systematic literature reviews concerning VA’s over the years. Table 1 presents the information for a few of the relevant works.
As highlighted in Table 1 , multiple systematic literature reviews have been carried out on VA's usability over the years. However, each study has a specific limitation and gap for improvement. For instance, some studies focus on the usability of voice assistants used only in specified fields such as education [ 25 ] and health [ 36 ]. Other studies focus on the usability of voice assistants concerning only specific age groups, such as older adults [ 28 ]. Likewise, although an in-depth analysis of the usability of the VA’s is carried out involving every usability measure in [ 32 ], this study does not use the ISO 9241 framework as a measuring standard. On the other hand, another study in [ 33 ] although uses the ISO 9241 framework as a measuring standard, however, the usage context was chatbots focusing primarily on text-based communication instead of voice. Overall, the available literature reviews on VA’s usability listed in Table 1 supports the view that very few of the current literature review studies on VA’s use the ISO 9241-11 framework as an in-depth tool for measuring usability.
The ISO 9242-11 Usability Framework
The ISO 9241-11 is a usability framework used to understand usability in situations where interactive systems are used and employed, which includes framework environments, products, and services [ 39 ]. Nigel et al. [ 40 ] conducted a study to revise the ISO 9241-11 framework standard, which reiterates the importance of the framework within the concept of usability. A number of studies have been conducted on various technologies using the ISO 9241–11 framework as a tool to measure their usability. This shows the diversified approach when using the framework. For instance, a study by Karima et al. (2016) proposed the use of ISO 9241-11 framework to measure the usability of mobile applications running on multiple operating systems by developers, in which the study identified display resolution and memory capacity as factors that affect the usability of using mobile applications [ 41 ]. Another study used the ISO 9241-11 framework to identify usability factors when developing e-government systems [ 42 ]. This study focused on the general aspect of e-Government system development and concluded the framework could be used as a usability guideline when developing a government portal. In addition, the ISO 9241-11 framework was also used to evaluate other available methods and tools. For instance, a study by Maria et al. [ 44 ] used the framework to evaluate existing tools used in the measurement of usability of software products and artifacts on the web. The study compared existing tools with the ISO 9241-11 measures for efficiency, effectiveness and satisfaction [ 43 ]. ISO 9241–11 framework has also been employed as a method of standardization tool in the geographic field [ 44 ], game therapy in dementia [ 45 ], and logistics [ 46 ]. Despite the ISO 9241-11 usability framework being utilized in different aspects of old and emergent technologies, it has not been used with a VA in the past.
We performed a systematic literature review is this study using the guidelines established by Barbara [ 47 ]. These guidelines have been widely used in other systematic review studies as a result of their rigor and inclusiveness [ 48 ]. In addition, we have added a new quality assessment process to our guidelines. The quality assessment is a list of questions that we use to independently measure each study to ensure its relevance for our review. Our quality evaluation checklists are derived from existing studies [ 49 , 50 ]. The complete guidelines used in this section comprises of four different stages:
Inclusion and exclusion criteria
Search query
Database and article selection.
Quality assessment.
Inclusion and Exclusion Criteria
The inclusion and exclusion criteria used in our study are developed for completeness and avoidance of bias. The criteria we used for our study are:
Studies that focus on VA, with voice being the primary modality. In scenarios where the text or graphical user interfaces are involved, they should not be the primary focus.
Studies are only in the English language to avoid mistakes during translation from another language
The studies include at least one user and one voice assistant to ensure that the focus is on usability, not system performance.
Study has a comprehensive conclusion.
Released between 2000 and 2021, because during this period the vocal assistants started to gain notable popularity
The exclusion criteria are:
Studies with poor research design, where the study's purpose is not clear are excluded.
White papers, posters, and academic thesis are excluded.
Search Query
We created the search query for our study using keywords arranged to search the relevant databases. We went through previous studies to find the most relevant search keyword to find what is commonly used in usability studies. After numerous debates among the researchers and seeking two HCI expert's opinion, we chose the following set of keywords: usability, user experience, voice assistants, personal assistants, conversational agents, Google Assistant, Alexa, and Siri. We connected the keywords with logical operators (AND and OR ) to yield accurate results. The final search string used was (“usability” OR “user experience “) AND (“voice assistants” OR “personal assistants” OR “conversational Agents” OR “Google Assistant” OR” Alexa” OR “Siri”). The search was limited to the abstract and title of the study.
Database and Article Selection
Figure 1 highlights the graphic presentation of the selection and filtering process. The figure is adapted from the Prisma flow diagram [ 51 ]. As earlier stated, two databases are used as the sources for our article selection: the Association for Computing Machinery (ACM) and the Institute of Electrical and Electronics Engineers (IEEE). Both databases we used in our study contain the most advanced studies on VA and are highly recognized among the HCI community. The search query returned 340 results from the ACM database and 280 results from the IEEE database. 720 items in both databases were checked for duplication and 165 documents (23%) were found to be duplicated and hence removed. Additionally, more items were filtered by title and abstract. We utilized keyword match to search the title; however, the abstract was read to identify the eligibility criteria. In addition, 399 documents (72%) were removed because they did not meet the eligibility criteria. Finally, 121 documents were removed that were not consistent with the research objectives of our study. At the end of the screening process 29 articles (19%) were finally included in this literature review.
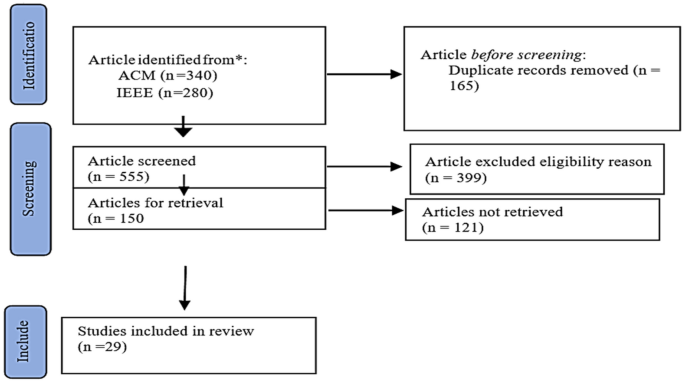
Article selection process
Quality Assessment
The selected items presented in Table 2 are used for assessing the quality of the selected articles. The process was deployed to ensure the reported contents fit into our research. The sections collected from articles such as the methodology used, analysis done, and the context of use within each article were vital to our study. Each question is a three-point scale: “Yes” is scored as 1 point, which means the question is fully answerable. “Partial” is scored as 0.5, which means the question is vaguely answered, and “NO “is scored as 0, which means it is not answered at all. All the 29 sets of finally included articles passed the quality assessment phase.
Result and Analysis
List of articles.
This section lists and discusses the articles collected in the previous stage. Table 3 presents the list of all the compiled articles. Moreover, we identified the usability focus of each study.
Voice Assistant Usability Timeline
We grouped the collected research into three categories, each representing a range of time frames (Fig. 2 ). The categorization is based on voice assistant period breakthroughs. The first category is from 2000 to 2006, which was the year of social media and camera phones, also known as the year of the Y2K bug in telecommunications. During these years, conversational agents started to get noticed with the introduction of the inventions such as the Honda’s Advanced Step in Innovative Mobility (ASIMO) humanoid robot [ 80 ]. The second category ranges from 2007 to 2014. During these years technological advancements got users more exposed to voice assistants through embedding them into smartphones and computers. For instance, Apple first introduced SIRI in 2011 [ 81 ], and Microsoft introduced Cortana in 2014. The last category ranges from 2015 to 2021. This was when the massive adoption of voice assistants took place, making it an all-time high.
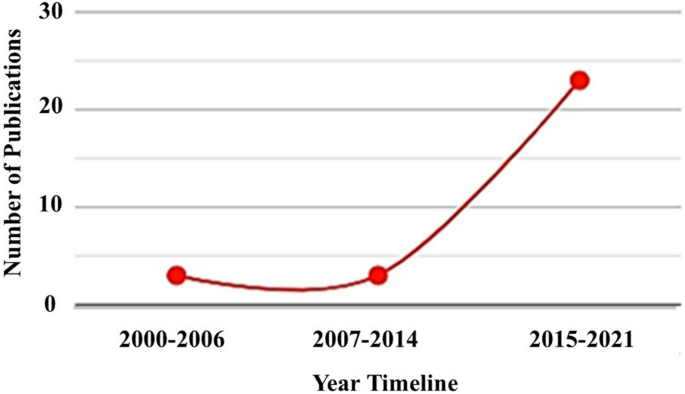
Year of publication of selected articles
Based on the year of publication of our selected articles, Fig. 2 clearly shows that the study on VA’s has expanded significantly over the last six years (2014–2021). This can be attributed to the invention of a smart speaker and phone with built-in voice agents [ 82 ]. Another reason for VA popularity is the COVID -19 outbreak that has given a fresh impetus towards touchless interaction technologies like voice [ 83 ].
Different Embodiment Types of VA’s
Smart speakers are the mostly used embodiment of VA’s used in our selected articles. This is due to the current popularity of commercial smart speakers such as Alexa, HomePod, etc. A 2019 study showed that 35% of US households have an intelligent smart speaker, and projected to reach 75% by 2025 [ 84 ]. Use of humanoids is also popular because usability measures such as anthropomorphism are essential for voice assistant usability [ 85 ]. Furthermore, Fig. 3 shows that only a few studies were done on car interface voice assistants. Car interfaces are vocal assistants that act as intermediaries between the driver and the car. The VA car interface allows drivers to access car information and also be able to perform the task without losing focus on driving. The fourth type of software interface refers to a voice assistant software embedded inside smartphones or computers. The studies we have collected have used either the commercialized form of the software interface, such as Alexa and Siri, while others have developed new voice interfaces that are easily accessible to users due to the adoption of smartphones and computers assistants using programming codes and skills. Nevertheless, both are in the forms of different software agents.
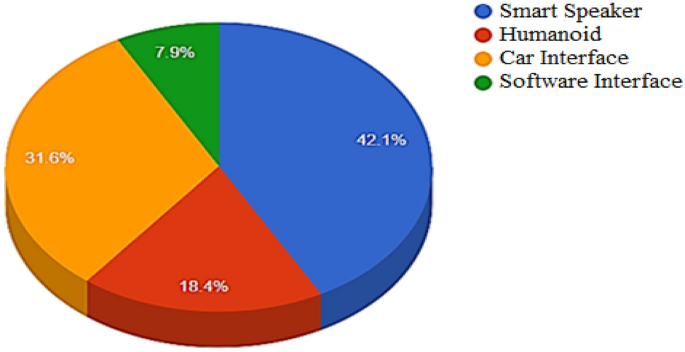
Embodiment of Voice assistant used in selected studies
Component of ISO 9241-11 Framework
The ISO 9241-11 framework highlights two components, the context of use and usability measure [ 18 ]. We concentrate on both components to highlight any correlations between usability metrics and the context of use in the selected articles. The context of use consists of the different independent variables along with the techniques used for analyzing them. Likewise, the usability measure represents the dependent variables, i.e., the effect that the independent variables have on the overall experience of the users. Accordingly, the analysis is presented in a bi-dimensional manner in the following sections.
Context of Use
Independent variable.
We split the context of use into an independent variable and the techniques used. The independent variables presented in our study are the physical and mental attributes used to measure a given user interaction outcome. Furthermore, our study grouped the independent variables into five main categories. The grouping is shown in Fig. 4 and is based on the similar themes identified from the collected studies. The five groups included people (user attributes), voice (voice assistant attributes), task, conversational style, and anthropomorphic cues. The voice and people categories are the oldest independent variables used to measure usability. Their relevance is also seen in the recent studies, which indicate that researchers have a high interest in correlating users with the VA’s. On the other hand, anthropomorphic clues and conversational styles are relatively new to the measurement of usability. The task-independent variable is the most used variable of late, perhaps because users always test the VA’s ability to perform certain tasks. It also indicates that VA’s are widely used for various functional and utilitarian aspects. The anthropomorphic cues are seldom used in the second phase (2007–2014). However, it is most widely used in the last range (2015–2021).
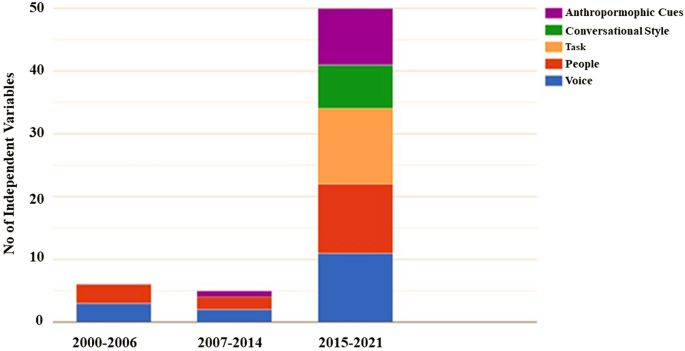
Categories of independent variable use over the years
In Table 4 we highlight more details with regards to the different groups of the independent variable collected, and also present examples of the independent variables for each category. We highlight how the independent variables have been applied by the previous studies and in which environment they have been used. We defined each independent variable category in Table 4 , and explained their sub-categories as well. As evident from Table 4 , different independent variables are used together in multiple studies. For example, independent voice variables and independent people variables are used simultaneously in various studies, such as personality, gender, and accent. Similarities between multiple independent variables aid to understand the relationship between the variables themselves and their relationship with the usability measures. Furthermore, the table also highlights the kind of experiments carried out. Controlled experiments are effective methods for understanding the immediate cause and effect between variables. However, a noticeable drawback of controlled experiments is the absence of external validity. The results might not be the same when applied in real-world settings. For instance, the simulation experiment on cars is a controlled environment, a driver has no control over the domain in real life. The usability experience of the driver might be different in natural settings and that might sometimes prove fatal.
Techniques Used
We identified seven techniques that researchers have used as shown in Fig. 5 . The quantitative experiments are the most used and the oldest technique used on voice assistants based on our data collected. The quantitative method is sometimes used as a standalone experiment and sometimes with other techniques [ 54 ]. It is worthy of notice that cars simulation experiments involving VA’s were first used in 2000. Other experiments on human communication with self-driving cars have been carried out since 1990’s. making it one of the oldest techniques for usability measurement. More accurate technique was introduced later, such as the interaction design. The interaction design employed by studies such as [ 61 ] provides a real-time experiment scenario. This avoids the drawback such as bias when using quantitative methods. Factorial design studies are majorly used by studies that compare two or more entities in a case study [ 55 ]. They are utilized mainly by studies using two or more independent variables together.
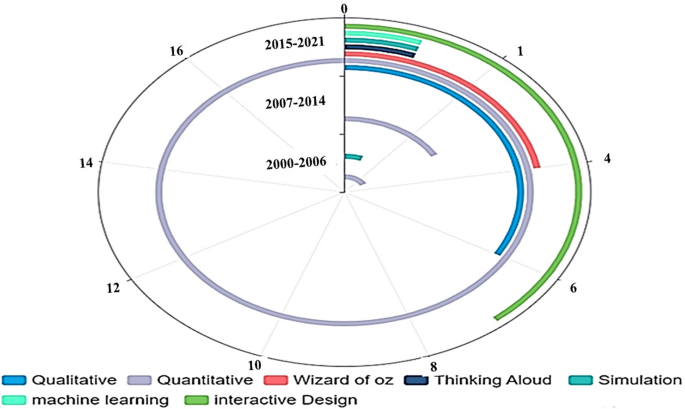
Technique used in our studies over the span period of time
Usability Measure (Dependent Variable)
This subsection of our study focuses on the usability measurement of our research. Moreover, the findings are used to answer RQ 1 and RQ 3 . The ISO 9241-11 framework grouped usability measures into three categories; effectiveness, efficiency, and satisfaction. According to the ISO 9241-11 framework, “effectiveness is the accuracy and completeness with which users achieve specified goals.”, Whereas “Efficiency is the resources expended concerning accuracy and completeness in which users achieve goals” and “satisfaction is the freedom from discomfort and positive attitudes towards the use of the product” [ 18 ].
In numerous studies, the usability measures used were clearly outside the scope of the ISO 9241-11 framework. In total, we identified three additional usability categories attitude, machine voice (anthropomorphism), and cognitive load. The graphical representation of the different usability measures identified in this study is presented in Figs. 6 and 7 . Futhermore, the figures also highlights the percentage of studies that used the mentioned usability measures in the ISO 9241-11 framework and those that are outside the framework. Based on our compiled result, the user satisfaction and effectiveness are the earliest usability measures used when measuring VA’s usability. Some studies used performance and productivity as subthemes to measure effectiveness [ 62 ]. The measure of usability has been carried out both subjectively and objectively. For instance, studies have measured the VA effectiveness by subjective means by using quantitative methods such as questionnaire tools [ 72 ]. In contrast, other studies have used objective methods such as average completed interaction [ 69 ]. Multiple usability measures are sometimes applied in the same research; for instance some studies measured effectiveness alongside efficiency and satisfaction [ 66 , 70 ]. Learnability, optimization, and ease of use have been used as subthemes to measure efficiency. Interactive design is the most effective experiment that provides real-time results employed [ 56 , 79 ]. The ISO 9241-11 framework works well with effectiveness, efficiency, and satisfaction; however, the users have more expectations from the voice assistant with the recent advancement of VA capabilities. Our compiled result showed that more than half of the studies are not carried out in accordance with the standard ISO 9241-11 framework (Fig. 7 ). The other usability measures we identified outside the ISO 9241-11 framework are attitude, machine voice, and cognitive load.
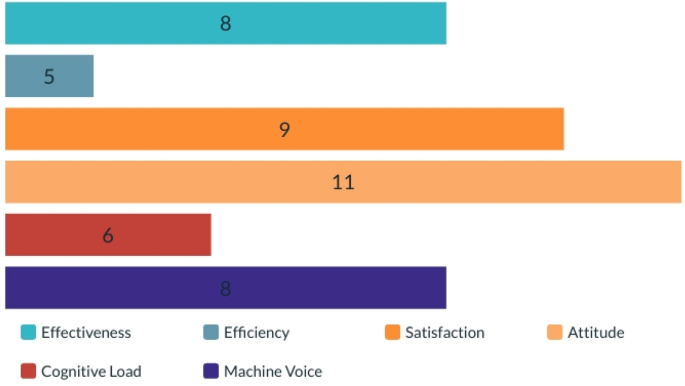
Usability measurement used over the years on our compiled articles
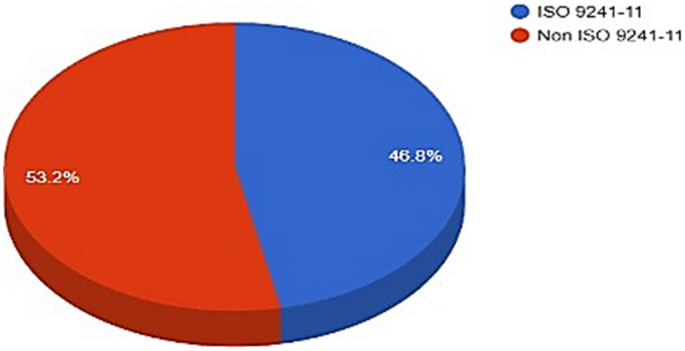
Percentage of ISO 9241–11 framework usability measures and non ISO 9241–11
Attitude is a set of emotions, beliefs, and behavior towards the voice assistants. Attitude results from a person’s experience and can influence user behavior. Attitude is subjected to change and is not constant. Understanding the user attitude towards the VA has become an active research area. Numerous studies have used different methods to measure subthemes of attitude such as trust, closeness, disclosure, smartness, and honesty [ 60 , 78 ]. Likeability is also a subtheme of attitude, and it has been used to measure the compatibility, trust, and strength between the user and VA’s [ 56 , 57 ]. Moreover, embodiment type affects the user attitude as well, A study highlighted how gaze affects the user attitude toward VA [ 59 ], and it shows VA with gaze creates trust.
We defined machine voice (anthropomorphism) as the user attribution of human characteristics and human similarity to the voice assistant. We considered machine voice an important usability measure that only applies to voice assistants due to their primary modality being the voice. Considering that fact, the measure of machine voice has also spiked currently it becomes obvious that it has been drawing a lot of interest. One of the direct purposes of the VA is to sound as humanly as possible. When the users will perceived the machines to be more human, it built more trust, which will result in a better usability experience.
The cognitive load might be mistaken for efficiency. Nevertheless, they are different. We defined cognitive load as the amount of mental capacity a person applies to communicate successfully with the VA. When it comes to VA, actions such as giving out commands require cognitive thinking and approach. The cognitive load is measured by specific characteristics unique to the VA, such as attention time during the use of the VA [ 76 ] and the user’s mental workload during use [ 77 ].
To answer RQ 1 ( can the ISO 9241–11 framework be used to measure the usability of the VA’s?), none of the existing works have used the ISO 9241-11 framework solely for the purpose of usability evaluation. It has been supplemented by other factors that we have presented above that are outside the scope of this framework.
Relationship Between the Independent variables and Usability Measures
After identifying the independent and dependent variables, in Tables 5 and 6 we show how they are inter-related for having a better understanding of the usability scenario of the VA’s. While Table 5 focuses on the ISO 9241-11 specific factors, Table 6 considers the non-ISO factors specifically.
The independent variables are grouped into categories and represented by table rows, with every category consisting of multiple independent variables. Moreover, the usability measures have been presented in the column of the table. Every usability measure is made up of different sub-themes, which are all presented on the table as well. The tables highlight the relationship between the independent and usability measures. An “X” mark present in each cell represents a study present between that independent variable and usability measure subtheme. Nonetheless, an empty cell indicates that there is no study carried out to link that relationship between the usability measure and independent variable.

Independent Variable and Usability Measures
Our study revealed what has been previously carried out in VA usability and revealed the gaps that are yet to be addressed. We analyzed the usability measures and their relationship to the so-called independent variables. There is an easy accessibility to VA’s due to the development of different embodiment types such as speakers, humanoids, and robots. However, there is so much less focus on embodiment types and their relationship to effectiveness and anthropomorphism, which needs more attention. Some relationship gaps and associations are apparent, while some are vague. For instance, the independent variable “ accent ”, has often been connected with its effectiveness on users. However, what is left unanswered is if the VA accents impart the same efficacy on users of the same or different genders. Another notable gap is gender and efficiency, with very few studies on that. This will be an essential aspect to understand and apply with the recent massive adoption of voice assistants in different contexts. Another obvious gap is the query expression relationship with any ISO 9241-11 framework measures. The query expression is how a user expresses their query to the voice assistants. The query expression has been known to increase the trust and attitude of the user towards the VA. However, its relationship to usability measures such as efficiency, satisfaction, and effectiveness is still under-researched. Knowing the right way to ask queries (questions) defines the type of response a user gets. An incorrect response will be received if the right question is expressed incorrectly. From a mental model, when a user has too much energy and thought to frame a question, it affects the VA efficiency and satisfaction. However, this has not been proven by any current study.
The VA response types increase effectiveness and trust. However, its relationship to user acceptance is still unknown. Another exciting intersection is the anthropomorphic cues and attitude, which results from anthropomorphic emotional response than a practical one. Attitude is an emotional response to a giving state, hence its strong connection with anthropomorphism. The attitude toward the VA is a highly researched area [ 86 ]. Trust, likeability, and acceptance are subthemes that focused on the attitude usability measure. This can be attributed to the importance of trust while using emergent technologies such as voice assistants. User trust in voice assistants is an essential aspect with the rise of IoT devices, and user mistrust affects the acceptance and effectiveness of the VA’s [ 87 ]. Multiple studies measured user trust while using machine voice categories as an independent variable. That could be attributed to the lack of GUI in VA. Furthermore, the voice modality must be enough to cultivate user trust. Noticeably subjective methods were widely employed when measuring the user attitudes; even though subjective measures often relate to the variables they are intended to capture; however, they are also affected by cognitive biases.
The ISO 9241-11 framework is an effective tool when measuring effectiveness, efficiency, and satisfaction. However, it is not applicable when measuring usability’s, such as attitude, machine voice, and mental load. These are all measurements that are uniquely associated with voice assistants. Therefore, the ISO 9241-11 framework could be expanded to include such usability aspects.
Technique Employed
The factorial design adapts well when used in a matched subject design experiments [ 56 ]. Based on the studies collected, machine learning is not well used as an analytic tool in usability. This could be attributed to the technical aspects of machine learning and it is still relatively a new field. However, with machine learning third-party tools more analysis will be carried out. Wizard of Oz, and interactive design started gaining popularity in 2015–2021. Moreover, the Wizard of Oz and interactive techniques are more effective when using independent variables such as anthropomorphic cues. The anthropomorphic cue independent variables is used with Wizard of Oz. techniques and interaction design more than any other techniques. This could be recognized to the importance of using objective methods to avoid biased human responses. Furthermore, “machine voice” is a fairly popular usability measure. This could be attributed to the VA developers trying to give the VA a more human and intelligent attributes. The more users perceive the machine voice as intelligent and humanlike, the more they trust and adopt it. More objective technique methods should be created and used on the independent variables when measuring machine voice. Subjective techniques such as Quantitative methods are easy to use and straightforward. However, they can produce biased results.
Interactive design experiments are the most commonly used technique employed to measure the usability. However, the interaction depends on voice modality, making it different from the traditional interaction design that uses visual cues as part of its essential components. Moreover, interaction design also triggers an emotional response, which makes it effective when measuring user attitude. The absence of visual elements in interactive design used might debatably defeat the purpose of clear communication. A new standard of interaction design uniquely for voice modality should be done.
Future Works and Limitation
One limitation in our study was using a few databases as our articles source; in future studies, we intend to add more journal databases such as Scopus, and Taylor and Francis. The majority of the experiment studies we collected was conducted in a controlled environment; future studies will focus on usability measures and independent variables, that are used in natural settings; furthermore, the results can be compared together More studies should be carried out on objective techniques, also how they could cooperate with subjective techniques. This is vital because, with the rise of user expectations of voice assistants, it will be essential to understand how techniques complement each other in each usability measurement.
Our study aimed to understand what is currently employed for measuring voice assistant usability, and we identified the different independent variables, dependent variables, and the techniques used. Furthermore, we also focused on using the ISO 9241-11 framework to measure the usability of voices assistants. Our study classified five independent variable classes used for measuring the dependent variables. These separate classes were categorized based on the similarities between the member groups. Also, our study used the three usability measures in the ISO 9241-11 framework in conjunction with the other three to serve as the dependable variables. We uncovered that voice assistants such as car interface speakers were not studied enough, and currently, smart speakers have the most focus. Dependent variables such as machine voice (anthropomorphism) and attitude recently have more concentration than the old usability measures, such as effectiveness. We also uncovered that usability is dependent on the context of use, such as the same independent variables could be used in different usability measures. Our study highlights the relationship between the independent and dependent variables used by other studies. In conclusion, our study used the ISO 9241-11 to analyse usability. We also highlight what has been carried out on VA’s usability and what gaps are left. Moreover, we concluded even though there is a lot of usability measurement carried out, there are still many aspects that have not been researched. Furthermore, the current ISO 9241-11 framework is not suitable for measuring the recent advancement of VA because the user needs and expectation have changed with the rise of technology. Using the ISO 9241-11 framework will create ambiguity in explaining some usability measures such as machine voice, attitude and cognitive load. However, it has the potential to be a foundation for future VA usability frameworks.
Hoy MB. Alexa, Siri, Cortana, and more: an introduction to v oice assistants. Med Ref Serv Quart. 2018;37(1):81–8.
Article Google Scholar
Zwakman DS, Pal D, Arpnikanondt C. Usability evaluation of artificial intelligence-based voice assistants: the case of amazon Alexa. SN Comput Sci. 2021. https://doi.org/10.1007/s42979-020-00424-4 .
Segi H, Takou R, Seiyama N, Takagi T, Uematsu Y, Saito H, Ozawa S. An automatic broadcast system for a weather report radio program. IEEE Trans Broadcast. 2013;59(3):548–55.
Noel S. Human computer interaction (HCI) based Smart Voice Email (Vmail) Application—Assistant f or Visually Impaired Users (VIU). In: 2020 third international conference on smart systems and inventive technology (ICSSIT) (pp 895–900). IEEE; 2020.
Sangle-Ferriere M, Voyer BG. Friend or foe? Chat as a double-edged sword to assist customers. J Serv Theory Pract. 2019;29:438–61.
Lugano G. Virtual assistants and self-driving cars. In: 2017 15th International Conf erence on ITS Telecommunications (ITST) (pp 1–5). IEEE; 2017.
Rybinski K, Kopciuszewska E. Will artif icial intelligence rev olutionise the student evaluation of teaching? A big data study of 1.6 million student reviews. Assessment & Evaluation in Higher Education; 2020, pp. 1–13
Tankovska H. Number of digital voice assistants in use worldwide 2019–2024 (in billions), 2020. https://www.statista.com/statistics/973815/worldwide-digital-voice-assistant-in-use/ , (accessed 17 Nov 2021)
Pal D, Arpnikanondt C, Funilkul S, Chutimaskul W. The adoption analysis of voice-based smart IoT products. IEEE Internet Things J. 2020;7(11):10852–67.
Zwakman DS et al. Voice usability scale: measuring the user experience with voice assistants. In: 2020 IEEE International Symposium on Smart Electronic Sy stems (iSES)(Formerly iNiS). IEEE; 2020.
Coronado E, Deuff D, Carreno-Medrano P, Tian L, Kulić D, Sumartojo S, et al. Towards a modul ar and distributed end-user dev elopment framework f or human-robot interaction. IEEE Access. 2021;9:12675–92.
Maguire M. Development of a heuristic evaluation tool f or voice user interf aces. In: International conference on human-computer interaction. Cham: Springer; 2019. p. 212–25.
Google Scholar
Fulfagar L, Gupta A, Mathur A, Shrivastava A. Development and evaluation of usability heuristics for voice user interfaces. In: International conference on research into design. Singapore: Springer; 2021. p. 375–85.
Nowacki C, Gordeeva A, Lizé AH. Improving the usability of voice user interfaces: a new set of ergonomic criteria. In: International conference on human-computer interaction. Cham: Springer; 2020. p. 117–33.
Pal D, Zhang X, Siyal S. Prohibitive factors to the acceptance of Internet of Things (IoT) technology in society: a smart-home context using a resistive modelling approach. Technol Soc. 2021;66: 101683.
Murad C, Munteanu C. “I don't know what you're talking about, HALexa" the case f or voice user interface guidelines. In: Proceedings of the 1st International Conference on Conversational User Interfaces,2019; pp. 1–3.
Budiu R, Laubheimer P. Intelligent assistants have poor usability: a user study of Alexa, Google assistant, and Siri. Nielsen Norman Group; 2018. Available online at https://www.nngroup.com/articles/intelligentassistant-usability/ (last accessed 4/12/2019).
Murphy CN, Yates J. The international organization f or standardization (ISO): global governance through voluntary consensus. Routledge; 2009.
Book Google Scholar
ISO 9241-11. Ergonomic requirements for office work with visual display terminals (VDTs)—Part II guidance on usability; 1998.
Weichbroth P. Usability attributes revisited: a time-framed knowledge map. In 2018 Federated Conference on Computer Science and Information Systems (FedCSIS) (pp 1005–1008). IEEE; 2018.
Petrock V. Voice assistant and smart speaker users 2020. Insider Intelligence; 2020. Retrieved November 22, 2021, from https://www.emarketer.com/content/voice-assistant-and-smart-speaker-users-2020
Pinelle D, Wong N and Stach T. Heuristic evaluation f or games: usability principles for video game design. In: Proceedings of SIGCHI Conference on Human Factors in Computing Sy stems (2008); 2008, pp. 1453–1462. https://doi.org/10.1145/1357054.1357282 .
Sutcliffe A, Gault B. Heuristic evaluation of virtual reality applications. Interact Comput 16. 2004;4:831–49. https://doi.org/10.1016/j.intcom.2004.05.00 .
Sharif K, Tenbergen B. Smart home voice assistants: a literature survey of user privacy and security vulnerabilities. Complex Syst Inform Model Quart. 2020;24:15–30.
de Barcelos Silva A, Gomes MM, da Costa CA, da Rosa Righi R, Barbosa JLV, Pessin G, et al. Intelligent personal assistants: a systematic literature review. Expert Syst Appl. 2020;147: 113193.
Gubareva R and Lopes RP. Virtual assistants for learning: a systematic literature review. In: CSEDU (1); 2020, pp. 97–103.
Bérubé C, Schachner T, Keller R, Fleisch E, Wangenheim F, Barata F, Kowatsch T. Voice-based conversational agents for the prevention and management of chronic and mental health conditions: systematic literature review. J Med Internet Res. 2021;23(3): e25933.
Chi OH, Gursoy D and Chi CG. Tourists’ attitudes toward the use of artificially intelligent (AI) devices in tourism service delivery: moderating role of service value seeking. J Travel Res. 2020; 0047287520971054.
Kim S. Exploring how older adults use a smart speaker-based voice assistant in their first interactions: qualitative study. JMIR Mhealth Uhealth. 2021;9(1): e20427.
Coursaris CK, Kim DJ. A meta-analytical review of empirical mobile usability studies. J Usability Stud. 2011;6(3):117–71.
Goh ASY, Wong LL, Yap KYL. Evaluation of COVID-19 information provided by digital voice assistants. Int J Digital Health. 2021;1(1):3.
Rapp A, Curti L, Boldi A. The human side of human-chatbot interaction: a systematic literature review of ten years of research on text-based chatbots. Int J Hum-Comput Stud. 2021;151: 102630.
Seaborn K, Miyake NP, Pennefather P, Otake-Matsuura M. Voice in human-agent interaction: a survey. ACM Comput Surv (CSUR). 2021;54(4):1–43.
Castro JW, Ren R, Acuña ST and Lara JD. Usability of chatbots: a systematic mapping study; 2019.
Bhirud N, Tataale S, Randive S, Nahar S. A literature review on chatbots in healthcare domain. Int J Sci Technol Res. 2019;8(7):225–31.
Ahmad NA, Che MH, Zainal A, Abd Rauf MF, Adnan Z. Review of chatbots design techniques. Int J Comput Appl. 2018;181(8):7–10.
Gentner T, Neitzel T, Schulze J and Buettner R. A Systematic literature review of medical chatbot research from a behavior change perspective. In: 2020 IEEE 44th annual computers, software, and applications conference (COMPSAC). IEEE; 2020, pp. 735–740.
Cunningham-Nelson S, Boles W, Trouton L and Margerison E. A review of chatbots in education: practical steps forward. In: 30th Annual Conference for the Australasian Association for Engineering Education (AAEE 2019): Educators Becoming Agents of Change: Innovate, Integrate, Motivate. Engineers Australia; 2019, pp. 299–306.
Van Pinxteren MM, Pluymaekers M, Lemmink JG. Human-like communication in conversational agents: a literature review and research agenda. J Serv Manag. 2020;31:203–25.
Weichbroth P. Usability attributes revisited: a time-framed knowledge map. In: 2018 Federated Conference on Computer Science and Information Systems (FedCSIS). IEEE; 2018, pp. 1005–1008.
Bevan N, Carter J, Earthy J, Geis T, Harker S. New ISO standards for usability, usability reports and usability measures. In: International conference on human-computer interaction. Cham: Springer; 2016. p. 268–78.
Moumane K, Idri A, Abran A. Usability evaluation of mobile applications using ISO 9241 and ISO 25062 standards. Springerplus. 2016;5(1):1–15.
Yahya H, Razali R. A usability-based framework for electronic government systems development. ARPN J Eng Appl Sci. 2015;10(20):9414–23.
Alva ME, Ch THS, López B. Comparison of methods and existing tools for the measurement of usability in the web. In: International conference on web engineering. Berlin: Springer; 2003. p. 386–9.
MATH Google Scholar
He X, Persson H, Östman A. Geoportal usability evaluation. Int J Spatial Data Infrastruct Res. 2012;7:88–106.
Dietlein CS, Bock OL. Development of a usability scale based on the three ISO 9241–11 categories “effectiveness, ”efficacy” and “satisfaction”: a technical note. Accred Qual Assur. 2019;24(3):181–9.
Nik Ahmad NA and Hasni NS. ISO 9241–11 and SUS measurement for usability assessment of dropshipping sales management application. In: 2021 10th International Conference on Software and Computer Applications. 2021; pp. 70–74.
Kitchenham B. Procedures f or perf orming systematic reviews. Keele Univ. 2004;33(2004):1–26.
Seaborn K, Miyake NP, Pennefather P, Otake-Matsuura M. Voice in human–agent interaction: a survey. ACM Comput Surv (CSUR). 2021;54(4):1–43.
Al-Qaysi N, Mohamad-Nordin N, Al-Emran M. Employing the technology acceptance model in social media: a systematic review. Educ Inf Technol. 2020;25(6):4961–5002.
Kitchenham B and Charters S. Guidelines f or performing systematic literature reviews in software engineering; 2007.
Page MJ, McKenzie JE, Bossuyt PM, Boutron I, Hoffmann TC, Mulrow CD CD, The PRISMA, et al. statement: an updated guideline f or reporting sy stematic rev iews. BMJ. 2020;2021(372): n71. https://doi.org/10.1136/bmj.n71 .
Martelaro N, Teevan J and Iqbal ST. An exploration of speech-based productivity support in the car. In: Proceedings of the 2019 CHI conference on human factors in computing systems. 2019; pp. 1–12
Jeong Y, Lee J and Kang Y. Exploring effects of conversational fillers on user perception of conversational agents. In: Extended Abstracts of the 2019 CHI Conference on Human Factors in Computing Systems (CHI EA ’19). 2019; 1–6. https://doi.org/10.1145/3290607.3312913 .
Yu Q, Nguyen T, Prakkamakul S and Salehi N. “I almost fell in love with a machine”: speaking with computers affects self-disclosure. In: Extended Abstracts of the 2019 CHI Conference on Human Factors in Computing Systems (CHI EA ’19). 2019; pp. 1–6. https://doi.org/10.1145/3290607.3312918
Kiesel J, Bahrami A, Stein B, Anand A, and Hagen M. Clarifying false memories in voice-based search. In: Proceedings of the 2019 Conference on Human Information Interaction and Retrieval (CHIIR ’19). 2019; 331–335. https://doi.org/10.1145/3295750.3298961 .
Kontogiorgos D, Pereira A, Andersson O, Koivisto M, Rabal EG, Vartiainen V and Gustafson J. The effects of anthropomorphism and non-verbal social behavior in virtual assistants. In: Proceedings of the 19th ACM International Conference on Intelligent Virtual Agents (IVA ’19). 2019; 133–140. https://doi.org/10.1145/3308532.3329466
Hoegen R, Aneja D, McDuff D and Czerwinski M. An end-to-end conversational style matching agent. In: Proceedings of the 19th ACM International Conference on Intelligent Virtual Agents (IVA ’19). 2019; 111–118. https://doi.org/10.1145/3308532.3329473
Luo Y, Lee B and Choe EK. TandemTrack: shaping consistent exercise experience by complementing a mobile app with a smart speaker. In: Proceedings of the 2020 CHI Conference on Human Factors in Computing Systems (CHI ’20). 2020; 1–13. https://doi.org/10.1145/3313831.3376616
Doyle PR, Edwards J, Dumbleton O, Clark L and Cowan BR. Mapping perceptions of humanness in intelligent personal assistant interaction. In: Proceedings of the 21st International Conference on Human-Computer Interaction with Mobile Devices and Services (MobileHCI ’19). 2019. https://doi.org/10.1145/3338286.3340116 .
Jaber R, McMillan D, Belenguer JS and Brown B. Patterns of gaze in speech agent interaction. In: Proceedings of the 1st International Conference on Conversational User Interfaces - CUI ’19 (the 1st International Conference). 2019; 1–10. https://doi.org/10.1145/3342775.3342791 .
Bortoli M, Furini M, Mirri S, Montangero M and Prandi C. Conversational interfaces for a smart campus: a case study. In: Proceedings of the international conference on advanced visual interfaces (AVI ’20). 2020. https://doi.org/10.1145/3399715.3399914 .
Wu Y, Edwards Y, Cooney O, Bleakley A, Doyle PR, Clark L, Rough D and Cowan BR. Mental workload and language production in non-native speaker IPA interaction. In: Proceedings of the 2nd Conference on Conversational User Interfaces (CUI ’20). 2020. https://doi.org/10.1145/3405755.3406118
Brüggemeier B, Breiter M, Kurz M and Schiwy J. User experience of Alexa when controlling music: comparison of face and construct validity of four questionnaires. In: Proceedings of the 2nd conference on conversational user interfaces (CUI ’20). 2020. https://doi.org/10.1145/3405755.3406122
Machine body language: expressing a smart speaker’s activity with intelligible physical motion. 57
Bartneck C, Kulić D, Croft E, Zoghbi S. Measurement instruments for the anthropomorphism, animacy, likeability, perceived intelligence, and perceived safety of robots. Int J Soc Robot. 2009;1(1):71–81. https://doi.org/10.1007/s12369-008-0001-3A .
Braun M, Mainz A, Chadowitz R, Pfleging B and Alt F. At your service: designing voice assistant personalities to improve automotive user interfaces. In: Proceedings of the 2019 CHI Conference on Human Factors in Computing Systems (CHI ’19), 2019;40:1–40:11. https://doi.org/10.1145/3290605.3300270
Burbach L, Halbach P, Plettenberg N, Nakayama J, Ziefle M and Valdez AC. “Hey, Siri”, “Ok, Google”, “Alexa”. Acceptance-relevant factors of virtual voice-assistants. In 2019: IEEE International Professional Communication Conference (ProComm) (ProComm ’19), 2019;101–111. https://doi.org/10.1109/ProComm.2019.00025 .
Pal D, Arpnikanondt C, Funilkul S, and Varadarajan V. User experience with smart voice assistants: The accent perspective. In 2019 10th International Conference on Computing, Communication and Networking Technologies (ICCCNT ’19), 2019;1–6. https://doi.org/10.1109/ICCCNT45670.2019.8944754 .
Chin H, Molefi L, and Yi Y. Empathy is all you need: How a conversational agent should respond to verbal abuse. In: Proceedings of the 2020 CHI Conference on Human Factors in Computing Systems (CHI ’20), 2020; 1–13. https://doi.org/10.1145/3313831.3376461 .
Crowell CR, Villanoy M, Scheutzz M and Schermerhornz P. Gendered voice and robot entities: Perceptions and reactions of male and female subjects. In: Proceedings of the 2009 IEEE/RSJ International Conference on Intelligent Robots and Systems (IROS 2009), 2009; 3735–3741. https://doi.org/10.1109/IROS.2009.5354204
Lee S, Cho M and Lee S. What if conversational agents became invisible? Comparing users’ mental models according to physical entity of AI speaker. In: Proc. ACM Interact. Mob. Wearable Ubiquitous Technol. 2020; 4, 3. https://doi.org/10.1145/3411840
Dahlbäck N, Wang QY, Nass C and Alwin J. Similarity is more important than expertise: Accent effects in speech interfaces. In Proceedings of the SIGCHI Conference on Human Factors in Computing Systems (CHI ’07), 2007; 1553–1556. https://doi.org/10.1145/1240624.1240859
Lee EJ, Nass C, and Brave S. Can computer-generated speech have gender?: An experimental test of gender stereotype. In Proceedings of the CHI’00 Extended Abstracts on Human factors in Computing Systems (CHI EA ’00), 2000; 289–290. https://doi.org/10.1145/633292.633461
Nass C, Jonsson I-M, Harris H, Reaves B, Endo J, Brave S and Takayama L. Improving automotive safety by pairing driver emotion and car voice emotion. In: CHI ’05 Extended Abstracts on Human Factors in Computing Systems (CHI EA ’05), 2005;1973–6. https://doi.org/10.1145/1056808.1057070 .
Shi Y, Yan X, Ma X, Lou Y and Cao N. Designing emotional expressions of conversational states for voice assistants: Modality and engagement. In: Extended Abstracts of the 2018 CHI Conference on Human Factors in Computing Systems (CHI ’18), 2018;1–6. https://doi.org/10.1145/3170427.3188560 .
Kim S, Goh J, and Jun S. The use of voice input to induce human communication with banking chatbots. In: Companion of the 2018 ACM/IEEE International Conference on Human-Robot Interaction (HRI Companion ’18), 2018;151–152. https://doi.org/10.1145/3173386.3176970 .
Shamekhi A, Liao QV, Wang D, Bellamy RKE and Erickson T. Face value? Exploring the effects of embodiment for a group facilitation agent. In: Proceedings of the 2018 CHI Conference on Human Factors in Computing Systems (CHI ’18),2018;391:1–391:13. https://doi.org/10.1145/3173574.3173965
Torre I, Goslin J, White L and Zanatto D. Trust in artificial voices: a “congruency effect” of first impressions and behavioral experience. In Proceedings of the 2018 Technology, Mind, and Society Conference (TechMindSociety ’18), 2018. Article No. 40. https://doi.org/10.1145/3183654.3183691 .
Yarosh S, Thompson S, Watson K, Chase A, Senthilkumar A, Yuan Y and Brush AJB. Children asking questions: Speech interface reformulations and personification preferences. In: Proceedings of the 17th ACM Conference on Interaction Design and Children (IDC ’18), 2018;300–12. https://doi.org/10.1145/3202185.3202207 .
Stucker BE, Wicker R. Direct digital manufacturing of integrated naval systems using ultrasonic consolidation, support material deposition and direct write technologies. UTAH STATE UNIV LOGAN; 2012.
Kaplan A, Haenlein M. Siri, Siri, in my hand: Who’s the fairest in the land? On the interpretations, illustrations, and implications of artificial intelligence. Bus Horiz. 2019;62(1):15–25.
Humphry J and Chesher C. Preparing for smart voice assistants: Cultural histories and media innovations. New media Soc. 2020;1461444820923679
Moar JS. Cov id-19 and the Voice Assistants Market. Juniper Research. Retrieved Nov ember 25, 2021, f rom https://www.juniperresearch.com/blog/august-2021/covid-19-and-the-voice-assistants-market
Vailshery LS. Topic: Smart speakers. Statista. Retrieved November 25, 2021, from https://www.statista.com/topics/4748/smart-speakers/#:~:text=As%20of%202019%20an%20estimated,increase%20to%20around%2075%20percent
Pal D, Vanijja V, Zhang X, Thapliyal H. Exploring the antecedents of consumer electronics IoT devices purchase decision: a mixed methods study. IEEE Trans Consum Electron. 2021;67(4):305–18. https://doi.org/10.1109/TCE.2021.3115847 .
Pal D, Arpnikanondt C, Razzaque MA, Funilkul S. To trust or not-trust: privacy issues with voice assistants. IT Professional. 2020;22(5):46–53. https://doi.org/10.1109/MITP.2019.2958914 .
Download references
This study was funded by The Asahi Glass Foundation.
Author information
Authors and affiliations.
Innovative Cognitive Computing (IC2) Research Center, King Mongkut’s University of Technology Thonburi, Bangkok, Thailand
Faruk Lawal Ibrahim Dutsinma, Debajyoti Pal & Jonathan H. Chan
School of Information Technology, King Mongkut’s University of Technology Thonburi, Bangkok, Thailand
Suree Funilkul
You can also search for this author in PubMed Google Scholar
Corresponding author
Correspondence to Debajyoti Pal .
Ethics declarations
Conflict of interest.
The author declares that they have no conflict of interest.
Additional information
Publisher's note.
Springer Nature remains neutral with regard to jurisdictional claims in published maps and institutional affiliations.
Rights and permissions
Reprints and permissions
About this article
Dutsinma, F.L.I., Pal, D., Funilkul, S. et al. A Systematic Review of Voice Assistant Usability: An ISO 9241–11 Approach. SN COMPUT. SCI. 3 , 267 (2022). https://doi.org/10.1007/s42979-022-01172-3
Download citation
Received : 06 January 2022
Accepted : 20 April 2022
Published : 03 May 2022
DOI : https://doi.org/10.1007/s42979-022-01172-3
Share this article
Anyone you share the following link with will be able to read this content:
Sorry, a shareable link is not currently available for this article.
Provided by the Springer Nature SharedIt content-sharing initiative
- Voice assistants
- Systematic literature review
- User experience
- ISO 9241-11 framework
- Find a journal
- Publish with us
- Track your research
Breadcrumbs Section. Click here to navigate to respective pages.

A literature review on virtual assistant for visually impaired
DOI link for A literature review on virtual assistant for visually impaired
Click here to navigate to parent product.
There are various communication barriers for visually impaired people, and they have to face various challenges and have to be dependent on other people. This literature review analyses the problems that are being faced by visually impaired people and seek to find an affordable, efficient, feasible, and reliable solution for them by trying to convert the visual world into an audio world and make the visually impaired feel empowered and independent.
- Privacy Policy
- Terms & Conditions
- Cookie Policy
- Taylor & Francis Online
- Taylor & Francis Group
- Students/Researchers
- Librarians/Institutions
Connect with us
Registered in England & Wales No. 3099067 5 Howick Place | London | SW1P 1WG © 2024 Informa UK Limited

An official website of the United States government
The .gov means it’s official. Federal government websites often end in .gov or .mil. Before sharing sensitive information, make sure you’re on a federal government site.
The site is secure. The https:// ensures that you are connecting to the official website and that any information you provide is encrypted and transmitted securely.
- Publications
- Account settings
Preview improvements coming to the PMC website in October 2024. Learn More or Try it out now .
- Advanced Search
- Journal List
- Springer Nature - PMC COVID-19 Collection

A Systematic Review of Voice Assistant Usability: An ISO 9241–11 Approach
Faruk lawal ibrahim dutsinma.
1 Innovative Cognitive Computing (IC2) Research Center, King Mongkut’s University of Technology Thonburi, Bangkok, Thailand
Debajyoti Pal
Suree funilkul.
2 School of Information Technology, King Mongkut’s University of Technology Thonburi, Bangkok, Thailand
Jonathan H. Chan
Voice assistants (VA) are an emerging technology that have become an essential tool of the twenty-first century. The VA ease of access and use has resulted in high usability curiosity in voice assistants. Usability is an essential aspect of any emerging technology, with every technology having a standardized usability measure. Despite the high acceptance rate on the use of VA, to the best of our knowledge, not many studies were carried out on voice assistants’ usability. We reviewed studies that used voice assistants for various tasks in this context. Our study highlighted the usability measures currently used for voice assistants. Moreover, our study also highlighted the independent variables used and their context of use. We employed the ISO 9241-11 framework as the measuring tool in our study. We highlighted voice assistant’s usability measures currently used; both within the ISO 9241-11 framework, as well as outside of it to provide a comprehensive view. A range of diverse independent variables are identified that were used to measure usability. We also specified that the independent variables still not used to measure some usability experience. We currently concluded what was carried out on voice assistant usability measurement and what research gaps were present. We also examined if the ISO 9241-11 framework can be used as a standard measurement tool for voice assistants.
Introduction
Voice assistants (VAs) which are also called intelligent personal assistants are computer programs capable of understanding and responding to users using synthetic voices. Voice assistants have been integrated into different technological devices, including smartphones and smart speakers [ 1 ]. The voice modality is the central mode of communication used by these devices, rendering the graphic user interface (GUI) inapplicable or less meaningful [ 2 ]. People use VA technology in different aspects of their lives, such as for simple tasks like getting the weather report [ 3 ] or managing emails [ 4 ]. In addition, the VA can perform complex tasks like client representative tasks [ 5 ] and controllers in autonomous vehicles [ 6 ]. In other words, VA’s can revolutionize the way people interact with computing systems [ 7 ]. Currently, there is a massive global adoption of voice assistants. A report in [ 8 ] indicates that 4.2 billion VA’s were adopted and used in 2020 alone, with a projected increase to 8.4 billion by 2024. The popularity of VA’s has led to a greater research attention to its usability and user experience aspect.
Usability is a critical factor in the adoption of voice assistants [ 9 ]. A study by Zwakman et al. [ 10 ] highlighted the importance of usability in voice assistants [ 9 ]. An additional study by Coronado et al. [ 11 ] reiterated the importance of usability in human–computer interaction tools. Numerous studies have been carried out on the usability heuristics used in a VA, each study adopting a unique approach. A study by Maguire [ 12 ] used the Nielsen and Molich versions of Voice User Interface (VUI), and the heuristic Voice User Interface (VUI), to evaluate the ease of use of the VA’s. The study affirmed both the two heuristics were appropriate. However, the study noted that one was less problematic to use than the other [ 12 ]. A further study tested VUI heuristics to measure VA efficacy [ 13 ]. However, a critical factor that prevents the VA from adopting the heuristic currently available is the absence of a graphical user interface (GUI). Despite numerous studies on heuristics, the level of satisfaction is still low [ 14 ]. Furthermore, heuristics cannot be used as a standardized approach because they are approximate strategies or empirical rules for decision-making and problem-solving that do not ensure a correct solution. According to a study by Murad [ 16 ], the absence of standardized usability guidelines when developing VA interface presents a challenge in the development of an effective VA [ 15 ]. Another report from Budi & Leipheimer [ 17 ] also suggests that the usability of the VA’s requires improvements and standardization [ 16 ]. To create a standard tool a globally recognized and well-known organization is critical in the process because it eliminates bias and promotes neutrality [ 17 ]. The International Organization for Standardization (ISO) 9241-11 framework is one of the standard usability frameworks widely used for measuring technology acceptance.
According to the ISO 9241–11 framework, usability is defined as “the degree to which a program may be utilized to achieve measurable objectives with effectiveness, efficiency, and satisfaction in a specific context of usage” [ 18 ]. ISO 9241-11 provides a framework for understanding and applying the concept of usability in an interactive system and environment [ 19 ]. The main advantage of using the ISO standard is that industries and developers do not need to build different design measurement tools. This standard is intended to create compatibility with new and existing technologies, and also create trust [ 20 ]. Currently, the system developers do not have any standardized tool created specifically for the measurement of VA usability, consequently, the measures are decentralized, causing confusion among developers. The lack of in-depth assessment of the current heuristics used in the VA design affects the trust and adaptability of their users [ 15 ]. Other emerging technologies such as virtual reality [ 21 ] and game design [ 22 ] have understood the importance of creating an acceptable standardized measurement tool when designing new interfaces. Therefore, VA technology could also benefit significantly from the same concept. As evident from the above discussion, there is little to no focus on VA standardization.
Our study presents a systematic literature review comprising works carried out on the usability of voice assistants. In addition, we use the ISO 9241-11 framework as a standardized measurement tool to analyze the findings from the studies we collected. We chose the ACM and IEEE databases for the selection of our articles because both contain a variety of studies dealing with the usability aspects of VA’s. The following are the contributions of this literature review to the Human--Computer Interaction (HCI) community:
- Our work highlights the studies currently carried out on VA usability. This includes the independent and dependent variables currently used.
- Our study highlights the factors that affect the voice assistants' acceptance and impact the user’s total experience.
- We identify and explain some attributes unique to only voice assistants, such as machine voice.
- We also highlight the evaluation techniques used in previous studies to measure usability.
- Finally, our study tries to compare the existing usability studies with the ISO 9241-11 framework. The decentralized approach of the VA usability measurement makes it vague to understand if the ISO 9241-11 framework is being adhered to whilst developing the usability metrics.
We hope that our input will highlight the integration of the current existing VA usability measures with the ISO 9241-11 framework. This will also verify whether the ISO 9241-11 framework can serve as a standard measure of usability in voice assistants. In conclusion, our study tries to answer the following four research questions:
- RQ 1 : Can the ISO 9241–11 framework be used to measure the usability of the VA’s?
- RQ 2 : What are the independent variables used when dealing with the usability of VA’s?
- RQ 3 : What current measures serve as the dependent variables when evaluating the usability of VA’s?
- RQ 4 : What is the relationship between the independent and dependent variables?
The remaining work is structured as follows. The second section presents the related work. This highlights what previous literature review studies had been carried out on voice agents’ usability; furthermore, the section also highlights the emergent technology that employed the ISO 9241-11 framework as a usability measuring tool. This is followed by the methodology section, which presents the inclusion and exclusion criteria used together with the review protocol. Furthermore, the query created for the database search is presented, and the database to be used is also selected. The fourth section presents the result and analysis. In this phase, the article used for this study is listed. Also, the research questions are answered. The fifth section contains discussion on the result analysis. This includes a more detailed explanation of the relationships between independent and dependent variables. Our insights and observations are included in this section as well.
Literature Review
Previous systematic reviews.
There have been a number of systematic literature reviews concerning VA’s over the years. Table Table1 1 presents the information for a few of the relevant works.
Current literature reviews
# | Article name | Summary | Limitations | Usability focus |
---|---|---|---|---|
[ ] | Smart Home Voice Assistants: A Literature Survey of User Privacy and Security Vulnerabilities | The study explores the potential use vulnerabilities encountered while using the voice assistant. The studies looked at the vulnerabilities, associated attack vectors, and possible mitigation measures that users can take to protect themselves during the use of voice assistant | Privacy and vulnerability are not the primary focus in usability | Personal Smart Home use |
[ ] | Intelligent personal assistants: A systematic literature review | The natural language interfaces allow the human–computer interaction by the translation of the human intention in the controls of the devices, the analysis of the speech or the gestures of the user. The article looked at the major trends, critical areas and challenges of an intelligent personal assistant. The study also proposed a taxonomy for IPA classification. The method used the population, intervention, comparison, outcome, and context (PICOC) criteria | The study did not conduct a thorough review of what was done with respect to the usability of the voice assistant | General use |
[ ] | Virtual Assistants for Learning: A Systematic Literature Review | The motivation, commitment and decreasing interest of students in the learning process has always existed, contributing to increased failures and dropouts. This can be attributed caused due to the difficulties with time management. The growing number of students in higher education makes it impossible to provide individual tutoring and support to each student. This paper systematically examines the use of virtual assistants in tertiary education It focuses on the technology which fuels them, their characteristics and their impact in the learning process | The Study focused on voice assistants used only within an educational environment that motivates users | Education |
[ ] | Voice-Based Conversational Agents for the Prevention and Management of Chronic and Mental Health Conditions: Systematic Literature Review | Chronic and mental diseases are increasingly prevalent throughout the world. As devices in our everyday lives offer more and more voice-based self-service, voice assistant can support the prevention and management of these conditions. This study highlights the current methods used in the evaluation of health interventions for the prevention and management of chronic and mental health conditions delivered through voice assistant | The study only focused on voice assistants used in the health environment alone | Health |
[ ] | Tourists’ Attitudes toward the Use of Artificially Intelligent (AI) Devices in Tourism Service Delivery: Moderating Role of Service Value Seeking | This study examines tourist attitudes towards the use of voice assistants in relatively more utilitarian or hedonic (air and hotel) tourism services. The results of the study suggest that tourism acceptance of VA is influenced by social influence, hedonistic motivation, anthropomorphism, expectation of performance and exertion, and emotions towards artificially intelligent devices. These results suggest that while the use of voice Assistants in the provision of functional services is acceptable, the use of AI devices in the delivery of hedonic services could backfire | The study was not on usability, to be specific, but on adopting voice assistants in the tourism environment | Tourism |
[ ] | Exploring How Older Adults Use a Smart Speaker–Based Voice Assistant in Their First Interactions: Qualitative Study Exploring How Older Adults Use a Smart Speaker–Based Voice Assistant in Their First Interactions: Qualitative Study | Smart speaker-based voice assistants promise support for the aging population, with the benefits of hands-free and eye-free interaction to process applications. This study explores how older adults experience and react to a voice assistant when they first interact with that person. The study discusses design implications that can positively influence older adults using voice assistant, including helping better understand how a voice assistant work and tailored to the needs of older adults | The Study group focused on was only older adults | General Use |
[ ] | A Meta-Analytical Review of Empirical Mobile Usability Studies | This document provides a usability assessment framework tailored to the context of a mobile IT environment. The study conducted a qualitative meta-analysis of more than 100 empirically based mobile usability studies. This study included the contextual factors studied, the dimensions of core and peripheral usage measured. Furthermore, open and unstructured tasks are under-utilized, and the effects of interaction between interactivity and complexity warrant further study | The impacts of User characteristics and environment on usability were not explored in the study | Mobile computing |
[ ] | Evaluation of COVID-19 Information Provided by Digital Voice Assistants | Digital voice assistants are widely used to search for health information during COVID-19. With the rapidly changing nature of COVID-19 information, there is a need to assess the COVID-19 information provided by voice assistants to meet consumer needs and prevent disinformation. The goal of this study is to evaluate the COVID-19 information provided by voice assistants in terms of relevance, accuracy, usability and reliability. The study found that information about this pandemic is evolving rapidly and that users must use good judgment when obtaining COVID-19 information from voice assistants | The study focused only on voice assistants used in Covid-19 related issues | Health |
[ ] | The human side of human-Chatbot interaction: A systematic literature review of ten years of research on text-based chatbot | Over the last ten years there has been a growing interest around text-based chatbot, software applications interacting with humans using natural written language. However, despite the enthusiastic market predictions, ‘conversing’ with this kind of agents seems to raise issues that go beyond their current technological limitations, directly involving the human side of the interaction. This study suggests a number of research opportunities that could be explored over the next few years | The study focused only on chatbot with textual modality. Moreover, chatbot use a Graphic User Interface that is not present in voice assistants | General Use |
[ ] | Voice in Human–Agent Interaction: A Survey | Social robots, conversational agents, voice assistants and other embodied AIs are increasingly a characteristic of daily life. The connection between these different types of intelligent agents is their ability to interact with people by voice. The voice becomes an essential mode of embodiment, communication and interaction between IT operators and end users. This study presents a meta-synthesis of the voice of agents in the conception and experience of agents from a man-centered point of view: voice assistant | The study did not use the ISO 9241–11 framework as a reference in their measurement scale | General Use |
[ ] | Usability of Chabot’s: A Systematic Mapping Study | The use of chatbot has increased considerably in recent years. As a result, it is essential to integrate conviviality into their development. For this reason, it is essential to integrate conviviality in their development. The study identifies the state of the art in the conviviality of chatbot and the applied techniques of human–computer interaction, to analyze how to assess the conviviality of chatbot | The study focused only on chatbot with textual modality. Moreover, chatbot use a Graphic User Interface that is not present in voice assistants | General Use |
[ ] | A Literature Review On Chatbot In Healthcare Domain | The study highlighted Chabot used in the healthcare environment. Also, it compares the techniques such as NLU, NLG, and ML used in chatbot development | The study deals with chatbot with textual modality. Also, the study deals with chatbot used only in a healthy environment | health |
[ ] | Review of Chatbot Design Techniques | The study reviewed the techniques and factors considered when designing a chatbot.Also it highlighted how chatbot worked and what are the type of approaches that are available for chatbot development | The study focuses on chatbot with textual modality, which is different from a voice assistant | Commerce |
[ ] | A Systematic Literature Review of Medical Chatbot Research from a Behavior Change Perspective | The study examined the literature on how people feel about using a medical chatbot in medical communication services. Moreover, The study recommended five design-orientation and highlighted the behavioral aspects such as acceptance, usage, and effectiveness when using chatbot | The study focuses on chatbot with textual modality, which has different factors than voice assistants | Health |
[ ] | A review of chatbot in education: Practical steps forward | The study focused on Chatbot applied within an educational environment; it highlighted how Chatbot are currently being used in a broader educational environment. Moreover, the study also recommended how Chatbot can be applied to enhance students learning experience | The study focuses on chatbot, with textual modality, with different factors than voice assistants | Education |
[ ] | Human-like communication in conversational agents: a literature review and research agenda | The study identified the voice assistant human-like behaviors that have the most effect on relational outcomes during communication | An in-depth analysis of user and conversation assistants attributes was not carried out. Moreover, the study only focused on voice assistants used in management alone | Management |
As highlighted in Table Table1, 1 , multiple systematic literature reviews have been carried out on VA's usability over the years. However, each study has a specific limitation and gap for improvement. For instance, some studies focus on the usability of voice assistants used only in specified fields such as education [ 25 ] and health [ 36 ]. Other studies focus on the usability of voice assistants concerning only specific age groups, such as older adults [ 28 ]. Likewise, although an in-depth analysis of the usability of the VA’s is carried out involving every usability measure in [ 32 ], this study does not use the ISO 9241 framework as a measuring standard. On the other hand, another study in [ 33 ] although uses the ISO 9241 framework as a measuring standard, however, the usage context was chatbots focusing primarily on text-based communication instead of voice. Overall, the available literature reviews on VA’s usability listed in Table Table1 1 supports the view that very few of the current literature review studies on VA’s use the ISO 9241-11 framework as an in-depth tool for measuring usability.
The ISO 9242-11 Usability Framework
The ISO 9241-11 is a usability framework used to understand usability in situations where interactive systems are used and employed, which includes framework environments, products, and services [ 39 ]. Nigel et al. [ 40 ] conducted a study to revise the ISO 9241-11 framework standard, which reiterates the importance of the framework within the concept of usability. A number of studies have been conducted on various technologies using the ISO 9241–11 framework as a tool to measure their usability. This shows the diversified approach when using the framework. For instance, a study by Karima et al. (2016) proposed the use of ISO 9241-11 framework to measure the usability of mobile applications running on multiple operating systems by developers, in which the study identified display resolution and memory capacity as factors that affect the usability of using mobile applications [ 41 ]. Another study used the ISO 9241-11 framework to identify usability factors when developing e-government systems [ 42 ]. This study focused on the general aspect of e-Government system development and concluded the framework could be used as a usability guideline when developing a government portal. In addition, the ISO 9241-11 framework was also used to evaluate other available methods and tools. For instance, a study by Maria et al. [ 44 ] used the framework to evaluate existing tools used in the measurement of usability of software products and artifacts on the web. The study compared existing tools with the ISO 9241-11 measures for efficiency, effectiveness and satisfaction [ 43 ]. ISO 9241–11 framework has also been employed as a method of standardization tool in the geographic field [ 44 ], game therapy in dementia [ 45 ], and logistics [ 46 ]. Despite the ISO 9241-11 usability framework being utilized in different aspects of old and emergent technologies, it has not been used with a VA in the past.
We performed a systematic literature review is this study using the guidelines established by Barbara [ 47 ]. These guidelines have been widely used in other systematic review studies as a result of their rigor and inclusiveness [ 48 ]. In addition, we have added a new quality assessment process to our guidelines. The quality assessment is a list of questions that we use to independently measure each study to ensure its relevance for our review. Our quality evaluation checklists are derived from existing studies [ 49 , 50 ]. The complete guidelines used in this section comprises of four different stages:
- Inclusion and exclusion criteria
- Search query
- Database and article selection.
- Quality assessment.
Inclusion and Exclusion Criteria
The inclusion and exclusion criteria used in our study are developed for completeness and avoidance of bias. The criteria we used for our study are:
- Studies that focus on VA, with voice being the primary modality. In scenarios where the text or graphical user interfaces are involved, they should not be the primary focus.
- Studies are only in the English language to avoid mistakes during translation from another language
- The studies include at least one user and one voice assistant to ensure that the focus is on usability, not system performance.
- Study has a comprehensive conclusion.
- Released between 2000 and 2021, because during this period the vocal assistants started to gain notable popularity
The exclusion criteria are:
- Studies with poor research design, where the study's purpose is not clear are excluded.
- White papers, posters, and academic thesis are excluded.
Search Query
We created the search query for our study using keywords arranged to search the relevant databases. We went through previous studies to find the most relevant search keyword to find what is commonly used in usability studies. After numerous debates among the researchers and seeking two HCI expert's opinion, we chose the following set of keywords: usability, user experience, voice assistants, personal assistants, conversational agents, Google Assistant, Alexa, and Siri. We connected the keywords with logical operators (AND and OR ) to yield accurate results. The final search string used was (“usability” OR “user experience “) AND (“voice assistants” OR “personal assistants” OR “conversational Agents” OR “Google Assistant” OR” Alexa” OR “Siri”). The search was limited to the abstract and title of the study.
Database and Article Selection
Figure 1 highlights the graphic presentation of the selection and filtering process. The figure is adapted from the Prisma flow diagram [ 51 ]. As earlier stated, two databases are used as the sources for our article selection: the Association for Computing Machinery (ACM) and the Institute of Electrical and Electronics Engineers (IEEE). Both databases we used in our study contain the most advanced studies on VA and are highly recognized among the HCI community. The search query returned 340 results from the ACM database and 280 results from the IEEE database. 720 items in both databases were checked for duplication and 165 documents (23%) were found to be duplicated and hence removed. Additionally, more items were filtered by title and abstract. We utilized keyword match to search the title; however, the abstract was read to identify the eligibility criteria. In addition, 399 documents (72%) were removed because they did not meet the eligibility criteria. Finally, 121 documents were removed that were not consistent with the research objectives of our study. At the end of the screening process 29 articles (19%) were finally included in this literature review.

Article selection process
Quality Assessment
The selected items presented in Table Table2 2 are used for assessing the quality of the selected articles. The process was deployed to ensure the reported contents fit into our research. The sections collected from articles such as the methodology used, analysis done, and the context of use within each article were vital to our study. Each question is a three-point scale: “Yes” is scored as 1 point, which means the question is fully answerable. “Partial” is scored as 0.5, which means the question is vaguely answered, and “NO “is scored as 0, which means it is not answered at all. All the 29 sets of finally included articles passed the quality assessment phase.
Quality assessment checklist
Checklist | Definition |
---|---|
C | Are the study aims and objectives clearly stated |
C | Is the article well designed to achieve these aims? |
C | Are the independent variable in the study clearly defined? |
C | Are the dependent variable in the study clearly defined? |
C | Is the study discipline stated clearly? |
C | Are the data collection methods clearly stated |
C | Does the study explain the reliability and validity of the measures? |
C | Are the analysis techniques described adequately? |
C | Are the users/participants’ numbers stated clearly? |
C | Do the results add to the literature? |
Result and Analysis
List of articles.
This section lists and discusses the articles collected in the previous stage. Table Table3 3 presents the list of all the compiled articles. Moreover, we identified the usability focus of each study.
List of compiled articles
# | Article name | Voice assistant type | Usability measure | Years |
---|---|---|---|---|
1 | An Exploration of Speech-Based Productivity Support in the Car [ ] | Car Interface | Effectiveness | 2019 |
Exploring Effects of Conversational Fillers on User Perception of Conversational Agents [ ] | Smart Speaker | Effectiveness, machine Voice(perceived intelligence) | 2019 | |
2 | ||||
3 | I Almost Fell in Love with a Machine”: Speaking with Computer Affects Self-disclosure [ ] | Software Interface | Trust | 2019 |
4 | Clarifying False Memories in Voice-based Search [ ] | Smart Speaker | Satisfaction, efficiency, cognitive load | 2019 |
5 | The Effects of Anthropomorphism and Non-verbal Social Behavior in Virtual Assistants [ ] | Smart Speaker Humanoid | machine Voice(perceived humanness, social presence), cognitive load(attention) | 2019 |
6 | An End-to-End Conversational Style Matching Agent [ ] | Smart Speaker | Trust | 2019 |
7 | Tandem Track: Shaping Consistent Exercise Experience by Complementing a Mobile App with a Smart Speaker [ ] | Smart Speaker Software Interface | Efficiency, Effectiveness | 2020 |
8 | Mapping Perceptions of Humanness in Intelligent Personal Assistant Interaction [ ] | Smart speaker Software Interface | Machine voice(perceived Humanness), Effectiveness | 2019 |
9 | Pattern of Gaze in Speech Agent Interaction [ ] | Humanoid | Machine voice (Social presence), cognitive workload | 2019 |
10 | Conversational Interfaces for a Smart Campus: A Case Study [ ] | Smart Speaker Software Interface | Effectivity | 2020 |
11 | Mental Workload and Language Production in Non-Native Speaker IPA Interaction [ ] | Smart Speaker Software Interface | Cognitive Load, Satisfaction | 2020 |
12 | User Experience of Alexa when controlling music – comparison of face and construct validity of four questionnaires [ ] | Smart speaker | User satisfaction | 2020 |
13 | Machine Body Language: Expressing a Smart Speaker’s Activity with Intelligible Physical Motion [ ] | Humanoid | Machine Voice (Perceived humanness) | 2020 |
14 | Measuring the anthropomorphism, animacy, likeability perceived intelligence and perceived safety of robots [ ] | Humanoid | Machine Voice (Perceived humanness, Anthropomorphism) | 2008 |
15 | At Your Service: Designing Voice Assistant Personalities to Improve Automotive [ ] | Car interface | Attitude(Likeability, acceptance) | 2019 |
16 | Hey, Siri”, “Ok, Google”, “Alexa”. Acceptance- Relevant Factors of Virtual Voice-Assistants [ ] | Smart Speaker Software Interface | Attitude (Trust acceptance) | 2019 |
17 | User experience with smart voice assistants: the accent perspective [ ] | Smart Speaker Software Interface | User satisfaction | 2019 |
18 | Empathy is all you need: How a conversational agent should respond to verbal abuse [ ] | Software Interface | Effective, User satisfaction,Machine voice (social presence) | 2020 |
19 | Gendered Voice and Robot Entities: Perceptions and Reactions of Male and Female Subjects [ ] | Humanoid | User satisfaction, attitude, effectiveness | 2009 |
20 | What If Conversational Agents Became Invisible? Comparing Users’ Mental Models According to Physical Entity of AI Speaker [ ] | Smart speaker | Attitude(trust), machine Voice(Anthropomorphism) | 2020 |
21 | Similarity is more important than expertise: Accent effects in speech interfaces [ ] | Smart Speaker | Effectiveness, attitude(Trust), Efficiency, satisfaction | 2007 |
22 | Can Computer-Generated Speech Have Gender? An Experimental Test of Gender Stereotype [ ] | Software Interface | Attitude, User satisfaction | 2020 |
23 | Designing Social Presence of Social Actors in Human Computer Interaction [ ] | Software Interface | satisfaction | 2003 |
24 | Improving Automotive Safety by Pairing Driver Emotion and Car Voice Emotion [ ] | Software Interface | Effectiveness, Efficiency | 2005 |
25 | Designing Emotional Expressions of Conversational States for Voice Assistants: Modality and Engagement [ ] | Humanoid | Cognitive load, Attitude | 2018 |
26 | The Use of Voice Input to Induce Human Communication with Banking Chabot’s [ ] | Smart Speaker | Attitude | 2018 |
27 | Face Value? Exploring the Effects of Embodiment for a Group Facilitation Agent [ ] | Smart Speaker Software Interface | Attitude | 2018 |
28 | Trust in artificial voices: A “congruency effect” of first impressions and behavioral experience [ ] | Humanoid | Attitude | 2018 |
29 | Children Asking Questions: Speech Interface Reformulations [ ] | Smart Speaker | Cognitive load | 2018 |
Voice Assistant Usability Timeline
We grouped the collected research into three categories, each representing a range of time frames (Fig. 2 ). The categorization is based on voice assistant period breakthroughs. The first category is from 2000 to 2006, which was the year of social media and camera phones, also known as the year of the Y2K bug in telecommunications. During these years, conversational agents started to get noticed with the introduction of the inventions such as the Honda’s Advanced Step in Innovative Mobility (ASIMO) humanoid robot [ 80 ]. The second category ranges from 2007 to 2014. During these years technological advancements got users more exposed to voice assistants through embedding them into smartphones and computers. For instance, Apple first introduced SIRI in 2011 [ 81 ], and Microsoft introduced Cortana in 2014. The last category ranges from 2015 to 2021. This was when the massive adoption of voice assistants took place, making it an all-time high.

Year of publication of selected articles
Based on the year of publication of our selected articles, Fig. 2 clearly shows that the study on VA’s has expanded significantly over the last six years (2014–2021). This can be attributed to the invention of a smart speaker and phone with built-in voice agents [ 82 ]. Another reason for VA popularity is the COVID -19 outbreak that has given a fresh impetus towards touchless interaction technologies like voice [ 83 ].
Different Embodiment Types of VA’s
Smart speakers are the mostly used embodiment of VA’s used in our selected articles. This is due to the current popularity of commercial smart speakers such as Alexa, HomePod, etc. A 2019 study showed that 35% of US households have an intelligent smart speaker, and projected to reach 75% by 2025 [ 84 ]. Use of humanoids is also popular because usability measures such as anthropomorphism are essential for voice assistant usability [ 85 ]. Furthermore, Fig. 3 shows that only a few studies were done on car interface voice assistants. Car interfaces are vocal assistants that act as intermediaries between the driver and the car. The VA car interface allows drivers to access car information and also be able to perform the task without losing focus on driving. The fourth type of software interface refers to a voice assistant software embedded inside smartphones or computers. The studies we have collected have used either the commercialized form of the software interface, such as Alexa and Siri, while others have developed new voice interfaces that are easily accessible to users due to the adoption of smartphones and computers assistants using programming codes and skills. Nevertheless, both are in the forms of different software agents.

Embodiment of Voice assistant used in selected studies
Component of ISO 9241-11 Framework
The ISO 9241-11 framework highlights two components, the context of use and usability measure [ 18 ]. We concentrate on both components to highlight any correlations between usability metrics and the context of use in the selected articles. The context of use consists of the different independent variables along with the techniques used for analyzing them. Likewise, the usability measure represents the dependent variables, i.e., the effect that the independent variables have on the overall experience of the users. Accordingly, the analysis is presented in a bi-dimensional manner in the following sections.
Context of Use
Independent variable.
We split the context of use into an independent variable and the techniques used. The independent variables presented in our study are the physical and mental attributes used to measure a given user interaction outcome. Furthermore, our study grouped the independent variables into five main categories. The grouping is shown in Fig. 4 and is based on the similar themes identified from the collected studies. The five groups included people (user attributes), voice (voice assistant attributes), task, conversational style, and anthropomorphic cues. The voice and people categories are the oldest independent variables used to measure usability. Their relevance is also seen in the recent studies, which indicate that researchers have a high interest in correlating users with the VA’s. On the other hand, anthropomorphic clues and conversational styles are relatively new to the measurement of usability. The task-independent variable is the most used variable of late, perhaps because users always test the VA’s ability to perform certain tasks. It also indicates that VA’s are widely used for various functional and utilitarian aspects. The anthropomorphic cues are seldom used in the second phase (2007–2014). However, it is most widely used in the last range (2015–2021).

Categories of independent variable use over the years
In Table Table4 4 we highlight more details with regards to the different groups of the independent variable collected, and also present examples of the independent variables for each category. We highlight how the independent variables have been applied by the previous studies and in which environment they have been used. We defined each independent variable category in Table Table4, 4 , and explained their sub-categories as well. As evident from Table Table4, 4 , different independent variables are used together in multiple studies. For example, independent voice variables and independent people variables are used simultaneously in various studies, such as personality, gender, and accent. Similarities between multiple independent variables aid to understand the relationship between the variables themselves and their relationship with the usability measures. Furthermore, the table also highlights the kind of experiments carried out. Controlled experiments are effective methods for understanding the immediate cause and effect between variables. However, a noticeable drawback of controlled experiments is the absence of external validity. The results might not be the same when applied in real-world settings. For instance, the simulation experiment on cars is a controlled environment, a driver has no control over the domain in real life. The usability experience of the driver might be different in natural settings and that might sometimes prove fatal.
Independent variables and their categorization
Category definition | Independent variable | Instances | Applications | Environment |
---|---|---|---|---|
Voice The voice category comprised of independent variables that are associated with the voice assistants, these are attributes that the voice assistants possess) | Voice personalities | (Energetic vs Subdued), (Introvert and extrovert) | A study Paired the driver's emotions with that of the Car Voice Emotion state (Energetic and Subdued) to test the effectiveness of similarity between voice and user personality [ ]. Another study showed that a voice personality that uses a similar personality like the user creates more social presence [ ] | Simulation Experiment, Controlled Environment, |
Voice gender | Male vs Female | Studies compared different gender voices (Male and Female) to measure social interaction and trust. Studies showed male voice has a more dominating effect on users than female voice [ , , ] | Controlled Environment, Free real environment | |
Voice Accent | Standard Southern British English accent VS Liverpool accent Vs Birmingham accent Vs synthetic voice, American Accent vs Swedish Accent, Native English speaker vs non-English speaker | Participants create trust expectancy based on the voice accent. The participants tend to trust information with a similar accent, more knowledgeable, sophisticated voice Assistants [ ][ ] | Controlled Environment, | |
People The people category comprised of independent variables that are associated with the users, these are attributes that the users can have | People gender | Male vs Female | Studies showed that Males and females view voice assistants differently in different aspects. Both genders have different takes in the form of embodiment of the voice assistants. Moreover, women trust voice assistants with a female voice. However, in a situation where there is a need for convincing the male voice assistants is more efficacious [ ] | Controlled Environment, Free real environment, mixed environment |
Personality | Introvert vs Extrovert, happy vs upset | A study showed that a person's emotional state or personality could be affected the personality of the voice assistant. [ ] A study showed that social presence is created when a person uses a voice assistant with a similar personality [ ] | Simulation Experiment, Controlled Environment, | |
Query expression | Abuse (Insult, Threat, Swearing) | A study instructed the user to insult the voice assistants while communicating with it, and the VA's response affected the user's outlook and involved usability [ ] | Controlled Environment | |
Experience | UX metric, Self-Efficacy | The study Measured the user face validity and construct validity by correlating UX scores of questionnaires with each other. Another study shows that Participant self-efficacy and experience affect the trust, privacy and language performance of the Voice Assistant [ ] | Controlled Environment, survey | |
Voice accent | American Accent vs Swedish Accent, Native English speaker vs non-English speaker | Participants tend to trust information with a similar accent, then more knowledgeable content, English native speakers do exhaust more mental models when interacting with voice assistants [ , , ] | Controlled Environment, Free real environment, mixed environment | |
Task characteristic The Task characteristic Comprised of independent variables that are associated with tasks that it’s expected the user to carry out during the interaction, this also include the modality of the task | Modality | Voice mode, Textual mode, VA Facial Expression mode. (Smiley) Mixed Interface | A study used modality to test the social presence of the VA. The study shows participants feel a strong social presence when textual modality personality matches the voice personality. Another study showed that nonverbal emotional expressions such as Text box movement and VA Facial Expression mode (Smiley) affect user engagement [ ] | emotional expression design experiment interactive task, controlled Environment, |
Context | Interactive Task, Drawing Task, Executable Task, Driving simulation task, auditory Task Controlling device Volume, audio speech to text | A study used the speech to text as a task on users during driving. The study measured driver engagement and concentration during driving.[ ]Another study used the game theory concept on the users and asked the users to trust the VA in an investment scheme, where the users have a different opinion on what VA to trust [ ] | emotional expression design experiment interactive task, controlled Environment, free real life environment, simulation | |
Conversational Style This is the nature of the conversation from either the user during query or the response of the voice assistants | Response type | Empathetic (Avoidance vs Empathy vs Counterattack) Clarifying Query (No modification vs direct Modification vs negatively clarified) Conversational Fillers (“um”,huh, uh) | The VA response affects the user usability experience, and A Study showed that When VA are insulted, their response type affects the participant's emotional engagement and attitude [ ]. Another study showed that when VA has more information on a query, the follow-up question affects user engagement and efficiency[ ] A study showed that Conversational fillers increase social interaction with the voice Assistant [ ] | Controlled Environment, |
Communication Form | High Consideration(indirect) VS High Involvement (direct) | A study used participant High Consideration and High involvement linguistic style to realize. It is effective when used with a similar voice assistant's linguistic style. [ ] | Controlled Environment, Mixed Environment | |
Anthropomorphic cues These are independent variables on voice assistants that exhibit human attributes and intelligence, this make the user perceive the voice assistants as human | Speech agents’ Personification vs Speech agent personalization | A study compared VA personation, personalization, and neither to measure users' trust and engagement when used by children and adults. The result showed the personalized VA has the highest concentration and trust [ ] | Controlled Environment, | |
Embodiment type (audio Vs smart speaker), or (gaze vs no gaze), humanoid robot, Smart Speaker vs Anthropomorphic Robot (AMR). vs The Anthropomorphic Social Robot (AMSR) | Numerous studies have used embodiment type to measure usability; a study compared a VA with gaze with another VA without gaze to measure the user anthropomorphism. Another study compared physical smart speakers with the absence of speakers but just voice to test the user trust and engagement [ , , , ] | Controlled Environment, |
Techniques Used
We identified seven techniques that researchers have used as shown in Fig. 5 . The quantitative experiments are the most used and the oldest technique used on voice assistants based on our data collected. The quantitative method is sometimes used as a standalone experiment and sometimes with other techniques [ 54 ]. It is worthy of notice that cars simulation experiments involving VA’s were first used in 2000. Other experiments on human communication with self-driving cars have been carried out since 1990’s. making it one of the oldest techniques for usability measurement. More accurate technique was introduced later, such as the interaction design. The interaction design employed by studies such as [ 61 ] provides a real-time experiment scenario. This avoids the drawback such as bias when using quantitative methods. Factorial design studies are majorly used by studies that compare two or more entities in a case study [ 55 ]. They are utilized mainly by studies using two or more independent variables together.

Technique used in our studies over the span period of time
Usability Measure (Dependent Variable)
This subsection of our study focuses on the usability measurement of our research. Moreover, the findings are used to answer RQ 1 and RQ 3 . The ISO 9241-11 framework grouped usability measures into three categories; effectiveness, efficiency, and satisfaction. According to the ISO 9241-11 framework, “effectiveness is the accuracy and completeness with which users achieve specified goals.”, Whereas “Efficiency is the resources expended concerning accuracy and completeness in which users achieve goals” and “satisfaction is the freedom from discomfort and positive attitudes towards the use of the product” [ 18 ].
In numerous studies, the usability measures used were clearly outside the scope of the ISO 9241-11 framework. In total, we identified three additional usability categories attitude, machine voice (anthropomorphism), and cognitive load. The graphical representation of the different usability measures identified in this study is presented in Figs. 6 and and7. 7 . Futhermore, the figures also highlights the percentage of studies that used the mentioned usability measures in the ISO 9241-11 framework and those that are outside the framework. Based on our compiled result, the user satisfaction and effectiveness are the earliest usability measures used when measuring VA’s usability. Some studies used performance and productivity as subthemes to measure effectiveness [ 62 ]. The measure of usability has been carried out both subjectively and objectively. For instance, studies have measured the VA effectiveness by subjective means by using quantitative methods such as questionnaire tools [ 72 ]. In contrast, other studies have used objective methods such as average completed interaction [ 69 ]. Multiple usability measures are sometimes applied in the same research; for instance some studies measured effectiveness alongside efficiency and satisfaction [ 66 , 70 ]. Learnability, optimization, and ease of use have been used as subthemes to measure efficiency. Interactive design is the most effective experiment that provides real-time results employed [ 56 , 79 ]. The ISO 9241-11 framework works well with effectiveness, efficiency, and satisfaction; however, the users have more expectations from the voice assistant with the recent advancement of VA capabilities. Our compiled result showed that more than half of the studies are not carried out in accordance with the standard ISO 9241-11 framework (Fig. 7 ). The other usability measures we identified outside the ISO 9241-11 framework are attitude, machine voice, and cognitive load.

Usability measurement used over the years on our compiled articles

Percentage of ISO 9241–11 framework usability measures and non ISO 9241–11
Attitude is a set of emotions, beliefs, and behavior towards the voice assistants. Attitude results from a person’s experience and can influence user behavior. Attitude is subjected to change and is not constant. Understanding the user attitude towards the VA has become an active research area. Numerous studies have used different methods to measure subthemes of attitude such as trust, closeness, disclosure, smartness, and honesty [ 60 , 78 ]. Likeability is also a subtheme of attitude, and it has been used to measure the compatibility, trust, and strength between the user and VA’s [ 56 , 57 ]. Moreover, embodiment type affects the user attitude as well, A study highlighted how gaze affects the user attitude toward VA [ 59 ], and it shows VA with gaze creates trust.
We defined machine voice (anthropomorphism) as the user attribution of human characteristics and human similarity to the voice assistant. We considered machine voice an important usability measure that only applies to voice assistants due to their primary modality being the voice. Considering that fact, the measure of machine voice has also spiked currently it becomes obvious that it has been drawing a lot of interest. One of the direct purposes of the VA is to sound as humanly as possible. When the users will perceived the machines to be more human, it built more trust, which will result in a better usability experience.
The cognitive load might be mistaken for efficiency. Nevertheless, they are different. We defined cognitive load as the amount of mental capacity a person applies to communicate successfully with the VA. When it comes to VA, actions such as giving out commands require cognitive thinking and approach. The cognitive load is measured by specific characteristics unique to the VA, such as attention time during the use of the VA [ 76 ] and the user’s mental workload during use [ 77 ].
To answer RQ 1 ( can the ISO 9241–11 framework be used to measure the usability of the VA’s?), none of the existing works have used the ISO 9241-11 framework solely for the purpose of usability evaluation. It has been supplemented by other factors that we have presented above that are outside the scope of this framework.
Relationship Between the Independent variables and Usability Measures
After identifying the independent and dependent variables, in Tables Tables5 5 and and6 6 we show how they are inter-related for having a better understanding of the usability scenario of the VA’s. While Table Table5 5 focuses on the ISO 9241-11 specific factors, Table Table6 6 considers the non-ISO factors specifically.
Relationship between independent variables and ISO 9241–11 framework measurement
Dependent variables | Effectivity | Efficiency | Satisfaction | ||||||||
---|---|---|---|---|---|---|---|---|---|---|---|
Independent variables | Productivity | Performance | Value | Learnability | Optimization | Ease of use | Feasibility | Decision making | User experience | Continued use | Conformity |
Voice Assistants | |||||||||||
Personalities | x | x | x | x | x | ||||||
Gender | x | x | x | x | x | ||||||
Accent | x | x | x | x | x | ||||||
People | |||||||||||
Gender | x | x | x | x | |||||||
Personality | x | x | x | ||||||||
Query expression | |||||||||||
Experience | x | x | x | ||||||||
Accent | x | x | x | x | x | x | |||||
Task characteristics | |||||||||||
Modility | x | x | x | x | x | x | x | ||||
Context | x | x | |||||||||
Communication type | |||||||||||
Response type | x | x | x | x | x | ||||||
Conversational type | x | x | x | x | x | x | |||||
Anthropomorphism | |||||||||||
Embodiement type | x | x | x | x | x | x | |||||
Humanoid/robott | x | x | |||||||||
Smart Speaker, Robot,Anthropormorphic Robot | x | x | x | x | x | x |
Relationship between independent variables and non- ISO 9241–11 framework measurement
Dependent variables | Attitude | Machine voice | Cognitive load | |||||
---|---|---|---|---|---|---|---|---|
Independent variables | Trust | Likeability | Acceptance | Perceived intelligence | Perceived humanness | Social presence | Mental workload | Attention |
Voice assistant | ||||||||
Personalities | x | x | x | x | ||||
Gender | x | x | x | x | x | |||
Accent | x | x | x | x | x | x | ||
People | ||||||||
Gender | x | x | x | |||||
Personality | x | x | x | x | x | |||
Query expression | x | x | ||||||
Experience | x | x | x | x | ||||
Accent | x | x | x | x | x | x | ||
Task Characteristics | ||||||||
Modality | x | x | x | x | x | x | x | |
Context | x | x | x | x | ||||
Communication Type | ||||||||
Response type | x | x | x | x | x | x | ||
Conversational type | x | x | x | x | x | x | ||
Anthropomorphism | ||||||||
Embodiment type | x | x | x | x | x | x | x | |
Humanoid/robot | x | x | x | x | x | |||
Smart speaker, robot, anthropomorphic robot | x | x | x | x |
The independent variables are grouped into categories and represented by table rows, with every category consisting of multiple independent variables. Moreover, the usability measures have been presented in the column of the table. Every usability measure is made up of different sub-themes, which are all presented on the table as well. The tables highlight the relationship between the independent and usability measures. An “X” mark present in each cell represents a study present between that independent variable and usability measure subtheme. Nonetheless, an empty cell indicates that there is no study carried out to link that relationship between the usability measure and independent variable.
Independent Variable and Usability Measures
Our study revealed what has been previously carried out in VA usability and revealed the gaps that are yet to be addressed. We analyzed the usability measures and their relationship to the so-called independent variables. There is an easy accessibility to VA’s due to the development of different embodiment types such as speakers, humanoids, and robots. However, there is so much less focus on embodiment types and their relationship to effectiveness and anthropomorphism, which needs more attention. Some relationship gaps and associations are apparent, while some are vague. For instance, the independent variable “ accent ”, has often been connected with its effectiveness on users. However, what is left unanswered is if the VA accents impart the same efficacy on users of the same or different genders. Another notable gap is gender and efficiency, with very few studies on that. This will be an essential aspect to understand and apply with the recent massive adoption of voice assistants in different contexts. Another obvious gap is the query expression relationship with any ISO 9241-11 framework measures. The query expression is how a user expresses their query to the voice assistants. The query expression has been known to increase the trust and attitude of the user towards the VA. However, its relationship to usability measures such as efficiency, satisfaction, and effectiveness is still under-researched. Knowing the right way to ask queries (questions) defines the type of response a user gets. An incorrect response will be received if the right question is expressed incorrectly. From a mental model, when a user has too much energy and thought to frame a question, it affects the VA efficiency and satisfaction. However, this has not been proven by any current study.
The VA response types increase effectiveness and trust. However, its relationship to user acceptance is still unknown. Another exciting intersection is the anthropomorphic cues and attitude, which results from anthropomorphic emotional response than a practical one. Attitude is an emotional response to a giving state, hence its strong connection with anthropomorphism. The attitude toward the VA is a highly researched area [ 86 ]. Trust, likeability, and acceptance are subthemes that focused on the attitude usability measure. This can be attributed to the importance of trust while using emergent technologies such as voice assistants. User trust in voice assistants is an essential aspect with the rise of IoT devices, and user mistrust affects the acceptance and effectiveness of the VA’s [ 87 ]. Multiple studies measured user trust while using machine voice categories as an independent variable. That could be attributed to the lack of GUI in VA. Furthermore, the voice modality must be enough to cultivate user trust. Noticeably subjective methods were widely employed when measuring the user attitudes; even though subjective measures often relate to the variables they are intended to capture; however, they are also affected by cognitive biases.
The ISO 9241-11 framework is an effective tool when measuring effectiveness, efficiency, and satisfaction. However, it is not applicable when measuring usability’s, such as attitude, machine voice, and mental load. These are all measurements that are uniquely associated with voice assistants. Therefore, the ISO 9241-11 framework could be expanded to include such usability aspects.
Technique Employed
The factorial design adapts well when used in a matched subject design experiments [ 56 ]. Based on the studies collected, machine learning is not well used as an analytic tool in usability. This could be attributed to the technical aspects of machine learning and it is still relatively a new field. However, with machine learning third-party tools more analysis will be carried out. Wizard of Oz, and interactive design started gaining popularity in 2015–2021. Moreover, the Wizard of Oz and interactive techniques are more effective when using independent variables such as anthropomorphic cues. The anthropomorphic cue independent variables is used with Wizard of Oz. techniques and interaction design more than any other techniques. This could be recognized to the importance of using objective methods to avoid biased human responses. Furthermore, “machine voice” is a fairly popular usability measure. This could be attributed to the VA developers trying to give the VA a more human and intelligent attributes. The more users perceive the machine voice as intelligent and humanlike, the more they trust and adopt it. More objective technique methods should be created and used on the independent variables when measuring machine voice. Subjective techniques such as Quantitative methods are easy to use and straightforward. However, they can produce biased results.
Interactive design experiments are the most commonly used technique employed to measure the usability. However, the interaction depends on voice modality, making it different from the traditional interaction design that uses visual cues as part of its essential components. Moreover, interaction design also triggers an emotional response, which makes it effective when measuring user attitude. The absence of visual elements in interactive design used might debatably defeat the purpose of clear communication. A new standard of interaction design uniquely for voice modality should be done.
Future Works and Limitation
One limitation in our study was using a few databases as our articles source; in future studies, we intend to add more journal databases such as Scopus, and Taylor and Francis. The majority of the experiment studies we collected was conducted in a controlled environment; future studies will focus on usability measures and independent variables, that are used in natural settings; furthermore, the results can be compared together More studies should be carried out on objective techniques, also how they could cooperate with subjective techniques. This is vital because, with the rise of user expectations of voice assistants, it will be essential to understand how techniques complement each other in each usability measurement.
Our study aimed to understand what is currently employed for measuring voice assistant usability, and we identified the different independent variables, dependent variables, and the techniques used. Furthermore, we also focused on using the ISO 9241-11 framework to measure the usability of voices assistants. Our study classified five independent variable classes used for measuring the dependent variables. These separate classes were categorized based on the similarities between the member groups. Also, our study used the three usability measures in the ISO 9241-11 framework in conjunction with the other three to serve as the dependable variables. We uncovered that voice assistants such as car interface speakers were not studied enough, and currently, smart speakers have the most focus. Dependent variables such as machine voice (anthropomorphism) and attitude recently have more concentration than the old usability measures, such as effectiveness. We also uncovered that usability is dependent on the context of use, such as the same independent variables could be used in different usability measures. Our study highlights the relationship between the independent and dependent variables used by other studies. In conclusion, our study used the ISO 9241-11 to analyse usability. We also highlight what has been carried out on VA’s usability and what gaps are left. Moreover, we concluded even though there is a lot of usability measurement carried out, there are still many aspects that have not been researched. Furthermore, the current ISO 9241-11 framework is not suitable for measuring the recent advancement of VA because the user needs and expectation have changed with the rise of technology. Using the ISO 9241-11 framework will create ambiguity in explaining some usability measures such as machine voice, attitude and cognitive load. However, it has the potential to be a foundation for future VA usability frameworks.
This study was funded by The Asahi Glass Foundation.
Declarations
The author declares that they have no conflict of interest.
Publisher's Note
Springer Nature remains neutral with regard to jurisdictional claims in published maps and institutional affiliations.
Contributor Information
Faruk Lawal Ibrahim Dutsinma, Email: [email protected] .
Debajyoti Pal, Email: [email protected] , Email: [email protected] .
Suree Funilkul, Email: ht.ca.ttumk.tis@eerus .
Jonathan H. Chan, Email: ht.ca.ttumk.tis@nahtanoj .
Academia.edu no longer supports Internet Explorer.
To browse Academia.edu and the wider internet faster and more securely, please take a few seconds to upgrade your browser .
Enter the email address you signed up with and we'll email you a reset link.
- We're Hiring!
- Help Center
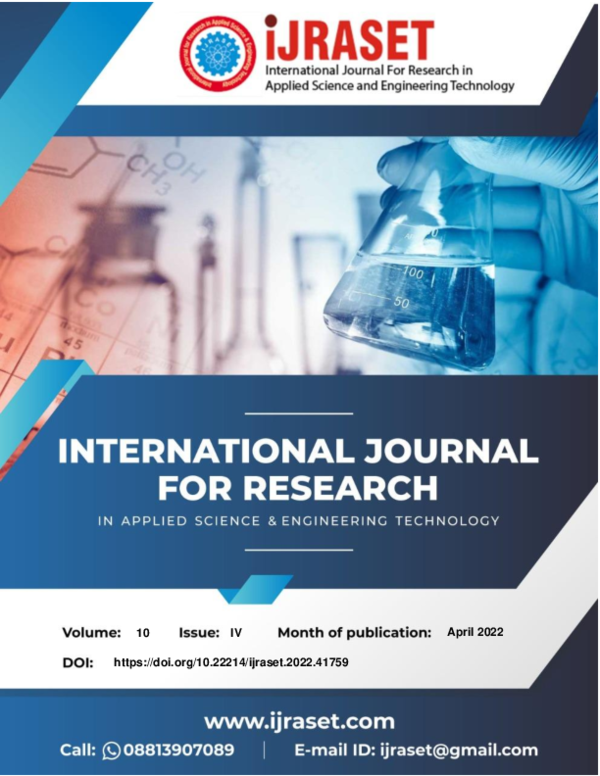
A Literature Survey on Voice Assistance

2022, International Journal for Research in Applied Science & Engineering Technology (IJRASET)
Artificial Intelligence has been in great use when it comes to day to day life. Computer science defines AI research as the study of brilliant agents. In almost any direction one turns today, some form of computer-based information processing technology intrudes, whether to the individual knowingly or not. Artificial Intelligence (AI) has already changed our lifestyle. AI device that perceives its environment and takes actions that maximize its chance of successfully achieving its goals. Input to recommendation algorithm can be a database of user and items and output recklessly will be the recommendations. The user is the input into system by voice or text. This paper presents a new approach for smart search. Overall, in world there are many people who use assistant. The paper presents applications of virtual assistant and also describes provocation of applying virtual assistant technology.
Related Papers
International Journal of Computer Applications
shahenda sarhan
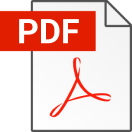
IRJET Journal
Artificial Intelligent has been in great use when it comes to day to day life. Computer science defines AI research as the study of brilliant agents. Every In almost any direction one turns today, some form of computer-based information processing technology intrudes, whether to the individual knowingly or not. Artificial Intelligence (AI) has already change our lifestyle. AI device that perceives its environment and takes actions that maximize its chance of successfully achieving its goals. Input to recommendation algorithm can be a database of user and items and output recklessly will be the recommendations. The user is the input into system by voice or text. This paper presents a new approach for smart search. Overall in world there are many people who use assistant. The paper presents applications of virtual assistant that helps in providing chance for humanity in various domains. This paper also describes provocation of applying virtual assistant technology.
In this modern era, day to day life become smarter & interlinked with technology. We already know some voice assistant like google, Siri etc. Now in our voice assistant system, it can act as your smart friend, daily schedule manager, to do writer, calculator & search tool. This project works on speech input & give output through speech &text on screen. This assistant attaches with the world wide web to provide result that the user required. Natural language processing algorithm helps machines to engage in communication using natural human language in many forms
International Journal for Research in Applied Science & Engineering Technology (IJRASET)
IJRASET Publication
Workers were replaced by machines throughout the commercial revolution, sending more people into the service industry. Chatbots and voice assistants, that might offer support to customers or users, square measure currently a part of the digital revolution's assault on this field. Voice assistants (VA) are the type of voice-enabled artificial intelligence (AI). AI refers to some level of intelligence displayed by digital interfaces, or the ability of algorithms to mimic intelligent human behaviour. However, AI refers to "cognitive" functions that we tend to escort the human mind, including problem solving and learning. The counselling response model provides a suitable response by combining the users' input and the emotional status of the user; this can have a consolatory impact which will create the user loaded down with depression feel higher. I.
This paper discusses about voice assistants, compares several voice assistants like Siri, Alexa, Cortana and Google Assistant. Advantages and disadvantages of each assistant have been summarised. Performance analysis has been conducted and market share of every assistant has been studied. Limitations and future scope of each voice assistant has been reviewed.
In recent years, Artificial Intelligence (AI) and Machine Learning (ML) has shown progress in the field of technology. We know that the future of technology can be full of robotics. One of the most useful application of AI is Natural Language Processing (NLP). Voice Assistants is one of the greatest innovation of all time. It changes the way of living of the people in many aspects. Mainly it was first introduced in smart-phones and then it gets its own popularity in the market. Voice assistants are using cloud computing for communication with the users. It is mainly used in households to control a lot of technology related devices. Most common devices that are using voice assistants are smart speakers and they are being used in colleges, homes, schools etc. There are many voice assistants present in the market such as 'SIRI' by Apple, 'Alexa' by Amazon, 'Bixby' by Samsung. We are also trying to create a basic Voice Assistant by using Python. There are so many useful technology such as speech recognition that will be used in this project. We are creating a VA names Arsenal that is capable of executing a lots of commands. The purpose of this paper is to study how voice assistants and smart speakers are used in everyday life and they way in which we can create a voice assistant.
Ganna Zavolodko
Natural user interfaces are becoming popular. One of the most common today is interfaces with activated voice, including smart personal assistants such as Google Assistant, Alexa, Cortana, Siri, Alice, Bixby, Mycroft. This article presents the results of their evaluation in three dimensions: capabilities, language support, and how natural responses users experience. Evaluations were performed by analyzing existing reviews. The results show that Alexa and Google Assistant are much better than Siri and Cortana. However, there is no statistically significant difference between Alexa and Google Assistant, and neither of them integrates into modern messengers with a note-taking function, which is a significant disadvantage of such devices.
Today's era is the era of digitalization. Having smart phones and desktops is no less than having the world on our fingertips. Our lifestyle is involving being busy day by day. That busy, that people even find it a load to even type something to perform a task. So here comes virtual assistant at rescue. Just speak to it and the task is done. From sending a hello on WhatsApp to your friend to sending a full fleshed email to your boss virtual assistant will do it all for you. With time voice search is dominating over text searching. But what are virtual assistants? A software program that helps us perform our daily task just by speaking to it is a virtual assistant. A waking word is necessary to activate the software. This system can be used efficiently on desktops. The premise behind starting this project was that the data present on the web is sufficient and is openly available that can be used to build a virtual assistant that can make and perform intelligent decision for the user.
thahir mohamed
Intelligent personal assistants (IPA) enable voice applications that facilitate people’s daily tasks. However, due to the complexity and ambiguity of voice requests, some requests may not be handled properly by the standard natural language understanding (NLU) component. In such cases, a simple reply like “Sorry, I don’t know” hurts the user’s experience and limits the functionality of IPA. In this paper, we propose a two-stage shortlister-reranker recommender system to match third-party voice applications (skills) to unhandled utterances. In this approach, a skill shortlister is proposed to retrieve candidate skills from the skill catalog by calculating both lexical and semantic similarity between skills and user requests. We also illustrate how to build a new system by using observed data collected from a baseline rule-based system, and how the exposure biases can generate discrepancy between offline and human metrics. Lastly, we present two relabeling methods that can handle the ...
Loading Preview
Sorry, preview is currently unavailable. You can download the paper by clicking the button above.
RELATED PAPERS
Journal of Advanced Research in Dynamical and Control Systems
Simhadri Madhuri
Vikrant Chole
IEEE Signal Processing Magazine
Algorithms for Intelligent Systems
Harsh Parmar
Larbi Guezouli
International Journal of Engineering Research & Technology (IJERT)
IJERT Journal
International Journal of Innovative Research in Technology
Brindha Manickavasakan
Information Processing & Management
Danny Crookes
Proceedings of the International Conference on Science and Technology (ICST 2018)
Irwan Afandi
International Journal of Advance Research, Ideas and Innovations in Technology
Ijariit Journal
International Journal of Scientific Research in Science and Technology
International Journal of Scientific Research in Science and Technology IJSRST
International Journal of Scientific Research in Science, Engineering and Technology
International Journal of Scientific Research in Science, Engineering and Technology IJSRSET
Binny Khanna
Restaurant Business
Mamta Brahmbhatt
Benedikt Schmidt
International Journal of Engineering Research and Technology (IJERT)
Frontiers in big data
Georgios Kouroupetroglou
International Journal IJRITCC , Bharathi K
RELATED TOPICS
- We're Hiring!
- Help Center
- Find new research papers in:
- Health Sciences
- Earth Sciences
- Cognitive Science
- Mathematics
- Computer Science
- Academia ©2024
Information
- Author Services
Initiatives
You are accessing a machine-readable page. In order to be human-readable, please install an RSS reader.
All articles published by MDPI are made immediately available worldwide under an open access license. No special permission is required to reuse all or part of the article published by MDPI, including figures and tables. For articles published under an open access Creative Common CC BY license, any part of the article may be reused without permission provided that the original article is clearly cited. For more information, please refer to https://www.mdpi.com/openaccess .
Feature papers represent the most advanced research with significant potential for high impact in the field. A Feature Paper should be a substantial original Article that involves several techniques or approaches, provides an outlook for future research directions and describes possible research applications.
Feature papers are submitted upon individual invitation or recommendation by the scientific editors and must receive positive feedback from the reviewers.
Editor’s Choice articles are based on recommendations by the scientific editors of MDPI journals from around the world. Editors select a small number of articles recently published in the journal that they believe will be particularly interesting to readers, or important in the respective research area. The aim is to provide a snapshot of some of the most exciting work published in the various research areas of the journal.
Original Submission Date Received: .
- Active Journals
- Find a Journal
- Proceedings Series
- For Authors
- For Reviewers
- For Editors
- For Librarians
- For Publishers
- For Societies
- For Conference Organizers
- Open Access Policy
- Institutional Open Access Program
- Special Issues Guidelines
- Editorial Process
- Research and Publication Ethics
- Article Processing Charges
- Testimonials
- Preprints.org
- SciProfiles
- Encyclopedia
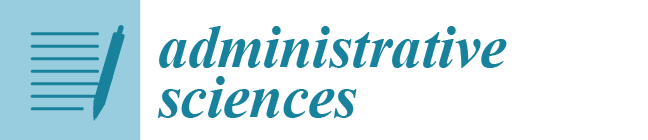
Article Menu
- Subscribe SciFeed
- Recommended Articles
- Google Scholar
- on Google Scholar
- Table of Contents
Find support for a specific problem in the support section of our website.
Please let us know what you think of our products and services.
Visit our dedicated information section to learn more about MDPI.
JSmol Viewer
Ai-driven chatbots in crm: economic and managerial implications across industries.
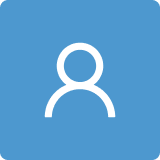
1. Introduction
1.1. historical background, 1.2. definition and significance.
- What are the economic impacts of integrating AI-driven chatbots into CRM systems across different industries?
- How do AI-driven chatbots influence managerial decision-making and operational efficiency within organizations?
- What are the potential future developments and challenges in the use of AI-driven chatbots for CRM?
2. Theoretical Background
2.1. implications of ai on data collection and analysis, 2.2. implications of ai on service availability, 2.3. implications of ai on decision-making, 2.4. implications of ai on customer experience, 2.5. ethical considerations, 3. materials and methods.
- How has the integration of AI-driven chatbots impacted customer relationship management in your industry?
- What specific economic benefits have you observed since implementing AI-driven chatbots in customer service operations?
- In what ways do AI-driven chatbots enhance managerial decision-making processes within your organization?
- Can you provide examples of successful industry applications of AI-driven chatbots in customer relationship management?
- How do you anticipate the role of AI-driven chatbots evolving in the future of customer service and relationship management within your industry?
4. Results and Discussion
5. conclusions, author contributions, institutional review board statement, informed consent statement, data availability statement, conflicts of interest.
- Abousaber, Inam, and Haitham F. Abdalla. 2023. Review of using technologies of artificial intelligence in companies. International Journal of Communication Networks and Information Security 15: 101–8. [ Google Scholar ] [ CrossRef ]
- Ande, Janaki Rama Phanendra Kumar, Aleena Varghese, Suman Reddy Mallipeddi, Dileep Reddy Goda, and Sridhar Reddy Yerram. 2017. Modeling and Simulation of Electromagnetic Interference in Power Distribution Networks: Implications for Grid Stability. Asia Pacific Journal of Energy and Environment 4: 71–80. [ Google Scholar ] [ CrossRef ]
- Anghel, Laurenţiu-Dan, Luminiţa Nicolescu, Valerică Olteanu, and Răzvan Zaharia. 2005. Marketing: Management Marketing . Bucuresti: Editura Politehnica Press. [ Google Scholar ]
- Bahari, T. Femina, and M. Sudheep Elayidom. 2015. An efficient CRM-data mining framework for the prediction of customer behaviour. Procedia Computer Science 46: 725–31. [ Google Scholar ] [ CrossRef ]
- Berry, Leonard L. 1995. Relationship marketing of services– Growing Interest, emerging perspectives. Journal of the Academy of Marketing Science 23: 236–45. [ Google Scholar ] [ CrossRef ]
- Boustani, Nada Mallah. 2020. Traditional banks and fintech: Survival, future and threats. In ICT for an Inclusive World: Industry 4.0–Towards the Smart Enterprise . Berlin and Heidelberg: Springer, pp. 345–59. [ Google Scholar ]
- Boustani, Nada Mallah. 2022. Artificial intelligence impact on banks clients and employees in an Asian developing country. Journal of Asia Business Studies 16: 267–78. [ Google Scholar ] [ CrossRef ]
- Boustani, Nada Mallah, May Merhej Sayegh, and Zaher Boustany. 2022. Attitude towards Online Shopping during Pandemics: Do Gender, Social Factors and Platform Quality Matter? Journal of Risk and Financial Management 15: 474. [ Google Scholar ] [ CrossRef ]
- Christopher, Martin, Adrian Payne, and David Ballantyne. 1991. Relationship Marketing: Bringing Quality, Customer Service and Marketing Together . Oxford: Butterworth Heinemann. [ Google Scholar ]
- Damania, Lesha. 2019. Use of Ai in customer relationship management. In Emerging Research Trends in Management and Social Science . Guwahati: Empyreal Publishing House, pp. 59–64. [ Google Scholar ]
- Dwyer, F. Robert, Paul H. Schurr, and Sejo Oh. 1987. Developing buyer-seller relationships. Journal of Marketing 51: 11–27. [ Google Scholar ] [ CrossRef ]
- Eisenhardt, Kathleen M. 1989. Building Theories from Case Study Research. Academy of Management Review 14: 532–50. [ Google Scholar ] [ CrossRef ]
- Hicham, Nouri, Habbat Nassera, and Sabri Karim. 2023. Strategic framework for leveraging artificial intelligence in future marketing decision-making. Journal of Intelligent Management Decision 2: 139–50. [ Google Scholar ] [ CrossRef ]
- Inavolu, Sai Mounika. 2024. Exploring AI-Driven Customer Service: Evolution, Architectures, Opportunities, Challenges and Future Directions. Available online: https://www.researchgate.net/profile/Sai-Mounika-Inavolu/publication/381224987_Exploring_AI-Driven_Customer_Service_Evolution_Architectures_Opportunities_Challenges_and_Future_Directions/links/6662072985a4ee7261aaed02/Exploring-AI-Driven-Customer-Service-Evolution-Architectures-Opportunities-Challenges-and-Future-Directions.pdf (accessed on 16 February 2024).
- Kalaiyarasan, B., K. Gurumoorthy, and A. Kamalakannan. 2023. AI-Driven Customer Relationship Management (CRM): A Review of Implementation Strategies. Paper presented at the International Conference on Computing Paradigms (ICCP2023), Yelagiri Hills, Tamil Nadu, India, December 15–16; pp. 33–38. Available online: https://www.researchgate.net/publication/376618237_AI-Driven_Customer_Relationship_Management_CRM_A_Review_of_Implementation_Strategies (accessed on 16 February 2024).
- Kanungo, Satyanarayan. 2024. AI-driven resource management strategies for cloud computing systems, services, and applications. World Journal of Advanced Engineering Technology and Sciences 11: 559–66. [ Google Scholar ] [ CrossRef ]
- Kouroupis, Konstantinos, Dimitrios Vagianos, and Aikaterini Totka. 2021. Artificial Intelligence and Customer Relationship Management: The Case of Chatbots and Their Legality Framework. East European Yearbook on Human Rights 4: 5–24. [ Google Scholar ] [ CrossRef ]
- Kumar, Virender, Divya Ramachandran, and Binay Kumar. 2020. Influence of new-age technologies on marketing: A research agenda. Journal of Business Research 125: 864–77. [ Google Scholar ] [ CrossRef ]
- Kumari, Sharda. 2021. Context-Aware AI-Driven CRM: Enhancing Customer Journeys Through Real-Time Personalization and Predictive Analytics. ESP Journal of Engineering and Technology Advancements 1: 7–13. [ Google Scholar ]
- Ledro, Cristina, Anna Nosella, and Ilaria Dalla Pozza. 2023. Integration of AI in CRM: Challenges and guidelines. Journal of Open Innovation 9: 100151. [ Google Scholar ] [ CrossRef ]
- Lokuge, Sachithra, Darshana Sedera, Thilini Ariyachandra, Saj Kumar, and Vadlamani Ravi. 2020. The next wave of CRM innovation: Implications for research, teaching, and practice. Communications of the Association for Information Systems 46: 23. [ Google Scholar ] [ CrossRef ]
- Marr, Bernard. 2015. The Most Revealing Big Data Quotes. World Economic Forum , January 9. Available online: https://www.weforum.org/agenda/2015/01/the-most-revealing-big-data-quotes/ (accessed on 16 February 2024).
- Mittelstadt, Brent. 2021. Common Ethical Challenges in AI—Human Rights. Human Rights and Biomedicine, December. Available online: https://www.coe.int/en/web/bioethics/common-ethical-challenges-in-ai (accessed on 16 February 2024).
- Morgan, Robert M., and Shelby D. Hunt. 1994. The commitment-trust theory of Relationship Marketing. Journal of Marketing 58: 20. [ Google Scholar ] [ CrossRef ]
- Mullangi, Kishore, Sai Sirisha Maddula, Mohamed Ali Shajahan, and Arun Kumar Sandu. 2018. Artificial Intelligence, Reciprocal Symmetry, and Customer Relationship Management: A Paradigm Shift in Business. Asian Business Review 8: 183–90. [ Google Scholar ] [ CrossRef ]
- Naslednikov, Mikhail. 2024. The Impact of Artificial Intelligence on Customer Relationship Management (CRM) Strategies. Bachelor’s thesis, Haaga-Helia University of Applied Sciences, Helsinki, Eastern Pasila, Finland. [ Google Scholar ]
- Osarenkhoe, Aihie, and Az-Eddine Bennani. 2007. An exploratory study of implementation of customer relationship management strategy. Business Process Management Journal 13: 139–64. [ Google Scholar ] [ CrossRef ]
- Owolabi, Omoshola S., Prince C. Uche, Nathaniel T. Adeniken, Christopher Ihejirika, Riyad Bin Islam, and Bishal Jung Thapa Chhetri. 2024. Ethical implication of artificial intelligence (AI) adoption in financial decision making. Computer and Information Science 17: 1–49. [ Google Scholar ] [ CrossRef ]
- Payne, Adrian, and Pennie Frow. 2005. A Strategic Framework for Customer Relationship Management. Journal of Marketing 69: 167–76. [ Google Scholar ] [ CrossRef ]
- Payne, Adrian, and Pennie Frow. 2013. Strategic Customer Management: Integrating Relationship Marketing and CRM . Cambridge: Cambridge University Press. [ Google Scholar ]
- Rababah, Khalid, Haslina Mohd, and Huda Ibrahim. 2011. Customer relationship management (CRM) processes from theory to practice: The pre-implementation plan of CRM system. International Journal of e-Education, e-Business, e-Management and e-Learning 1: 22–27. [ Google Scholar ]
- Rahman, Muhammad Sabbir, Surajit Bag, Shivam Gupta, and Uthayasankar Sivarajah. 2023. Technology readiness of B2B firms and AI-based customer relationship management capability for enhancing social sustainability performance. Journal of Business Research 156: 113525. [ Google Scholar ] [ CrossRef ]
- Reichheld, F. Frederick, and Earl Sasser. 1990. Zero Defections: Quality Comes to Services. Harvard Business Review 68: 105–11. [ Google Scholar ]
- Romelaer, Pierre. 2005. L’entretien De Recherche. In Management Des Ressources Humaines: Méthodes De Recherche En Sciences Humaines Et Sociales . Edited by P. Dans Roussel and F. Wacheux. Paris: De Boeck, pp. 101–37. [ Google Scholar ]
- Sheth, Jagdish N., and Atul Parvatiyar. 1995. The evolution of relationship marketing. International Business Review 4: 397–418. [ Google Scholar ] [ CrossRef ]
- Sinha, Dr Gaurav. 2020. Customer Performance Management: The Following Trends in Customer Management? International Journal of Management 11: 1125–34. [ Google Scholar ]
- Stone, Merlin, Eleni Aravopoulou, Yuksel Ekinci, Geraint Evans, Matt Hobbs, Ashraf Labib, Paul Laughlin, Jon Machtynger, and Liz Machtynger. 2020. Artificial intelligence (AI) in strategic marketing decision-making: A research agenda. The Bottom Line 33: 183–200. [ Google Scholar ] [ CrossRef ]
- Viana, Julio A., Eric Scisly, and Olaf Reinhold. 2022. Ethical Issues in Social CRM: A Literature Review. Paper presented at the 2022 IEEE/WIC/ACM International Joint Conference on Web Intelligence and Intelligent Agent Technology (WI-IAT), Niagara Falls, ON, Candana, November 17–20; pp. 511–18. [ Google Scholar ] [ CrossRef ]
- Wolf, Christine T. 2020. AI Ethics and Customer Care: Some Considerations from the Case of “Intelligent Sales”. Proceedings of the 18th European Conference on Computer-Supported Cooperative Work, IBM Research–Almaden . Available online: https://dl.eusset.eu/server/api/core/bitstreams/c8c0e854-8095-4736-8b57-57fc640b5aa5/content (accessed on 16 February 2024).
- Yeboah-Boateng, Ezer Osei, and Stephane Nwolley. 2022. Developing Use Cases for Big Data Analytics: Data Integration with Social Media Metrics. In Handbook on ICT in Developing Countries . Nordjylland: River Publishers, pp. 171–99. [ Google Scholar ]
- Zaki, Mohamed. 2019. Digital transformation: Harnessing digital technologies for the next generation of services. Journal of Services Marketing 33: 429–35. [ Google Scholar ] [ CrossRef ]
Click here to enlarge figure
The statements, opinions and data contained in all publications are solely those of the individual author(s) and contributor(s) and not of MDPI and/or the editor(s). MDPI and/or the editor(s) disclaim responsibility for any injury to people or property resulting from any ideas, methods, instructions or products referred to in the content. |
Share and Cite
Khneyzer, C.; Boustany, Z.; Dagher, J. AI-Driven Chatbots in CRM: Economic and Managerial Implications across Industries. Adm. Sci. 2024 , 14 , 182. https://doi.org/10.3390/admsci14080182
Khneyzer C, Boustany Z, Dagher J. AI-Driven Chatbots in CRM: Economic and Managerial Implications across Industries. Administrative Sciences . 2024; 14(8):182. https://doi.org/10.3390/admsci14080182
Khneyzer, Chadi, Zaher Boustany, and Jean Dagher. 2024. "AI-Driven Chatbots in CRM: Economic and Managerial Implications across Industries" Administrative Sciences 14, no. 8: 182. https://doi.org/10.3390/admsci14080182
Article Metrics
Further information, mdpi initiatives, follow mdpi.
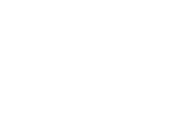
Subscribe to receive issue release notifications and newsletters from MDPI journals
A Study on Chatbots and Virtual Assistants in Customer Engagement: A Review
- International Journal of Engineering and Management Research 14(1):204-208
- 14(1):204-208

- K. S. Rangasamy College of Technology
Discover the world's research
- 25+ million members
- 160+ million publication pages
- 2.3+ billion citations
- Alfredo Daza Vergaray
- Wilfredo Fabriccio Peralta Robles
- Jahaira Arely Salazar Jiménez

- KNOWL-BASED SYST

- J MARKETING
- Katherine N. Lemon

- Recruit researchers
- Join for free
- Login Email Tip: Most researchers use their institutional email address as their ResearchGate login Password Forgot password? Keep me logged in Log in or Continue with Google Welcome back! Please log in. Email · Hint Tip: Most researchers use their institutional email address as their ResearchGate login Password Forgot password? Keep me logged in Log in or Continue with Google No account? Sign up

IMAGES
COMMENTS
In education, a virtual assistant can help organising the study process, manage time, increasing motivation and engagement in the study process. This paper performs a sys- tematic literature review of the use of virtual assistants in higher education. It focuses on the technology that powers them, their features and their impact in the learning ...
The current study presents a systematic literature review designed to provide a comprehensive overview of the IPA research area, summarizing the technology regarding IPA and identifying promising directions, without in-depth analysis and synthesis. ... A model to measure QoE for virtual personal assistant. Multimedia Tools and Applications, 76 ...
To guide this research, a review literature method was applied based on framework on literature review proposed by [27, 28], which sets out the stages to be followed such as: (1) identify the research questions; (2) Identify relevant studies; (3) Study selection; (4) Charting the data. 3.1 Identify the Research Questions
Information and Communication Technologies are driving the improvement of industrial processes. According to the Industry 4.0 (I4.0) paradigm, digital systems provide real-time information to humans and machines, increasing flexibility and efficiency in production environments. Based on the I4.0 Design Principles concept, Virtual Assistants can play a vital role in processing production data ...
Intelligent virtual assistants such as Amazon Alexa, Siri, and Google Assistant are mainly used to request information or play music. ... This study conducts a systematic literature review (SLR ...
In the last years, Intelligent Virtual Assistants such as Amazon Alexa or Google Assistant have gained great relevance in the market. This traditionally occurs mainly in personal and home assistance; however, in recent years, several solutions have been developed and used by teachers and students in the educational environment [].A virtual assistant is a conversational agent that can execute ...
During the literature review, ... This systematic review paper explored Virtual Assistant applications in the context of I4.0, discussing the Technical Assistance Design Principle and identifying ...
Therefore, it becomes highly crucial to review the performance of chatbots and virtual assistants. This paper aims to review the literature by eminent researchers in the form of authors, keywords and major contributing organizations.,Systematic literature review and bibliometric analysis is used to analyze the growth of literature on chatbots.
Though AI-powered virtual assistants (AIVAs) have gained traction among service providers, these are still lagging on the demand front. This study intends to develop an 'AIVA adoption model' delineated under a holistic framework based on structural equation modelling and deep neural network incorporating multilayer perceptron algorithm.
A systematic literature review of the use of virtual assistants in higher education focuses on the technology that powers them, their features and their impact in the learning process, motivation and productivity, according to this paper. A problem of students' motivation, engagement and declining interest in the learning process has always existed, contributing to increasing failures and ...
This systematic review paper explored Virtual Assistant applications in the context of I4.0, discussing the Technical Assistance Design Principle and identifying the characteristics, services, and ...
Virtual assistants (VAs) have gained widespread popularity across a wide range of applications, and the integration of Large Language Models (LLMs), such as ChatGPT, has opened up new possibilities for developing even more sophisticated VAs. However, this integration poses new ethical issues and challenges that must be carefully considered, particularly as these systems are increasingly used ...
Strengths and Limitations. This scoping review is the most rigorous attempt at synthesizing the literature regarding the effects of design characteristics on the user experience of virtual health assistants. It followed the PRISMA-ScR guidelines for scoping reviews and searched a large number of databases.
This research is based on a literature review around several topics including the definition and current scope of VTAs, the emerging market space and potential opportunities of virtual tutor-related products, application and research of VTAs in an intelligent classroom, exploration of the future direction of VTAs, and policy and ethical challenges.
The application of Virtual Assistants (VAs) is growing fast in our personal and professional life. It has been predicted that 25% of households using a VA will have two or more devices by 2021 . A virtual assistant is an intelligent application that can perform tasks or provide services for a person responding to orders or inquiries.
3 Literature Review. A computer primarily based approach for performing a comm and via a voice consumer. ... Virtual assistants are a boon for everyone in this new era of the 21st century. It has ...
Gubareva R and Lopes RP. Virtual assistants for learning: a systematic literature review. In: CSEDU (1); 2020, pp. 97-103. Bérubé C, Schachner T, Keller R, Fleisch E, Wangenheim F, Barata F, Kowatsch T. Voice-based conversational agents for the prevention and management of chronic and mental health conditions: systematic literature review.
A literature review on virtual assistant for visually impaired . ... This literature review analyses the problems that are being faced by visually impaired people and seek to find an affordable, efficient, feasible, and reliable solution for them by trying to convert the visual world into an audio world and make the visually impaired feel ...
A virtual assistant capable of being accessed via web chat is sometimes called a "chatbot." ... Tanmay Bore, Rohit Bhatt, Rajat Singh,"A Literature Review on Smart Assistant" International ...
Virtual Assistants for Learning: A Systematic Literature Review: The motivation, commitment and decreasing interest of students in the learning process has always existed, contributing to increased failures and dropouts. This can be attributed caused due to the difficulties with time management.
This paper presents a new approach for smart search. Overall, in world there are many people who use assistant. The paper presents applications of virtual assistant and also describes provocation of applying virtual assistant technology. Keywords: Voice Assistance, Python, AI, Review, Text to Speech, Speech to Text, Voice Recognition. I.
This work is a systematic review of the literature on Voice assistants (VA). Innovative mode of interaction, the Voice Assistant definition is derived from advances in artificial intelligence ...
The article begins with an introduction that outlines the background and significance of the study, highlighting the growing role of AI-driven chatbots in customer relationship management (CRM). This is followed by a literature review that provides an overview of existing research on AI in CRM, with a specific focus on AI-driven chatbots.
This paper presents a comprehensive review of. the literature on this topic, aiming to provide insights into the. impact of chatbots and virtual assistants on customer. experience, satisfaction ...